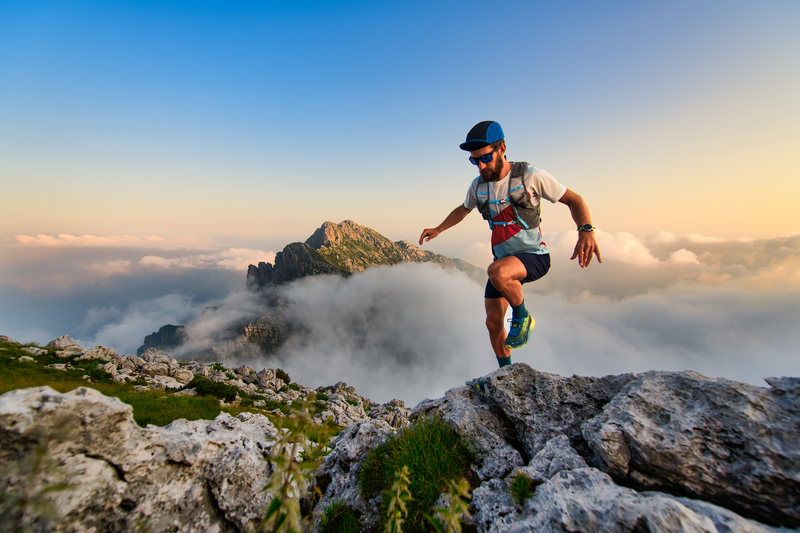
95% of researchers rate our articles as excellent or good
Learn more about the work of our research integrity team to safeguard the quality of each article we publish.
Find out more
ORIGINAL RESEARCH article
Front. Public Health , 01 August 2022
Sec. Health Economics
Volume 10 - 2022 | https://doi.org/10.3389/fpubh.2022.963234
This article is part of the Research Topic Global Excellence in Health Economics: Asia and Australasia View all 9 articles
Individuals' health status is an essential indicator of the overall strength of a country. Existing literature has studied the determinants of individuals' health, but there is no direct evidence to date on the impact of mobile payment on health. To supplement relevant research and provide insightful policy suggestions to families, government and societies, based on data of 32,058 observations from the 2017 China Household Finance Survey, we estimate the effects of mobile payment on physical health using ordinary least squares and two-stage least squares strategy. This paper provides direct evidence that mobile payment has a positive impact on citizens' physical health. Heterogeneity analysis shows that mobile payment has a more profound impact on the health of citizens who are rural and less educated. Finally, further findings in this paper suggest that commercial insurance and leisure consumption are the mechanism through which the link between mobile payment and individuals' health operates.
Citizens' health is an important issue in both developed and developing countries. Individuals' health status not only affects their happiness, labor supply, wages, and productivity, but also family income, asset allocation, and economic well-being (1–5). Therefore, research on the determinants of health has long been a focus of attention in industry and academia. From a macro perspective, urbanization (6), environmental pollution (7), and international trade (8) may affect the health of a country's residents. In addition, other factors, such as the financial crisis (9), minimum wage (10), skilled migration (11), the level of national corruption (12), and extreme weather (13) may also exert an impact on their health. From a micro perspective, personal religious beliefs (14), social capital (15, 16), self-employment (17), physical exercise (18), unemployment (19), and income (20) can affect residents' health.
In recent decades, the emergence of the internet and the digital economy has changed human lifestyles and social behaviors. These changes may have an impact on people's health. Against this background, research on the determinants of health should focus not only on traditional perspectives but should also take internet use into account (21, 22). The development of the internet, which has enabled mobile payment, online shopping, and online ordering, makes daily life more convenient and information more accessible. In particular, mobile payment has been incorporated into people's way of life and has taken off in recent years. On the one hand, mobile payment has facilitated private consumption, while on the other it has enabled better access to social services, provident funds, medical services, transportation and other government services. Generally speaking, mobile payment is gradually replacing traditional cash payment methods and becoming an important way of carrying out transactions.
To date, there has not yet been an empirical study that examines the direct link between mobile payment and people's physical health. An urgent need for academic evidence on the question of whether mobile payment has an impact on health is raised. As the abovementioned studies indicate, mobile payment could have an impact on individuals' health through several channels. First, the use of mobile payment smooths consumption, which helps improve the diversity of food types in the family's daily diet and thus ensures that the individual's nutritional intake is more balanced, ultimately affecting the individual's health. Second, the use of mobile payment reduces transaction costs and facilitates access to online private insurance and medical services, which in turn affects the individual's health. To fill the gap in the literature on the direct link between mobile payment and individuals' health, we investigate the impact of mobile payment on residents' physical health in the case of China, using a dataset sourced from the 2017 China Household Finance Survey.
