- 1Jangho Architecture College, Northeastern University, Shenyang, China
- 2Human Settlements Research Center, Liaoning Normal University, Dalian, China
- 3School of Humanities and Law, Northeastern University, Shenyang, China
- 4Institute of Geographic Sciences and Natural Resources Research, Chinese Academy of Sciences, Beijing, China
- 5Department of Microbiology and Plant Biology, Center for Spatial Analysis, University of Oklahoma, Norman, OK, United States
- 6School of Earth and Planetary Sciences (EPS), Curtin University, Perth, WA, Australia
Urban heat islands (UHIs) and their energy consumption are topics of widespread concern. This study used remote sensing images and building and meteorological data as parameters, with reference to Oke's local climate zone (LCZ), to divide urban areas according to the height and density of buildings and land cover types. While analyzing the heat island intensity, the neural network training method was used to obtain temperature data with good temporal as well as spatial resolution. Combining degree-days with the division of LCZs, a more accurate distribution of energy demand can be obtained by different regions. Here, the spatial distribution of buildings in Shenyang, China, and the law of land surface temperature (LST) and energy consumption of different LCZ types, which are related to building height and density, were obtained. The LST and energy consumption were found to be correlated. The highest heat island intensity, i.e., UHILCZ 4, was 8.17°C. The correlation coefficients of LST with building height and density were −0.16 and 0.24, respectively. The correlation between urban cooling energy demand and building height was −0.17, and the correlation between urban cooling energy demand and building density was 0.17. The results indicate that low- and medium-rise buildings consume more cooling energy.
Introduction
Climate change has become a formidable challenge faced by all countries in the world because of its possible consequences (1). It impacts sea level rise, biodiversity, agricultural production, frequency of extreme weather, human health, and energy demand (2). There is a direct relationship between climate change and energy demand. Climate warming mainly results from the excessive absorption of long-wave radiation from the underlying surface by greenhouse gases (3). Large quantities of fossil fuels are utilized to meet the needs of human production and life processes, resulting in the emission of greenhouse gases (4). Among them, global building energy consumption accounts for ~20 to 40% of total energy consumption and ~33% of greenhouse gas emission (5).
To cope with rising temperatures and manage living standards, residents increase the use of air-conditioning and other refrigeration facilities to reduce indoor temperatures, but these measures in turn intensify the heat island effect. The environmental changes in cities are more complex, owing to many impervious surfaces and human activities (6). High-density buildings change the path and quantity of regional energy absorption and storage, flow and reflection, release, and consumption (7). The response of energy consumption and demand to temperature rise is more complicated. There are differences in the temperature of cities in different regions and scales, at different times and seasons, and even in the distribution characteristics of temperature within cities (8, 9). In general, the growth in expenditure caused by the increase in cooling demand exceeds the savings brought about by the reduction in heating demand, especially at low latitudes. For every 2°C increase in the average land surface temperature (LST), the global net building energy expenditure will increase by 0.1% of the total global economic expenditure (10).
The estimation of energy consumption concentrates on the city scale, and the estimation methods can be divided into two categories: top-down and bottom-up (11). The top-down approach is based on statistics and uses regional urban data to estimate the spatial distribution of energy consumption across cities, based on macroeconomic indicators such as population density, income, energy prices and types, and urban morphology (12). The bottom-up approach is based on each individual building and can be a statistical or physical-based hybrid model (13). Previous research has shown that the net energy impact of the urban environment depends on climate type (14), the urban context density, and functional and structural characteristics of buildings (15).
To improve the accuracy of bottom-up methods, some studies have incorporated local climate into the energy performance simulation of urban buildings. The urban heat island (UHI) effect, which refers to the phenomenon wherein the temperature in cities is higher than that in suburban rural areas (16), is also considered in the estimation of building energy consumption. Palme et al. (17) put forward the method of incorporating the UHI effect into building performance simulation and found that when UHI is incorporated, the energy demand increased by 15–200%. However, simulation methods are often calculation-heavy, and the relationship between urban features and energy consumption is indirect and unclear.
The intensity of heat islands is generally measured by the temperature difference between urban and rural areas. However, there has been no unified statement on how to define urban and rural areas. Over the past century, many scholars have made the division based on population density, building density, and landscape differences, or used gradients to illustrate problems. The duality of urban and rural areas has weakened with the development of cities (18, 19). Stewart and Oke (20) proposed the local climate zone (LCZ) concept to study UHIs, in which the city is divided into different areas according to the height density of buildings and other ground cover. Wong et al. (21) found that the influence of floor area ratio on temperature in Singapore is as high as 2°C, and buildings can save 4.5% on energy consumption by improving urban form. This deconstruction of urban internal structure divides it into organizational units, which can account for the internal form of buildings while segmenting urban areas, and is suitable for studying the influence of urban form on the thermal environment and energy demand.
