- 1China Institute for Urban Governance, Shanghai Jiao Tong University, Shanghai, China
- 2School of International and Public Affairs, Shanghai Jiao Tong University, Shanghai, China
- 3School of Media and Communication, Shanghai Jiao Tong University, Shanghai, China
The internet use intensity of human has increased substantially during the COVID-19 Pandemic, and it is severely impacting the well-being of chronic patients. This study aimed to explore the underlying mechanism of the relationship between internet use intensity and quality of life in chronic patients, based on the cross-sectional data from China Family Panel Studies (CFPS) during the COVID-19 Pandemic in 2020. The results showed that the internet use intensity had significant positive association with quality of life among chronic patients, and such association has been found in both urban and rural samples. Among the relationship of internet use intensity and quality of life in chronic patients, the mediating effect of physical exercise reached 10.25%. Furthermore, health insurance positively moderated this relationship. There are new insights for policy recommendations and clinical guidance on the role of physical activity and health insurance aimed at improving chronic patients' quality of life. Meanwhile, in both rural and urban governance, public health agencies should promote the “Internet + Healthcare” program to improve health insurance and physical activity literacy, thus providing a higher level of quality of life for patients with chronic diseases during the COVID-19 Pandemic.
Introduction
Since the outbreak of the COVID-19 Pandemic in China at the end of 2019, the pandemic has become a global public health event with a continuous impact on social development and people's daily life and health (1, 2). Numerous studies have pointed out that people experienced significantly lower quality of life and more mental health issues during the COVID-19 pandemic (3–5). As a vulnerable group, the quality of life of patients with chronic diseases deserves special attention. Currently, studies show that chronic diseases which contain cardiovascular diseases, cancer, chronic respiratory diseases, and diabetes are the largest cause of death globally (6, 7). As the population aging dramatically, the number of patients with chronic diseases substantially increases in China, and China faces a higher mortality rate than developed countries (8, 9). The previous study showed that chronic patients reported lower levels of quality of life during the COVID-19 Pandemic (10), such as drop-in emotional functioning and social functioning, and severe psychological problems (11). Therefore, it's essential to explore the factors influencing quality of life among chronic patients.
According to the World Health Organization (WHO), quality of life refers to “an individual's perception of their position in the life in the context of the culture in which they live and in relation to their goals, expectations, standards, and concerns” (12). The appraisal of quality of life is subjective, however, it is also affected by many objective factors, among which internet use is a significant factor. The use of digital technology has increased dramatically after the outbreak of COVID-19, up to 90% of the Chinese participants reported longer screen time for study, work, and entertainment (13–15). Many studies have shown that internet use was positively associated with quality of life from different aspects, such as decreasing loneliness (16), improving social relationships and personal well-being (17), and enhancing physical and mental health (18, 19). A study showed that moderate amounts of time spent on online activities are beneficial for enhancing the level of quality of life (19). However, some studies hold different views. The prevalence of internet addiction among vulnerable people in China has increased during the pandemic (20). While more time spent on the internet might increase social isolation and loss of contact with the social environment (21). This means that excessive use of the internet has a negative effect on the quality of life (22, 23). Thus, the relationship between internet use intensity and quality of life is still unclear, and its underlying mechanism is still to be confirmed during COVID-19.
Although a large number of studies have shown that internet use intensity was associated with quality of life, few studies have explored its underlying mediating mechanisms. One study pointed out that physical exercise mediated the relationship between internet use and mental health (24). The internet provides a favorable tool for physical exercises, such as searching for exercise information, using exercise apps (25), providing exercise guidance (26), self-monitoring (27), and transferring exercise data (28). Physical exercise has long been used as a means of rehabilitation for chronic patients. Some study have shown that internet-based physical activity is an effective way for quality of life improvement (29). Meanwhile, physical exercise has a positive effect on the quality of life among patients with chronic conditions, such as chronic brain disorders, chronic liver disease, and type 2 diabetes (30–32). As a significant intervention for patients with chronic diseases, physical exercise plays an irreplaceable role in optimizing bodily functioning (33), reducing morbidity and mortality (34), and improving patients' quality of life. In conclusion, internet use intensity is positively associated with physical exercise, and physical exercise predicts quality of life. Therefore, it can be assumed that physical exercise plays a mediating role in the relationship between internet use intensity and quality of life.
Health insurance has not to be sufficiently considered as a potential moderator for quality of life. The benefits of health insurance improved health-related outcomes in chronic patients (35). Ronksley et al. demonstrate that chronic conditions and distress were significantly related to unsatisfied healthcare needs (36). At the same time, the inclusion of chronic disease drugs in medical insurance reimbursement will benefit patients (37). With the promotion of China's “Internet +Healthcare” program, the internet provides a convenient way for medical consultation, treatment, and health insurance reimbursement. One study pointed that the higher the frequency of internet usage, the more likely Chinese households are to participate in private insurance (38). And patients who had private reimbursement insurance reported a higher quality of life than those with public insurance (39). Besides, some studies revealed that individuals who have health insurance reported a higher quality of life than those with no health insurance (40, 41). Hence, health insurance may moderate the association between internet use intensity and quality of life.
Taken together, the main goal of the current study is to explore the impact mechanism of internet use intensity on the quality of life of chronic patients during the COVID-19 pandemic. In order to improve the quality of life of chronic patients, policy recommendations and clinical guidance will be provided. There were the following hypotheses: (H1) There will be a positive relationship between internet use intensity and quality of life. (H2) Physical exercise mediates the relationship between internet use intensity and quality of life. (H3) Health insurance plays a moderating role in the relationship between internet use intensity and quality of life (see Figure 1).
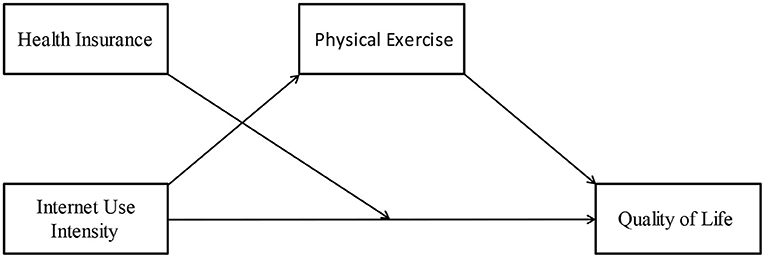
Figure 1. The relationship among internet use intensity, quality of life, physical exercise, and health insurance.
