- 1Department of Population Health Sciences, University of Wisconsin-Madison, Madison, WI, United States
- 2Department of Consumer Science, University of Wisconsin-Madison, Madison, WI, United States
- 3University of Michigan, Ann Arbor, MI, United States
Relationships between debt and poor health are worrisome as access to expensive credit expands and population health worsens along certain metrics. We focus on payday lenders as one type of expensive credit and investigate the spatial relationships between lender storefronts and premature mortality rates. We combine causes of death data from the Centers for Disease Control and Prevention (CDC) and payday lender locations at the county-level in the United States between 2000 and 2017. After accounting for county socioeconomic and demographic characteristics, the local presence of payday lenders is associated with an increased incidence risk of all-cause and specific-cause premature mortality. State regulations may attenuate these relationships, which provides insights on policy strategies to mitigate health impacts.
Introduction
Adverse health conditions such as hypertension, cardiovascular disease, and declines in mental health are associated with debt burdens from ubiquitous access to expensive credit (1–5). Such health concerns are worrisome amidst rising debt (6) and recent deterioration in U.S. life expectancies. While overall premature mortality rates have been declining over the past two decades, since 2014, premature deaths have increased for some groups primarily due to drug overdoses and suicides (7–9). Although debt burdens have been shown to contribute to poor health (2, 5) and even premature death (1, 10, 11), the mechanisms by which communities' increasing access to expensive credit impact mortality are not well understood.
One trend in the availability of expensive credit is the expansion of higher-cost financial services like payday lenders in communities across the United States. The number of these storefront locations has increased nationwide since the mid-1990s (12–16), and the debt that borrowers accumulate from these higher-cost lenders contribute to their financial difficulties such as struggling to pay bills and delaying routine medical care (12, 13). This debt may also have effects in the aggregate, such as by contributing to communities' economic distress and worsened health outcomes regardless of whether any particular resident has borrowed expensive debt. One obstacle to identifying and testing these mechanisms is limited data on the extent of communities' financial services, making it difficult to associate communities' access to expensive credit with residents' health and premature mortality. A broad literature explores the spatial nature of business locational decisions such as fast food restaurants (17, 18), blood and plasma donation clinics (19, 20), and dollar stores (21, 22) and their associations to community economic distress with implications for public health. However, similar investigations of higher-cost lenders are limited.
In the current study, we investigate whether the presence of payday lenders is associated with premature mortality and hypothesize two mechanisms for explaining these relationships: residents living in areas with a higher number of payday lenders accumulate more higher-cost debt, and a higher density of payday lenders indicates areas' economic distress. We combine novel data including causes of death from the Centers for Disease Control and Prevention (CDC) WONDER database and the locations of payday lenders from InfoGroup USA at the county level between 2000 and 2017. Results indicate that the concentration of payday lenders may matter; though, the associations are conditional on the regulatory environment that informs payday lending practices. Importantly, we find evidence that state regulations can attenuate these relationships, especially for counties with high concentrations of payday lenders. Our findings offer new vantage points regarding the impacts of payday lending regulation. Beyond reducing financial difficulties related to paying bills, affording rent, and filing for bankruptcy that have been a focus of existing research (12–16), we suggest that regulating higher-cost financial services might advance community public health and protect against premature mortality for some groups.
The rise of consumer debt
The use and accumulation of consumer debt are often considered indicators of a person's access to financial services and their integration into the economy (23–26). Consumer debt is increasingly required to subsidize the costs of participating in today's economy (27), which is characterized by reduced collective bargaining power (28–31), low and stagnant wages (32, 33), and widening inequality (34, 35). People rely on debt to cope with these economic trends, as indicated by steadily rising debt burdens from medical expenses, student loans, credit cards, and payday and installment loans (6). Consumer debt increased in the years following the Great Recession and reached $14 trillion in 2019 (6).
Consumer debt is also an area of stratification, where heterogeneity in the quality and cost of certain types of debt may indicate exploitation or exclusion from the economy as opposed to integration (24). Debt from higher-cost, lower quality or “alternative” financial services—such as payday and installment lenders, auto title lenders, and tax refund and anticipation lenders—is expanding, growing by about 6% each year and reaching $141 billion in 2016 (36). The alternative financial services industry has expanded with the advent of online lending, and payday loans in particular comprised $14 billion of all online lending in 2016 (26–38). State regulations that restrict or prohibit certain usurious financial services appear to effectively constrain online lenders from crossing physical geographic boundaries (39–41). As such, increases in online lending may indicate a reinforcement of the industry's spatial ties to economically distressed communities where these lenders' storefronts are disproportionately located, and allude to concerning trends in the rise of consumer debt (16, 23).
The existing literature on debt typically focuses on individual borrowing behavior (see Borck et al. (42), O'Neill et al. (43), and Simone and Walks (44) for exceptions). This includes people's borrowing from the alternative financial services industry (45–48) and the potential consequences to their finances and health (1–5, 12, 13, 49–51). Yet the rising debt burdens of individuals (5) may also accumulate to produce effects that are observable at ecological or community levels, particularly given the extent to which lending and borrowing are spatially arranged (16, 52). For instance, people are more likely to borrow, and to borrow more often, when they live in areas with an increasing concentration of alternative financial services storefronts such as payday lenders (53).
The payday loan is a specific type of higher-cost credit product among the suite of alternative financial services. Payday loans have finance fees and an average annual interest rate of about 400%, which often prevent borrowers from repaying their original loans in full. Fifteen percent of borrowers renew their loans more than 10 times (47, 52). People who borrow payday loans are often younger, between the ages of 25 and 44, and have lower levels of education and income (46, 47). There is evidence of structural racism in borrowing (54), which contributes to Black Americans being more likely to borrow relative to White Americans, all else equal (46, 55). Borrowers of higher-cost debt report using payday loans to afford routine or recurring expenses (47), and experience financial difficulties related to paying bills, affording rent, filing for bankruptcy, and receiving routine medical care (12, 13, 49–51).
The locations of payday lender storefronts
A set of mutually reinforcing policies and practices have created spatial arrangements whereby higher-cost, lower-quality financial services are expanding and disproportionately locating in economically distressed and racially marginalized communities (15, 16, 56–64). Examining the locations of payday lenders in Colorado in 2007, a year when the state passed new legislation regulating payday loans, Gallmeyer and Roberts found that payday lender storefronts were disproportionately concentrated in census block groups with lower median incomes and higher poverty rates (62). Alternative financial services concentrate in White communities that are poor and economically distressed; though, unlike in predominantly White communities, these lenders' presence remains constant in Black communities regardless of economic indicators like income and poverty (16). Black and Latino communities have nearly twice the number of alternative financial services than do White communities (58, 60)—disparities that are amplified by segregation (16). Notably, the places where payday lenders concentrate could be the same places abandoned by other resources such as grocery stores and hospitals, making residents more susceptible to health-related concerns. In other words, a higher concentration of payday lenders could dissuade the types of development activities that have the potential to improve public health outcomes and enable economic distress, although these potential connections have yet to be evaluated.
The alternative financial services industry's expansion, and growth in payday lender storefronts in particular, has happened more rapidly in some years and in some communities than in others. For example, the number of alternative financial services storefronts increased nearly five-fold nationally between the mid-1980s and -1990s (15), before continuing to grow at an annual rate of 15% (36, 59). The notable growth in storefronts experienced by some communities coincided with the Great Recession in the mid- to late-2000s and the continued rise in consumer debt (58, 63, 64). Check cashers in New York City capitalized on the foreclosure crisis by opening new storefronts in Black and Latino communities between 2006 and 2011 (58). In California, Michigan, Ohio, and Tennessee, the number of new payday lender storefronts peaked between approximately 2006 and 2008, before leveling off in some places (64–68). Michigan's payday lender storefronts initially concentrated their expansion within the state's most populous counties during the early 2000s. Lenders deepened their presence and broadened to other counties across the state after 2005, with notable increases in counties' storefront densities occurring in 2009 and 2013 (67).
State regulation plays a role in where payday lenders are located. Given concerns that payday loans trap borrowers in cycles of debt and worsen their financial difficulties (12, 13, 15), some states have moved to regulate the industry in order to protect their residents. Payday lending densities tend to be lower in states that have strong regulations, including interest rate caps, whereas densities are relatively higher in states with permissive regulations (69). Six states and the District of Columbia currently prohibit payday lending of any kind, while 21 states do not regulate payday lending at all. In the remainder of states, regulation varies between permissive and restrictive with more restrictive regulations capping annual interest rates, preventing rollover or repeat borrowing, and assessing borrowers' ability to repay loans (70–72).
