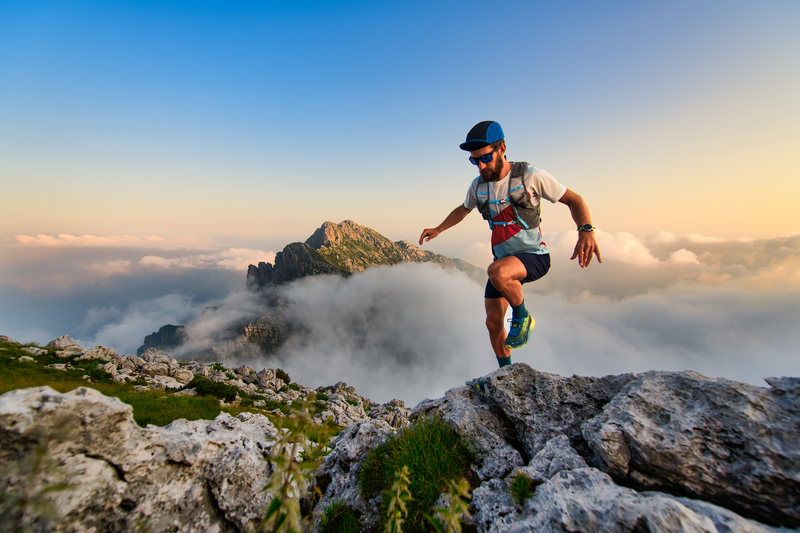
95% of researchers rate our articles as excellent or good
Learn more about the work of our research integrity team to safeguard the quality of each article we publish.
Find out more
ORIGINAL RESEARCH article
Front. Public Health , 24 January 2023
Sec. Infectious Diseases: Epidemiology and Prevention
Volume 10 - 2022 | https://doi.org/10.3389/fpubh.2022.978237
Objective: This study aimed to analyze the association between the activity of daily living (ADL), coronavirus disease (COVID-19), and the value of the Barthel Index in predicting the prognosis of patients.
Methods: This study included 398 patients with COVID-19, whose ADL at admission to hospital were assessed with the Barthel Index. The relationship between the index and the mortality risk of the patients was analyzed. Several regression models and a decision tree were established to evaluate the prognostic value of the index in COVID-19 patients.
Results: The Barthel Index scores of deceased patients were significantly lower than that of discharged patients (median: 65 vs. 90, P < 0.001), and its decrease indicated an increased risk of mortality in patients (P < 0.001). After adjusting models for age, gender, temperature, pulse, respiratory rate, mean arterial pressure, oxygen saturation, etc., the Barthel Index could still independently predict prognosis (OR = 0.809; 95% CI: 0.750–0.872). The decision tree showed that patients with a Barthel Index of below 70 had a higher mortality rate (33.3–40.0%), while those above 90 were usually discharged (mortality: 2.7–7.2%).
Conclusion: The Barthel Index is of prognostic value for mortality in COVID-19 patients. According to their Barthel Index, COVID-19 patients can be divided into emergency, observation, and normal groups (0–70; 70–90; 90–100), with different treatment strategies.
Coronavirus disease (COVID-19) has grown rapidly and become a serious medical crisis that the whole world has had to confront (1–3). With the global trend of the epidemic, more than 500 million people are estimated to have suffered from COVID-19, with over 6 million people dead (4). The disease influences multiple organs and the overall function of the body, causing various symptoms including cough, breathing difficulties, headache, diarrhea, and muscle or joint pain (5). Some patients have severe symptoms such as stroke, seizures, and kidney failure (6–8), which greatly affect their activity of daily living (ADL). Meanwhile, the huge number of patients puts much pressure on medical staff and public health services (9, 10), and many patients have to take care of themselves. Therefore, the evaluation of ADL is a necessary part of clinical management. A quick assessment model of patients' prognosis is also required to stratify the patients and identify those at high risk of death, in order to promote the allocation of medical resources and reduce mortality and the burden on the medical system and society.
Some factors have been reported to be able to indicate the prognosis of patients, such as various routine laboratory parameters, cytokines, and features of lung computed tomography (CT) scans (11–16); great prediction models based on these factors have been developed, like the ISARIC score (17). However, these models are complicated, depend on additional clinical tests, and require further calculations. Given the large number of patients, a simple evaluation method that does not require any complex or expensive clinical trials would be valuable. ADL, which can effectively reflect patients' functional state by assessing their self-care ability, is simple to measure and practical. Notably, impaired ADL is shown to be a risk factor for death in COVID-19 patients (18), but its association with COVID-19 patients' prognosis has not been well-elucidated. Thus, a quick and concise prediction method based on it is needed.
The Barthel Index has been proposed as a prevalent tool for evaluating patients' ADL due to its reliability, sensitivity, and utility (19–21). It assesses patients' state in ten representative aspects of self-care ability, and has been widely utilized for clinical practices like predicting physical function alterations of geriatric rehabilitation patients (22), survival time of older patients (23), and functional recovery of stroke patients (24, 25). Whether it can be used as a prognostic predictor for COVID-19 has been rarely discussed. This study aims to evaluate the connection between ADL and COVID-19, and explore the value of the Barthel Index in predicting the prognosis of patients with COVID-19, further providing evidence for clinical treatment and management.