This paper focuses on China for two reasons. First, China has become the largest market in the world for mobile payment, and so it provides the best experimental sample to conduct this research. According to the “Statistical Report on China's Internet Development Status” Released by the China Internet Network Information Center, by the end of 2020, there were more than 850 million users of mobile payment in China, and the proportion of internet users using mobile payment was close to 90%. Mobile payment has brought profound impact to both society and individuals. Against the backdrop of the growing global digital economy, studying the impact of mobile payment on physical health for the case of China is of major significance.
Second, Chinese citizens prefer to go to public hospitals at their country or city to seek medical treatment, and these hospitals usually ask for a deposit before treatment. The use of mobile payment can help patients to quickly obtain loans through certain payment platforms such as WeChat and Alipay, thereby enabling patients to obtain essential medical resources in a timely fashion. Moreover, mobile payment platforms in China such as Alipay and WeChat provide convenient channels for users to purchase various private medical insurance. As an important supplement to public medical services, private insurance can substantially alleviate the financial pressure of treatment, further improve the level of medical services received during treatment, and thereby improve the health of residents.
The contributions of this paper are mainly 4-fold. First, to the best of our knowledge, this is the first paper to provide direct evidence that mobile payment could have an impact on individuals' physical health. Second, this paper enriches the related research on the digital economy by focusing on the effect of mobile payment at the micro level. Third, this paper provides evidence on the mechanisms through which mobile payment affects individuals' physical health. Finally, the results of this paper enlighten families, society, and the government by providing several policy implications against the background of COVID-19.
The remainder of this paper is structured as follows. Section 2 Data and methods describes the data source, and explains the variables and methods. The empirical results are detailed in Section 3 Empirical analysis and results. Further tests such as mechanism analyses are presented in Section 4 Mechanism. Section 5 Discussion discusss the results. Section 6 Conclusions and policy implications provides the conclusions and policy implications.
The data used in this article are drawn from the 2017 China Household Finance Survey, which uses the Probability Proportional to Size (PPS) sampling method to cover 29 provinces, including Beijing, Guangdong, Wuhan, and Sichuan, and 355 counties (districts and county-level cities), with a sample size of 40,011 households. The overall data are of high quality and are nationally representative. The survey includes not only individual-level personal information such as physical health, marital status, education, age, and gender, but also family-level information such as household size, transfer payments, insurance, and security. The total number of individuals surveyed in 2017 was approximately 127,012. After deleting variables with missing values and outliers in health status, mobile payment usage, etc., we left with 32,058 valid survey responses.
For the measurement of physical health, most studies use respondents' self-evaluation of their health (23–27), and this study does the same. The related question in the questionnaire is “How do you feel about your physical condition?” Respondents can select one of five options: “not good,” “very bad,” “fair,” “good,” and “very good,” corresponding to a score of 1–5, respectively. In our setting, the higher the score, the better the respondents' health status.
The question about mobile payment in the questionnaire is “Which of the following payment methods do you and your family generally use while shopping (including online shopping)? (Multiple choices available).” Respondents can choose from the following: (1). Cash, (2). Credit card, (3). Payment via computer (Including online banking, Alipay, etc.), (4). Payment via mobile phone, tablet, and other mobile terminals (Including Alipay APP, WeChat Pay, mobile banking, Apple pay, etc.). If the respondent's answer includes option 4, the mobile payment variable is assigned a value of 1, otherwise it is assigned a value of 0.
In order to alleviate the problem of endogeneity caused by the omitted variables, this paper includes a series of control variables; namely, gender, age, marital status, rural household registration, family size, employment status, home ownership, the family's transfer income, the economic development status of the province, and geographical factors that do not change over time, such as whether the respondent lives in the eastern, central, or western region. From the descriptive statistics shown in Table 1, it can be seen that the mean value of mobile payment is 0.339, meaning just under 34% of people use mobile payment. In addition, the mean of gender is 0.496, indicating that there is not much difference between the numbers of males and females in the sample. The mean of education is 10, which indicate that the education level of residents in China is not high enough. The mean value of agriculture is 0.235, meaning urban residents account for a large proportion in CFPS. The minimum and maximum of variables in Table 1 indicate that outlier is not a concern in our case.