Methods
Data and participants
The current study obtains the dataset from China Family Panel Studies (CFPS), funded by Peking University and carried out by the Institute of Social Science Survey of Peking University. It covers 25 provinces/municipalities/autonomous regions, and was officially launched in 2010 with a target sample size of 16,000 households. The CFPS sample is a multi-stage probability sample drawn using the implicit stratification method, and each subsample is drawn in three stages. The first two stages of the sampling process use official administrative division information. This is because the administrative division structure of mainland China is strictly hierarchical and covers the entire population of mainland China. The third stage was to create end sampling frames in the selected sample villages/residences using the list of households obtained from the village survey map, and to draw a sample of households by expanding the sample size according to circular equidistant sampling with a random starting point to ensure that each sample village/residences could fulfill the target of 25 households. The 2020 wave of CFPS was conducted from July to December 2020 during the COVID-19 Pandemic and contained 28,590 individuals. The target population of this study was respondents with chronic diseases, and a final sample of 3,313 cases with chronic diseases was used after screening and cleaning invalid samples. Among them, 1,777 (53.64%) were female and 1,536 (46.36%) were male; 1,582 (47.75%) were rural residents while 1,731 (52.25%) were urban residents.
Variables
Dependent variable
Quality of Life. Since Flanagan developed the Quality of Life Scale (QOLS) in 1978 (42), measuring quality of life in terms of five dimensions has been accepted in many studies (43–45). The five dimensions of the QOLS include physical and material well-being; relationships with other people; social, community and civic activities; personal development and fulfillment; and recreation. Based on these five dimensions, this study selected 14 approximate question items to measure the quality of life from CFPS 2020, namely: (1) have health insurance (From the question “What health insurance do you have?”); (2) have high local income (From the question “How would you rate your income in your local area?”); (3) have good health (From the question “How do you consider yourself to be in good health?”); (4) have good interpersonal relationships (From “How well-connected do you think you are?”); (5) have a sense of subjective well-being (From the question “How happy do you think you are?”); (6) post comments related to political issues and national events (From the question “In the past 12 months, have you made any statements on your website related to political issues and national issues?”); (7) vote in village/neighborhood council elections (From the question “In the last 5 years, have you voted in village/neighborhood council elections?”); (8) read books in the past year (From the question “In the past 12 months, excluding reading for work and exams, have you read any books?”); (9) have high life satisfaction (From the question “How satisfied do you rate yourself with your life? “); (10) have confidence in the future (From the question “What is your level of confidence in your future?”); (11) think the living standard is likely to improve (From the question “In today's society, there are still many opportunities for people like me to improve their living standards”); (12) play online games (From the question “In the past week, have you played online games?”); (13) spend time watching TV, movies and other video programs (From the question “In general, how many hours per week do you spend watching TV, movies and other video programs by any means?”); (14) share my work or life in social media frequently (From the question “In the past year, how often did you share your work or life in your WeChat Friend Circle?”). For each of the above question items, respondents were coded “1” if they exceeded the median of the sample, otherwise they were coded “0.” Finally, the coded answers of the 14 questions were summed to obtain a score between 0 and 14, with higher values indicating a higher quality of life.
Independent variable
Internet Use Intensity. According to the related studies (46–49), time spent online is measured by two questions: “In general, how long do you spend online with your mobile devices each day?” and “In general, how long do you spend online with the internet each day?”. The total time spent online using computers and mobile devices was summed by hours per day to measure the internet use intensity of the respondents. The more time spent on the internet per day, the higher the internet use intensity of the respondents.
Mediating variable
Physical Exercise. Physical activity was measured with a single-item question that asked “How often did you participate in physical fitness and leisure activities in the past 12 months?” that ranged from 1 (never participate) to 8 (twice a day or more). The higher the coded value, the more frequent the physical activity participation.
Moderating variable
Health Insurance. In CFPS 2020, respondents were asked about their health insurance coverage by one question “What health insurance coverage do you have?”. According to the respondent's participation in health insurance, “have at least one type of health insurance” was coded as “1”; “none of health insurance” was coded as “0.”
Control variables
Based on previous studies (50–52), socio-demographic characteristics, residential status, health behavior, and economic status were selected as control variables. Socio-demographic characteristics included gender (male, female), age, marital status (unmarried, other), and educational experience (code “not educated” as “1,” “primary school” as “2,” “junior high school” as “3,” “high School/junior high school/technical school/vocational high school” as “4,” and “college and above” as “5”; higher coding value indicates higher education level). Residence status includes residence type (urban, rural). Health behaviors include smoking, drinking, and self-assessed health status (Likert five-degree scale; the higher the number, the healthier it is). Economic status included annual personal income (unit is 100,000 RMB) and annual medical costs (include total hospital expenses and other injury expenses; unit is 100,000 RMB).
Statistical analysis
Descriptive analyses were conducted for all variables. The effect of internet use intensity on quality of life was analyzed using the ordered logistic regression model (Model 1) and separately for the urban subsample (Model 2) and the rural subsample (Model 3). The mediating effect of physical exercise was examined using the macro PROCESS4.0 tool (53) (Model 4, Model 5, Model 6). The moderating effect of health insurance was examined by constructing an interaction of health insurance × internet use intensity via ordered logistic regression model (Model 7). Robustness tests were conducted by recoding the independent and dependent variables and selecting urban and rural subsamples using OLS (Model 8, Model 9, Model 10). All the above analyses were performed with the help of SPSS26 and Stata17.
Results
Descriptive statistics of all variables
Table 1 presents the descriptive statistics for all variables. The mean value of quality of life is 6.08 (SD, 2.03), indicating that the respondents' quality of life is low on the average, and many respondents spend close to the average amount of time using the internet. the mean value of internet use intensity is 1.41(SD, 2.80) hours per day, indicating that most of the respondents spend very little time using the internet, and only a few users spend a lot of time using the internet, with great variation between respondents. The mean value of physical exercise is 2.89 (SD, 2.60), indicating that most respondents have very little time doing physical exercise. And the mean value for health insurance was 0.93 (SD, 0.26), indicating that the majority of respondents had at least one type of insurance. In addition, the male and female samples were approximately equal in proportion, with an average age of 56.44; most samples were married, and had a low overall education level; more samples live in urban than rural but only a small number of people smoking and drinking; poor self-assessed health status, and low average annual personal income and average annual medical costs.
