- 1Section on Ethnicity and Health, Diabetes, Endocrinology, and Obesity Branch, National Institute of Diabetes, Digestive and Kidney Diseases, National Institutes of Health, Bethesda, MD, United States
- 2Hypertension in Africa Research Team, North-West University, Potchefstroom, South Africa
- 3South African Medical Research Council, Unit for Hypertension and Cardiovascular Disease, North-West University, Potchefstroom, South Africa
- 4National Institute of Minority Health and Health Disparities, National Institutes of Health, Bethesda, MD, United States
- 5Institute of Global Health Equity Research, University of Global Health Equity, Kigali, Rwanda
- 6Department of Health and Nutrition Sciences, Brooklyn College, City University of New York, New York, NY, United States
Background: Emerging data suggests that in sub-Saharan Africa β-cell-failure in the absence of obesity is a frequent cause of type 2 diabetes (diabetes). Traditional diabetes risk scores assume that obesity-linked insulin resistance is the primary cause of diabetes. Hence, it is unknown whether diabetes risk scores detect undiagnosed diabetes when the cause is β-cell-failure.
Aims: In 528 African-born Blacks living in the United States [age 38 ± 10 (Mean ± SE); 64% male; BMI 28 ± 5 kg/m2] we determined the: (1) prevalence of previously undiagnosed diabetes, (2) prevalence of diabetes due to β-cell-failure vs. insulin resistance; and (3) the ability of six diabetes risk scores [Cambridge, Finnish Diabetes Risk Score (FINDRISC), Kuwaiti, Omani, Rotterdam, and SUNSET] to detect previously undiagnosed diabetes due to either β-cell-failure or insulin resistance.
Methods: Diabetes was diagnosed by glucose criteria of the OGTT and/or HbA1c ≥ 6.5%. Insulin resistance was defined by the lowest quartile of the Matsuda index (≤ 2.04). Diabetes due to β-cell-failure required diagnosis of diabetes in the absence of insulin resistance. Demographics, body mass index (BMI), waist circumference, visceral adipose tissue (VAT), family medical history, smoking status, blood pressure, antihypertensive medication, and blood lipid profiles were obtained. Area under the Receiver Operator Characteristics Curve (AROC) estimated sensitivity and specificity of each continuous score. AROC criteria were: Outstanding: >0.90; Excellent: 0.80–0.89; Acceptable: 0.70–0.79; Poor: 0.50–0.69; and No Discrimination: 0.50.
Results: Prevalence of diabetes was 9% (46/528). Of the diabetes cases, β-cell-failure occurred in 43% (20/46) and insulin resistance in 57% (26/46). The β-cell-failure group had lower BMI (27 ± 4 vs. 31 ± 5 kg/m2 P < 0.001), lower waist circumference (91 ± 10 vs. 101 ± 10cm P < 0.001) and lower VAT (119 ± 65 vs. 183 ± 63 cm3, P < 0.001). Scores had indiscriminate or poor detection of diabetes due to β-cell-failure (FINDRISC AROC = 0.49 to Cambridge AROC = 0.62). Scores showed poor to excellent detection of diabetes due to insulin resistance, (Cambridge AROC = 0.69, to Kuwaiti AROC = 0.81).
Conclusions: At a prevalence of 43%, β-cell-failure accounted for nearly half of the cases of diabetes. All six diabetes risk scores failed to detect previously undiagnosed diabetes due to β-cell-failure while effectively identifying diabetes when the etiology was insulin resistance. Diabetes risk scores which correctly classify diabetes due to β-cell-failure are urgently needed.