First, to investigate the impact of mobile payment on health, we employ a multiple linear regression model, specified as follows:
where, Healthi denotes the health status of individual i; paymenti is a dummy variable that takes the value 1 if individual i uses mobile payment, and 0 otherwise. X is a vector of the following observable determinants of health status: gender, age, marital status, family size, a dummy for employment status, home ownership, and household transfer income. We also control for the level of economic development of the province and regional dummies for the West, Center and East. εi denotes the error term.
When analyzing the causal relationship between mobile payment and physical health, strong correlations between some explanatory variables may cause multicollinearity problems. Severe multicollinearity leads to unstable results, with a biased estimation of the causal relationship between mobile payment and health. Hence, a test must be carried out to check for multicollinearity. The results in Table 2 show that the variance inflation factor (VIF) of each variable is <3, indicating that multicollinearity is not a concern in our case (28).
Table 3 reports the results of the impact of mobile payment on health. Column (1) includes only the dummy of mobile payment, and the result is positive and statistically significant at the 1% level. We include the control variables in columns (2) and (3) without controlling for and controlling for regional fixed effects, respectively. These results show that the estimation coefficient of mobile payment is still positive and significant at the 1% level. In order to alleviate the impact of heteroskedasticity on the estimation results, column (4) reports the estimation results of clustering at the household level. The relationship between mobile payment and physical health remains statistically significant. Overall, these results indicate that mobile payment has a positive impact on health status. Our preferred estimator is in column (4), indicating that an individual who uses mobile payment has a predicted health score about 0.13 points higher than a comparable individual who does not use mobile payment. Additionally, the effects of gender, education, marital status, family size, employment status, agricultural status, home owner and economic development level have the expected signs and are statistically significant overall in our results. Specifically, gender, education, family size, employment status, home owner and economic development level have positive impact on health status. The estimated coefficients of age and agriculture are negative and significant at the 1% level, indicating that age and agriculture have a negative impact on physical health.
The results of the Ordinary Least Squares (OLS) estimation in Table 3 may be biased due to problems with endogeneity. First, omitted variable bias may occur if individuals' willingness to use mobile payment is correlated with unobserved factors that affect their physical health. Second, the effect of mobile payment on individuals' physical health may be subject to bias from reverse causality since physical health may also have a strong influence on the use of mobile payment. Third, not controlling for potential measurement errors in mobile payment and physical health may result in an upward or downward bias in the estimated effect of mobile payment on physical health. Thus, potential endogeneity issues need to be considered.
To address the endogeneity problems, we estimate the impact of mobile payment on individuals' physical health using a Two-stage Least Squares (2SLS) strategy. Similar to Bucher-Koenen and Lusardi (29), we employ exposure to mobile payment by other individuals in the same village as an instrumental variable (IV).
To confirm the validity of the IV, several relevant statistical tests have been employed. First, the value of the minimum eigenvalue statistic in the Wald test is 2203.64, which is far above the critical value (8.96), meaning that the null hypothesis that the IV is not valid can be rejected (30). Column (1) of Table 4 reports the results of first stage of the 2SLS, which indicate that the F-value is 565.45, well-above the critical value of 10 (31), and the IV has a positive effect on mobile payment. Furthermore, we employ the Limited Information Maximum Likelihood (LIML) method to study the health impact of mobile payment. The LIML results are consistent with the results of 2SLS in Table 4. These results indicate that the weak instrument problem is not a major concern in our study.
Column (2) of Table 4 reports the results of the second stage of the 2SLS, which indicate that mobile payment has a positive impact on individuals' health status. Column (3) reports the results of the LIML estimation. The estimated coefficient of the health impact of mobile payment is still positive and significant at the 1% level. These results confirm that mobile payment can be said to affect individuals' physical health even after accounting for endogeneity problems.
In order to check the robustness of our results, we use various additional tests to examine the impact of mobile payment on health. The first one is to check for self-selection bias by using the Propensity Score Matching (PSM) method. In the second, we replace the health indicator with an alternative measure. Finally, we employ a different methodology to check the robustness of our results.
Individuals' decision about whether to use mobile payment is not exogenous and random but rather is affected by external factors such as their education level, work, age, marital status, children and so on. As such, there might be an issue with self-selection. To address this potential selection bias, we employ the PSM method to estimate the health impact of mobile payment. The core idea of the PSM method is matching a suitable counterfactual control group (individuals who do not use mobile payment) with the treatment group (individuals who use mobile payment).