The scope of energy consumption is highly complex, and all climate changes determined by the urban environment should be considered when performing simulations. For this reason, some coupling techniques between the building energy model and microclimate CFD model have been proposed (22, 23). However, this considers too many measured data (such as climate, wind speed, temperature, building texture, and thermal radiation), which require longer calculation times and higher costs. This is unfavorable for discussing the continuous changes of heating and cooling energy demand in the future. Invidiata and Ghisi (24) also emphasized that meteorological data were not updated in time in the process of estimating indoor air temperature. Obtaining reliable time-sensitive data to evaluate energy consumption is a major challenge in this research.
The scope of energy consumption is highly complex. Cooling energy consumption for the thermal environment under the UHI effect can be calculated from electricity consumption and remote sensing inversion data (25), such as the effective U-value method, degree-days, bin method, load frequency table method, equivalent full load hours, weighting factor method, and heat balance method (26). For cities, the degree-days method is simple and suitable for larger areas (27); however, traditional degree-days can only describe a single region at once and cannot analyze the distribution of energy consumption within the entire city. Combined with local climatic zone, the influence of different building forms and land use types on the urban thermal environment and refrigeration energy consumption can be examined in more detail. This macroscopic and easy-to-measure method is more universal and operable.
This new attempt requires more spatial distribution density of meteorological data, and traditional station data cannot meet the requirements. Traditional interpolation analysis using meteorological station data is less accurate. Remote sensing data is appropriate as continuous data. LST data is used frequently for calculations of the surface heat island, whereas for the cooling energy demands of urban dwellers indoors, air temperature is clearly more appropriate. Mesoscale weather Research and Forecasting Model and neighborhood-scale microclimate simulations, for example, are not suitable for urban studies (28). Of the methods that can be applied, regression statistics is the simplest and easiest to build; however, establishing a relevant model depends on the time and place of data acquisition, which may cause large errors. The temperature-vegetation index method is highly dependent on plants; therefore, it is not suitable for cities with a large impermeable surface coverage. The surface energy balance method has good portability and universality, but many physical parameters needed by the model cannot be obtained directly by remote sensing, and the data cannot be updated in real time. Such models yield sufficiently accurate estimates, provided that all the required parameters are involved in the calculation. The parameters is not always possible, and thus, the data-driven model was selected. Neural network machine learning relies on large amounts of sample data, which can experience difficult modeling problems starting as a black box and express the nonlinear relationship between LST and air temperature (29, 30).
This study used remote sensing images, building data, and meteorological data as parameters and referred to Oke's LCZ method to divide urban areas according to the height and density of buildings and land cover types. The research attempted to apply neural networks for retrieving air temperature to calculate cooling energy demand, combining the number of degree days with the division of local climatic zones. The aims of the study were to improve energy demand structure of space and provide a relevant reference for urban planning under the prerequisite of ensuring the comfort of urban residents.
Materials and methods
Study area
Shenyang City (Figure 1) is located in Northeast China, central Liaoning (122°24′59″-123°48′30 E; 43°2′25″-41°11′53″N). The terrain gradually changes from hills in the northeast to plains in the southwest. The region has a temperate, semi-humid continental climate. Rainfall is concentrated in the summer months, but the area is sunnier than South China, making it conducive to the acquisition of remote sensing images. It is the political, economic, and cultural center of the Northeast region, as well as an important transportation hub. The building types are diverse and comprised of varying building height densities. Human activities have affected this area, leading to local climate change. In recent years, the annual maximum temperature in Shenyang exceeded 38°C in the summer.
Data sources and processing
The data used mainly included MODIS remote sensing, temperature, building outline, land cover, and administrative division. Table 1 contains detailed information on the remote sensing platforms and related data processing. In terms of time selection, according to the “Uniform Standard for Civil Building Design” GB 50352-2019, building climate zoning uses the average temperature in July as the main reference for summer. In traditional Chinese solar terms, dog days, the days of highest temperature generally start in mid-July. Considering the availability of data, the study period ranged from mid-July to early August. The technical flowchart is shown in Supplementary Figure 1.
Local climate zone
The urban form and nature of the surface are one of the main reasons for the difference between the urban LST and air temperature. In this study, we referred the definitions of Stewart and Oke (20) to divide the city into LCZ A–G according to the types of natural cover (Detailed classification diagrams are in the Supplementary material). Vertically, the height of the building class was divided from low-rise to super high-rise buildings at consistent intervals of three floors. Horizontally, the building densities were divided into dense and open building groups with a boundary of 40%. Accordingly, LCZ 1–10 were obtained (31). Finally, 17 categories were obtained, as shown in Supplementary Table 1. The height (BH) and density (BD) of the buildings were calculated on a 30 × 30 m grid using Eqs. (1) and (2).