Theoretical mechanisms linking payday lenders to mortality
There are several hypothesized mechanisms through which access to the higher-cost, lower-quality debt made available by the alternative financial services industry may influence premature mortality. One mechanism may operate at the individual level through accumulated debt. Individuals living in communities with higher concentrations of payday lenders tend to use these services at higher rates (12, 53, 73), contributing to their accumulated debt burdens and financial difficulties (13, 48, 50, 51, 74). For example, Friedline and Kepple find that individuals' increased use of alternative financial services is associated with more dense concentrations of higher-cost storefronts in their communities (53). In other words, residents who live in communities with higher concentrations of payday lenders may accumulate more debt. Among people who borrow, the financial burdens of their debt, which is an enduring source of stress that can compound over the life course (4), may place strains on their health and contribute to premature mortality. Higher-cost, lower-quality debt is associated with a range of health effects with implications for premature mortality including weight gain, depression, and suicide (2, 4, 5, 75, 76). Individuals who have accumulated debt such as from payday lenders are more likely to experience negative health consequences, including cardiovascular disease and premature mortality (1–5). In examining debt as a mediator of physical health disparities, Batomen and colleagues find that individuals with the highest amounts of unsecured debt, such as that from payday lenders, experienced an increased risk of premature death due to hypertension and cardiovascular disease, compared to their counterparts with the lowest amounts of unsecured debt (1). Taken together, these findings suggest that the presence of higher-cost, lower-quality alternative financial services like payday lenders in a person's community, as well as the debts that borrowers accumulate from these services, could contribute to rates of premature death.
Another explanatory mechanism may operate as an emergent effect (53, 77–81) and affect all residents in a community regardless of whether or not they borrow payday loans. From one perspective, the presence and or concentration of alternative financial services within a community may be a proxy for economic distress. Residents' longevity may be compromised by the extent to which the presence and or concentration of payday lenders indicate communities' economic marginalization and distress. In Toronto, Canada, a neighborhood's higher density of check cashing storefronts, which served as a proxy for economic distress, was associated with residents' increased risk of premature death (81). In a longitudinal study examining associations between county-level economic distress as indicated by unemployment rates and subprime credit ratings and mortality rates, counties that experienced the greatest distress in 2000 and 2010 had significantly higher baseline mortality rates and rates of increase (79).
Economic distress may also be causally linked to premature mortality. From this perspective, the presence and or concentration of alternative financial services is not simply a proxy for economic distress. An increase in the concentration of payday lenders may subsequently increase a community's economic distress and therefore drive up premature mortality (82–84). Existing research implies a potential causal relationship between economic distress and premature mortality (79–81) and suggests that the concentration of alternative financial services influences community economic distress (62, 78), even if these relationships are not tested directly.
Prior ecological research finds supportive evidence for effects to emerge at the community level (79, 85–87). Higher concentrations of nuisance establishments like bars and alcohol outlets that often indicate economic marginalization and distress are associated with higher rates of child abuse and neglect, a relationship hypothesized to operate through community-level mechanisms (88–91). Relationships between the spatial arrangements of marijuana dispensaries and communities' crime rates are also hypothesized to operate through community-level mechanisms (92–94). Similar relationships exist between communities' payday lender storefronts and crime rates (80, 95). Along these lines, it is plausible that individuals' increased payday loan debts contribute to premature mortality, and that lenders' presence impacts premature mortality rates vis-à-vis economic marginalization and distress.
The current study
Using national county level data between 2000 and 2017, we examine how changes over time in the concentration of payday lender storefronts are associated with all-cause premature mortality. Among middle-aged Americans, ages 25–64, all-cause mortality rates were declining in 2000, plateaued by 2010, and began to increase after 2010 (8). These trends were especially pronounced from 2010 to 2017 when age-adjusted mortality rates increased by 6% primarily due to a substantial increase in drug overdoses, suicides, and alcoholic liver disease (8). Since most of these premature deaths are highly preventable, it is imperative to identify factors that exacerbate these deaths (81). Our analysis sheds light on two potential mechanisms that may lead to preventable premature deaths. We hypothesize that residents living in counties with higher concentrations of payday lenders have debt burdens that place strains on their health. We also hypothesize that payday lenders themselves may be a proxy of, and potential contributor to, community economic distress, which may worsen community public health outcomes. Our study cannot fully disentangle these mechanisms, but evidence of associations between payday lender presence and premature mortality will offer new pathways for scholarship on debt, access to financial services, and health. Further, a national perspective enables an evaluation of how state-level regulatory environments may impact the relationship between payday lender presence and premature deaths. For example, strong regulations that improve the affordability of payday loan products, such as capped interest rates and fees, limits on loan rollovers, or extensions of time to repayment, may subsequently attenuate any positive relationship.
Data
We combine data from several sources to develop a novel dataset for this study. First, we obtain historical data on payday lender storefront locations in the United States between 2000 and 2017 from InfoGroup. These data include the address, business name, and annual operating status for every payday lending storefront in the United States. We generate an annual file of active payday lenders using Standard Industry Classification business codes and word searches within company names (e.g., “cash advance”, “payday”). We then match geocoded business addresses to county boundaries to generate a county-level data file that captures the number of active storefronts in each county and each year. We then bring in data on premature mortality at the county level using data from the Center for Disease Control and Prevention's (CDC) WONDER database (96). We also include county-level socio-economic and demographic information using data from the Census and American Community Survey (97). Finally, we include annual data on state-level payday lending regulations from the National Conference of State Legislatures (72).
Key measures
Our outcome of interest is derived from the count of premature deaths in each county in each year. We define premature deaths as deaths from any cause among 20–59 year-olds, following the approach used by Matheson et al. (81). The CDC suppresses mortality counts between 0 and 9 and considers rates that use counts below 20 deaths to be unreliable. As such, we restrict our analytic sample to counties that have 20 or more premature deaths in a given year. Of the 3,134 eligible counties in the United States, 2,626 meet this criteria for at least 1 year between 2000 and 2017. In secondary models, we also evaluate cause-specific premature deaths for cardiac-, mental health- and assault-related deaths1. These models use subsets of counties that have non-suppressed counts of these deaths and seek to provide additional insights on possible individual- and community-level mechanisms linking payday lender presence to premature deaths.
Our key variable of interest is a three-level categorical measure of payday lender presence. The reference group is 0 lenders within a county, which we compare to counties that have 1–3 payday lenders, and those that have 4 or more. We base these categories on the average numbers of alternative financial services storefronts found in previous research (16, 60)2.
A second variable of interest is a constructed measure of regulatory strength. This measure refers to the strength of each state's payday lending regulatory environment in a given year. We use a four category measure. The reference group is states with no regulations, which is compared to states with weak regulations, moderate regulations, and strong regulations. A weak regulatory environment is defined as one where the state has a law on the books requiring payday lending licensing and registration. A moderate regulatory environment refers to those that limit rollovers or require lower interest rates. A strong regulatory environment refers to states that have fully prohibited payday lending or have strict interest rate caps set to 36% APR. For our analysis, we include all states, including those that prohibit payday lending. Supplemental models using only states that allow payday lenders produce similar results.
We include several time-varying control variables to better isolate the relationship between local payday lending environments and mortality outcomes. We include continuous measures of the county's share of poverty, share of male residents, share of Black and Latino residents, share of new residents moving into the county in the prior year, and share of urban residents in a given year. Covariates for race, sex assigned at birth, poverty, and urbanicity are standard controls included in analyses on payday lending [see, e.g., Faber (16)]. We additionally include the measure of county residential mobility as a proxy for duration of exposure to the county environment.
Variation in the population at risk of premature death across counties is accounted for using the population of individuals ages 20–59 as an exposure term in our models, which converts our count of premature deaths to a rate. All measures come from the Census and the American Community Survey (ACS) (97). We create annual measures for years 2008–2017 using the five-year ACS data with the year of interest as the midpoint. For years 2001 - 2007, we use linear interpolation between the 2000 Census and the 2006–2010 ACS (where 2008 is the midpoint) to generate annual estimates.