First, we estimate a propensity score according to the characteristics of the control variables. Second, depending on the propensity score, we decide the matching partners for each treated individual. Finally, we compute the mean of the difference between control group and treatment group, and we get the average effect of mobile payment on health level (Average Treatment Effect on Treated, ATT). The equation is as follows:
To compute the PSM estimation:
To apply PSM, the assumption of conditional independence must be satisfied. That is, given a set of observable covariatesp(X), individual health status must be independent of mobile payment. Figure 1 depicts the standardized bias. The figure shows the standardized bias of all variables is <10% after matching (we use k-nearest neighbor matching with k = 4). These results indicate that the covariates are balanced in the two groups.
In addition to the assumption of conditional independence, the assumption of common support must also be satisfied. The assumption of common support ensures that the propensity score of treatment group and control group are in the same range. Figures 2, 3 show the density distribution of the propensity score before and after matching, respectively. These two figures indicate that only a small number of observations will be lost after matching.
According to Rosenbaum and Rubin (32), there are six matching methods that could be used in this paper. They include k (k = 1, k = 4) nearest-neighbor matching, radius matching, kernel matching, local linear regression matching, and spline matching. The results in Table 5 show that the ATT of these six matching methods is positive and significant, indicating that mobile payment has a positive impact on health.
In the previous section, we employ the self-evaluation of health registered as a score from 1 to 5 to represent individuals' health status. In order to confirm the reliability of our baseline results, we use another measurement of health. Specifically, we replace the dependent variable with a dummy. This dummy takes the value 1 if the individuals' self-evaluation of their health is “good” or “very good,” and 0 otherwise. As our dependent variable is now a dummy, it allows us to use an IV-Probit model to investigate the impact of the use of mobile payment on health. The results are shown in column (1) of Table 6. The coefficient of mobile payment is positive and statistically significant at the 1% level, indicating that mobile payment has a positive effect on health.
The health status score is an ordinal variable: a higher score represents better health status. Following Hassen (33), we employ an IV-Ordered Probit model to analyze the effect of mobile payment on health. Column (2) in Table 6 reports the estimated results, indicating that mobile payment has a positive effect on health.
The results can be driven by different characteristics of individuals in the sample, thus, further analysis of such heterogeneity is conducted in this subsection. We first check the heterogeneity of the effect between rural and urban regions, and then investigate the impact of mobile payment on health across different educational levels.
Rural regions differ from urban regions in many respects, such as geographic environment, attitudes to consumption, and consumption habits. Therefore, the impact of mobile payment on the health of rural and urban residents may not be homogeneous. To study the heterogeneous effect of mobile payment on health between urban and rural individuals, we split our sample into urban and rural groups and apply the 2SLS technique to each group. The results are shown in columns (1) and (2) of Table 7. They show that the impact of mobile payment is positive and statistically significant for both rural and urban regions; however, the magnitude of the effect is greater for rural individuals than for urban ones. The results for the p-value obtained by Bootstrap sampling (1,000 times) confirm that we can reject the null hypothesis (The null hypothesis of no significant difference between rural and urban). This indicates that mobile payment has a greater impact on health for rural individuals than for urban cohorts.
The impact of mobile payment on health can vary according to education level. To examine this heterogeneous effect, we separate the sample into two different groups by years of education: a group of more-educated individuals who have completed more than 10 years of education (the mean value of our observations), and a less-educated group with fewer years. The 2SLS method is again employed to study the effect of mobile payment for these two groups. Columns (3) and (4) of Table 7 report the results, which indicate that the impact of mobile payment is positive and statistically significant for both groups. However, the magnitudes of the two coefficients show that the effect of mobile payment is greater for less-educated individuals. The results of the p-value obtained by Bootstrap sampling (1,000 times) lead us to reject the null hypothesis (The null hypothesis of no significant difference between the group of high level of education and low level of education). This indicates that mobile payment has a greater impact on health for less-educated individuals than more-educated ones.
To examine the possibility that private health insurance might be a channel through which mobile payment has an impact on health, we use two measures of private health insurance as our dependent variable. First, we create a dummy variable for private health insurance: it equals 1 if the individual purchases any private health insurance, and zero otherwise. Second, the integrated purchase level of private health insurance is also considered by using the amount of the insurance. We estimate an IV-Probit model to study the impact of mobile payment on the purchase of private health insurance. Since some people might not have any private health insurance at all and the value would be 0 for these people, we employ an IV-Tobit model to examine the impact of mobile payment on the level of private health insurance purchase. Results are shown in Table 8. The positive coefficient of mobile payment in column (1) indicates that the use of mobile payment has a positive impact on the purchase of private health insurance. Furthermore, the results in column (2) show that the effect of mobile payment on the level of private health insurance purchase is positive and statistically significant. These results suggest that the use of mobile payment might promote the purchase and the amount of private health insurance, ultimately improving individuals' health.