Correlation analysis between main variables
Table 2 shows the results of the bivariate correlation analysis using Pearson correlation for the main variables. Quality of life and internet use intensity (r = 0.099, p < 0.001), health insurance (r = 0.172, p < 0.001) and physical exercise (r = 0.149, p < 0.001) were significantly and positively correlated. It indicates that respondents with higher intensity of internet use, having health insurance and more frequent physical exercise have a higher quality of life. Physical exercise and internet use intensity (r = 0.112, p < 0.001) and health insurance (r = 0.047, p < 0.001) were significantly positively correlated. This suggests that respondents with more physical exercise and higher internet use intensity are more likely to have health insurance, but there is no association between internet use intensity and health insurance.
Internet use intensity and quality of life
In this part of the analysis, three ordered logistic regression models were constructed separately to examine the impact of internet use intensity on quality of life. Considering the large gap between rural and urban areas in China, this effect still presents in terms of internet use. Separate regression models were constructed for the rural sample and the urban sample. The total sample size is 3,313, 1,582 for the rural sample, and 1,731 for the urban sample.
Table 3 shows the regression results of internet use intensity on quality of life after excluding the effect of multicollinearity. The odds ratios (OR) of the ordered logistic regression model are reported here. The results indicate that there is a significant positive effect of internet use intensity on quality of life among respondents with chronic diseases, supporting hypothesis H1. The full sample regression of model 1 indicates that the OR of internet use intensity on quality of life is 1.078, which is statistically significant at the 1% level of significance. By increasing the internet use intensity of respondents with chronic diseases by 1 h per day, it improves the quality of life by 7.8%, holding other control variables constant. This reveals that the stronger the internet use intensity, the greater the probability of improving the quality of life for respondents with chronic diseases. For model 2, the regression results for the rural subsample show that the OR of the effect of internet use intensity on quality of life is 1.138, which is statistically significant at the 1% level of significance. This suggests that a 1 h increase in daily internet use intensity among respondents with chronic diseases in rural areas can bring about a 13.8% improvement in quality of life, with other control variables held constant. In model 3, the regression results for the urban subsample show that the OR of internet use intensity on quality of life is 1.059, which is statistically significant at the 1% level of significance. This indicates that an increase in internet use intensity of 1 h per day among chronic disease respondents in urban areas can lead to a 5.9% improvement in quality of life, when keeping other control variables constant.
It also can be found that the positive effect of internet use intensity on quality of life is strongest in the rural sample, second strongest in the overall sample, and weakest in the urban sample. The reason for this difference is that since the COVID-19 Pandemic, the number of new rural internet users far exceeds that of urban internet users (54, 55), making this effect relatively stronger. In addition, age, education level, health insurance, and self-assessed health status all had significant positive effects on quality of life.
The mediating effect test of physical exercise
Table 4 shows that physical exercise plays a mediating effect in the process of internet use intensity influencing quality of life after excluding the effect of multicollinearity. Specifically, model 4 shows that internet use intensity significantly and positively affects physical exercise (β = 0.104, p < 0.01); model 5 suggests that internet use intensity significantly and positively affects quality of life (β = 0.078, p < 0.01); model 6 indicates that internet use intensity (β = 0.07, p < 0.01) and physical exercise (β = 0.08, p < 0.01) significantly and positively affected quality of life. It suggests that physical exercise mediates the relationship between internet use intensity and quality of life. And hypothesis H2 was supported. Moreover, the physical exercise here exerts a partial mediating effect.
After confirming the mediating effect of physical exercise, this study proceeded to calculate the total effect, direct effect, and mediating effect (see Table 5). It showed that in the relationship between internet use intensity and quality of life, the mediating effect of physical exercise (0.008) accounted for 10.25% of the total effect (0.078), and 11.94% of the direct effect (0.067). This suggests that 10.25% of the positive effect of internet use intensity on quality of life was mediated by the mediating effect of physical exercise. The 95% confidence intervals do not overlap with 0, which means that they are statistically significant.
The moderating effect test of health insurance
The regression results in Table 6 indicate that health insurance positively moderates the relationship between internet use intensity and quality of life. To avoid the effect of multicollinearity, internet use intensity and health insurance were centered separately, and then the interaction (Health Insurance × Internet Use Intensity) was constructed. Internet use intensity, health insurance, and the interaction (Health Insurance × Internet Use Intensity) were placed together in Model 7 for regression analysis. The results showed that the OR of the interaction (Health Insurance × Internet Use Intensity) on quality of life was 1.085, which was statistically significant at the 5% significance level; internet use intensity (OR = 1.082, p < 0.01) and health insurance (OR = 3.360, p < 0.01) positively affected quality of life, respectively. This suggests that, holding the control variables constant, an increase of 1 h per day in internet use among respondents with chronic diseases is linked with a 36% improvement in quality of life for respondents with health insurance compared to those without health insurance. It implies that the positive relationship between internet use intensity and quality of life is stronger in the sample with health insurance than in the sample without health insurance. These results also indicate that health insurance strengthened the positive relationship between internet use intensity and quality of life. Therefore, hypothesis H3 is supported.
Robustness test
The purpose of the robustness test is to examine the stability of the regression analysis. In other words, the effect of the independent variable on the dependent variable remains stable when the variable measurement is changed, other models are used, or the sample size is changed. At present, there is no standardized robustness test, and subsample regression, reselecting the regression model, making variable substitutions, and changing the sample size are all commonly used robustness tests. We use OLS models to re-test the effect of internet use intensity on quality of life by recoding the independent and dependent variables. Specifically, the 14 question items were formed into one variable using principal component analysis to measure quality of life; the measure of internet use intensity was a categorical variable recoded in five degrees for internet use time. The newly formed internet use intensity and quality of life were then used to conduct regression analyses for the full sample (Model 8), the rural subsample (Model 9), and the urban subsample (Model 10), respectively. The results are shown in Table 7, indicating that the effect of internet use intensity on quality of life is consistent with the results in Table 3, and the results are robust and reliable.