Table 1 presents descriptive statistics for all variables used in analysis. The premature mortality count for a typical county is 191 but with substantial variation in these counts, which range from 20 in some counties to well over 5,000 annually in large urban counties like Cook County, IL and Los Angeles County, CA. In our data, 38% of county-years have no payday lenders, about 27% have one to three payday lenders, and about 35% have four or more. In this latter category, it is not uncommon for a county to have numerous lenders in a given year; close to 37% of counties in this category (13% of the overall sample) have 10 or more lenders in at least 1 year. Regulatory environments are also mixed; for example, 47% of county observations are in weak state regulatory environments and 24% are in strong state regulatory environments.
Empirical strategy
We first present descriptive associations between the local payday lending environment and premature deaths. Because our outcome of interest is a count variable across space and time, we next fit longitudinal Poisson regressions with random effects (98). Inclusion of the exposure term, population aged 20–59, adjusts the model results to reflect incidence risk of premature deaths at the county level (i.e., converts the count to a rate). We report incidence risk ratios3, and standard errors are clustered at the county level in all models. All models include state and year fixed effects to account for omitted variables that vary by state and year.
We proceed in two stages. First, we model the association between payday lender presence and premature death. In this analysis, results from our first Poisson regression (M1) provide estimates from a model of the change in incidence risk of premature deaths as a function of lender presence, net of state and year fixed effects. M2 adds our set of time-varying county-level controls. M3 presents results from a model that interacts payday lender presence with all covariates. We include an interacted model to underscore that associations between payday lender presence and premature death rates may be conditional on other community characteristics, given known demographic and socio-economic disparities in both premature deaths and payday lender locations.
Second, we evaluate how payday lending regulation moderates the relationship between lender density and premature deaths, first with a model interacting lender presence and regulatory strength net of state and year fixed effects (M4), and then adding the set of time varying controls (M5). This set of models provides insights on whether and how regulation of high-cost lending may reduce premature mortality. For ease of interpretation, we do not interact these models with the demographic and socio-economic covariates.
Limitations
Our analysis is not without limitations. Data limitations include suppression of counties with < 20 mortality counts. Main models are not spatially weighted to account for geographic clustering of premature death and payday lender counts due to modeling limitations (see Appendix A for discussion). Although we hypothesized individual- and community-level mechanisms to explain higher-cost lenders' effects on premature death, data limitations make direct tests of these mechanisms suggestive. Future research should attempt to elucidate these explanatory mechanisms, particularly how and the extent to which the concentration of payday lenders represents economic distress, encourages economic distress by dissuading other types of development activities, and contributes to poor public health outcomes. Moreover, payday lenders have not been present for very long by our first year of data, meaning that exposure to lenders could be limited and our data do not fully capture cumulative effects. Any associations that are suggestive of cumulative effects may be underestimated. Finally, we look at overall premature death counts and do not examine the effects of payday lender presence on premature deaths by subgroup (e.g., by sex assigned at birth and race/ethnicity), and future research should examine the potential heterogeneity in effects across subgroups.
Results
Descriptive maps of key variables
Figure 1 presents counts of premature deaths and counts of payday lenders for 2 years in our analysis, 2008 and 2011, using all available data from the CDC and InfoGroup. These years correspond with the start and end of the Great Recession, which impacted both premature death rates (99) and use of payday loans (100). The top panel, which features county-level counts of premature deaths from all causes, shows a consistent clustering of premature deaths in the South, with more counties experiencing premature counts in the 75th percentile or higher in 2011. It also shows that the majority of the suppressed CDC data is largely from rural counties in the central and western United States; these counties will be excluded from our analyses.
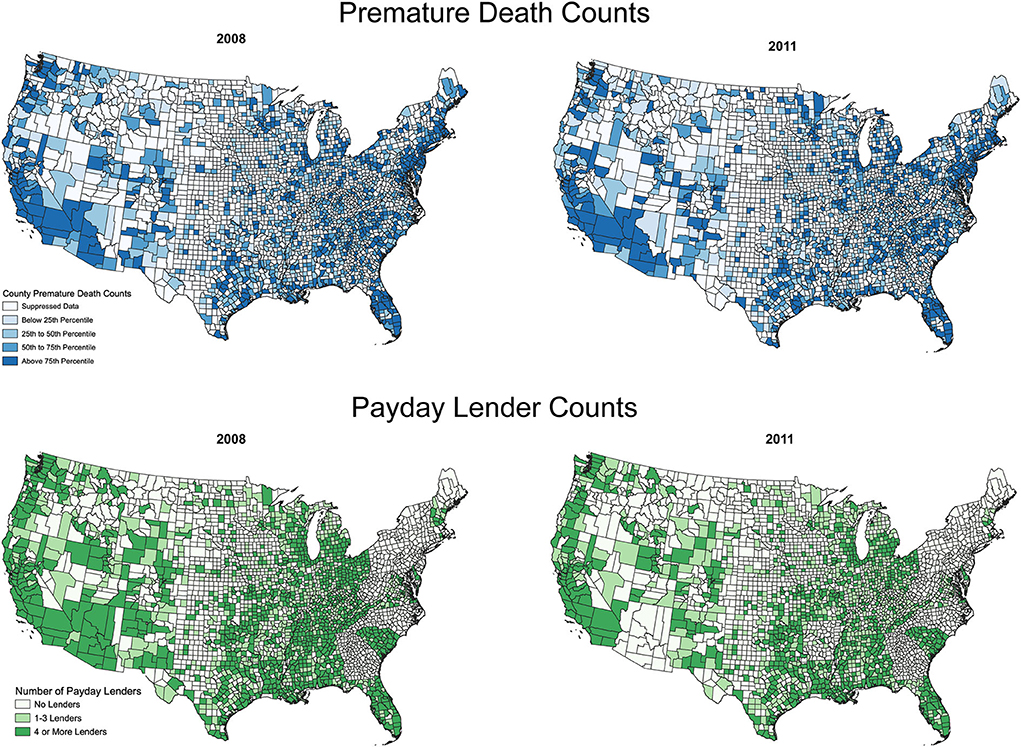
Figure 1. Counts of premature deaths and payday lenders over time (by county and year) for counties in the continental United States. Maps created using QGIS software, county boundaries reflect 2010 Census boundaries.
The bottom panel, which features counts of payday lenders, shows that the count of counties with four or more lenders (top categories) was strongest in 2008, with fewer counties reporting 4 or more payday lenders in 2011. Much of the decline by 2011 is likely due to regulatory interventions that several states enacted, rather than a decline in demand. Use of payday loans increased during the Great Recession (100), but payday lenders also faced increased oversight in several states, which has an impact on where they operate (101). Many of the counties that have suppressed CDC data also have few payday lenders (e.g., central United States). Beyond that, the two panels suggest positive associations between premature deaths and payday lenders; many of the counties with relatively high counts of premature deaths also have four or more payday lenders (see, e.g., parts of Texas and the Florida panhandle). These descriptive correlations also reflect other factors like urbanicity, which will be accounted for in our modeling.
Spatial autocorrelation of variables
Our models do not account for spatial clustering of premature deaths and payday lenders. Given the spatial clustering observed in Figure 1, we assessed the spatial autocorrelation of the dependent and independent variables for the inclusion of spatial weights in our models using Moran's I. There is spatial autocorrelation for our measures of payday lender counts and premature deaths in each year of our analysis (p < 0.001). In appendix A, we compare our main results to those from spatially weighted OLS models, which are less ideal for a count outcome but permit an evaluation of potential spatial spillover effects among neighboring counties. The spatially weighted models indicate that spatial autocorrelation does impact results; the models presented below are likely underestimating the effects of payday lender presence on premature mortality.
Association between payday lender presence and all-cause premature mortality
Table 2 presents the predicted incidence risk ratios adjusted for state and year fixed effects (M1) and incidence risk ratios that are also adjusted for controls (M2) and interactions with controls (M3). Full model results are available in Appendix B. Model 1, which includes no controls, shows a significant, positive association between counties with four or more lenders and the risk of premature deaths, with a 2% increase in risk of premature death over counties without lenders (RR = 1.020, p < 0.05). Counties with 1–3 lenders have little difference in risk of premature death compared to counties without lenders (RR = 1.009, p > 0.05). After adjusting for controls, M2 shows that the adjusted risk ratio continues to be significant and positive for counties with 4+ lenders compared to counties without lenders (RR = 1.021, p < 0.05). Appendix B also confirms that results for covariates in M2 have directions that are largely in line with expectations. For example, an increase in the share of urban residents is associated with a reduction in risk of premature mortality (p < 0.05), in line with prior research that shows that premature deaths are higher in rural areas (89). Further, an increase in the share of mobility in the county is significantly associated with a reduced risk of premature death; other covariates' coefficients have suggestive directions and most are significant (e.g., as the share of male residents increases, there is an association with a slightly elevated risk of premature death, in line with work showing differences in sex assigned at birth in premature deaths (p < 0.05).