Where n represents the number of single buildings in a grid, Hi represents the number of floors of the i-th building in a grid, Sgrid represents the area of a grid, and Sbuilding represents the base area of all buildings in the grid.
Heat island effect intensity
The LST was retrieved using Tan Zhihao's single-window algorithm, and Landsat was selected for heat island intensity analysis (32) (see the calculation section of the Supplementary materials). The intensity of the heat island effect is usually measured by the temperature difference between urban and rural areas. Stewart and Oke combined this temperature difference with the local climate zoning of the city and defined the formula of UHI intensity as the difference between a certain type of LCZ (X) and LCZ D (low vegetation, such as grass) (33). The intensity of the heat island effect is shown by Eq. (3).
Where UHILCZX and TLCZXrepresent the heat island effect intensity and LST of a certain type of LCZ X, respectively, and TLCZD represents the LST of the LCZ D type.
Cooling energy demand
Using the method of combining cooling degree-days (CDD) (34) with LCZ, we calculated the change in building energy demand for different types of LCZ at different summer temperatures. The basic formula is shown in Eq. (5). According to previous experience, 23–24°C is the most comfortable ambient temperature for human life (35), and the difference between the interior design temperature of a building and the heat balance temperature of the building is usually 3–7°C (36). Finally, the base temperature of the cooling day was determined to be 26°C.
Where CDD26 represents the number of cooling degree-days with 26°C as the base temperature, t is the average temperature of approximately a month, and tref is the base temperature.
Weather station data are generally used when calculating the number of degree-days. However, the meteorological station data were discrete point data, and were not detailed enough for research on urban interior building patterns. Therefore, we used remote sensing images in this study to retrieve air temperature as the basic temperature data calculated by CCD to evaluate the energy demand of buildings in a typical month.
Considering the accuracy and difficulty of calculation, the four factors, namely remote sensing albedo, normalized difference vegetation index (NDVI), elevation, and LST, were selected (37–39). The meteorological station data in the study area were used as the verification data, and the neural network regression model (40, 41) was used to train a model for inversion of air temperature. After several attempts, the training algorithm was finally settled on Levenberg-Marquardt, a toolbox already written in Matlab. For MODIS MCD43C3 products, the daily surface albedo α of the short-wave band can be calculated using Eqs. (5) and (6) (42–44). Finally, the formula for calculating the building energy demand under a certain type of LCZ is shown in Eq. (7).
In the formulas, αW represents the albedo of the white sky, αBrepresents the albedo of the black sky, r represents the ratio of the sky scattered radiation to the downward solar radiation, and θ represents the solar zenith angle of the study area at noon. The values required in Eqs. (5) and (6) are given in MODIS MCD43C3. tLCZX is the average air temperature within the study time range of X under the LCZ system.
Results
Spatial pattern distribution of local climate zones
The main urban area of Shenyang is dominated by low-rise buildings, which are widely and evenly distributed, followed by the middle-rise and mid-high-rise buildings, which are concentrated in the center of the main urban area. The number of buildings below 40% density is evenly distributed, rising steeply up to 40%, reaching a peak, and then slowly falling. In terms of spatial distribution, urban buildings were high-density and low-density, with low-density buildings dominating, but with no specific area of concentration. The LCZ distributions are shown in Figure 2.
LCZ 10 accounted for 11.69% and featured the largest proportion of building coverage due to the large number of villages outside the built-up area; LCZ 9 ranked second, accounting for 1.66%, and contained mostly residential land. LCZ 2 accounted for the smallest proportion (0.94%) and was concentrated in the city center, around the factory, near the high-speed railway station, and on the south bank of the Hunhe River. The proportion of LCZ 7 was slightly higher than that of LCZ 2. Natural cover was dominated by cultivated land in the northwest and lush forest land in the southeast, accounting for 39.21 and 25.99%, respectively; sparse forest land accounted for the lowest coverage (0.30%). The city center of Shenyang is surrounded by arable land and woodland, but the center itself has less green space and is crowded by buildings. The surrounding development requires strengthening.
Urban thermal environment space
The LST (Figure 3) of the building coverage was generally higher than that of the natural coverage. The average temperature of various LCZ overall displayed the following distribution law: LCZ 4 > LCZ 9 > LCZ 5 > LCZ 8 > LCZ 3 > LCZ 10 > LCZ 7 > LCZ 2 > LCZ 6 > LCZ 1 > LCZ E > LCZ F > LCZ A > LCZ B > LCZ D > LCZ G > LCZ C (Figure 4).