This retrospective cross-sectional study of COVID-19 collected simple health care clinical records of patients and intended to develop a simple evaluation method to predict the prognosis of the patients without the aid of any complex or expensive clinical tests. It adopted a convenient sampling method and included patients with COVID-19 who were hospitalized in a tertiary hospital in Wuhan from January 2020 to February 2020. Patients were divided into discharged or deceased groups according to their outcomes.
A total of 413 patients were retrospectively surveyed, while 398 were included in the final study. The inclusion criteria included: (1) patients diagnosed with COVID-19 by symptoms, polymerase chain reaction test, and other clinical examinations; (2) patients who completed the Barthel Index test. The exclusion criteria included: (1) patients who were younger than 18 years (n = 12); (2) patients who had serious organic diseases or lost self-care ability before onset (n = 3). The ethical approval of this study was waived by the local Ethics Committee of the Union Hospital, Tongji Medical College, Huazhong University of Science and Technology, in view of the retrospective nature of the study and that all the procedures being performed did not interfere with routine care.
The sample size was calculated as follows. For regression analysis, the sample size should be 5–10 times the number of variables. All variables should be obtained directly and simply from the health care clinical records at admission without the use of other tools. Finally, 12 variables were collected. Therefore, at least 60 samples were required for both the discharged and the deceased groups. Meanwhile, the overall mortality in the early stages of the pandemic was 16.4% (26) to 20.3% (27). We took 18% as the estimated mortality, thus the total samples should be at least 333. In our study, 413 patients met the inclusion criteria and 15 patients were excluded. A final total of 398 patients were analyzed.
Individual assessments of the index were conducted by a trained team, strictly according to the standard of the index. The ADL of the patients in the present study were assessed on the day of admission to the hospital by Barthel Index, which includes feeding, bathing, grooming, dressing, bowel control, bladder control, toileting, chair transfer, ambulation, and stair climbing (28). Each item was divided into different grades of 15 (10), 5, and 0, depending on how much help they needed to complete the relevant items. Zero points meant patients could not do the relevant item independently and were completely reliant on help from others, while 15 (10) indicated that they did not need any help from others. Patients who needed a little or more help to complete the activities would be given scores somewhere between 0 and 15 (10). Then, the score of each item was added to get the final score, which had a range of 0–100. One hundred meant patients could take care of themselves without any help, while 0 meant they could not live without help from others. Finally, patients were given different scores to show their ability to perform daily activities.
Other information, including gender, age, temperature, pulse, respiratory rate, mean arterial pressure, oxygen saturation (SPO2), whether or not they could walk to the hospital, and total hospital days, were collected from patients to explore their association with the patients' prognosis as well. Additionally, the Braden score and Caprini score, which are usually used to assess the risk of pressure ulcers and venous thrombus embolism, were also recorded. All these variables reflected the patients' physical condition before receiving any treatment.
Firstly, in the analysis of the demographic characteristics, normality was tested using the Shapiro-Wilk test, and then, based on the result, variables were presented as median (25–75% interquartile range) or frequency (proportion). Differences of the continued variables between the discharged and the deceased were analyzed by t-test or Mann–Whitney U test, while differences of categorical variables were analyzed by chi-square test. Then, univariate logistic regression analysis was applied to test the correlation between the clinical characteristics and patient outcomes. As the minimum unit of measurement of the Barthel Index is 5, logistic regression analysis also explored the association between the index (5 points) and prognosis. The dose-effect relationship between Barthel Index and death risk was analyzed by the linear trend of the chi-square test. Moreover, the 100-Barthel Index was utilized to draw the receiver operating characteristic (ROC) curve to effectively show the relationship between the index and the prognosis of the patients. The area under the curve (AUC) was also measured and compared with the baseline area (0.5) to verify whether it was significantly different.
Additionally, in order to test the independent predictive ability of the Barthel Index, four regression models were constructed: (1) model 1 was adjusted for age and gender; (2) model 2 additionally included Braden score and Caprini score; (3) model 3 further included respiratory rate, SPO2, and whether or not patients could walk to the hospital; and (4) for model 4, the analysis was further adjusted for temperature, pulse, and mean arterial pressure. The added covariates were selected from the confounding factors, which were clinically associated with the prognosis of patients or statistically associated in univariate analysis (p < 0.05). Finally, a decision tree model was constructed by the method of split sample verification. All variables could be obtained from the health care clinical records, so there were no missing data to be addressed. SPSS version 25.0 (IBM) was used to analyze the data and Adobe Illustrator CC 2018 (Adobe Inc., Mountain View, CA, USA) was used to draw the figures. All P-values were two-tailed and P < 0.05 was considered statistically significant.