When the model is interacted (M3), the main effects for counties with 1–3 lenders and 4 or more lenders are significant (p < 0.05), as are some interaction effects with county socio-economic and demographic covariates (see Appendix B). As shown in Table 2, counties with 1–3 lenders have a 2.3% increase in risk of premature mortality, and counties with 4 or more lenders have a 1.7% increase in risk, compared to counties without lenders (p < 0.05 for both). To put this in context, Figure 2 presents predicted premature mortality counts by county type. Counties with no lenders have an average predicted premature mortality count of 235.7 deaths when all covariates are at their means. Compared to counties without lenders, the presence of 1–3 lenders was associated with a predicted excess of 4.8 deaths and the presence of 4 or more lenders was associated with a predicted excess of 4.2 deaths4.
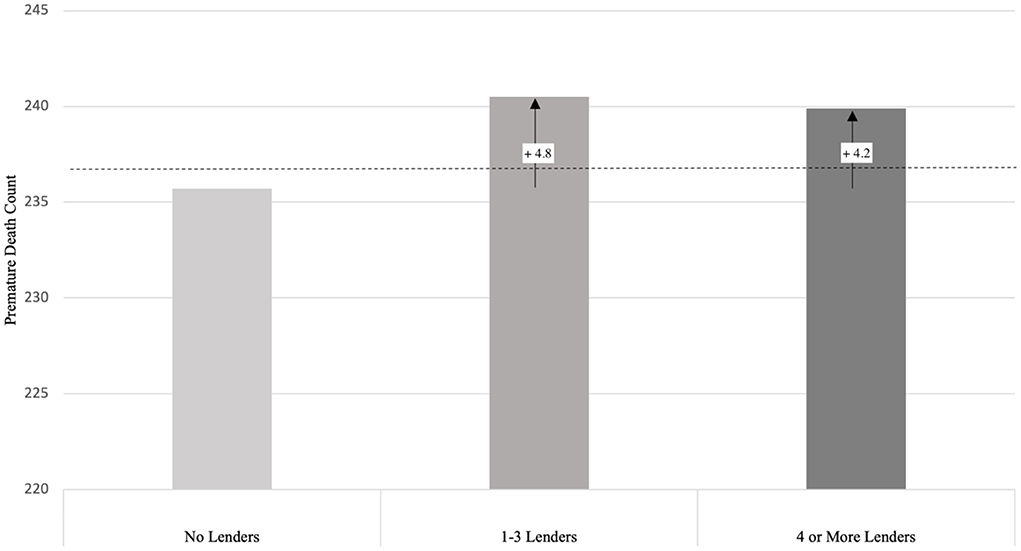
Figure 2. Predicted excess premature deaths in counties with lenders. The higher adjusted incidence risk ratios (p < 0.05) in M3 of Table 2 for counties with 1 to 3 and 4 or more lenders translate to 4.8 and 4.2 excess deaths, respectively, compared to counties with no lenders. Estimated death counts are derived from Model 3 in Appendix B using Stata margins and lincom commands. Dashed line represents overall grand mean (238.4 deaths), derived from same model. 2,626 counties contributed 42,230 observations.
Associations between payday lender presence and cause-specific premature mortality
Though data are more limited, we also evaluate three cause-specific rates of premature mortality, evaluating deaths that stem from mental and behavioral health disorders, cardiac issues, and assaults. Deaths due to mental health (e.g., suicides) in communities with higher-cost lenders may speak to more immediate individual-level mechanisms where stress related to increased debt burdens is linked to premature deaths, given known literature that finds debt can negatively impact mental health [e.g., Fowler et al. (60)]. Cardiac-related deaths may provide a longer-term view of the health consequences of the accumulation of personal debt over time [e.g., Batomen et al. (1), Eisenberg-Guyot et a. (2), Nelson et al. (3), Sweet et al. (4), and Fitch et al. (5)]. Deaths due to assault may speak to broader community-level factors, where the presence of payday lenders as an indicator of economic distress leads to higher risks of these deaths. We know from prior work that the presence of payday lenders has been linked to increased violent crime in local areas [e.g., Kubrin et al. (80)], and assault-related deaths may speak to the more immediate ecological impacts of payday lender presence.
The number of counties with non-suppressed information on these deaths is lower, and thus these analyses are more limited. We evaluate mental health and cardiac related deaths using a shared sample of 1,213 counties with 11,519 observations. We separately evaluate assault related deaths using an even smaller sample of 193 counties and 2,184 observations; this cause of death is rarer and few counties have sufficient counts of deaths to be included in analysis. For the analysis of assault related deaths, we reduce our measure of lender presence to two categories: no lenders, or any lenders: this is because the vast majority of counties with lenders have 4 or more locations (only five counties had one to three lenders). Models for these disaggregated analyses mirror Table 2 using the same covariates and interactions. The smaller sample sizes reduce the precision of estimates, and we caution that these results reflect a select set of counties that may not be nationally representative.
Results in Table 3A show strong evidence of a positive association between payday lender presence and mental-health related deaths. In the model for mental-health related deaths net of covariates (M2), having 1–3 lenders or 4 or more lenders is associated with a substantially higher risk of premature mortality compared to counties with no lenders (RR = 1.178, p < 0.01 and RR = 1.167, p < 0.05). When interacted with county covariates, the adjusted risks remain elevated, although with large confidence intervals (RR = 1.082, p > 0.05, and RR = 1.068, p > 0.05, respectively). In these same counties, we see more modest evidence of connections to cardiac-related deaths (M4 - M6). Having 1–3 lenders is positively associated with a higher risk of cardiac-related premature mortality compared to having no lenders; however, the results are only significant for those counties with 1–3 lenders in Model 5 (RR = 1.054, p < 0.05). Finally, as shown in Table 3B, in the more limited set of counties for premature deaths due to assaults (M3), there are positive associations between having any lenders in a county compared to no lenders, with magnitudes similar to that for the risk of mental-health related premature deaths (e.g., RR = 1.077, p > 0.05 in M3 of Table 3B).
Together, these results show initial support for both individual- and community-level mechanisms, with some indication that the public health impacts of payday lender presence may be more immediate as shown by the large magnitudes of the relative risks for mental health and assault related deaths in counties with lenders. The comparatively more modest associations between cardiac deaths and payday lender presence may be due to the fact that the full cumulative effects of local industry presence on public health have not been realized. In our analytic period, payday lending is relatively new; most storefronts started opening nationwide in the early 2000s, and connections to longer term health issues may not be known for some time. Additional analyses (not shown) of all-cause mortality that include a control for state-level credit card debt per capita find that this control has a significant, positive association with premature deaths; though, it does not meaningfully change the payday lender—premature death association. This further suggests that the mechanisms behind the relationship may extend beyond individual-level debt burdens. These analyses remain suggestive; more research is needed to fully understand mechanisms behind the association between communities' payday lender presence and premature deaths.
Impact of regulatory interventions on relationship of interest
We return to our main analysis of all-cause premature mortality and include an interaction between payday lender presence and regulatory strength to understand whether any relationship between lender presence and premature deaths may be dependent upon the regulatory environment. Table 4 presents results of this lender presence by regulation interaction with just state and year fixed effects (M1) and results from a model that also controls for other covariates (M2). These results indicate that the impacts of payday lender presence are conditional on the regulatory context. Full model results are available in Appendix C.
There are significant differences that are large in magnitude for premature death—payday lender associations across regulatory contexts. M2 shows that, relative to counties with no lenders in states without regulations, every other combination of regulatory environment and lender presence has a significantly higher risk of premature mortality. The only exception is among counties with 1–3 lenders in states without regulation, which remains marginally higher (RR = 1.003, p > 0.05). In weak and moderate regulatory environments, relative risks range between 1.07 and 1.11, or seven to 11% higher, compared to counties with no lenders and no regulations, while relative risks tend to be lower in strong regulatory environments (around 1.05 for each county type, or 5% higher). These patterns suggest that the variation in the degree of attenuation that regulation can achieve depends on its strength. For example, counties with 1–3 lenders move from a relative risk of 1.09 in a context of weak regulations to 1.11 in a context of moderate regulations; in other words, the risk of premature death actually increases by 2% when moving from weak to moderate regulations. However, the shift from moderate to strong regulations for these counties results in a reduction in risk by 6% (moving from 1.11 to 1.05). This suggests that strong regulations do comparatively better in dampening the risk of premature mortality compared to weak and moderate regulations, which actually experience increases in risk compared to even less regulation.