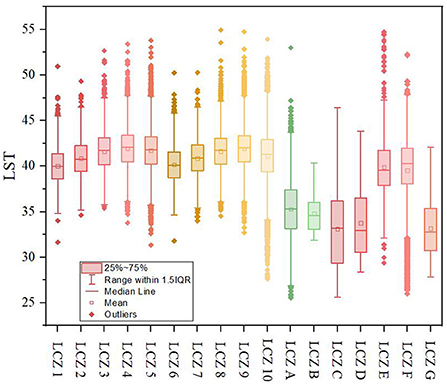
Figure 4. Distribution of LST in LCZ (LST unit:°C). LST, land surface temperature. LCZ, local climate zone. The results are sampled and counted according to the calculation results associated with the LCZ grids.
Among them, the temperature of LCZ D is 33.77°C. The highest heat island strength, UHILCZ 4, was 8.17°C, and the lowest, UHILCZC, was −0.68. The lowest heat island strength of the building coverage UHILCZ1 was 6.23°C. The LST changes of LCZ 1 were the most stable, with a standard deviation of 1.96, while LCZ G showed the most dramatic changes, with a standard deviation of 4.11, and the standard deviations of the other types were concentrated in the range of 2.8 ± 0.96. LST was negatively correlated with building height, with a Pearson correlation coefficient of −0.16, reaching significance at the 0.01 level, and positively correlated with building density, with a correlation coefficient of 0.24, reaching significance at the 0.01 level. These results can be explained by the fact that although higher buildings accumulate artificial materials and create more light reflections in three dimensions, they also cast larger shadows on the ground, which reduce the LST.
Urban cooling energy demand
In neural network estimation of air temperature, 70% of the data were randomly selected as the training sample, 15% as the verification sample, and 15% as the test sample to invert the air temperature. The model mean square error was RMSE = 0.32, R = 0.92, and R2 = 0.84 (Supplementary Figure 4). According to these data, the overall range of air temperature was included in the distribution interval of the LST, and the value was between 28 and 33°C. The various types of air temperature change ranges were relatively stable and exhibited low volatility.
The average energy consumption of the building covering generally presented the distribution law of LCZ 8 > LCZ 3 > LCZ 9 > LCZ 4 > LCZ 2 > LCZ 7 > LCZ 5 > LCZ 6 > LCZ 1 > LCZ 10 (Figure 5). Among them, the maximum value of CDD was 7.00°C · d, the lowest value was 2.06°C · d, and the standard deviation of the different building coverage types varied between 0.232 and 0.76°C · d. The Pearson correlation between urban cooling energy demand and building height reached −0.17, and the correlation with building density was 0.17, both of which passed the 0.01 level two-tailed significance test. Compared with the distribution of cooling energy demand, LST is more affected by building height and density.
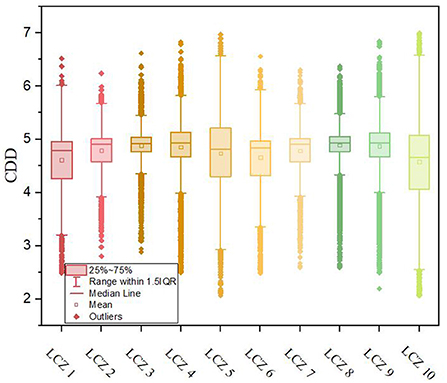
Figure 5. Distribution of CDD in LCZ (CDD unit:°C d). CDD, cooling degree-days. The results are sampled and counted according to the calculation results associated with the grid of the different LCZ plots.
Discussion
Impact of local climatic zones on cities
The distribution of air temperature is more concentrated than that of LST, and the temperature is lower; however, the distribution difference between different local climatic zones is not so obvious. LST and cooling energy demand are positively correlated with building density. Compared with cooling energy demand, LST is more affected by building density (Figure 6); however, there is a weak negative correlation between LST and building height (Figure 7). The negative correlation may be explained by the addition of super high-rise buildings (43–45). Although the taller buildings accumulate man-made materials and produce more light reflection in three dimensions, they also cast larger shadows on the ground, thus lowering the LST. The correlation with building height is not as high as that with building density because of these complex relationships.