In total, 413 patients were retrospectively reviewed. After excluding patients who were younger than 18 years (n = 12) and those who had serious organic diseases before onset (n = 3), the final study included 398 patients, consisting of 331 discharged patients and 211 male patients (Figure 1). The participants ranged from 20 to 96 years old, and the mean age was 58 years old (Supplementary Figure 1). Overall, 25.6% of patients could not walk to the hospital and half of the patients had an oxygen saturation higher than 95%. More information about the patients' characteristics are shown in Table 1. Notably, the median score of the Barthel Index in the discharged group (90) was higher than that in the deceased group (65), and the distributions of the Barthel Index in the two groups were dissimilar (Figure 2). In fact, the Barthel Index was significantly different (P < 0.001) between the discharged (mean rank = 218.64) and the deceased (mean rank = 111.93) groups.
Figure 1. A graphical abstract of the study. The Barthel Index is significantly associated with the prognosis of COVID-19 patients, and a three-group classification scheme based on the index has been developed to facilitate disease management.
Figure 2. The distribution of the Barthel Index in the deceased and the discharged patients. The histogram shows the obvious difference in the distribution of the Barthel Index between the deceased and discharged patients. Deceased patients are presented on the (Right), while discharged patients are presented on the (Left).
We conducted logistic regression analysis to figure out the association between the prognosis and the diverse factors. Eligible factors (P < 0.05) included gender, age, temperature, respiration, oxygen saturation, whether or not patients could walk to the hospital, Barthel Index, Braden score, and Caprini score (Table 2). Among these factors, the Barthel Index was a potential indicator for its efficiency (per 5-point, OR = 0.817, 95%CI = 0.771–0.866, P < 0.001). In addition, the dose-effect relationship between the Barthel Index and the mortality risk of COVID-19 patients was analyzed, showing that a decrease in the Barthel Index indicated an increased risk of mortality (P < 0.001, by linear trend of the chi-square test).
Table 2. Univariate logistic regression analysis of the association between the factors and the prognosis of the patients.
To verify whether the Barthel Index could independently predict prognosis, four regression models were constructed by multivariable logistic regression. Model 1 showed the Barthel Index had a significant association with the outcome (Table 3, OR = 0.816, 95%CI: 0.763–0.873). Adjusting for Braden score and Caprini score (model 2), it remained a significant indicator for COVID-19 (OR = 0.814, 95%CI: 0.762–0.868). Model 3 revealed that including more factors only slightly weakened the association between the Barthel Index and the prognosis (OR = 0.825, 95%CI: 0.768–0.885). The consequences of model 4 (OR = 0.809, 95%CI: 0.750–0.872) showed that the Barthel Index was still a positive factor for survival and could be an independent indicator. Additionally, other independent predictors were also found in model 4, which included gender, temperature, pulse, and SPO2.
Table 3. Logistic regression models for Barthel Index as a predictor for the prognosis of COVID-19 patients.
The Barthel Index and the outcomes of the patients were then used to draw the ROC curve (Figure 3). As a result, the AUC of the Barthel Index was 0.764 (95%CI: 0.701–0.827). When the Youden Index was maximum, the value of the Barthel Index was 76.5 and the sensitivity and the specificity were 0.740 and 0.687 (P < 0.001), respectively. To further improve the prediction efficiency, a prognostic prediction model was constructed by decision tree. By means of the split sample verification, all the subjects were randomly divided into training samples (Figure 4A) and test samples (Figure 4B). The training samples showed that patients whose Barthel Index were below 70 had a high risk of death, while people whose scores were above 90 were more likely discharged (mortality rates: 33.3 vs. 7.2%). The conclusion was still effective in the test samples (mortality rates: 40.0 vs. 2.7%).
Figure 3. ROC curve of the Barthel Index in patients with COVID-19. ROC curve indicates the sensitivity and specificity of the Barthel Index in predicting the prognosis of patients with COVID-19. The AUC was 0.764 (95%CI: 0.701–0.827).
Figure 4. The training samples and the test samples of the decision tree. By means of the split sample verification, the entire study population is divided into the training samples (n = 197) and the test samples (n = 201). (A) The training samples of the decision tree are divided into three groups which had different mortality. (B) The divided groups in test samples show similar mortality with the corresponding groups in the training samples.
This cross-sectional study aimed to develop a rapid evaluation method that did not require any complex or expensive clinical trials to predict the prognosis of COVID-19 patients. In this study, we observed that the Barthel Index was significantly associated with the prognosis of COVID-19 patients and we developed a potential prediction model based on the index to facilitate disease management.
As a serious epidemic, COVID-19 impairs body function, can cause serious sequelae (28), and affects patients' ADL. ADL is closely associated with a patient's quality of life and has been used to evaluate the development of diseases like stroke (29). There are several tools to measure patients' ADL (30) and the Barthel Index, which estimates 10 items concerning functional state, is a simple and widely utilized one in, for instance, patients with neuromuscular or musculoskeletal disorders (28, 31). Notably, it has been reported that COVID-19 worsens all aspects of the Barthel Index in patients (32), and in this study the Barthel Index of deceased patients had also declined to a much lower level than the patients who were discharged. What is more, although the discharged patients also suffered from COVID-19, the Barthel Index in many of them remained at 100, which strongly indicates the index is a positive factor for the patients' survival. Thus, the Barthel Index can be of great value in disease management, and more attention should be paid to it.