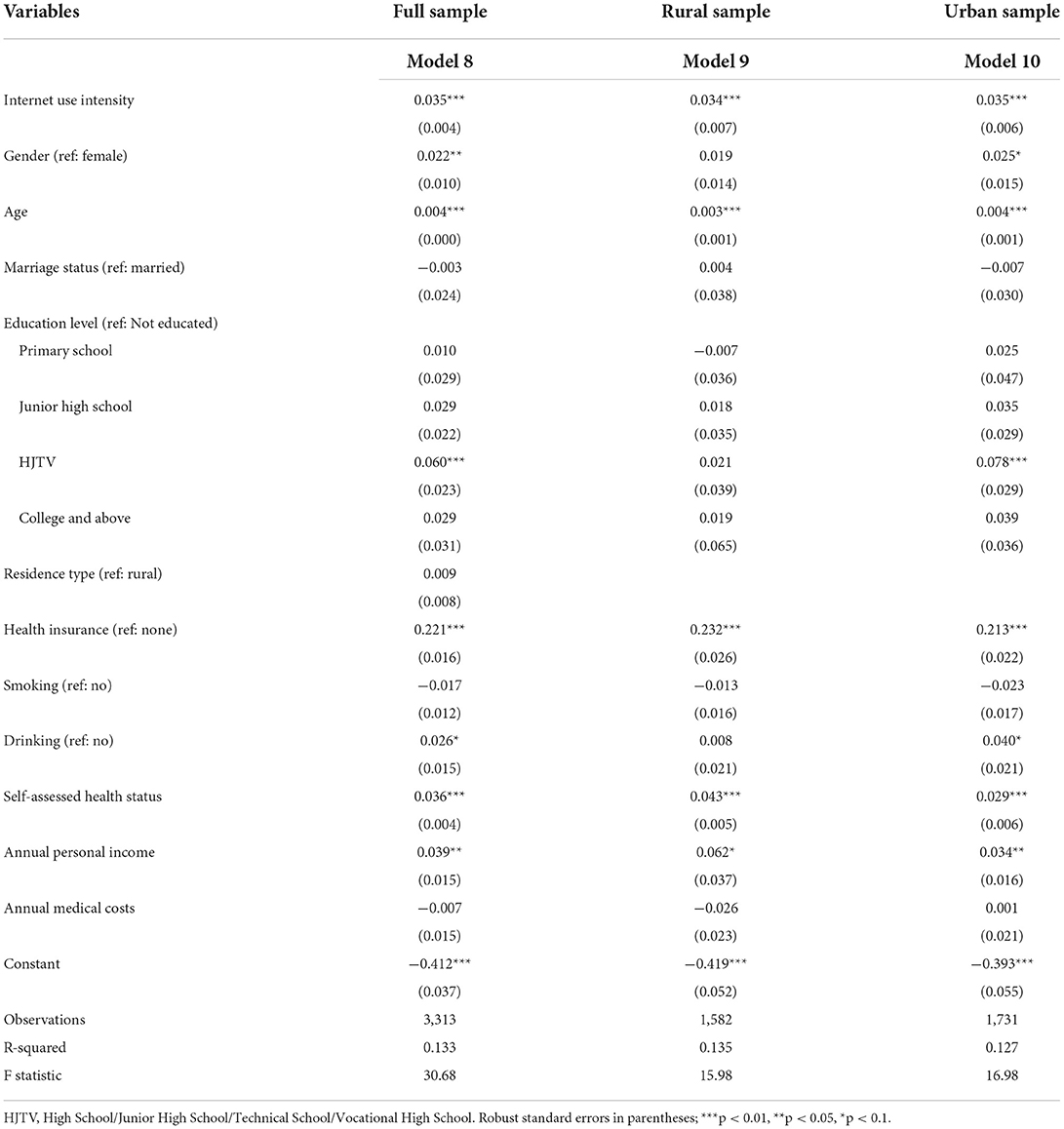
Table 7. Results of the relationship between internet use intensity and quality of life (N = 3,313).
Discussion
Chronic patients' quality of life during the COVID-19 Pandemic should be given attention. Based on the cross-sectional data from the CFPS dataset of wave 2020, the current study validated the underlying mechanism between internet use intensity and quality of life among chronic patients during the COVID-19 Pandemic in China mainland.
This study found that internet use intensity was significantly and positively related to quality of life. In other words, the more frequently individuals use the internet, the higher level of quality of life they experience, and this relationship is strongest in the rural sample, followed by the overall sample and the urban sample. However, this finding is inconsistent with previous studies that more use of the internet could reduce social communication, increase loneliness (56), and enhance sedentary risk (57). A likely explanation is that chronic patients could gain social, emotional, and experiential support from the internet during the lockdown. According to the uses and gratifications theory (58), users seek gratifications from the internet to fill their basic needs. The internet can not only be used for entertainment, work, study, and information seeking, but also as an important intervention tools for people to promote psychological empowerment and rehabilitation (19, 59). This indicates that providing physical activity interventions via the internet will help chronic patients improve their quality of life.
Furthermore, it revealed that physical exercise mediated the association between internet use intensity and quality of life. This finding indicates that people who use the internet more frequently are more likely to participate in physical exercise and report a higher quality of life. It is acknowledged that physical exercise is an effective way to prevent and treat chronic diseases (60, 61). Previous studies have shown that users with higher eHealth literacy are more likely to be physically active, while physical inactivity is a primary cause of most chronic diseases (33, 62, 63). The lockdown policy during the COVID-19 outbreak may lead to sedentary and reduced regular activities, and fewer visits to the hospital to prevent infection, thereby increasing health risks (64, 65). However, people who use the internet more frequently may gain more exercise-related knowledge from online social media platforms, become more accustomed to using social media apps or smart exercise tools for physical exercise, and display physical exercise achievement (66, 67). Therefore, encouraging appropriate physical activity through the internet can help improve the health conditions and quality of life of people with chronic patients.
Besides, this is the first study that revealed the moderating role of health insurance in the relationship between internet use intensity and quality of life. One possible explanation may be that China rolls out online healthcare to tackle a growing number of patients with chronic conditions (68). Patients could use online medical services during the pandemic, and online medical care was covered by Chinese health insurance (69, 70). Prior research revealed that the internet is a major source of health insurance information (71). Another study found that individuals with no medical insurance have had higher disease risk anxiety and lower life satisfaction than those who had at least one insurance (72). The “Internet +Healthcare” policy encourages people to seek medical treatment through the internet. This finding suggests that more online medical insurance policies should be improved to address the medical needs of chronic patients during the lockdown. All in all, this study enlightens us that the internet use intensity can promote quality of life in chronic patients, but its effect is limited, if the internet as an independent factor. There are two mechanisms that play the influence role. One is that physical exercise mediates the positive association between internet use and quality of life, where 10.25% of the effect is mediated by physical exercise. The other is that health insurance enhances the positive relationship between internet use intensity and quality of life.