To study the mechanism between mobile payment and individual's physical health, we explore two lines: the total amount of leisure spending and the proportion of spending on leisure activities. The family leisure consumption includes the expenditure on books, magazines, CD, films, bars, Internet cafes, pets, amusement parks, toys, sporting equipment and tourism expenses. We add up the money spent on leisure activities including newspapers, magazines, music, films, bars, web bars, pets, amusement parks, toys, sporting equipment and tourism expenses.
There might be some zero values in our dependent variable because some families might not spend any money at all on leisure activities. Thus, to investigate the effect of mobile payment on the spending on leisure activities, we estimate an IV-Tobit model. The positive results in columns (1) and (2) of Table 9 show that the use of mobile payment has a positive impact on the proportion and amount of leisure spending, indicating that the use of mobile payment has an impact on individual health through the channel of increased spending on leisure activities.
Given the important role played by mobile payment, some studies try to understand whether mobile payment has an impact on health; to date, however, there is only indirect evidence of a relationship. For instance, mobile payment helps to reduce transaction costs, thereby improving the accessibility of financial services (34). Some mobile payment platforms (such as WeChat, Alipay, etc.) can provide small loans and alleviate individuals' credit constraints, which can effectively increase the family's use of health care services (35). At the meantime, Jack and Suri (36) found that mobile payment can smooth the consumption of food and somewhat reduce the impact of negative shocks such as unemployment or major disease. Another strand of the literature on mobile payment focuses on its possible impact on people's consumption (37) and societal welfare (38, 39). In addition, the factors that influence the use of mobile payment is another area of interest in the existing academic research (40–42). It can be concluded that previous studies have either only explored the indirect impact of mobile payment on individual's health, or examined the factors that influence the use of mobile payment.
Different from existing research, based on the data from 2017 CHFS, we estimate the effect of mobile payment on individual's physical health, providing the direct evidence of a relationship between mobile payment and individual's physical health. In research methodology, most of the previous studies relevant mobile payment do not consider the endogenous problem and selection bias. To accurately identify the impact of mobile payment on individual's physical health, this paper considers the endogeneity and selection bias of mobile payment. To address the endogeneity problems, we employ the 2SLS method to investigate the impact of mobile payment on individual's physical health. Considering the selection bias, we employ the PSM method to estimate the health impact of mobile payment. We found that mobile payment has a positive impact on individual's physical health.
Private health insurance is an essential part of the insurance industry, and it is an important supplement to the national health care system (43, 44). It can reduce individual's health care expenditure, and improve the accessibility of medical services. Furthermore, private health insurance can reduce the psychological burden caused by disease, and improve the willingness to seek medical treatment. A large number of studies have demonstrated that private health insurance has a positive impact on health (23–25). Mobile payment facilitates the purchase of private health insurance and thus reduces transaction costs. For example, WeChat and Alipay provide various kinds of private health insurance online, which reduce the search costs and transportation cost of purchasing private health insurance. The convenient process helps mobile payment users to purchase private health insurance and thus eases access to health services. Eventually, mobile payment might further improve the health of individuals by promoting the purchase of private health insurance. The results of mechanisms prove that the private health insurance is shown to be the potential mechanism through which the impact of mobile payment on individual's physical health.
Furthermore, the results of mechanisms also indicate that mobile payment have a positive impact on household consumption, which are in line with the previous research (36, 37). The results can be explained by the following reasons: the mobile payment platforms may promote some information about leisure for users, and improve the household leisure consumption. Compared to traditional physical cash, mobile payment can make the payment process more convenient (45). It can also reduce the stress of paying and increase the net utility of consumption. Consumption can be considered as an investment in health (46). Previous studies show that entertainment activities have a positive impact on health (45). In this case, the consumption on leisure items such as films, TV, magazines, amusement parks, and travel might have a positive influence on an individual's health. For these reasons, the use of mobile payment can promote the consumption of leisure activities, and further improve individuals' health status.
The limitation of this paper is as follows: first, this study does not include sophisticated theoretical framework to reveal the causal relationship between mobile payment and individual's physical health. Second, the measurement of physical health is based on the respondents' self-evaluation of health, it is very general measurement of individual's physical health. Third, individual's mental health might be related to mobile payment. Given the lack of the relevant data to mental health, we do not estimate the effect of mobile payment on individual's mental health. Finally, we investigate the short-run effects of mobile payment on individual's physical health. Regretfully, due to data constraints, we fail to consider the long-term impact of mobile payment. An interesting future research avenue could be projected on the long-term and dynamic effects of mobile payment on individual's physical health and mental health.