Meanwhile, some other demographic characteristics of the discharged and deceased patients were also different. For instance, 79.2% of the discharged patients could walk to hospital, while only 50.7% of the deceased could do so. Notably, the univariate logistic regression analysis showed younger, female patients and those with high levels of SPO2 and low respiration rate had lower mortality. At the same time, the risks of pressure ulcers and venous thrombus embolism were lower in discharged patients than in the deceased patients, as measured by the Braden score and the Caprini score. Regarding the length of hospital admission, the discharged patients stayed in hospital much longer than the deceased (25 vs. 8 days), suggesting patients needed more time to recover and further illustrating that functional state or ADL, reflected by Barthel Index, is vital for patients. However, compared to the Barthel Index, these factors above could only partially reflect the patients' condition and were not suitable as evaluation tools for prognosis. In contrast, the Barthel Index could provide a comprehensive and effective evaluation of patients.
Furthermore, the Barthel Index showed great predictive value of prognosis by univariate logistic regression analysis, and had a negative relationship with mortality by linear trend of the chi-square test. To test whether it could be an independent indicator, different logistic regression models based on the Barthel Index were constructed, where many other factors that were clinically or statistically associated with the prognosis of COVID-19 were adjusted. Therefore, after constructing four models and finally adjusting for age, gender, Braden score, Caprini score, respiratory rate, SPO2, whether or not patients could walk to hospital, temperature, pulse, and mean arterial pressure, the Barthel Index still showed great value and could be independently utilized in the prediction of the prognosis.
Some other studies also found the Barthel Index was associated with the mortality of COVID-19 patients (33–35), but none of them offered a simple and practical evaluation method. Another study reported that the Barthel Index was not a significant predictor (P = 0.128), but that may be due to its small sample size (n = 146) (36). According to the ROC curve, the AUC of the Barthel Index was 0.764 (95%CI: 0.701–0.827), which was different from the reference (0.5), and the value of the Barthel Index was 76.5 when the Youden Index was maximum. Although the cut-off value could be used to predict prognosis, its sensitivity and specificity was not satisfactory. To further improve the prediction efficiency, a decision tree was constructed, by which we could conveniently use the Barthel Index to make a quick judgement concerning the risk of death of the patients. The decision tree established distinct classification schemes based on the Barthel Index values, and automatically compared their results to obtain the best scheme that could effectively distinguish patients with different outcomes. Meanwhile, the patients were randomly divided into training and test groups, and the classification scheme obtained from the training group was applied to the test group to verify its validity. Ultimately, in the training samples (n = 197), the high scorers (Barthel Index >90) had low mortality (7.2%) while the mortality of low scorers (Barthel Index ≤ 70) was quite high (33.3%). Moreover, the mortality of the remaining individuals (Barthel Index: 70–90) was between the two values (10.5%). Therefore, patients could be divided into three subgroups by the threshold 70 and 90: an emergency group (0–70), observation group (70–90), and normal group (90–100). Patients in the emergency group require all-round care as soon as possible, while patients in the normal group can receive routine treatment and are likely to be discharged after treatment. The observation group should be observed for longer. Applying the findings to the test samples (n = 201), we found that the model performed even better (mortality of emergency, observation and normal groups: 40.0, 16.7, and 2.7%). All in all, medical staff can quickly evaluate a COVID-19 patient's functional state with this model, and predict prognosis in order to provide them with appropriate and effective treatment.
There were other potential predictors of the patients' prognosis, including gender, age, temperature, respiratory rate, SPO2, whether or not they could walk to the hospital, Braden score, and Caprini score. However, after being adjusted in logistic regression models, only gender, temperature, pulse, and SPO2 had independent correlations with the disease. Braden score and Caprini score were also commonly featured in health care clinical records like the Barthel Index. In univariate logistic regression analysis, both were associated with the prognosis, while in the multivariate models, their association with the prognosis was not significant, indicating that other factors in the models were more relevant to prognosis. For other significant factors in model 4, they were either unstable or only partially reflected the patient's physical condition. In addition, the most frequently reported predictors of prognosis in COVID-19 patients were age, symptoms, comorbidities, and features derived from CT (15, 16, 37–40). A high proportion of severe cases and high mortality were observed in elderly COVID-19 patients (41, 42). But in our study, after being incorporated into the model with the Barthel Index, this no longer mattered (P = 0.139 vs. Barthel index: P < 0.001). Patients showed various symptoms, and some symptoms would also change over time, indicating that they were not appropriate or reliable indicators for all patients. Comorbidities or the relevant evaluation indicator, Charlson's Index, was associated with mortality (40). However, Charlson's Index was always concerned with long-term prognosis and could not provide a real-time view of a patient's functional state after being affected by COVID-19 like the Barthel Index. More importantly, the Barthel Index could more concretely reflect the effect of comorbidities on functional states and was also more accessible. Compared with the CT, the Barthel Index could be implemented on the spot and provided a quick assessment of patients, with features of comprehensiveness, test-retest, and easy execution. Some studies also showed that hypertension was a risk factor (43, 44), while our study and others indicated that after adjustment for other clinical and demographic parameters, hypertension was not an independent predictor of the prognosis (45, 46). To sum up, the Barthel Index has great predictive and practical value in the prognosis of COVID-19 patients.