Figure 8 shows that the CDD value gradually increases from the outskirts of the city to the center, but the range greater than 5.0°C · d surrounds the range of 4.5–5.0°C · d, showing a concave distribution. This phenomenon was attributed to the effects of the Hun River, which flows east-to west and appears in the south-central part of the study area, and Youth Street that runs north to south and is located in the middle of the city. The summer winds blow north through the Hunhe River and pass through Qingnian Street, forming a ventilation corridor that somewhat controls the regional temperature and reduces the potential cooling energy demand (46). Based on the dark red area in Figure 8, for general planning we recommend that coverage by green spaces (47, 48) or water bodies is increased to reduce energy consumption, and LCZ8, LCZ3, and LCZ9 should be focused on and reduced.
The difference of air temperature is not as large as that of land surface temperature, which also shows the importance of the material selection of the underlying surface from the side. It is recommended to increase vegetation and water area, sprinkle water on the road, and appropriately reduce the density of urban buildings to reduce the temperature.
Limitations
The Landsat image for heat island intensity analysis has relatively high resolution. Although Landsat's temporal resolution cannot meet the needs of daily data in the study time range, the spatial distribution of LST in sunny weather is still representative.
As a calculation method of cooling energy demand, the degree-days are simple and effective in characterizing urban energy; however, this method does not fully consider the effect of individual building differences (49, 50). For the study of overall characteristics, these parameters are simplified in the modeling process, but for urban planning, these factors are closely integrated with energy conservation.
The accuracy of the model used in this study was mainly limited by the remote sensing data and the number of meteorological stations in the study area. Because the calculation of energy consumption requires a high temporal resolution of remotely sensed images, MODIS products were finally selected from the available data (51, 52). The air temperature retrieved by combining various factors can show the characteristics of distribution in space, but the improvement of the initial data quality can greatly improve the accuracy of the results. Due to the limited availability of data, this study selected provincial capital cities with richer building data for analysis; however, there are still missing data in some areas. Future studies should focus on other thermal environment characteristic indicators, simultaneously consider the energy consumption requirements of cooling and heating, and use long time series and high-resolution data.
Conclusions
This study used remote sensing images, building data, meteorological data, and other basic data, referring to Oke's LCZ, to divide urban areas according to the height and density of buildings and land cover types, and explored the distribution characteristics of energy consumption that may be generated by urban residents' refrigeration demand in different regional thermal environments. The following conclusions were obtained.
The main urban area of Shenyang is dominated by low-rise buildings, which are widely and evenly distributed, and mid-high-rise buildings, which are crowded in the center.
The distribution of air temperature is more concentrated than that of LST, and the temperature is lower; thus, the difference in the distribution among the various local climatic zones is not evident.
The LST of the building covering is generally higher than that of the natural covering. The average temperature overall displays the distribution law as LCZ 4 > LCZ 9 > LCZ 5 > LCZ 8 > LCZ 3 > LCZ 10 > LCZ 7 > LCZ 2 > LCZ 6 > LCZ 1> LCZ E > LCZ F > LCZ A > LCZ B > LCZ D > LCZ G > LCZ C. Among them, the temperature of LCZ D is 33.77°C. The highest heat island intensity of UHILCZ4 was 8.17°C. The LST is negatively correlated with building height, with a correlation coefficient of −0.16, reaching significance at a level of 0.01; and a positive correlation with building density, with a correlation coefficient of 0.24, reaching significance at the 0.01 level.
The average energy consumption of the building covering generally presents the distribution law of LCZ 8 > LCZ 3 > LCZ 9 > LCZ 4 > LCZ 2 > LCZ 7 > LCZ 5 > LCZ 6 > LCZ 1 > LCZ 10. Among them, the maximum value of CDD was 6.997°C · d, and the lowest value was 2.06°C · d. The correlation between urban cooling energy demand and building height reached −0.17, and the correlation between urban cooling energy demand and building density was 0.17, with both correlation coefficients shown to be statistically significant through a two-tailed significance test (p < 0.01).
Data availability statement
The original contributions presented in the study are included in the article/Supplementary material, further inquiries can be directed to the corresponding author/s.
Author contributions
RY wrote the main manuscript text. JY directed and revised the manuscript and contributed to all aspects of this work. LW, XX, and JX conducted the experiment and analyzed the data. All authors reviewed the manuscript.
Funding
This research study was supported by the National Natural Science Foundation of China (Grant Nos. 41771178 and 42030409), the Fundamental Research Funds for the Central Universities (Grant No. N2111003), Basic Scientific Research Project (Key Project) of the Education Department of Liaoning Province (Grant No. LJKZ0964), and Innovative Talents Support Program of Liaoning Province (Grant No. LR2017017).
Acknowledgments
The authors would like to acknowledge all colleagues and friends who have voluntarily reviewed the translation of the survey and the manuscript of this study.
Conflict of interest
The authors declare that the research was conducted in the absence of any commercial or financial relationships that could be construed as a potential conflict of interest.