In this paper, we investigate the causal relationship between mobile payment and individuals' physical health using CHFS survey data from 2017. One of the most important findings to emerge from our research is that mobile payment has a positive impact on individuals' physical health. The heterogeneity analyses show that the influence of mobile payment is slightly weaker for urban residents than for rural residents, and is greater for less-educated people than for their better-educated counterparts. Our study also investigates the mechanisms through which mobile payment can have an impact on physical health, indicating that mobile payment influences physical health through its effect on the purchase of private health insurance and spending on family enjoyment.
Through the research in this article, we can draw a number of policy implications. First, against the background of the Covid-19 pandemic, which is still a threat around the world, individuals use mobile payment tools more often to carry out contactless routine transactions. By so doing, consumers can avoid direct exposure to the coronavirus, thereby reducing the possibility of contracting the virus and protecting their health. Second, Private investors related to the health industry could harness the convenience of mobile payment by making more cooperative connections with payment platforms to promote health services or by offering discounts. With the help of modern network and information technology, investors will benefit the symmetric information in the market and provide their services in low marginal costs. For consumers, it will receive the services in a lower price and increases the flexibility in product selection. Finally, the government should combine the internet, big data, cloud computing, artificial intelligence, blockchain and other technologies to vigorously support innovation by third-party payment companies and encourage them to enrich the mobile payment market and enhance the value of mobile payment. In addition, while guiding financial institutions to provide financial support for health care, the government should also strengthen the risk management of third-party payment companies in order to reduce their security risks and improve consumer protection.
The raw data supporting the conclusions of this article will be made available by the authors, without undue reservation.
Conceptualization: RZ, YZ, and JX. Methodology, software, resources, and visualization: RZ. Validation, data curation, writing—original draft preparation, supervision, and project administration: RZ and YZ. Formal analysis: RZ and JX. Investigation, writing—review and editing, and funding acquisition: YZ. All authors contributed to the article and approved the submitted version.
This research was funded by the National Social Science Foundation Youth Project (20CFX054).
We are grateful to the anonymous referees for their constructive and valuable comments and suggestions that helped us to greatly improve the quality of this paper. We would like to thank Camelia Turcu, Bing Jia, Zhe Dai, and Wenxuan Tan for their helpful remarks.
The authors declare that the research was conducted in the absence of any commercial or financial relationships that could be construed as a potential conflict of interest.
All claims expressed in this article are solely those of the authors and do not necessarily represent those of their affiliated organizations, or those of the publisher, the editors and the reviewers. Any product that may be evaluated in this article, or claim that may be made by its manufacturer, is not guaranteed or endorsed by the publisher.
1. Schultz TP, Tansel A. Wage and labor supply effects of illness in Cote d'Ivoire and Ghana: instrumental variable estimates for days disabled. J Develop Econom. (1997) 53:251–86. doi: 10.1016/S0304-3878(97)00025-4
2. Atella V, Brunetti M, Maestas N. Household portfolio choices, health status and health care systems: a cross-country analysis based on SHARE. J Bank Fin. (2012) 36:1320–35. doi: 10.1016/j.jbankfin.2011.11.025
3. Ogundari K, Awokuse T. Human capital contribution to economic growth in Sub-Saharan Africa: does health status matter more than education?. Eco Anal Pol. (2018) 58:131–40. doi: 10.1016/j.eap.2018.02.001
4. Blundell R, Britton J, Dias MC, French E. The impact of health on labor supply near retirement. J Hum Res. (2020) 1217–9240R4. doi: 10.3368/jhr.58.3.1217-9240R4