This study has several advantages. First, prediction models based on clinical trials, like ISARIC score, have been well-reported, while there are few models based on care records. This study is a pioneer in demonstrating a rapid prediction model of the Barthel Index in the care records of COVID-19 patients. It uses only the information available at admission, rather than the results of other sophisticated clinical tests or tools. Moreover, the Barthel Index is easily accessible to health care workers, and its predictive value becomes even more precious when medical resources are scarce. Second, possessing a prediction for each patient in advance promotes the implementation of precision and personalized medicine to improve the therapeutic effect and the patient's feelings during treatment. Third, medical staff can distinguish critical cases from ordinary patients and treat them differently to reduce the mortality of the disease. Meanwhile, this model could reduce overtreatment of patients and also ease the burden on public health services and the economy. Therefore, this model can effectively help address this public health crisis, which affects millions of people and consumes massive public resources. Finally, the threshold of the index was determined in the training samples by the decision tree and verified in the test samples. Therefore, the conclusion was not affected by subjective factors and at the same time its practicability was proven.
On the other hand, there are still some limitations in the current study. Comparatively speaking, the index was not particularly effective in the observation group (Barthel index: 70–90), which indicates the outcomes of patients with a slight decrease in the Barthel Index are uncertain and different. Studies with larger sample sizes may help solve this problem. Secondly, the Barthel Index may begin to decline from the onset of the disease, and some studies have reported residual effects and persistent symptoms of COVID-19, such as fatigue, dyspnea, chest pain, cognitive disturbances, and a decline in quality of life after the discharge (47–49). Thus, the Barthel index should be evaluated before onset, at onset, and after rehabilitation to achieve dynamic results. By analyzing the alteration of the index, we can have a more comprehensive understanding of the disease. Thirdly, as a retrospective study, this study investigated the patients in the early stages of the pandemic, when the lethality of the disease was at its highest. The patients in our study were relatively affected and required hospitalization. Our findings were significant for them, but more studies are needed to understand the role of the Barthel Index in asymptomatic or mild cases. Additionally, there was selection bias in the process because we collected data from a single hospital and did not adopt the method of random sampling. Research involving multiple regions should be conducted in the future to verify the conclusion.
Assessed by the Barthel Index, the ADL of COVID-19 patients was significantly different between the discharged group and the deceased group. It was associated with the mortality of the patients, and the Barthel Index was a rapid and useful tool for predicting the prognosis of patients. The decision tree showed that patients with a Barthel Index below 70 had higher mortality, while those above 90 were commonly discharged after treatment. This is of value for guiding clinical management.
The datasets analyzed and generated during the current study are not publicly available due to the confidential policy of National Health Commission of China, but are available from Jun Song upon reasonable request.
The ethical approval of this study was waived by Ethics Committee of the Union Hospital, Tongji Medical College, Huazhong University of Science and Technology in view of the retrospective nature of the study.
JS, TB, and XH designed the study. AL, TB, and EW performed the data analysis. AL and TB wrote the manuscript. JS and EW revised the manuscript. ZW, XS, LZ, YJ, YM, and LZ collected the data and conducted preliminary data processing. All authors contributed to the article and approved the submitted version.
This research was funded by the scientific and technological project in Hubei Province, China, grant number 2018YFC0910502.
We would like to thank all medical workers fighting COVID-19.
The authors declare that the research was conducted in the absence of any commercial or financial relationships that could be construed as a potential conflict of interest.
All claims expressed in this article are solely those of the authors and do not necessarily represent those of their affiliated organizations, or those of the publisher, the editors and the reviewers. Any product that may be evaluated in this article, or claim that may be made by its manufacturer, is not guaranteed or endorsed by the publisher.
The Supplementary Material for this article can be found online at: https://www.frontiersin.org/articles/10.3389/fpubh.2022.978237/full#supplementary-material
Supplementary Figure 1. Age distribution of the participants in this study.
1. Arabi YM, Murthy S, Webb S. Covid-19: a novel coronavirus and a novel challenge for critical care. Intensive Care Med. (2020) 46:833–6. doi: 10.1007/s00134-020-05955-1
2. Dong E, Du H, Gardner L. An interactive web-based dashboard to track Covid-19 in real time. Lancet Infect Dis. (2020) 20:533–4. doi: 10.1016/S1473-3099(20)30120-1
3. Angell B, Sanuade O, Adetifa IMO, Okeke IN, Adamu AL, Aliyu MH, et al. Population health outcomes in Nigeria compared with other west African countries, 1998–2019: a systematic analysis for the global burden of disease study. Lancet. (2022) 399:1117–29. doi: 10.1016/S0140-6736(21)02722-7
4. World Health Organization. Who Coronavirus (Covid-19) Dashboard. Available online at: https://covid19.who.int/ (accessed June 16, 2022).