That these patterns are true irrespective of the number of lenders is somewhat puzzling. Similar patterns occur for counties that have no lenders across these regulatory contexts, and we would expect regulation to impact counties with lenders but have little effect on counties without. This might be due in part to compositional changes that occur when regulation takes effect. For example, when a state shifts from moderate to strong regulations, there are some counties where all lenders leave, as shown by prior work (101–103). When this occurs, the county would get reclassified as part of the “no lenders, strong regulations” group in our models. The average adjusted risk of 1.05 for this group might be picking up some of the higher initial risk of counties that had been in the 1–3 or 4+ lender categories under a moderate regulatory context. More research is needed to fully understand why regulation impacts the public health of counties without lenders.
Figure 3 displays estimated relative risks within regulatory context, derived from Model 2. This figure does not show increases in levels relative to one common reference group, as shown in Table 4, but rather compares across county lender categories within the same regulatory environment. In the absence of regulation, the relative risk of premature deaths is the highest for counties with four or more lenders (RR = 1.06, p < 0.001), and then differences between high concentration counties and those with zero and 1–3 lenders diminish as regulations strengthen. In moderately regulated environments, the risk for counties with four or more lenders is marginally lower relative to that of counties without lenders (RR = 0.97, p > 0.05). Relative risks return to parity under strong regulatory environments, all else equal. This within-context comparison underscores that the lack of regulation impacts public health in counties with high concentrations of lenders the most, and that these counties gain relatively greater public health benefits under contexts with enhanced regulation.
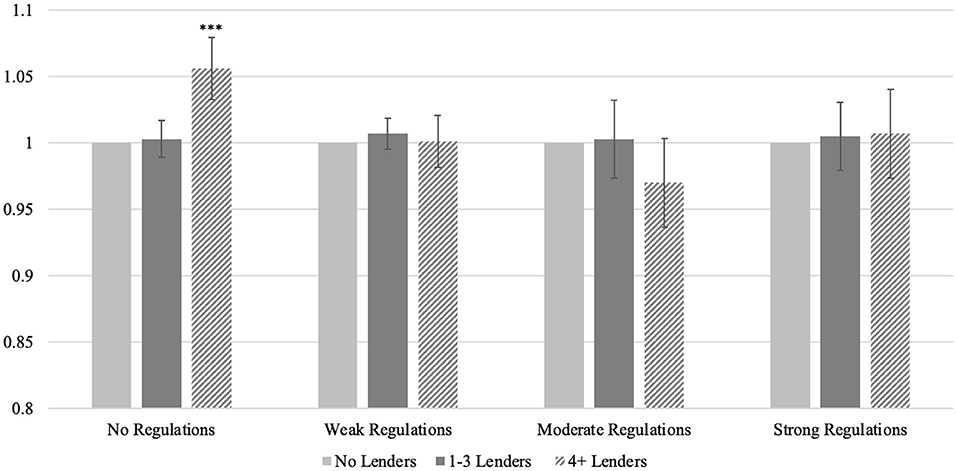
Figure 3. Within-regulatory group relative risks for premature deaths. Within-period incidence risk ratios relative to counties with no lenders (left-most column in each group); error bars reflect 95% confidence intervals. ***p < 0.001, compared to counties with no lenders. Exposure term is population ages 20–59; 2,626 counties contributed 42,230 observations. Derived from Model 2 in Appendix C using Stata margins and nlcom commands.
Concluding discussion
The relationship between high debt burdens and poor health raises concerns about the expansion of the payday lending industry, which sells expensive loans that are hard to repay (47, 52) and contribute to borrowers' financial difficulties (12, 13). Robust literatures explore the relationships between payday lenders and financial difficulties [e.g., (12, 77)] and high debt burdens and poor health [e.g., Batomen et al. (1) and Eisenberg-Guyot et al. (2)], laying the groundwork to connect these lines of inquiry. Importantly, as the number of these industry storefronts expanded, some states began trying to protect borrowers by placing new restrictions on payday lenders such as capping usurious interest rates and preventing the renewal or re-borrowing of these loans, which may have public health benefits. We explore these associations spatially at the county level and our findings, described below, offer new pathways for inquiries into payday lending and effective regulation.
Several key findings elucidate any relationships between the distribution of payday lenders and community health. First, we find that after accounting for socioeconomic covariates, the risk of all-cause premature death is significantly higher in counties with four or more payday lenders and one to three lenders, relative to counties without. Secondary analyses that disaggregate causes of premature deaths lend some support for both proposed mechanisms for this association. Higher risks of mental health related deaths and modestly higher risks of cardiac related deaths suggest that residents' longevity in these communities may be compromised by individual-level stress due to increased debt burdens. Higher risks of assault related deaths suggest that exposure to heightened community economic distress as proxied by payday lender presence may also compromise longevity. Because our analysis remains at the county level, it is not possible to fully disentangle community- and individual-level mechanisms; though, taken together, the all-cause and specific cause analyses underscore that payday lender presence is associated with poorer community health, even after accounting for community demographic composition, poverty, and urbanicity.
Second, we find evidence of moderating effects of regulation, whereby the influence of a county's concentration of payday lenders on the risk of all-cause premature death is conditional on regulatory context. These findings provide evidence that better regulations may have beneficial public health impacts in areas with a relatively large number of lenders. This modest attenuation lends some support to the notion that improved regulation may have positive spillover effects on community health. These findings are notable because they allude to the importance of broadening policy conversations on financial regulation to include the effects on social, physical, and mental well-being. Depending on the extent to which the effectiveness of regulation is evaluated in economic terms, current policy conversations may underestimate the economic benefits of regulation by focusing primarily on financial difficulties and well-being.
Regulation appears to matter even for counties without lenders. This puzzling finding could be explained by the extent to which regulations targeting payday lenders also discourage or supplant other types of storefronts and businesses that contribute to a county's economic marginalization and distress. Similar to regulation's positive spillover effects on community health, perhaps there are also spillover effects onto usurious and other predatory businesses that undermine community health even in absence of payday lenders. Future research should investigate this possibility.
In the United States, geographic inequalities in health and mortality are growing. The substantial spatial variation in mortality rates makes it important to understand the links between local built environments, policy contexts that inform those environments, and public health. For instance, the absence of grocery stores and hospitals from communities—forms of food and healthcare apartheid enabled by policy decisions and that often accompany other indicators of economic marginalization and distress—has implications for public health (104, 105). We provide evidence that the availability of expensive credit also matters, using geographic variation in the presence of payday lenders and connections to mortality. Understanding the contributions of payday and other high-cost lenders to mortality can aid in identifying potential underlying mechanisms and the possibility for regulation to attenuate their effects. We suggest that, in this context, regulation has the potential to protect against premature mortality for some groups. Future research will need to investigate these relationships in the years during and after the COVID-19 pandemic, which notably changed people's life expectancy and experiences with financial difficulties, as well as influenced business turnover and storefront locational decisions.
Data availability statement
The data analyzed in this study is subject to the following licenses/restrictions: The Center for Disease Control and Prevention's WONDER database is available for public use (https://wonder.cdc.gov/wonder/help/ucd.html). Annual data on state-level payday lending regulations from the National Conference of State Legislatures is available for public use (https://www.ncsl.org/research/financial-services-and-commerce/payday-lending-state-statutes.aspx). Historical data on payday lender storefront locations in the United States between 2000 and 2017 is available for purchase from InfoGroup or available through some university library subscriptions, such as the University of Michigan. Requests to access these datasets should be directed to https://www.data-axle.com/contact-us/?gclid=CjwKCAjw2rmWBhB4EiwAiJ0mtSffVEq4u6aa2W9P7ScizvyA-RQXNDJt-PpXLJoMQ9EXQHzAMNBPkRoC4kgQAvD_BwE#contact_us_location_3?utm_term=data%20axle%20headquarters&utm_campaign=Corporate+Brands+%7C+PR&utm_source=google&utm_medium=cpc&hsa_acc=9152831390&hsa_cam=13480869329&hsa_grp=123161790413&hsa_ad=529715951002&hsa_src=g&hsa_tgt=kwd-1307381766427&hsa_kw=data%20axle%20headquarters&hsa_mt=b&hsa_net=adwords&hsa_ver=3.