5. Zhang R, Zhang Y, Dai Z. Impact of natural disasters on mental health: a cross-sectional study based on the 2014 China family panel survey. Int J Environ Res Pub Health. (2022) 19:2511. doi: 10.3390/ijerph19052511
6. Li X, Song J, Lin T, Dixon J, Zhang G, Ye H. Urbanization and health in China, thinking at the national, local and individual levels. Environ Health. (2016) 15:113–23. doi: 10.1186/s12940-016-0104-5
7. Delfino RJ. Epidemiologic evidence for asthma and exposure to air toxics: linkages between occupational, indoor, and community air pollution research. Environ Health Persp. (2002) 110(suppl 4):573–89. doi: 10.1289/ehp.02110s4573
8. Wang H, Zhang Y, Zhao H, Lu X, Zhang Y, Zhu W, et al. Trade-driven relocation of air pollution and health impacts in China. Na Commun. (2017) 8:1–7. doi: 10.1038/s41467-017-00918-5
9. Yilmazer T, Babiarz P, Liu F. The impact of diminished housing wealth on health in the United States: Evidence from the Great Recession. Soc Sci Med. (2015) 130:234–41. doi: 10.1016/j.socscimed.2015.02.028
10. Andreyeva E, Ukert B. The impact of the minimum wage on health. Int J Health Eco Manag. (2018) 18:337–75. doi: 10.1007/s10754-018-9237-0
11. Uprety D. Skilled migration and health outcomes in developing countries. Int J Health Eco Manag. (2019) 19:1-14. doi: 10.1007/s10754-018-9242-3
12. Achim MV, Văidean V L, Borlea SN. Corruption and health outcomes within an economic and cultural framework. Eu J Health Eco. (2020) 21:195–207. doi: 10.1007/s10198-019-01120-8
13. Weilnhammer V, Schmid J, Mittermeier I, Schreiber F, Jiang L, Pastuhovic V, et al. Extreme weather events in Europe and their health consequences–A systematic review. Int J Hyg Environ Health. (2021) 233:113688. doi: 10.1016/j.ijheh.2021.113688
14. Ransome Y. Religion, spirituality, and health: new considerations for epidemiology. Am jJ Epidemiol. (2020) 189:755–8. doi: 10.1093/aje/kwaa022
15. Hung N, Lau L. The relationship between social capital and self-rated health: a multilevel analysis based on a poverty alleviation program in the Philippines. BMC Pub Health. (2019, 19:1–13. doi: 10.1186/s12889-019-8013-5
16. Xue X, Reed W R, Menclova A. Social capital and health: a meta-analysis. J Health Eco. (2020) 72:102317. doi: 10.1016/j.jhealeco.2020.102317
17. Nikolova M. Switching to self-employment can be good for your health. J Busi Vent. (2019, 34:664–91. doi: 10.1016/j.jbusvent.2018.09.001
18. Li C, Chen JH, Liu XH, Ren SQ. Can physical exercise improve the residents' health? Front Pub Health. (2021) 9:1–12. doi: 10.3389/fpubh.2021.707292
19. Schaller J, Stevens AH. Short-run effects of job loss on health conditions, health insurance, and health care utilization. J Health Eco. (2015) 43:190–203. doi: 10.1016/j.jhealeco.2015.07.003
20. Carrieri V, Jones AM. The income–health relationship ‘beyond the mean': new evidence from biomarkers. Health Eco. (2017) 26:937–56. doi: 10.1002/hec.3372
21. Heo J, Chun S, Lee S, Lee KH, Kim J. Internet use and well-being in older adults. Cyberpsychol Behav Soc Network. (2015) 18:268–72. doi: 10.1089/cyber.2014.0549
22. Cohall AT, Nye A, Moon-Howard J, Kukafka R, Dye B, Vaughan RD, et al. Computer use, internet access, and online health searching among Harlem adults. Am J Health Promot. (2011) 25:325–33. doi: 10.4278/ajhp.090325-QUAN-121
23. Dor A, Sudano J, Baker DW. The effect of private insurance on the health of older, working age adults: evidence from the health and retirement study. Health Serv Res. (2006) 41:759–87. doi: 10.1111/j.1475-6773.2006.00513.x
24. Hadley J, Waidmann T. Health insurance and health at age 65: implications for medical care spending on new Medicare beneficiaries. Health Serv Res. (2006) 41:429–51. doi: 10.1111/j.1475-6773.2005.00491.x
25. Hullegie P, Klein TJ. The effect of private health insurance on medical care utilization and self-assessed health in Germany. Health Eco. (2010) 19:1048–62. doi: 10.1002/hec.1642
26. Imlach Gunasekara F, Carter KN, Crampton P, Blakely T. Income and individual deprivation as predictors of health over time. Int J Public Health. (2013) 58:501–11. doi: 10.1007/s00038-013-0450-9
27. Kim S, Koh K. The effects of income on health: evidence from lottery wins in Singapore. J Health Econ. (2021) 76:102414. doi: 10.1016/j.jhealeco.2020.102414
29. Bucher-Koenen T, Lusardi A. Financial literacy and retirement planning in Germany. J Pens Eco Fin. (2011) 10:565–84. doi: 10.1017/S1474747211000485