Publisher's note
All claims expressed in this article are solely those of the authors and do not necessarily represent those of their affiliated organizations, or those of the publisher, the editors and the reviewers. Any product that may be evaluated in this article, or claim that may be made by its manufacturer, is not guaranteed or endorsed by the publisher.
Supplementary material
The Supplementary Material for this article can be found online at: https://www.frontiersin.org/articles/10.3389/fpubh.2022.992050/full#supplementary-material
References
1. He B. Potentials of meteorological characteristics and synoptic conditions to mitigate urban heat Island effects. Urban Climate. (2018) 18:2426–33. doi: 10.1016/j.uclim.2018.01.004
2. De Coninck H, Revi A, Babiker M, Bertoldi P, Buckeridge M, Cartwright A, et al. “Strengthening and implementing the global response,” in Global Warming Of 1.5°C: Summary for Policy Makers, IPCC - The Intergovernmental Panel On Climate Change. (2018), p. 313–443.
3. Montzka SA, Dlugokencky EJ, Butler JH. Non-Co2 greenhouse gases and climate change. Nature. (2011) 476:43–50. doi: 10.1038/nature10322
4. Rodhe H. A comparison of the contribution of various gases to the greenhouse effect. Science. (1990) 248960:1217–9. doi: 10.1126/science.248.4960.1217
5. Wei Y, Zhang X, Shi Y, Xia L, Pan S, Wu J, et al. A review of data-driven approaches for prediction and classification of building energy consumption. Renew. Sustain. Energy Rev. (2018) 18:821027–1047. doi: 10.1016/j.rser.2017.09.108
6. Ren J, Yang J, Zhang Y, Xiao X, Xia JC, Li X, et al. Exploring thermal comfort of urban buildings based on local climate zones. J Clean Prod. (2022) 22:340130744. doi: 10.1016/j.jclepro.2022.130744
7. Theeuwes NE, Barlow JF, Teuling AJ, Grimmond SB, Kotthaus S. Persistent cloud cover over mega-cities linked to surface heat release. Npj Clim Atmosph Sci. (2019) 9:2. doi: 10.1038/s41612-019-0072-x
8. Jaber SM, Abu-Allaban MM. Modis-based land surface temperature for climate variability and change research: the tale of a typical semi-arid to arid environment. Eur J Remote Sens. (2020) 53:81–90. doi: 10.1080/22797254.2020.1735264
9. Peng J, Ma J, Liu Q, Liu Y, Hu Y, Li Y, et al. Spatial-temporal change of land surface temperature across 285 cities in china: an urban-rural contrast perspective. Sci Total Environ. (2018) 18:635487–497. doi: 10.1016/j.scitotenv.2018.04.105
10. Clarke L, Eom J, Marten EH, Horowitz R, Kyle P, Link R, et al. Effects of long-term climate change on global building energy expenditures. Energy Econ. (2018) 18:72667–677. doi: 10.1016/j.eneco.2018.01.003
11. Frayssinet L, Merlier L, Kuznik F, Hubert J, Milliez M, Roux J. Modeling the heating and cooling energy demand of urban buildings at city scale. Renew Sustain Energy Rev. (2018) 18:812318–2327. doi: 10.1016/j.rser.2017.06.040
12. De Cian E, Lanzi E, Roson R. The Impact Of Temperature Change On Energy Demand: A Dynamic Panel Analysis. (2007). doi: 10.2139/ssrn.984237
13. Swan LG, Ugursal VI. Modeling of end-use energy consumption in the residential sector: a review of modeling techniques. Renew Sustain Energy Rev. (2009) 13:1819–35. doi: 10.1016/j.rser.2008.09.033
14. Wan KKW, Tsang CL, Lam JC. Sensitivity analysis of building energy use in different climates. Ifac Proceed Vol. (2010) 43:58–62. doi: 10.3182/20100329-3-PT-3006.00013
15. Salvati A, Coch H, Cecere C. Urban morphology and energy performance: the direct and indirect contribution in mediterranean climate. In: Plea2015 Architecture In (R)Evolution – 31st International Plea Conference. Italy. (2015).