Author contributions
All authors listed have made a substantial, direct, and intellectual contribution to the work and approved it for publication.
Funding
MA's time for this research was supported by the T32 AG00129 grant, awarded to the Center for Demography of Health and Aging at the University of Wisconsin-Madison by the National Institute on Aging.
Conflict of interest
The authors declare that the research was conducted in the absence of any commercial or financial relationships that could be construed as a potential conflict of interest.
Publisher's note
All claims expressed in this article are solely those of the authors and do not necessarily represent those of their affiliated organizations, or those of the publisher, the editors and the reviewers. Any product that may be evaluated in this article, or claim that may be made by its manufacturer, is not guaranteed or endorsed by the publisher.
Supplementary material
The Supplementary Material for this article can be found online at: https://www.frontiersin.org/articles/10.3389/fpubh.2022.993585/full#supplementary-material
Footnotes
1. ^We use the CDC WONDER database to pull county-level counts of premature deaths due to mental health [IC10 codes - F01-F99 (mental and behavioral health disorders) and X60 - X84 (intentional self-harm)], cardiac [I10 - I51 (e.g., hypertension, heart disease)], and assault (X85-Y09 “assault”).
2. ^Adjusting the categories to evaluate counties with 0 lenders relative to counties with 1–3, 4–10, and 11 or more lenders produces substantively similar results.
3. ^With our exposure term, incidence risk is defined as the number of premature deaths divided by the population ages 20–59 (i.e., the population at risk of a premature death) for each county in each year.
4. ^Calculated using results from Model 3 in Appendix B, using the margins postestimation commands in Stata. We keep covariates at their means to approximate an average U.S. county during our analytic period.
References
1. Batomen B, Sweet E, Nandi A. Social inequalities, debt, and health in the United States. SSM Popul Health (2021) 13:100736. doi: 10.1016/j.ssmph.2021.100736
2. Eisenberg-Guyot J, Firth C, Klawitter M, Hajat A. From payday loans to pawnshops: Fringe banking, the unbanked, and health. Health Aff. (2018) 37:429–37. doi: 10.1377/hlthaff.2017.1219
3. Nelson MC, Lust K, Story M, Ehlinger E. Credit card debt, stress and key health risk behaviors among college students. Am J Health Promot. (2008) 22:400–6. doi: 10.4278/ajhp.22.6.400
4. Sweet E, Kuzawa CW, McDade TW. Short-term lending: Payday loans as risk factors for anxiety, inflammation and poor health. SSM - Popul Health. (2018) 5:114. doi: 10.1016/j.ssmph.2018.05.009
5. Fitch C, Hamilton S, Bassett P, Davey R. The relationship between personal debt and mental health: a systematic review. Mental Health Rev J. (2011) 16:153–66. doi: 10.1108/13619321111202313
6. Federal Reserve Bank of New York. Quarterly Report on Household Debt and Credit: 2019 Q3. New York: Federal Reserve Bank of New York, Research and Statistics Group 2019. Available online at: https://www.newyorkfed.org/microeconomics/hhdc.html
7. Ho JY, Hendi AS. Recent trends in life expectancy across high income countries: retrospective observational study. BMJ. (2018) 15:362:k2562. doi: 10.1136/bmj.k2562
8. Woolf SH, Schoomaker H. Life expectancy and mortality rates in the United States, 1959-2017. JAMA. (2019) 322:1996–2016. doi: 10.1001/jama.2019.16932
9. Shiels MS, Chernyavskiy P, Anderson WF, Best AF, Haozous EA, Hartge P, et al. Trends in premature mortality in the USA by sex, race, and ethnicity from 1999 to 2014: An analysis of death certificate data. Lancet. (2017) 389:1043–54. doi: 10.1016/S0140-6736(17)30187-3
10. Argys LM, Friedson AI, Pitts MM. Killer debt: The impact of debt on mortality. Federal Reserve Bank of Atlanta Working Paper Series. (2016). Available online at: https://www.atlantafed.org/-/media/documents/research/publications/wp/2016/14-killer-debt-the-impact-of-debt-on-mortality-2017-04-10.pdf
11. Clayton, M. Liñares-Zegarra J, Wilson JOS. Does debt affect health? Cross country evidence on the debt-health nexus. Social Sci Med. (2015) 130:51–8. doi: 10.1016/j.socscimed.2015.02.002
12. Melzer BT. The real costs of credit access: Evidence from the payday lending market. Q J Econ. (2011) 126:517–55. doi: 10.1093/qje/qjq009
13. Skiba PM, Tobacman J. Do payday loans cause bankruptcy? J Law Econ. (2019) 62:485–518. doi: 10.1086/706201
14. Apgar W, Herbert C. Subprime Lending and Alternative Financial Service Providers: a Literature Review and Empirical Analysis. Washington, DC: US Department of Housing and Urban Development. Available online at: http://www.huduser.gov/Publications/pdf/sublending.pdf
15. Caskey JP. Fringe Banking: Check-Cashing Outlets, Pawnshops, and the Poor. New York: Russell Sage Foundation (1994). 184 p.
16. Faber JW. Segregation and the cost of money: Race, poverty, and the prevalence of alternative financial institutions. Social Forces. (2019) 98:819–48. doi: 10.1093/sf/soy129
17. Shannon J. Food deserts: Governing obesity in the neoliberal city. Prog Hum Geogr. (2014) 38:248–66. doi: 10.1177/0309132513484378
18. Yenerall, J, You, W, Hill, J. Investigating the spatial dimension of food access. Int J Environ Res Public Health. (2017) 14:866. doi: 10.3390/ijerph14080866
19. Balentine MD. A Geographical Analysis of US Plasma Centers and Their Economic, Social, and Public Health Impacts [dissertation]. (2020). Greensboro, NC: The University of North Carolina at Greensboro.
20. Robert JC, Mustard CA. Geographic location of commercial plasma donation clinics in the United States, 1980-1995. Am J Public Health. (2004) 94:1224–9. doi: 10.2105/AJPH.94.7.1224
21. Shannon J. Dollar stores, retailer redlining, and the metropolitan geographies of precarious consumption. Ann Am Assoc Geogr. (2021) 111:1200–18. doi: 10.1080/24694452.2020.1775544
22. Sharkey JR, Horel S, Han D, Huber JC. Association between neighborhood need and spatial access to food stores and fast food restaurants in neighborhoods in Colonias. Int J Health Geogr. (2009) 8:1–17. doi: 10.1186/1476-072X-8-9
23. Charron-Chénier R. Predatory inclusion in consumer credit: Explaining black and white disparities in payday loan use. Sociol Forum. (2020) 35:370–92. doi: 10.1111/socf.12586
24. Charron-Chénier R, Seamster L. Racialized debts: Racial exclusion from credit tools and information networks. Crit Sociol. (2021) 47:977–92. doi: 10.1177/0896920519894635
25. Dwyer RE. Credit, debt, and inequality. Annu Rev Sociol. (2018) 44:237–61. doi: 10.1146/annurev-soc-060116-053420
26. Friedline T, Freeman A. The potential for savings accounts to protect young-adult households from unsecured debt in periods of macroeconomic stability and decline. Soc Serv Review. (2016) 90:83–129. doi: 10.1086/685791
27. Lin KH, Neely MT. Divested: Inequality in Financialized America. Oxford, UK: Oxford University Press. (2020). 240 p.
28. Antonczyk D, Fitzenberger B, Sommerfeld K. Rising wage inequality, the decline of collective bargaining, and the gender wage gap. Labour Econ. (2010) 17:835–47. doi: 10.1016/j.labeco.2010.04.008
29. Jacobs D, Myers L. Union strength, neoliberalism, and inequality: Contingent political analyses of U.S. income differences since 1950. Am Sociol Rev. (2014) 79:752–74. doi: 10.1177/0003122414536392
30. Wallerstein M, Western B. Unions in decline? What has changed and why. Annu Rev Polit Sci. (2000) 3:355–77. doi: 10.1146/annurev.polisci.3.1.355
31. Western B, Rosenfeld J. Unions, norms, and the rise in US wage inequality. Am Sociol Rev. (2011) 76:513–37. doi: 10.1177/0003122411414817
32. Autor DH, Katz LF, Kearney MS. Trends in US wage inequality: Revising the revisionists. Rev Econ Stat. (2008) 90:300–23. doi: 10.1162/rest.90.2.300
33. Mishel L, Bivens J, Gould E, Shierholz H. The State of Working America. 12th ed. New York: ILR Press (2012).
34. Kim C, Sakamoto A. The rise of intra-occupational wage inequality in the United States, 1983 to 2002. Am Sociol Rev. (2008) 73:129–57. doi: 10.1177/000312240807300107
35. Piketty T. Capital in the Twenty-First Century. Cambridge: Harvard University Press (2014). 816 p.
36. Schmall T, Wolkowitz E. 2016 Financially Underserved Market Size Study. Center for Financial Services Innovation. (2016) 54 p. Available online at: https://cfsi-innovation-files-2018.s3.amazonaws.com/wp-content/uploads/2017/02/27001759/2016-Financially-Underserved-Market-Size-Study_Center-for-Financial-Services-Innovation.pdf
37. Hecht J. The Industry, the Customer, the Experience: Online Consumer Lending Industry Trends and Observations. Miami: Online Lending Alliance Conference (2012).