30. Cragg JG, Donald SG. Testing identifiability and specification in instrumental variable models. Econom Theory. (1993) 9:222–40. doi: 10.1017/S0266466600007519
31. Andrews DW, Stock JH. (Eds) Identification and Inference for Econometric Models: Essays in Honor of Thomas Rothenberg. Cambridge: Cambridge University Press. (2005). doi: 10.1017/CBO9780511614491
32. Rosenbaum PR, Rubin DB. The central role of the propensity score in observational studies for causal effects. Biometrika. (1983) 70:41–55. doi: 10.1093/biomet/70.1.41
33. Hassen S. The effect of farmyard manure on the continued and discontinued use of inorganic fertilizer in Ethiopia: an ordered probit analysis. Land use policy. (2018) 72:523–32. doi: 10.1016/j.landusepol.2018.01.002
34. Suri T, Jack W. The long-run poverty and gender impacts of mobile money. Science. (2016). 354:1288–92. doi: 10.1126/science.aah5309
35. Dupas P. What matters (and what does not) in households' decision to invest in malaria prevention?. Am Eco Rev. (2009) 99:224–30. doi: 10.1257/aer.99.2.224
36. Jack W, Suri T. Risk sharing and transactions costs: evidence from Kenya's mobile money revolution. Am Eco Rev. (2014) 104:183–223. doi: 10.1257/aer.104.1.183
37. Soman D. The effect of payment transparency on consumption: quasi-experiments from the field. Mark Lett. (2003) 14:173–83. doi: 10.1023/A:1027444717586
38. Humphrey DB, Kim M, Vale B. Realizing the gains from electronic payments: Costs, pricing, and payment choice. J Money Credit Bank. (2001) 33:216–34. doi: 10.2307/2673882
39. Von Kalckreuth U, Schmidt T, Stix H. Choosing and using payment instruments: evidence from German microdata. Emp Eco. (2014) 46:1019–55. doi: 10.1007/s00181-013-0708-3
40. Dahlberg T, Mallat N, Ondrus J, Zmijewska A. Past, present, and future of mobile payments research: a literature review. Elec Comm Res App. (2008) 7:165–81. doi: 10.1016/j.elerap.2007.02.001
41. Kim C, Mirusmonov M, Lee I. An empirical examination of factors influencing the intention to use mobile payment. Comp Human Behav. (2010) 26:310–22. doi: 10.1016/j.chb.2009.10.013
42. Zhou T. An empirical examination of continuance intention of mobile payment services. Decis Support Syst. (2013) 54:1085–91. doi: 10.1016/j.dss.2012.10.034
43. Propper C. An econometric analysis of the demand for private health insurance in England and Wales. App Eco. (1989) 21:777–92. doi: 10.1080/758520273
44. Drechsler D, Jutting J. Different countries, different needs: the role of private health insurance in developing countries. J Health Polit Pol Law. (2007) 32:497–534. doi: 10.1215/03616878-2007-012
45. Coleman D. Leisure based social support, leisure dispositions and health. J Leis Res. (1993) 25:350–61. doi: 10.1080/00222216.1993.11969933
Keywords: mobile payment, physical health, commercial insurance, leisure consumption, heterogeneity analysis, mechanism
Citation: Zhang R, Zhang Y and Xia J (2022) Impact of mobile payment on physical health: Evidence from the 2017 China household finance survey. Front. Public Health 10:963234. doi: 10.3389/fpubh.2022.963234
Received: 07 June 2022; Accepted: 15 July 2022;
Published: 01 August 2022.
Edited by:
Mihajlo Jakovljevic, Hosei University, JapanReviewed by:
Shaobin Wang, Institute of Geographic Sciences and Natural Resources Research, ChinaCopyright © 2022 Zhang, Zhang and Xia. This is an open-access article distributed under the terms of the Creative Commons Attribution License (CC BY). The use, distribution or reproduction in other forums is permitted, provided the original author(s) and the copyright owner(s) are credited and that the original publication in this journal is cited, in accordance with accepted academic practice. No use, distribution or reproduction is permitted which does not comply with these terms.
*Correspondence: Yunzhi Zhang, eXVuemhpLnpoYW5nQGhvdG1haWwuY29t
Disclaimer: All claims expressed in this article are solely those of the authors and do not necessarily represent those of their affiliated organizations, or those of the publisher, the editors and the reviewers. Any product that may be evaluated in this article or claim that may be made by its manufacturer is not guaranteed or endorsed by the publisher.
Research integrity at Frontiers
Learn more about the work of our research integrity team to safeguard the quality of each article we publish.