These findings shed new light on China's healthcare policy. The internet has been used as a substitute for medical suggestions among patients who lack insurance or have difficulty accessing medical treatment (73). In the background of Chinese “Internet +Healthcare” policy, on the one hand, the internet provides online medical services which have improved the efficiency of medical treatment for chronic patients and may improve their quality of life. On the other hand, medical insurance reimbursement through the internet provides convenience for patients with chronic diseases. During the Pandemic, it's necessary to further expand the scope of beneficiaries of the “Internet +Healthcare” policy, such as the application in rural groups, simplify the process of online medical treatment and medical insurance reimbursement, so as to improve the quality of life among chronic patients.
Our study also has some clinical significance. Our findings showed that internet-based physical activity interventions have great potential to improve chronic patients' quality of life. Besides, physical exercise helps to improve the physical function of patients with chronic diseases (74). The internet provides chronic patients with health plans, physical activity testing, goal setting, feedback functions, and self-health management. Generally, internet-based physical activity provides a non-invasive way for chronic disease prevention and treatment (33). In future studies, we should further develop internet-related treatment strategies for patients with chronic diseases clinically.
It is necessary to revise Flanagan's study, which considered quality of life to include five dimensions (physical and material well-being; relationships with other people; social, community and civic activities; personal development and fulfillment; and recreation) (42), but the background in which this idea was developed was in 1978, when the Internet was not shaping human life as profoundly as it does today. It should be taken into consideration that the Internet as a factor is included in the concept of measuring quality of life. This study also responds to Link and Phelan's fundamental-causes theory, which holds that social condition is the underlying cause of health differentiation among people and has a persistent effect on health quality (75). Since the COVID-19 pandemic, the intensity of human internet use has dramatically increased, making the internet a new and important key influence on quality of life among the chronic patients. This extends the explanatory scope of the fundamental-causes theory.
Limitations
The strength of this study is reflected in several aspects. Firstly, the data we use are from CFPS of wave 2020, which is a national survey covering mainland China that has been ongoing for more than a decade, and ensures the scientific validity and representativeness of the data. Secondly, our data were conducted in the background of the COVID-19 Pandemic in mainland China in 2020, and the latest data were only allowed to be applied for use at the end of 2021, which reflects the latest situation regarding the quality of life of chronic patients. Then, to ensure the reliability of the study findings, we performed robustness tests on the data during the empirical analysis. Finally, we explored the effect mechanism of the relationship between internet use intensity and quality of life among chronic patients by introducing physical exercise (mediating variable) and health insurance (moderating variable).
However, there are some limitations of our study that should be acknowledged. Firstly, a cross-sectional survey has been used so that the causative interpretations could not be determined. Secondly, Due to the limitation of secondary data, we could only refer to the five dimensions of The Flanagan Quality of Life Scale (QOLS) with 14 approximate question items to measure the quality of life among chronic patients, which is still flawed despite validity and reliability tests. Thirdly, the present study focuses only on the internet use intensity and ignores the role of excessive internet use. To further promote chronic patients' quality of life in the future study, we can use the Flanagan Quality of Life Scale to measure quality of life, and focus on the overuse of the internet in chronic patients during the pandemic.
Conclusions
This paper empirically tested the underlying mechanism of the association between internet use intensity and quality of life among patients with chronic diseases. The findings show that internet use intensity is positively related to the quality of life directly, and indirectly through the mediating role of physical exercise and the moderating role of health insurance. Relevant policies should be developed to improve subjective and objective quality of life during the COVID-19 Pandemic. For patients with chronic diseases, the government can encourage them to use the internet in moderation and maintain physical activity to obtain a higher quality of life. In addition, in the context of universal healthcare, medical insurance should be further covered for each individual to reduce the economic pressure on chronic patients. Thirdly, the “Internet +Healthcare” program should be further promoted so that patients can get better and faster treatment during the COVID-19 Pandemic. Last but not least, even though our results showed that internet use had improved the quality of life of chronic patients, the risks of internet use should not be ignored. The frequency of people's internet usage has increased during the epidemic, so it is necessary to prevent internet dependence from adversely affecting the quality of life.
Data availability statement
The raw data supporting the conclusions of this article will be made available by the authors, without undue reservation.
Ethics statement
This study was conducted based on de-identified, publicly available CFPS data and did not interact with any individuals or use identifiable private information. Therefore, the ethics approval was waived. Written informed consent from the [patients/participants OR patients/participants legal guardian/next of kin] was not required to participate in this study in accordance with the national legislation and the institutional requirements.
Author contributions
YW: framework, model analyses, data curation, writing—original draft, and review and editing. TX: writing—original draft, review and editing, and funding acquisition. JX: supervision. All authors have read and agreed to the published version of the manuscript.
Acknowledgments
We would like to acknowledge the Institute of Social Science Survey (ISSS) of Peking University for the approval to use the wave 2020 of CFPS. We express our sincere gratitude to all subjects who participated in the survey.
Conflict of interest
The authors declare that the research was conducted in the absence of any commercial or financial relationships that could be construed as a potential conflict of interest.
Publisher's note
All claims expressed in this article are solely those of the authors and do not necessarily represent those of their affiliated organizations, or those of the publisher, the editors and the reviewers. Any product that may be evaluated in this article, or claim that may be made by its manufacturer, is not guaranteed or endorsed by the publisher.