16. Rizwan AM, Dennis LYC, Liu C. A review on the generation, determination and mitigation of urban heat Island. J Environ Sci. (2008) 20:120–8. doi: 10.1016/S1001-0742(08)60019-4
17. Palme M, Inostroza L, Villacreses G, Lobato-Cordero A, Carrasco C. From urban climate to energy consumption. Enhanc Build Perform Simul Includ Urban Heat Island Effect Energy Build. (2017) 145107–120. doi: 10.1016/j.enbuild.2017.03.069
18. Dymitrow M, Biegańska J, Grzelak-Kostulska E. Deprivation And The Rural-Urban Trap. Tijdschrift Voor Economische En Sociale Geografie. (2018) 109:87–108. doi: 10.1111/tesg.12263
19. Lin C. Changing theoretical perspectives on urbanization in asian developing countries. Vol. (1994) 16:1–23. doi: 10.3828/twpr.16.1.4862425322u55h41
20. Stewart ID, Oke TR. Local climate zones for urban temperature studies. Bull Am Meteorol Soc. (2012) 93:1879–900. doi: 10.1175/BAMS-D-11-00019.1
21. Wong NH, Jusuf SK, Syafii NI, Chen Y, Hajadi N, Sathyanarayanan H, et al. Evaluation of the impact of the surrounding urban morphology on building energy consumption. Solar Energy. (2011) 85:57–71. doi: 10.1016/j.solener.2010.11.002
22. Lauzet N, Rodler A, Musy M, Azam M, Guernouti S, Mauree D, et al. How building energy models take the local climate into account in an urban context – a review. Renew Sustain Energy Rev. (2019) 116109390. doi: 10.1016/j.rser.2019.109390
23. Yang X, Zhao L, Bruse M, Meng Q. An integrated simulation method for building energy performance assessment in urban environments. Energy Build. (2012) 54243–251. doi: 10.1016/j.enbuild.2012.07.042
24. Invidiata A, Ghisi E. Impact of climate change on heating and cooling energy demand in houses in Brazil. Energy Build. (2016) 13020–32. doi: 10.1016/j.enbuild.2016.07.067
25. Jin C, Zhang Y, Yang X, Zhao N, Ouyang Z, Yue W. Mapping China's electronic power consumption using points of interest and remote sensing data. Remote Sens. (2021) 13:1058. doi: 10.3390/rs13061058
26. Al-Homoud MS. Computer-aided building energy analysis techniques. Build Environ. (2001) 36:421–33. doi: 10.1016/S0360-1323(00)00026-3
27. Parti M, Parti, C,. The Total Appliance-Specific Conditional Demand For Electricity In The Household Sector Author(S): Michael Parti Cynthia Parti Source: The Bell Journal Of Economics. 11, No. 1 (Spring, 1980), 309-321. Published By: Rand Corporation Stable. (1980). Available online at: http://www.Jstor.Org/Stable/3003415. (accessed 28/06/2014). doi: 10.2307/3003415
28. Jandaghian Z, Berardi U. Analysis of the cooling effects of higher albedo surfaces during heat waves coupling the weather research and forecasting model with building energy models. Energy Build. (2020) 20:7109627. doi: 10.1016/j.enbuild.2019.109627
29. Shanyou Z, Guixin Z. Progress in near surface air temperature retrieved by remote sensing technology. Adv Earth Sci. (2011) 26:724–30.
30. Yang Y, Cai W, Yang J. Evaluation of modis land surface temperature data to estimate near-surface air temperature in northeast China. Rem Sens. (2017) 9:410. doi: 10.3390/rs9050410
31. Yang J, Wang Y, Xiao X, Jin C, Xia JC, Li X. Spatial differentiation of urban wind and thermal environment in different grid sizes. Urban Climate. (2019) 19:28100458. doi: 10.1016/j.uclim.2019.100458
32. Tan Z, Zhang M, Arnon K, Pedro B. A single window algorithm for land surface temperature calculation using tm6 data. Acta Geographica Sinica. (2001) 1:456–466.
33. Stewart ID, Oke TR, Krayenhoff ES. Evaluation of the ‘local climate zone' scheme using temperature observations and model simulations. Int J Climatol. (2014) 34:1062–80. doi: 10.1002/joc.3746
34. Alsayed MF, Tayeh RA. Life cycle cost analysis for determining optimal insulation thickness in palestinian buildings. J Build Eng. (2019) 22101–112. doi: 10.1016/j.jobe.2018.11.018
35. Chen X, Xue P, Gao L, Du J, Liu J. Physiological and thermal response to real-life transient conditions during winter in severe cold area. Build Environ. (2019) 157284–296. doi: 10.1016/j.buildenv.2019.04.004
36. Martincevic A, Vasak M, Lesic V. Model predictive control for energy-saving and comfortable temperature control in buildings. Inieee. (2016) 2:298–303. doi: 10.1109/MED.2016.7536001
37. Deng Z, Chen Q. Artificial neural network models using thermal sensations and occupants' behavior for predicting thermal comfort. Energy Build. (2018) 174587–602. doi: 10.1016/j.enbuild.2018.06.060
38. Lin B, Yongming XU, Miao HE, Ning LI, Abu-Allaban MM. Remote sensing inversion of near surface air temperature based on random forest. J Geo-Inform Sci. (2017) 19:390–7.