Introduction
Improving detection of undiagnosed type 2 diabetes is an urgent concern in sub-Saharan Africa, where 54% of diabetes is undiagnosed and prevalence is expected to increase by 143% by 2,045 (1). Compared to high income countries, many patients with type 2 diabetes living in sub-Saharan African countries are younger, do not have obesity, and present with relative β-cell-failure (2) rather than insulin resistance (2–6). Yet this phenotype is not unique in sub-Saharan Africa and has been shown in population studies from Asia [China (7), Korea (8) and India (9)], Europe [Switzerland (10)], and the Americas [Brazil (11), US (12) and Trinidad and Tobago (13)]. Indeed, a high rate of diabetes has been reported in people without obesity from low-and middle income countries (14). Current type 2 diabetes screening guidelines are based on studies in populations where insulin resistance is the primary pathogenic mechanism of type 2 diabetes.
The American Diabetes Association and International Diabetes Federation encourage the use of non-invasive risk prediction scores as affordable approaches to identifying patients at increased risk of type 2 diabetes who should be referred for diagnostic tests (1, 15, 16). Although diabetes risk scores are not applied when an individual's diagnosis is known, for individuals whose outcome is unknown, such risk scores are applied for the purpose of screening. Type 2 diabetes risk scores rely on demographic, anthropometric, and clinical risk factors that commonly appear together in at-risk individuals such as age, family history of diabetes, BMI, and blood pressure, yet none of these risk tools consider distinct type 2 diabetes phenotypes and etiologies (5, 17).
Type 2 diabetes risk scores, including the simplified Cambridge (18), simplified Finnish Diabetes Risk Score (FINDRISC) (19, 20), Kuwaiti (21), Omani (22), Rotterdam (23) and SUNSET (24) universally suppose that diabetes occurs due to obesity-linked insulin resistance since they all rely on body mass index (BMI) and/or waist circumference. Yet, data in Black people from sub-Saharan African countries suggest that β-cell-failure in the absence of obesity is a frequent cause of abnormal glucose tolerance (a term combining prediabetes and diabetes) (3, 5). Type 2 Diabetes due to β-cell-failure may often take longer to diagnose than insulin resistance (3–5). As β-cell-failure may only be diagnosed later in the course of the disease, it may in fact be a diabetes complication which triggered the screening for diabetes. Indeed, evidence from sub-Saharan African countries show that due to limited access to non-communicable disease screening, in low resource areas where access to blood testing and biomarker analyses are limited, and diagnosis of type 2 diabetes is likely to occur after the onset of clinical complications such as blindness, renal failure, and amputations (1, 3, 25). Thus, Africans with type 2 diabetes due to β-cell-failure may not be detected by current screening tools. It is unknown how well these non-invasive type 2 Diabetes risk scores perform in the detection of undiagnosed diabetes primarily due to either β-cell-failure or insulin resistance.
Therefore, the goals of this study were to determine within the Africans in America cohort the (1) prevalence of previously undiagnosed type 2 diabetes within the total cohort; (2) prevalence of type 2 diabetes due to β-cell-failure and insulin resistance; and (3) the ability of six diabetes risk scores (Cambridge, FINDRISC, Kuwaiti, Omani, Rotterdam, and SUNSET) to correctly classify patients who should be referred for diagnostic tests and detect previously undiagnosed type 2 diabetes due to either β-cell-failure or insulin resistance.
Methods
Population
The Africans in America cohort is a clinical protocol designed to assess cardiometabolic and psychosocial status of Black Africans born in sub-Saharan African countries and currently living in the United States (5, 26–28). Recruitment was achieved by advertisements in newspapers, flyers, social media, and posters at community events. The study was approved by the NIDDK Institutional Review Board (ClinicalTrials.gov Identifier: NCT00001853). Written informed consent was obtained.
To determine eligibility a telephone interview was conducted. To be eligible for a screening visit at the NIH Clinical Center, the prospective enrollee had to be state at their telephone interview that they were 18–65 years of age, self-identified as healthy, and that they and their parents self-identified as Black and were born in sub-Saharan Africa. In addition, they had to specifically deny a history of diabetes, liver or kidney disease, anemia, or thyroid disease. Pregnancy tests were performed in women of child-bearing age and had to be negative.