References
1. Louvardi M, Pelekasis P, Chrousos GP, Darviri C. Mental health in chronic disease patients during the COVID-19 quarantine in Greece. Palliative Support Care. (2020) 18:394–9. doi: 10.1017/S1478951520000528
2. Qiu J, Shen B, Zhao M, Wang Z, Xie B, Xu Y. A nationwide survey of psychological distress among Chinese people in the COVID-19 epidemic: implications and policy recommendations. Gen Psychiatr. (2020) 33:e100213. doi: 10.1136/gpsych-2020-100213
3. Sayin Kasar K, Karaman E. Life in lockdown: social isolation, loneliness and quality of life in the elderly during the COVID-19 pandemic: a scoping review. Geriatr Nurs. (2021) 42:1222–9. doi: 10.1016/j.gerinurse.2021.03.010
4. Ravens-Sieberer U, Kaman A, Erhart M, Devine J, Schlack R, Otto C. Impact of the COVID-19 pandemic on quality of life and mental health in children and adolescents in Germany. Eur Child Adolesc Psychiatry. (2021) 31:879–89. doi: 10.2139/ssrn.3721508
5. Chen K-Y, Li T, Gong F-H, Zhang J-S, Li X-K. Predictors of health-related quality of life and influencing factors for COVID-19 patients, a follow-up at one month. Front Psychiatry. (2020) 11:668. doi: 10.3389/fpsyt.2020.00668
6. Beaglehole R, Ebrahim S, Reddy S, Voûte J, Leeder S. Prevention of chronic diseases: a call to action. Lancet. (2007) 370:2152–7. doi: 10.1016/S0140-6736(07)61700-0
7. Yach D, Hawkes C, Gould CL, Hofman KJ. The global burden of chronic diseases overcoming impediments to prevention and control. JAMA. (2004) 291:2616–22. doi: 10.1001/jama.291.21.2616
8. Thomas SA, Qiu Z, Chapman A, Liu S, Browning CJ. Editorial: chronic illness and ageing in China. Front Public Health. (2020) 8:104. doi: 10.3389/fpubh.2020.00104
9. Lyu J, Zhang W, Li W, Wang S, Zhang J. Epidemic of chronic diseases and the related healthy lifestyle interventions in rural areas of Shandong Province, China. BMC Public Health. (2020) 20:606. doi: 10.1186/s12889-020-08729-y
10. Algahtani FD, Hassan S-N, Alsaif B, Zrieq R. Assessment of the quality of life during COVID-19 pandemic: a cross-sectional survey from the kingdom of Saudi Arabia. Int J Environ Res Public Health. (2021) 18:847. doi: 10.3390/ijerph18030847
11. Bargon C, Batenburg M, van Stam L, van der Molen DM, van Dam I, van der Leij F, et al. The impact of the COVID-19 pandemic on quality of life, physical and psychosocial wellbeing in breast cancer patients – a prospective, multicenter cohort study. Eur J Cancer. (2020) 138:S17. doi: 10.1016/S0959-8049(20)30563-3
12. The WHOQOL Group. The World Health Organization quality of life assessment (WHOQOL): Position paper from the World Health Organization. Soc Sci Med. (1995) 41:1403–9. doi: 10.1016/0277-9536(95)00112-K
13. De' R, Pandey N, Pal A. Impact of digital surge during Covid-19 pandemic: a viewpoint on research and practice. Int J Inform Manag. (2020) 55:102171. doi: 10.1016/j.ijinfomgt.2020.102171
14. Hu Z, Lin X, Chiwanda Kaminga A, Xu H. Impact of the COVID-19 epidemic on lifestyle behaviors and their association with subjective well-being among the general population in mainland China: cross-sectional study. J Med Internet Res. (2020) 22:e21176. doi: 10.2196/21176
15. Huang Q, Chen X, Huang S, Shao T, Liao Z, Lin S, et al. Substance and Internet use during the COVID-19 pandemic in China. Transl Psychiatry. (2021) 11:1–8. doi: 10.1038/s41398-021-01614-1
16. Shaw LH, Gant LM. In defense of the internet: the relationship between internet communication and depression, loneliness, self-esteem, and perceived social support. Cyberpsychol Behav. (2002) 5:157–71. doi: 10.1089/109493102753770552
17. Castellacci F, Tveito V. Internet use and well-being: a survey and a theoretical framework. Res Policy. (2018) 47:308–25. doi: 10.1016/j.respol.2017.11.007
18. Khalaila R, Vitman-Schorr A. Internet use, social networks, loneliness, and quality of life among adults aged 50 and older: mediating and moderating effects. Qual Life Res. (2018) 27:479–89. doi: 10.1007/s11136-017-1749-4
19. Leung L, Lee PSN. Multiple determinants of life quality: the roles of internet activities, use of new media, social support, and leisure activities. Telemat Inform. (2005) 22:161–80. doi: 10.1016/j.tele.2004.04.003
20. Li Y-Y, Sun Y, Meng S-Q, Bao Y-P, Cheng J-L, Chang X-W, et al. Internet addiction increases in the general population during COVID-19: evidence from China. Am J Addict. (2021) 30:389–97. doi: 10.1111/ajad.13156
21. Nie NH, Erbring L. Internet and society: a preliminary report. Society. (2002) 1:275–83. Available online at: http://143.107.236.240/documentos/textos/cultura_digital/tics_arq_urb/internet_society%20report.pdf
22. Gao L, Gan Y, Whittal A, Lippke S. Problematic internet use and perceived quality of life: findings from a cross-sectional study investigating work-time and leisure-time internet use. Int J Environ Res Public Health. (2020) 17:4056. doi: 10.3390/ijerph17114056
23. Takahashi M, Adachi M, Nishimura T, Hirota T, Yasuda S, Kuribayashi M, et al. Prevalence of pathological and maladaptive Internet use and the association with depression and health-related quality of life in Japanese elementary and junior high school-aged children. Soc Psychiatry Psychiatr Epidemiol. (2018) 53:1349–59. doi: 10.1007/s00127-018-1605-z
24. Zhang S, Zhang Y. The relationship between internet use and mental health among older adults in China: the mediating role of physical exercise. Risk Manag Healthc Policy. (2021) 14:4697–708. doi: 10.2147/RMHP.S338183
25. Litman L, Rosen Z, Spierer D, Weinberger-Litman S, Goldschein A, Robinson J. Mobile exercise apps and increased leisure time exercise activity: a moderated mediation analysis of the role of self-efficacy and barriers. J Med Internet Res. (2015) 17:e4142. doi: 10.2196/jmir.4142
26. Huiqiang T. Analysis of fluctuation factors of healthy exercise based on machine data mining and Internet of things. Environ Technol Innov. (2021) 23:101647. doi: 10.1016/j.eti.2021.101647