5. Struyf T, Deeks JJ, Dinnes J, Takwoingi Y, Davenport C, Leeflang MM, et al. Signs and symptoms to determine if a patient presenting in primary care or hospital outpatient settings has Covid-19. Cochrane Database Syst Rev. (2022) 5:CD013665. doi: 10.1002/14651858.CD013665.pub3
6. Modin D, Claggett B, Sindet-Pedersen C, Lassen MCH, Skaarup KG, Jensen JUS, et al. Acute Covid-19 and the incidence of ischemic stroke and acute myocardial infarction. Circulation. (2020) 142:2080–2. doi: 10.1161/CIRCULATIONAHA.120.050809
7. Mao L, Jin H, Wang M, Hu Y, Chen S, He Q, et al. Neurologic manifestations of hospitalized patients with coronavirus disease 2019 in Wuhan, China. JAMA Neurol. (2020) 77:683–90. doi: 10.1001/jamaneurol.2020.1127
8. Fisher M, Neugarten J, Bellin E, Yunes M, Stahl L, Johns TS, et al. Aki in hospitalized patients with and without Covid-19: a comparison study. J Am Soc Nephrol. (2020) 31:2145–57. doi: 10.1681/ASN.2020040509
9. Dragioti E, Tsartsalis D, Mentis M, Mantzoukas S, Gouva M. Impact of the Covid-19 pandemic on the mental health of hospital staff: an umbrella review of 44 meta-analyses. Int J Nurs Stud. (2022) 131:104272. doi: 10.1016/j.ijnurstu.2022.104272
10. Debie A, Khatri RB, Assefa Y. Successes and challenges of health systems governance towards universal health coverage and global health security: a narrative review and synthesis of the literature. Health Res Policy Syst. (2022) 20:50. doi: 10.1186/s12961-022-00858-7
11. Fabris M, Del Ben F, Sozio E, Beltrami AP, Cifu A, Bertolino G, et al. Cytokines from bench to bedside: a retrospective study identifies a definite panel of biomarkers to early assess the risk of negative outcome in Covid-19 patients. Int J Mol Sci. (2022) 23:4830. doi: 10.3390/ijms23094830
12. Krishnan A, Prichett L, Tao X, Alqahtani SA, Hamilton JP, Mezey E, et al. Abnormal liver chemistries as a predictor of Covid-19 severity and clinical outcomes in hospitalized patients. World J Gastroenterol. (2022) 28:570–87. doi: 10.3748/wjg.v28.i5.570
13. Olivieri F, Sabbatinelli J, Bonfigli AR, Sarzani R, Giordano P, Cherubini A, et al. Routine laboratory parameters, including complete blood count, predict Covid-19 in-hospital mortality in geriatric patients. Mech Ageing Dev. (2022) 204:111674. doi: 10.1016/j.mad.2022.111674
14. Shiri I, Salimi Y, Pakbin M, Hajianfar G, Avval AH, Sanaat A, et al. Covid-19 prognostic modeling using Ct radiomic features and machine learning algorithms: analysis of a multi-institutional dataset of 14,339 patients. Comput Biol Med. (2022) 145:105467. doi: 10.1016/j.compbiomed.2022.105467
15. Wynants L, Van Calster B, Collins GS, Riley RD, Heinze G, Schuit E, et al. Prediction models for diagnosis and prognosis of Covid-19: systematic review and critical appraisal. BMJ. (2020) 369:m1328. doi: 10.1136/bmj.m1328
16. Cremades-Martinez P, Parker LA, Chilet-Rosell E, Lumbreras B. Evaluation of diagnostic strategies for identifying Sars-Cov-2 infection in clinical practice: a systematic review and compliance with the standards for reporting diagnostic accuracy studies guideline (stard). Microbiol Spectr. (2022) 10:e0030022. doi: 10.1128/spectrum.00300-22
17. Knight SR, Ho A, Pius R, Buchan I, Carson G, Drake TM, et al. Risk stratification of patients admitted to hospital with Covid-19 using the isaric who clinical characterisation protocol: development and validation of the 4c mortality score. BMJ. (2020) 370:m3339. doi: 10.1136/bmj.m3339
18. Cangiano B, Fatti LM, Danesi L, Gazzano G, Croci M, Vitale G, et al. Mortality in an Italian nursing home during Covid-19 pandemic: correlation with gender, age, Adl, vitamin D supplementation, and limitations of the diagnostic tests. Aging. (2020) 12:24522–34. doi: 10.18632/aging.202307
19. Wade DT, Collin C. The Barthel Adl index: a standard measure of physical disability? Int Disabil Stud. (1988) 10:64–7. doi: 10.3109/09638288809164105
20. Martinez-Velilla N, Saez de Asteasu ML, Ramirez-Velez R, Zambom-Ferraresi F, Garcia-Hermoso A, Izquierdo M. Recovery of the decline in activities of daily living after hospitalization through an individualized exercise program: secondary analysis of a randomized clinical trial. J Gerontol A Biol Sci Med Sci. (2021) 76:1519–23. doi: 10.1093/gerona/glab032