Five hundred and eighty African-born Black people living in metropolitan Washington, DC completed the telephone interview and proceeded to the screening visit for a medical history, physical examination, electrocardiogram, and routine blood tests (Figure 1). Questionnaires determined age of immigration, years in the U.S., family history of diabetes, use of antihypertensive medication, and smoking status.
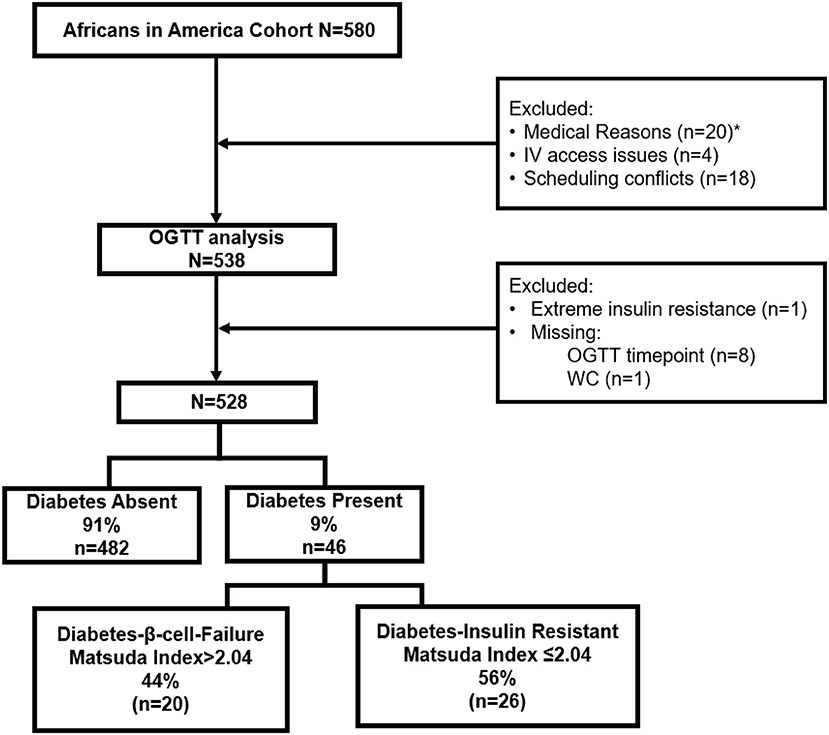
Figure 1. Flow diagram of study design. *Medical Reasons included anemia (n = 12), elevated liver transaminases (n = 1), declined blood draw (n = 3), pregnancy (n = 3), hypothyroidism (n = 1).
Forty-one individuals did not proceed from the Screening Visit to the oral glucose tolerance test (OGTT). Reasons for exclusion were anemia (n = 12), elevated liver transaminases (n = 1), declined blood draw (n = 3), pregnancy (n = 3), hypothyroidism (n = 1), IV access issues (n = 4) and scheduling conflicts (n = 18).
Following OGTT analysis, 9 individuals were excluded. One participant had a fasting insulin concentration of 172 pmol/L (normal < 30 pmol/L) and was diagnosed with extreme insulin resistance. Eight individuals had a missing value for glucose or insulin during the multi-sampled OGTT.
One participant did not have a waist circumference value, and as waist circumference is part of the risk score calculations, was excluded from analyses.
The remaining 528 participants were included in all data analyses.
Oral glucose tolerance test visit
After an overnight 12 h fast, participants came to the NIH Clinical Center at 7AM. Resting vitals were measured. Height, weight, waist circumference, and blood pressure (BP) were measured. Body mass index (BMI) was calculated (kg/m2). Obesity was defined as BMI≥30 kg/m2 (29). Waist circumference was measured at the superior border of the iliac crest at the end of expiration, and the mean of three values was recorded (30).
Baseline blood samples were obtained for fasting plasma glucose (FPG), insulin, and HbA1c. Post Glucola consumption (Trutol 75, Custom Laboratories) blood samples were taken at 0.5 h, 1 h, 1.5 h, and 2 h to determine glucose and insulin concentrations and calculate the AUC for glucose and insulin responses. Timepoints baseline, 0.5 h, 1 h and 2 h were used to calculate the Matsuda Index.