27. Glasgow R, Christiansen SM, Kurz D, King DK, Woolley T, Faber AJ, et al. Engagement in a diabetes self-management website: usage patterns and generalizability of program use. J Med Internet Res. (2011) 13:e1391. doi: 10.2196/jmir.1391
28. Tate DF, Lyons EJ, Valle CG. High-tech tools for exercise motivation: use and role of technologies such as the internet, mobile applications, social media, and video games. Diabetes Spectrum. (2015) 28:45–54. doi: 10.2337/diaspect.28.1.45
29. Broekhuizen K, Gelder J de, Wijsman CA, Wijsman LW, Westendorp RG, Verhagen E, et al. An internet-based physical activity intervention to improve quality of life of inactive older adults: a randomized controlled trial. J Med Internet Res. (2016) 18:e4335. doi: 10.2196/jmir.4335
30. Dauwan M, Begemann MJH, Slot MIE, Lee EHM, Scheltens P, Sommer IEC. Physical exercise improves quality of life, depressive symptoms, and cognition across chronic brain disorders: a transdiagnostic systematic review and meta-analysis of randomized controlled trials. J Neurol. (2021) 268:1222–46. doi: 10.1007/s00415-019-09493-9
31. Williams FR, Berzigotti A, Lord JM, Lai JC, Armstrong MJ. Review article: impact of exercise on physical frailty in patients with chronic liver disease. Alimentary Pharmacol Ther. (2019) 50:988–1000. doi: 10.1111/apt.15491
32. Jing X, Chen J, Dong Y, Han D, Zhao H, Wang X, et al. Related factors of quality of life of type 2 diabetes patients: a systematic review and meta-analysis. Health Qual Life Outcomes. (2018) 16:189. doi: 10.1186/s12955-018-1021-9
33. Anderson E, Durstine JL. Physical activity, exercise, and chronic diseases: a brief review. Sports Med Health Sci. (2019) 1:3–10. doi: 10.1016/j.smhs.2019.08.006
34. Booth FW, Gordon SE, Carlson CJ, Hamilton MT. Waging war on modern chronic diseases: primary prevention through exercise biology. J Appl Physiol. (2000) 88:774–87. doi: 10.1152/jappl.2000.88.2.774
35. McWilliams JM. Health consequences of uninsurance among adults in the United States: recent evidence and implications. Milbank Q. (2009) 87:443–94. doi: 10.1111/j.1468-0009.2009.00564.x
36. Ronksley PE, Sanmartin C, Quan H, Ravani P, Tonelli M, Manns B, et al. Association between chronic conditions and perceived unmet health care needs. Open Med. (2012) 6:e48–58.
37. Diao Y, Lin M, Xu K, Huang J, Wu X, Li M, et al. How government health insurance coverage of novel anti-cancer medicines benefited patients in China – a retrospective analysis of hospital clinical data. BMC Health Serv Res. (2021) 21:856. doi: 10.1186/s12913-021-06840-3
38. Liu Z, Li W, Zhang T. Internet and private insurance participation. Int J Finance Econ. (2022) 27:1495–509. doi: 10.1002/ijfe.2227
39. Müller T, Woitalla D. Quality of life, caregiver burden and insurance in patients with Parkinson's disease in Germany. Eur J Neurol. (2010) 17:1365–9. doi: 10.1111/j.1468-1331.2010.03033.x
40. Glasgow RE, Ruggiero L, Eakin EG, Dryfoos J, Chobanian L. Quality of life and associated characteristics in a large national sample of adults with diabetes. Diabetes Care. (1997) 20:562–7. doi: 10.2337/diacare.20.4.562
41. Bharmal M, Thomas J. Health insurance coverage and health-related quality of life: analysis of 2000 medical expenditure panel survey data. J Health Care Poor Underserv. (2005) 16:643–54. doi: 10.1353/hpu.2005.0064
42. Flanagan JC. A research approach to improving our quality of life. Am Psychol. (1978) 33:138. doi: 10.1037/0003-066X.33.2.138
43. Anderson KL, Burckhardt CS. Conceptualization and measurement of quality of life as an outcome variable for health care intervention and research. J Adv Nurs. (1999) 29:298–306. doi: 10.1046/j.1365-2648.1999.00889.x
44. Burckhardt CS, Anderson KL. The Quality of Life Scale (QOLS): reliability, validity, and utilization. Health Qual Life Outcomes. (2003) 1:60. doi: 10.1186/1477-7525-1-60
45. Zucoloto ML, Martinez EZ. General aspects of quality of life in heterogeneous populations: notes on Flanagan's Quality of Life Scale (QoLS). Trends Psychiatry Psychother. (2019) 41:268–275. doi: 10.1590/2237-6089-2018-0077
46. Middleton CA, Leith J. Intensity of internet use in Canada: exploring Canadians' engagement with the Internet. In: Statistics Canada Socio-economic Conference. Ottawa, ON (2007).
47. Middleton C, Veenhof B, Leith J. Intensity of Internet Use in Canada: Understanding Different Types of Users. Statistics Canada. Business Special Surveys and Technology Statistics Division (2010).
48. Bélanger RE, Akre C, Berchtold A, Michaud P-A. A U-shaped association between intensity of internet use and adolescent health. Pediatrics. (2011) 127:e330–5. doi: 10.1542/peds.2010-1235
49. Dufour M, Brunelle N, Tremblay J, Leclerc D, Cousineau M-M, Khazaal Y, et al. Gender difference in internet use and internet problems among quebec high school students. Can J Psychiatry. (2016) 61:663–8. doi: 10.1177/0706743716640755
50. Tseng H-Y, Löckenhoff C, Lee C-Y, Yu S-H, Wu I, Chang H-Y, et al. The paradox of aging and health-related quality of life in Asian Chinese: results from the Healthy Aging Longitudinal Study in Taiwan. BMC Geriatr. (2020) 20:1–10. doi: 10.1186/s12877-020-1446-y
51. Conaghan PG, Doane MJ, Jaffe DH, Dragon E, Abraham L, Viktrup L, et al. Are pain severity and current pharmacotherapies associated with quality of life, work productivity, and healthcare utilisation for people with osteoarthritis in five large European countries. Clin Exp Rheumatol. (2021) 39:819–28. doi: 10.55563/clinexprheumatol/t5nm6l
52. Ikeda T, Igarashi A, Odani S, Murakami M, Tabuchi T. Health-related quality of life during COVID-19 pandemic: assessing impacts of job loss and financial support programs in Japan. Appl Res Qual Life. (2022) 17:541–57. doi: 10.1007/s11482-021-09918-6
53. Hayes AF. An index and test of linear moderated mediation. Multivar Behav Res. (2015) 50:1–22. doi: 10.1080/00273171.2014.962683
54. China Internet Network Information Center (CNNIC). The 45th China Statistical Report on Internet Development. Cyberspace Administration of China (2020). Available online at: http://www.cac.gov.cn/2020-04/27/c_1589535470378587.htm (accessed April 12, 2022).