21. Wei L, Gao F, Chen L, Li J, Zhao X, Qu N, et al. The Joint association of malnutrition and activities of daily living dependence with adverse health outcomes among patients initiating maintenance dialysis. Clin Nutr. (2022) 41:1475–82. doi: 10.1016/j.clnu.2022.05.012
22. Bouwstra H, Smit EB, Wattel EM, van der Wouden JC, Hertogh C, Terluin B, et al. Measurement properties of the barthel index in geriatric rehabilitation. J Am Med Dir Assoc. (2019) 20:420–5 e1. doi: 10.1016/j.jamda.2018.09.033
23. Ryg J, Engberg H, Anru PL, Pedersen SGH, Jorgensen MG, Vinding KL, et al. Activities of daily living at hospital admission and estimated survival time of older patients. Age Ageing. (2021) 50:1200–7. doi: 10.1093/ageing/afaa251
24. Douiri A, Grace J, Sarker SJ, Tilling K, McKevitt C, Wolfe CD, et al. Patient-specific prediction of functional recovery after stroke. Int J Stroke. (2017) 12:539–48. doi: 10.1177/1747493017706241
25. Chen WC, Hsiao MY, Wang TG. prognostic factors of functional outcome in post-acute stroke in the rehabilitation unit. J Formos Med Assoc. (2022) 121:670–8. doi: 10.1016/j.jfma.2021.07.009
26. Bennett TD, Moffitt RA, Hajagos JG, Amor B, Anand A, Bissell MM, et al. Clinical characterization and prediction of clinical severity of Sars-Cov-2 infection among US adults using data from the US national Covid cohort collaborative. JAMA Netw Open. (2021) 4:e2116901. doi: 10.1001/jamanetworkopen.2021.16901
27. Rosenthal N, Cao Z, Gundrum J, Sianis J, Safo S. Risk factors associated with in-hospital mortality in a US national sample of patients with Covid-19. JAMA Netw Open. (2020) 3:e2029058. doi: 10.1001/jamanetworkopen.2020.29058
28. Mahoney FI, Barthel DW. Functional evaluation: the Barthel Index. Md State Med J. (1965) 14:61–5. doi: 10.1037/t02366-000
29. Preston E, Ada L, Stanton R, Mahendran N, Dean CM. Prediction of independent walking in people who are nonambulatory early after stroke: a systematic review. Stroke. (2021) 52:3217–24. doi: 10.1161/STROKEAHA.120.032345
30. Cohen ME, Marino RJ. The tools of disability outcomes research functional status measures. Arch Phys Med Rehabil. (2000) 81(12 Suppl. 2):S21–9. doi: 10.1053/apmr.2000.20620
31. Alijanpour S, Mostafazdeh-Bora M, Ahmadi Ahangar A. Different stroke scales; which scale or scales should be used? Caspian J Intern Med. (2021) 12:1–21. doi: 10.22088/cjim.12.1.1
32. Trevisson-Redondo B, Lopez-Lopez D, Perez-Boal E, Marques-Sanchez P, Liebana-Presa C, Navarro-Flores E, et al. Use of the Barthel Index to assess activities of daily living before and after Sars-Covid 19 infection of institutionalized nursing home patients. Int J Environ Res Public Health. (2021) 18:2021050612. doi: 10.20944/preprints202105.0612.v1
33. Laosa O, Pedraza L, Alvarez-Bustos A, Carnicero JA, Rodriguez-Artalejo F, Rodriguez-Manas L. Rapid assessment at hospital admission of mortality risk from Covid-19: the role of functional status. J Am Med Dir Assoc. (2020) 21:1798–802 e2. doi: 10.1016/j.jamda.2020.10.002
34. Burgana Agoues A, Serra Gallego M, Hernandez Resa R, Joven Llorente B, Lloret Arabi M, Ortiz Rodriguez J, et al. Risk factors for Covid-19 morbidity and mortality in institutionalised elderly people. Int J Environ Res Public Health. (2021) 18:10221. doi: 10.3390/ijerph181910221
35. Fumagalli C, Ungar A, Rozzini R, Vannini M, Coccia F, Cesaroni G, et al. Predicting mortality risk in older hospitalized persons with Covid-19: a comparison of the Covid-19 mortality risk score with frailty and disability. J Am Med Dir Assoc. (2021) 22:1588–92 e1. doi: 10.1016/j.jamda.2021.05.028
36. Lovicu E, Faraone A, Fortini A. Admission Braden scale score as an early independent predictor of in-hospital mortality among inpatients with Covid-19: a retrospective cohort study. Worldviews Evid Based Nurs. (2021) 18:247–53. doi: 10.1111/wvn.12526
37. Li K, Fang Y, Li W, Pan C, Qin P, Zhong Y, et al. Ct image visual quantitative evaluation and clinical classification of coronavirus disease (Covid-19). Eur Radiol. (2020) 30:4407–16. doi: 10.1007/s00330-020-06817-6
38. Guan WJ, Zhong NS. Clinical characteristics of Covid-19 in China. Reply. N Engl J Med. (2020) 382:1861–2. doi: 10.1056/NEJMc2005203