38. Peterson A. Predatory Payday Lending. Washington, DC: Center for American Progress (2013). Available online at: https://www.americanprogress.org/article/predatory-payday-lending/
39. Brown T, Swartz M. Competition policy on consumer financial services: The disparate regulation of online marketplace lenders and bankers. Compet Policy Int. (2016) 11:38–45. Available online at: https://www.competitionpolicyinternational.com/wp-content/uploads/2016/03/Competition-Policy-in-Consumer.pdf
40. Nuñez S, Schaberg K, Hendra R, Servon L, Addo M, Mapillero-Colomina A. Online payday and installment loans: Who uses them and why? New York, NY: MDRC (2016). 134 p.
41. Wang H, Overby EM. How does online lending influence bankruptcy filings? Evidence from a natural experiment. SSRN Journal. (2017). Available online at: http://www.ssrn.com/abstract=2958916
42. Borck R, Fossen FM, Freier R, Martin T. Race to the debt trap?—Spatial econometric evidence on debt in German municipalities. Reg Sci Urban Econ. (2015) 53:20–37. doi: 10.1016/j.regsciurbeco.2015.04.003
43. O'Neill KK, Kennedy I, Harris A. Debtors' blocks: How monetary sanctions make between-neighborhood racial and economic inequalities worse. Sociol Race Ethnic. (2022) 8:43–61. doi: 10.1177/23326492211057817
44. Simone D, Walks A. Immigration, race, mortgage lending, and the geography of debt in Canada's global cities. Geoforum. (2019) 98:286–99. doi: 10.1016/j.geoforum.2017.10.006
45. Pew Charitable Trusts. Payday Loan Facts and the CFPB's Impact. Washington, DC: Pew Charitable Trusts (2016). 2 p.
46. Kindle PA, RB. Do we know what we think we know about payday loan borrowers? Evidence from the Survey of Consumer Finances. J Soc Soc Welfare. (2017) 44:19–44. Available online at: https://heinonline.org/HOL/LandingPage?handle=hein.journals/jrlsasw44&div=48&id=&page=
47. Pew Charitable Trusts. Payday Lending in America: Who Borrows, Where They Borrow, and Why. Washington, DC: Pew Charitable Trusts (2012). 48 p.
48. Gathergood J, Guttman-Kenney B, Hunt S. How do payday loans affect borrowers? Evidence from the UK market The Review of Financial Studies. (2018) 32:496–523. doi: 10.1093/rfs/hhy090
49. Bickham T, Lim Y. In sickness and in debt: Do mounting medical bills predict payday loan debt?. Soc Work Health Care. (2015) 54:518–31. doi: 10.1080/00981389.2015.1038410
50. Fitzpatrick K, Coleman-Jensen A. Food on the fringe: Food insecurity and the use of payday loans. Soc Serv Rev. (2014) 88:553–93. doi: 10.1086/679388
51. Morgan DP, Strain MR, Seblani I. How payday credit access affects overdrafts and other outcomes. J Money Credit Bank. (2012) 44:519–31. doi: 10.1111/j.1538-4616.2011.00499.x
52. Consumer Financial Protection Bureau. CFPB Data Point: Payday Lending. Washington, DC: CFPB Office of Research (2014). 33 p.
53. Friedline T, Kepple N. Does community access to alternative financial services relate to individuals' use of these services? Beyond individual explanations. J Consum Policy. (2017) 40:51–79. doi: 10.1007/s10603-016-9331-y
54. Baradaran M. The Color of Money: Black Banks and the Racial Wealth Gap. Cambridge, MA: Harvard University Press (2017). doi: 10.4159/9780674982284
55. Charron-Chénier R. Payday loans and household spending: How access to payday lending shapes the racial consumption gap. Soc Sci Res. (2018) 76:40–54. doi: 10.1016/j.ssresearch.2018.08.004
56. Cover J, Fuhrman Spring A, Garshick Kleit R. Minorities on the margins? The spatial organization of fringe banking services. J Urban Affairs. (2011) 33:317–44. doi: 10.1111/j.1467-9906.2011.00553.x
57. Dunham IM, Foster A. Proximate landscapes of economic inclusion in Southeastern Pennsylvania. Prof Geogr. (2015) 67:132–44. doi: 10.1080/00330124.2014.907697
58. Faber JW. Cashing in on distress: The expansion of fringe financial institutions during the Great Recession. Urban Affairs Rev. (2018) 54:663–96. doi: 10.1177/1078087416684037
59. Federal Deposit Insurance Corporation. Alternative financial services: a primer. FDIC Q. (2009) 3:39–47. Available online at: https://www.fdic.gov/analysis/quarterly-banking-profile/fdic-quarterly/2009-vol3-1/fdic140-quarterlyvol3no1-afs-final.pdf
60. Fowler CS, Cover JK, Kleit RG. The geography of fringe banking. J Reg Sci. (2014) 54:688–710. doi: 10.1111/jors.12144
61. Friedline T, Dunham IM, O'Brien M. The financial services environment and schools' savings rates in the San Francisco Kindergarten to College Program. J Consum Affairs. (2019) 53:1797–824. doi: 10.1111/joca.12264
62. Gallmeyer A, Roberts WT. Payday lenders and economically distressed communities: a spatial analysis of financial predation. Soc Sci J. (2009) 46:521–38. doi: 10.1016/j.soscij.2009.02.008
63. Graves SM. Landscapes of predation, landscapes of neglect: a location analysis of payday lenders and banks. Prof Geogr. (2003) 55:303–17. doi: 10.1111/0033-0124.5503017
64. Jorgensen M, Akee R. Access to Capital Credit in Native Communities: A Data Review, Digital Version. Tuscon, AZ: Native Nations Institute (2017). 62 p. Available online at: http://nni.arizona.edu/application/files/6514/8642/4513/Accessing_Capital_and_Credit_in_Native_Communities__A_Data_Review.pdf
65. Agarwal S, Gross T, Mazumder B. How did the Great Recession affect payday loans? Econ Perspect. (2016) 2:1–12. Available online at: https://fraser.stlouisfed.org/files/docs/historical/frbchi/economicperspectives/frbchi_econper_2016n2.pdf
66. Kasper SD. Payday lending: the case of Tennessee. J Econ Issues. (2014) 48:905–25. doi: 10.2753/JEI0021-3624480402
67. Richardson K. Petty debt: a comprehensive examination of Michigan's Deferred Presentment Industry and its growth between 2000-2018. [dissertation]. (2019). Lund, Sweden: Lunds Universitet.
68. Rothstein D, Dillman JD. The Continued Growth of Payday Lending in Ohio. Columbus, OH: Policy Matters Ohio (2008). 18 p.
69. Barth JR, Hilliard J, Jahera JS, Sun Y. Do state regulations affect payday lender concentration? J Econ Bus. (2016) 84:14–29. doi: 10.1016/j.jeconbus.2015.08.001
70. Bhutta N. Payday loans and consumer financial health. J Bank Fin. (2014) 47:230–42. doi: 10.1016/j.jbankfin.2014.04.024
71. Bhutta N, Goldin J, Homonoff T. Consumer borrowing after payday loan bans. J Law Econ. (2016) 59:225–59. doi: 10.1086/686033
72. National Conference of State Legislatures. Payday Lending State Statutes. (2021). Available online at: https://www.ncsl.org/research/financial-services-and-commerce/payday-lending-state-statutes.aspx (accessed on February 3, 2022).