After the OGTT, a computerized tomographic (CT) scan (Siemens and Somatom Force Scanner) with adipose windows designed to measure visceral adipose tissue (VAT) was performed (31).
Diabetes diagnosis
A diagnosis of diabetes required: FPG≥126 mg/dL (7.0 mmol/L) and/or 2 h glucose≥200 mg/dL (11.1 mmol/L) and/or HbA1c ≥ 6.5% (48 mmol/mol) (32).
Insulin resistance status
Insulin resistance status was determined by Matsuda Index (5, 33):
A higher Matsuda Index denotes greater insulin sensitivity. As diagnostic category insulin sensitive was defined as Matsuda Index > 2.04, and insulin resistance was defined as the lowest quartile for our population distribution of Matsuda Index (≤ 2.04).
The oral Disposition Index, which is a measure of β-cell-function adjusted for insulin sensitivity, was calculated using the Matsuda index, as the product of insulin secretion (ISI) and insulin sensitivity (Matsuda Index). Where insulin secretion index was calculated as:
Group assignment by diabetes and insulin resistance status
Participants were categorized in two ways: presence or absence of type 2 diabetes, and presence or absence of insulin resistance, resulting in two etiology groups (Figure 1) (2, 5):
Group 1: Diabetes-β-Cell-Failure
This group had diabetes due to β-cell-failure because diabetes occurred in the absence of insulin resistance (Matsuda > 2.04).
Group 2: Diabetes-Insulin-Resistance
This group had diabetes due to insulin resistance (Matsuda ≤ 2.04).
Assays
Hemoglobin and hematocrit were measured in EDTA-anticoagulated whole blood (Sysmex XE-5000). Insulin was measured in serum and glucose, cholesterol, TG, HDL in plasma using a Roche Cobas 6000 analyzer (Roche Diagnostics, Indianapolis, Indiana). LDL was calculated using the Friedewald equation (34). HbA1c values were determined by HPLC using National Glycohemoglobin Standardization Program (NGSP)-certified instruments manufactured by BioRad Laboratories (Hercules, CA, USA).
Diabetes scores
We included six diabetes risk scores and tested for their ability to detect previously undiagnosed type 2 diabetes in African descent populations: Cambridge, Simplified FINDRISC, Kuwaiti, Omani, Rotterdam, and SUNSET (35). Risk score components included age, sex, family history of diabetes, antihypertensive medication/hypertension, resting heart rate, BMI, waist circumference, and smoking (Table 1). Scores were applied as continuous variables and dichotomized at optimal cut-points.
Statistical analysis
Unless stated otherwise, data are presented as Mean ± SE. Comparisons with the Diabetes-Absent group were adjusted for age. One-way analysis of variance (ANOVA) with Bonferroni corrections for multiple comparisons were used to compare the three groups (Diabetes-Absent, Diabetes-β-Cell-Failure and Diabetes-Insulin-Resistance) and categorical variables were compared by chi-square and the Dunn test. Unpaired t-tests were used to compare Diabetes-Absent and Diabetes Present groups. Area Under the curve for glucose and insulin were calculated using the trapezoidal rule.
Six risk scores were calculated for each participant and mean values compared between groups. Receiver operator characteristics (ROC) curves showed the relationship between specificity and sensitivity. Area under the receiver operator characteristic curve (AROC) assessed the ability of each risk score to detect diabetes by etiology group (P < 0.05). ROC was calculated for three models:
Model A (n = 528)
• n = 46 participants with Diabetes-β-Cell-Failure or Diabetes-Insulin-Resistance
• n = 482 participants without diabetes
Model B (n = 502)
• n = 20 participants with Diabetes-β-Cell-Failure
• n = 482 participants without diabetes
Model C (n = 508)
• n = 26 participants with Diabetes-Insulin-Resistance
• n = 482 participants without diabetes
The optimal cut-point for each risk score to detect previously undiagnosed diabetes in the total cohort (Model A) was calculated as:
(16).