39. Papantoniou S, Kolokotsa D. Prediction of outdoor air temperature using neural networks: application in 4 european cities. Energy Build. (2016) 16:11472–79. doi: 10.1016/j.enbuild.2015.06.054
40. Jang JD, Viau AA, Anctil F. Neural network estimation of air temperatures from avhrr data. Int J Remote Sens. (2004) 25:4541–54. doi: 10.1080/01431160310001657533
41. Dengzhong Z, Wanchang Z, Shijin X. “A neural network algorithm to retrieve near- surface air temperature from landsat etm+ imagery over the hanjiang river basin, china,” in 2007 IEEE International Geoscience And Remote Sensing Symposium. (2008). doi: 10.1109/IGARSS.2007.44234146
42. Cadeddu MP, Liljegren JC, Turner DD. The atmospheric radiation measurement (arm) program network of microwave radiometers: instrumentation, data, and retrievals. Atmosph Measure Techniq. (2013) 6:2359–72. doi: 10.5194/amt-6-2359-2013
43. Yang J, Ren J, Sun D, Xiao X, Xia JC, Jin C, et al. Understanding land surface temperature impact factors based on local climate zones. Sustainable Cit Soc. (2021) 21:69102818. doi: 10.1016/j.scs.2021.102818
44. Yang J, Wang Y, Xue B, Li Y, Xiao X, Xia JC, et al. Contribution of urban ventilation to the thermal environment and urban energy demand: different climate background perspectives. Sci Total Environ. (2021) 21:795148791. doi: 10.1016/j.scitotenv.2021.148791
45. Yang X, Li Y. The impact of building density and building height heterogeneity on average urban albedo and street surface temperature. Build Environ. (2015) 15:90146–156. doi: 10.1016/j.buildenv.2015.03.037
46. Xie P, Yang J, Sun W, Xiao X, Cecilia Xia J. Urban scale ventilation analysis based on neighborhood normalized current model. Sustain Cities Soc. (2022) 80103746. doi: 10.1016/j.scs.2022.103746
47. Liu W, Zhao H, Sun S, Xu X, Huang T, Zhu J. Green space cooling effect and contribution to mitigate heat island effect of surrounding communities in beijing metropolitan area. Front Public Health. (2022) 22:10870403–870403. doi: 10.3389/fpubh.2022.870403
48. Wei D, Yang L, Bao Z, Lu Y, Yang H. Variations in outdoor thermal comfort in an urban park in the hot-summer and cold-winter region Of China. Sustain Cities Soc. (2022) 22:77103535. doi: 10.1016/j.scs.2021.103535
49. Abu-Jdayil B, Mourad A, Hittini W, Hassan M, Hameedi S. Traditional, state-of-the-art and renewable thermal building insulation materials: an overview. Construct Build Mater. (2019) 214709–735. doi: 10.1016/j.conbuildmat.2019.04.102
50. Crawley DB, Hand JW, Kummert M, Griffith BT. Contrasting the capabilities of building energy performance simulation programs. Build Environ. (2008) 43:661–73. doi: 10.1016/j.buildenv.2006.10.027
51. Xu Y, Qin Z, Shen Y. Study on the estimation of near-surface air temperature from modis data by statistical methods. Int J Remote Sens. (2012) 33:7629–43. doi: 10.1080/01431161.2012.701351
Keywords: urban heat island, energy consumption, degree-days, neural network, air temperature inversion
Citation: Yang R, Yang J, Wang L, Xiao X and Xia J (2022) Contribution of local climate zones to the thermal environment and energy demand. Front. Public Health 10:992050. doi: 10.3389/fpubh.2022.992050
Received: 12 July 2022; Accepted: 21 July 2022;
Published: 09 August 2022.
Edited by:
Yibin Ao, Chengdu University of Technology, ChinaReviewed by:
Dongrui Han, Shandong Academy of Agricultural Sciences, ChinaXiaoping Zhang, Shandong Jianzhu University, China
Copyright © 2022 Yang, Yang, Wang, Xiao and Xia. This is an open-access article distributed under the terms of the Creative Commons Attribution License (CC BY). The use, distribution or reproduction in other forums is permitted, provided the original author(s) and the copyright owner(s) are credited and that the original publication in this journal is cited, in accordance with accepted academic practice. No use, distribution or reproduction is permitted which does not comply with these terms.
*Correspondence: Jun Yang, yangjun8@mail.neu.edu.cn; Lingen Wang, wangle@igsnrr.ac.cn