39. Chung M, Bernheim A, Mei X, Zhang N, Huang M, Zeng X, et al. Ct imaging features of 2019 novel coronavirus (2019-Ncov). Radiology. (2020) 295:202–7. doi: 10.1148/radiol.2020200230
40. Sonaglioni A, Lombardo M, Albini A, Noonan DM, Re M, Cassandro R, et al. Charlson comorbidity index, neutrophil-to-lymphocyte ratio and undertreatment with renin-angiotensin-aldosterone system inhibitors predict in-hospital mortality of hospitalized Covid-19 patients during the omicron dominant period. Front Immunol. (2022) 13:958418. doi: 10.3389/fimmu.2022.958418
41. Imam Z, Odish F, Gill I, O'Connor D, Armstrong J, Vanood A, et al. Older age and comorbidity are independent mortality predictors in a large cohort of 1305 Covid-19 patients in Michigan, United States. J Intern Med. (2020) 288:469–76. doi: 10.1111/joim.13119
42. Zhou F, Yu T, Du R, Fan G, Liu Y, Liu Z, et al. Clinical course and risk factors for mortality of adult inpatients with Covid-19 in Wuhan, China: a retrospective cohort study. Lancet. (2020) 395:1054–62. doi: 10.1016/S0140-6736(20)30566-3
43. Guan WJ, Liang WH, Zhao Y, Liang HR, Chen ZS, Li YM, et al. Comorbidity and its impact on 1590 patients with Covid-19 in China: a nationwide analysis. Eur Respir J. (2020) 55:2001227. doi: 10.1183/13993003.01227-2020
44. Chen T, Wu D, Chen H, Yan W, Yang D, Chen G, et al. Clinical characteristics of 113 deceased patients with coronavirus disease 2019: retrospective study. BMJ. (2020) 368:m1091. doi: 10.1136/bmj.m1091
45. Tadic M, Cuspidi C, Mancia G, Dell'Oro R, Grassi G. Covid-19, hypertension and cardiovascular diseases: should we change the therapy? Pharmacol Res. (2020) 158:104906. doi: 10.1016/j.phrs.2020.104906
46. Wang X, Fang J, Zhu Y, Chen L, Ding F, Zhou R, et al. Clinical characteristics of non-critically ill patients with novel coronavirus infection (Covid-19) in a Fangcang hospital. Clin Microbiol Infect. (2020) 26:1063–8. doi: 10.1016/j.cmi.2020.03.032
47. Tenforde MW, Kim SS, Lindsell CJ, Billig Rose E, Shapiro NI, Files DC, et al. Symptom duration and risk factors for delayed return to usual health among outpatients with Covid-19 in a multistate health care systems network - United States, March-June 2020. MMWR Morb Mortal Wkly Rep. (2020) 69:993–8. doi: 10.15585/mmwr.mm6930e1
48. Huang C, Huang L, Wang Y, Li X, Ren L, Gu X, et al. 6-Month consequences of Covid-19 in patients discharged from hospital: a cohort study. Lancet. (2021) 397:220–32. doi: 10.1016/S0140-6736(20)32656-8
Keywords: COVID-19, Barthel Index, prognosis, activities of daily living, decision tree
Citation: Wang E, Liu A, Wang Z, Shang X, Zhang L, Jin Y, Ma Y, Zhang L, Bai T, Song J and Hou X (2023) The prognostic value of the Barthel Index for mortality in patients with COVID-19: A cross-sectional study. Front. Public Health 10:978237. doi: 10.3389/fpubh.2022.978237
Received: 25 June 2022; Accepted: 23 December 2022;
Published: 24 January 2023.
Edited by:
Chiara de Waure, University of Perugia, ItalyReviewed by:
Max Carlos Ramírez-Soto, Universidad Peruana Cayetano Heredia, PeruCopyright © 2023 Wang, Liu, Wang, Shang, Zhang, Jin, Ma, Zhang, Bai, Song and Hou. This is an open-access article distributed under the terms of the Creative Commons Attribution License (CC BY). The use, distribution or reproduction in other forums is permitted, provided the original author(s) and the copyright owner(s) are credited and that the original publication in this journal is cited, in accordance with accepted academic practice. No use, distribution or reproduction is permitted which does not comply with these terms.
*Correspondence: Tao Bai, ZHJiYWl0YW9AMTI2LmNvbQ==; Jun Song,
c29uZzExMWp1bkAxMjYuY29t
†These authors have contributed equally to this work
Disclaimer: All claims expressed in this article are solely those of the authors and do not necessarily represent those of their affiliated organizations, or those of the publisher, the editors and the reviewers. Any product that may be evaluated in this article or claim that may be made by its manufacturer is not guaranteed or endorsed by the publisher.
Research integrity at Frontiers
Learn more about the work of our research integrity team to safeguard the quality of each article we publish.