73. Carter SP. Payday loan and pawnshop usage: The impact of allowing payday loan rollovers. J Consum Affairs. (2015) 49:436–56. doi: 10.1111/joca.12072
74. Dobbie W, Skiba PM. Information asymmetries in consumer credit markets: Evidence from payday lending. Am Econ J Appl Econ. (2013) 5:256–82. doi: 10.1257/app.5.4.256
75. Hodson R, Dwyer RE, Neilson LA. Credit card blues: The middle class and the hidden costs of easy credit. Sociol Q. (2014) 55:315–40. doi: 10.1111/tsq.12059
76. Sweet E, DuBois LZ, Stanley F. Embodied neoliberalism: Epidemiology and the lived experience of consumer debt. Int J Health Serv. (2018) 48:495–511. doi: 10.1177/0020731418776580
77. Allen H, Swanson A, Wang J, Gross T. Early Medicaid expansion associated with reduced payday borrowing in California. Health Aff. (2017) 36:1769–76. doi: 10.1377/hlthaff.2017.0369
78. Hundley CL, Wilson RW, Chenault J, Smimble JL. Is fringe banking a component of socially determined health? Am J Health Stud. (2017) 32:149–55. doi: 10.47779/ajhs.2017.83
79. Knapp EA, Bilal U, Dean LT, Lazo M, Celentano DD. Economic insecurity and deaths of despair in US counties. Am J Epidemiol. (2019) 188:2131–9. doi: 10.1093/aje/kwz103
80. Kubrin CE, Squires GD, Graves SM, Ousey GC. Does fringe banking exacerbate neighborhood crime rates? Criminol Public Policy. (2011) 10:437–66. doi: 10.1111/j.1745-9133.2011.00719.x
81. Matheson FI, Creatore MI, Gozdyra P, Park AL, Ray JG, A. population-based study of premature mortality in relation to neighbourhood density of alcohol sales and cheque cashing outlets in Toronto, Canada. BMJ Open. (2014) 4:e006032. doi: 10.1136/bmjopen-2014-006032
82. Collin L, Gaglioti A, Beyer K, Zhou Y, Moore M, Nash R, et al. Neighborhood-level redlining and lending bias are associated with breast cancer mortality in a large and diverse metropolitan area. Cancer Epidemiol Prevent Biomark. (2021) 30:53–60. doi: 10.1158/1055-9965.EPI-20-1038
83. Denney J, Saint Onge J, Dennis J. Neighborhood concentrated disadvantage and adult mortality: insights for racial and ethnic differences. Popul Res Policy Rev. (2018) 37:301–21. doi: 10.1007/s11113-018-9461-9
84. Finkelstein A, Gentzkow M, Williams H. Place-Based Drivers of Mortality: Evidence from Migration. Am Econ Rev. (2019) 111:2697–735. doi: 10.3386/w25975
85. Miller S, Soo CK. Do neighborhoods affect credit market decisions of low-income borrowers? Evidence from the moving to opportunity. Exp Rev Fin Stud. (2021) 34:827–63. doi: 10.1093/rfs/hhaa060
86. Cylus J, Glymour MM, Avendano M. Health effects of unemployment benefit program generosity. Am J Public Health. (2015) 105:317–23. doi: 10.2105/AJPH.2014.302253
87. Cylus J, Glymour MM, Avendano M. Do generous unemployment benefit programs reduce suicide rates? A state fixed-effect analysis covering 1968-2008. Am J Epidemiol. (2014) 180:45–52. doi: 10.1093/aje/kwu106
88. Freisthler B. A spatial analysis of social disorganization, alcohol access, and rates of child maltreatment in neighborhoods. Child Youth Serv Rev. (2004) 26:803–19. doi: 10.1016/j.childyouth.2004.02.022
89. Freisthler B, Gruenewald PJ. Where the individual meets the ecological: a study of parent drinking patterns, alcohol outlets, and child physical abuse. Alcohol Clin Exp Res. (2013) 37:993–1000. doi: 10.1111/acer.12059
90. Freisthler B, Maguire-Jack K. Understanding the interplay between neighborhood structural factors, social processes, and alcohol outlets on child physical abuse. Child Maltreat. (2015) 20:268–77. doi: 10.1177/1077559515598000
91. Morton CM, Simmel C, Peterson NA. Neighborhood alcohol outlet density and rates of child abuse and neglect: moderating effects of access to substance abuse services. Child Abuse Negl. (2014) 38:952–61. doi: 10.1016/j.chiabu.2014.01.002
92. Contreras C. A block-level analysis of medical marijuana dispensaries and crime in the city of Los Angeles. Justice Q. (2017) 34:1069–95. doi: 10.1080/07418825.2016.1270346
93. Freisthler B, Ponicki WR, Gaidus A, Gruenewald PJ, A. micro-temporal geospatial analysis of medical marijuana dispensaries and crime in Long Beach California. Addiction. (2016) 111:1027–35. doi: 10.1111/add.13301
94. Kepple NJ, Freisthler B. Exploring the ecological association between crime and medical marijuana dispensaries. J Stud Alcohol Drugs. (2012) 73:523–30. doi: 10.15288/jsad.2012.73.523
95. Lee AM, Gainey R, Triplett R. Banking options and neighborhood crime: does fringe banking increase neighborhood crime? Am J Crim Just. (2014) 39:549–70. doi: 10.1007/s12103-013-9228-0
96. Centers for Disease Control Prevention. Underlying Cause of Death, 1999-2020. Available online at: https://wonder.cdc.gov/ucd-icd10.html [Accessed June 1, 2022].
97. Manson Steven, Schroeder, Jonathan, Van, Riper, David, Kugler, Tracy, Ruggles, Steven. National Historical Geographic Information System: Version 16.0. Minneapolis, MN: IPUMS (2021). Available online at: https://www.nhgis.org/
98. Cameron, AC, Trivedi, PK. Regression Analysis of Count Data. New York: Cambridge University Press (2013). 566 p. doi: 10.1017/CBO9781139013567
99. Strumpf EC, Charters TJ, Harper S, Nandi A. Did the Great Recession affect mortality rates in the metropolitan United States? Effects on mortality by age, gender and cause of death. Soc Sci Med. (2017) 189:11–6. doi: 10.1016/j.socscimed.2017.07.016
100. Lee J, Kim KT. The increase in payday loans and damaged credit after the Great Recession. J Fam Econ Iss. (2018) 39:360–9. doi: 10.1007/s10834-017-9557-0
101. Ramirez SR. Regulation and the payday lending industry. Contemp Econ Policy. (2020) 38:675–93. doi: 10.1111/coep.12469
102. Bea M, Amorim M, Friedline T. Public Cash Assistance and Spatial Predation: How State Cash-Transfer Environments Shape High-Interest Lender Geography. Madison, WI: University of Wisconsin-Madison (2022).
103. Bea MD, Bley K. (Un)conditional consumer protections in high-cost lending regulation: Impacts on local lending geographies. J Consum Affairs. (2022) 1–32. doi: 10.1111/joca.12481
104. Bower KM, Thorpe RJ, Rohde C, Gaskin DJ. The intersection of neighborhood racial segregation, poverty, and urbanicity and its impact on food store availability in the United States. Prev Med. (2014) 58:33–9. doi: 10.1016/j.ypmed.2013.10.010
105. Nguyen C, Chernew M. Ostrer I, Beaulieu N. Comparison of healthcare delivery systems in low- and high-income communities. Am J Account Care (2019) 7:11–18. Available online at: https://www.ajmc.com/view/comparison-of-healthcare-delivery-systems-in-low-and-highincome-communities
Keywords: payday lending, debt, health, premature death, regulation
Citation: Agnew M, Bea MD and Friedline T (2022) Payday lenders and premature mortality. Front. Public Health 10:993585. doi: 10.3389/fpubh.2022.993585
Received: 13 July 2022; Accepted: 30 September 2022;
Published: 18 October 2022.
Edited by:
Shannon Monnat, Syracuse University, United StatesReviewed by:
Jason Houle, Dartmouth College, United StatesAdam Lippert, University of Colorado, United States
Copyright © 2022 Agnew, Bea and Friedline. This is an open-access article distributed under the terms of the Creative Commons Attribution License (CC BY). The use, distribution or reproduction in other forums is permitted, provided the original author(s) and the copyright owner(s) are credited and that the original publication in this journal is cited, in accordance with accepted academic practice. No use, distribution or reproduction is permitted which does not comply with these terms.
*Correspondence: Terri Friedline, tfriedli@umich.edu
†These authors have contributed equally to this work and their names are listed alphabetically