The six risk scores were dichotomized at the optimal cut point of Model A.
All statistical analyses were performed with STATA17 (College Station, Texas).
Results
The final sample of 528 Black people born in sub-Saharan African countries was 64% male, mean age 38 ± 10y (range 20–65y), and mean BMI 28 ± 5 kg/m2 (range: 18.4–42.2 kg/m2). African regions of birth were West (50%, 266/528), Central (19%, 103/528), East (30%, 159/528). The six participants from southern African countries were grouped with the Central African countries. Most participants were male and from West Africa, similar to known immigration patterns to the United States. The prevalence of type 2 diabetes did not differ between African regions of origin, nor were there any significant differences in risk score components i.e., age, sex, BMI, waist circumference, visceral adipose tissue, FPG, 2-h glucose, HbA1c, family history of diabetes or blood pressure medication usage. Therefore, we analyzed the cohort as a single group.
Diabetes etiology and diabetes prevalence
Prevalence of previously undiagnosed diabetes was 9% (46/528). Of the diabetes cases, β-cell-failure occurred in 43% (20/46), and insulin-resistance in 57% (26/46). The participants with Diabetes-β-Cell-Failure had been living in the U.S. longer and immigrated at a younger age compared to the Diabetes-Insulin-Resistance group (both P < 0.01). Mean 2 h-glucose and HbA1c did not differ between diabetes etiology groups. Yet, the Diabetes-β-Cell-Failure group had lower FPG, lower OGTT glucose levels at 0.5 h and 1 h (P < 0.05) than the Diabetes-Insulin-Resistance group. In addition, fasting insulin levels, and OGTT insulin at 0.5 h, 1 h, 1.5 h, and 2 h were significantly lower in the Diabetes-β-Cell-Failure group (P < 0.001). Compared to the Diabetes-β-Cell-Failure group, AUC for both glucose and insulin responses during the OGTT were significantly greater in the Diabetes-Insulin-Resistance group (P < 0.001). However, oral disposition index was significantly higher in the Diabetes-β-Cell-Failure group compared to the Diabetes-Insulin-Resistance group (P = 0.002) (Table 2).
The Diabetes-β-Cell-Failure group had lower BMI, lower waist circumference and lower VAT compared to the Diabetes-Insulin-Resistance group (all P < 0.001) (Figure 2). Obesity prevalence was also lower in Diabetes-β-Cell-Failure vs. Diabetes-Insulin-Resistance (10% vs. 77%) (P < 0.001). TG levels were lower in the Diabetes-β-Cell-Failure group (P < 0.05), but no significant differences were noted for TC, LDL, and HDL levels between etiology groups.
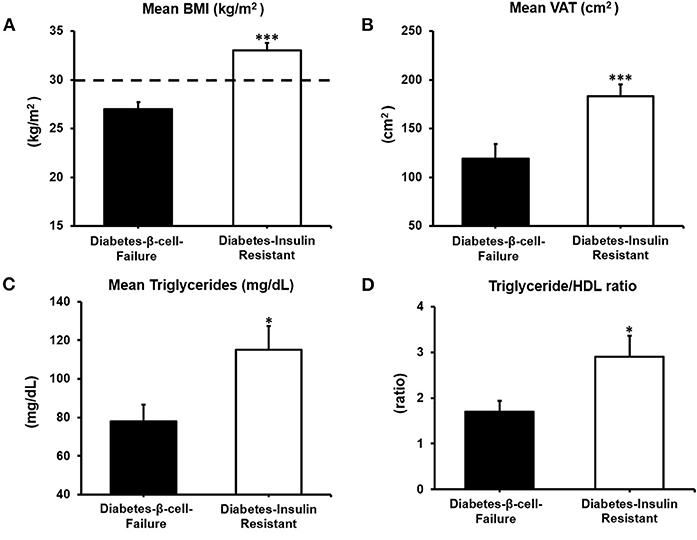
Figure 2. Body composition and lipid parameters according to etiology of diabetes: (A) Body Mass Index (BMI). (B) Visceral Adipose Tissue (VAT). (C) Triglycerides and (D) Triglyceride/HDL ratio. ***P < 0.001; **P < 0.01; *P < 0.05.