55. China Internet Network Information Center (CNNIC). The 47th China Statistical Report on Internet Development. Cyberspace Administration of China (2021). Available online at: http://www.cac.gov.cn/2021-02/03/c_1613923423079314.htm (accessed April 12, 2022).
56. Kraut R, Patterson M, Lundmark V, Kiesler S, Mukophadhyay T, Scherlis W. Internet paradox: a social technology that reduces social involvement and psychological well-being? Am Psychol. (1998) 53:1017–31. doi: 10.1037/0003-066X.53.9.1017
57. Chandrasekaran B, Ganesan TB. Sedentarism and chronic disease risk in COVID 19 lockdown – a scoping review. Scott Med J. (2021) 66:3–10. doi: 10.1177/0036933020946336
58. Katz E, Blumler JG, Gurevitch M. Uses and gratifications research. Public Opin Q. (1973) 37:509–23. doi: 10.1086/268109
59. Hu SLY, Leung L. Effects of expectancy-value, attitudes, and use of the Internet on psychological empowerment experienced by Chinese women at the workplace. Telemat Inform. (2003) 20:365–82. doi: 10.1016/S0736-5853(03)00036-4
60. Sigal RJ, Kenny GP, Wasserman DH, Castaneda-Sceppa C, White RD. Physical Activity/Exercise and Type 2 Diabetes: a consensus statement from the American Diabetes Association. Diabetes Care. (2006) 29:1433–8. doi: 10.2337/dc06-9910
61. Arne M, Janson C, Janson S, Boman G, Lindqvist U, Berne C, et al. Physical activity and quality of life in subjects with chronic disease: chronic obstructive pulmonary disease compared with rheumatoid arthritis and diabetes mellitus. Scand J Prim Health Care. (2009) 27:141–7. doi: 10.1080/02813430902808643
62. Mitsutake S, Shibata A, Ishii K, Oka K. Associations of eHealth literacy with health behavior among adult internet users. J Med Internet Res. (2016) 18:e5413. doi: 10.2196/jmir.5413
63. Booth FW, Roberts CK, Laye MJ. Lack of exercise is a major cause of chronic diseases. Compr Physiol. (2012) 2:1143–211. doi: 10.1002/cphy.c110025
64. Ma S, Yuan Z, Peng Y, Chen J, Li H, Luo Q, et al. Experience and suggestion of medical practices for burns during the outbreak of COVID-19. Burns. (2020) 46:749–55. doi: 10.1016/j.burns.2020.03.014
65. Liu N, Huang R, Baldacchino T, Sud A, Sud K, Khadra M, et al. Telehealth for noncritical patients with chronic diseases during the COVID-19 pandemic. J Med Internet Res. (2020) 22:e19493. doi: 10.2196/19493
66. Maher C, Ferguson M, Vandelanotte C, Plotnikoff R, Bourdeaudhuij ID, Thomas S, et al. A web-based, social networking physical activity intervention for insufficiently active adults delivered via facebook app: randomized controlled trial. J Med Internet Res. (2015) 17:e4086. doi: 10.2196/jmir.4086
67. Dong X, Yi X, Gao D, Gao Z, Huang S, Chao M, et al. The effects of the combined exercise intervention based on internet and social media software (CEIBISMS) on quality of life, muscle strength and cardiorespiratory capacity in Chinese postoperative breast cancer patients:a randomized controlled trial. Health Qual Life Outcomes. (2019) 17:109. doi: 10.1186/s12955-019-1183-0
68. Lv Q, Jiang Y, Qi J, Zhang Y, Zhang X, Fang L, et al. Using mobile apps for health management: a new health care mode in China. JMIR mHealth uHealth. (2019) 7:e10299. doi: 10.2196/10299
69. Sun S, Yu K, Xie Z, Pan X. China empowers internet hospital to fight against COVID-19. J Infect. (2020) 81:e67–8. doi: 10.1016/j.jinf.2020.03.061
70. Wu J, Xie X, Yang L, Xu X, Cai Y, Wang T, et al. Mobile health technology combats COVID-19 in China. J Infect. (2021) 82:159–98. doi: 10.1016/j.jinf.2020.07.024
71. Bundorf MK, Singer SJ, Wagner TH, Baker L. Consumers' use of the Internet for health insurance. Am J Manag Care. (2004) 10, pp.609–6. https://www.researchgate.net/profile/Sara-Singer/publication/8204348_Consumers'_Use_of_Internet_for_Health_Insurance/links/00463531e576b77a02000000/Consumers-Use-of-Internet-for-Health-Insurance.pdf
72. Appleton S, Song L. Life satisfaction in urban China: components and determinants. World Dev. (2008) 36:2325–40. doi: 10.1016/j.worlddev.2008.04.009
73. Millard RW, Fintak PA. Use of the internet by patients with chronic illness. Dis Manage Health Outcomes. (2002) 10:187–94. doi: 10.2165/00115677-200210030-00006
74. Qiu Z, Zheng K, Zhang H, Feng J, Wang L, Zhou H. Physical exercise and patients with chronic renal failure: a meta-analysis. BioMed Res Int. (2017) 2017:e7191826. doi: 10.1155/2017/7191826
Keywords: COVID-19 pandemic, internet use intensity, quality of life, health insurance, physical exercise, health management of rural and urban governance
Citation: Wang Y, Xu J and Xie T (2022) Relationship between internet use intensity and quality of life in chronic patients during the COVID-19 pandemic: The role of physical exercise and health insurance. Front. Public Health 10:947465. doi: 10.3389/fpubh.2022.947465
Received: 18 May 2022; Accepted: 08 July 2022;
Published: 16 September 2022.
Edited by:
Caress Alithia Dean, Oakland University, United StatesReviewed by:
Changle Li, Inner Mongolia Medical University, ChinaWei Wang, Xuzhou Medical University, China
Copyright © 2022 Wang, Xu and Xie. This is an open-access article distributed under the terms of the Creative Commons Attribution License (CC BY). The use, distribution or reproduction in other forums is permitted, provided the original author(s) and the copyright owner(s) are credited and that the original publication in this journal is cited, in accordance with accepted academic practice. No use, distribution or reproduction is permitted which does not comply with these terms.
*Correspondence: Tian Xie, xietian@sjtu.edu.cn