Risk score comparison
The Diabetes-β-Cell-Failure group showed significantly lower scores for FINDRISC, Kuwaiti, Rotterdam, and SUNSET than the Diabetes-Insulin-Resistance group (all P < 0.05). However, there were no significant differences for the Cambridge and Omani scores between Diabetes-β-Cell-Failure and Diabetes-Insulin-Resistance groups (Figure 3; Supplementary Table 3).
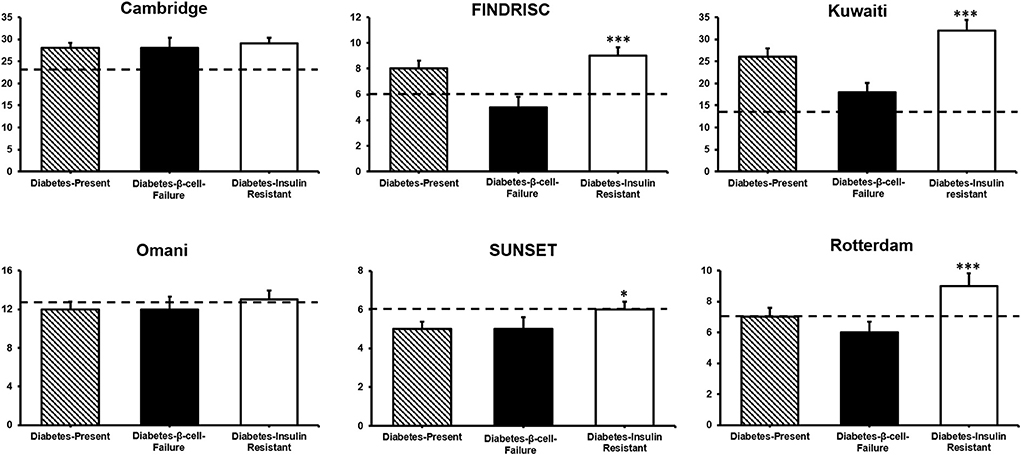
Figure 3. Diabetes risk scores by diabetes etiology. Comparison between diabetes β-Cell-Failure and diabetes-insulin-resistance ***P < 0.001; *P < 0.05. Dotted line shows the respective cut-point of each score predicting diabetes in this cohort.
Table 3 shows AROC for the Cambridge, FINDRISC, Kuwaiti, Omani, Rotterdam, and SUNSET in the Model A (Diabetes-Absent + Total Diabetes), Model B (Diabetes-Absent + Diabetes-β-Cell-Failure), and Model C (Diabetes-Absent + Diabetes-Insulin-Resistance). All scores showed indiscriminate to poor predictability in Diabetes-β-Cell-Failure. Scores had poor to excellent predictability for Diabetes-Insulin-Resistance.
When examining the ability of each risk score to correctly identify ten participants with Diabetes-β-Cell-Failure, Cambridge correctly detected 9/10 (85%), FINDRISC detected 6/10 (55%), Kuwaiti detected 4/10 (40%), Omani detected 5/10 (50%), Rotterdam detected 3/10 (25%), and SUNSET detected 4/10 (40%). In contrast, when predicting Diabetes-Insulin-Resistance, Cambridge detected 9/10 (92%), FINDRISC detected 9/10 (88%), Kuwaiti detected 9/10 (88%), Omani detected 8/10 (77%), Rotterdam detected 7/10 (65%) and SUNSET detected 6/10 (62%) (Figures 4A,B).
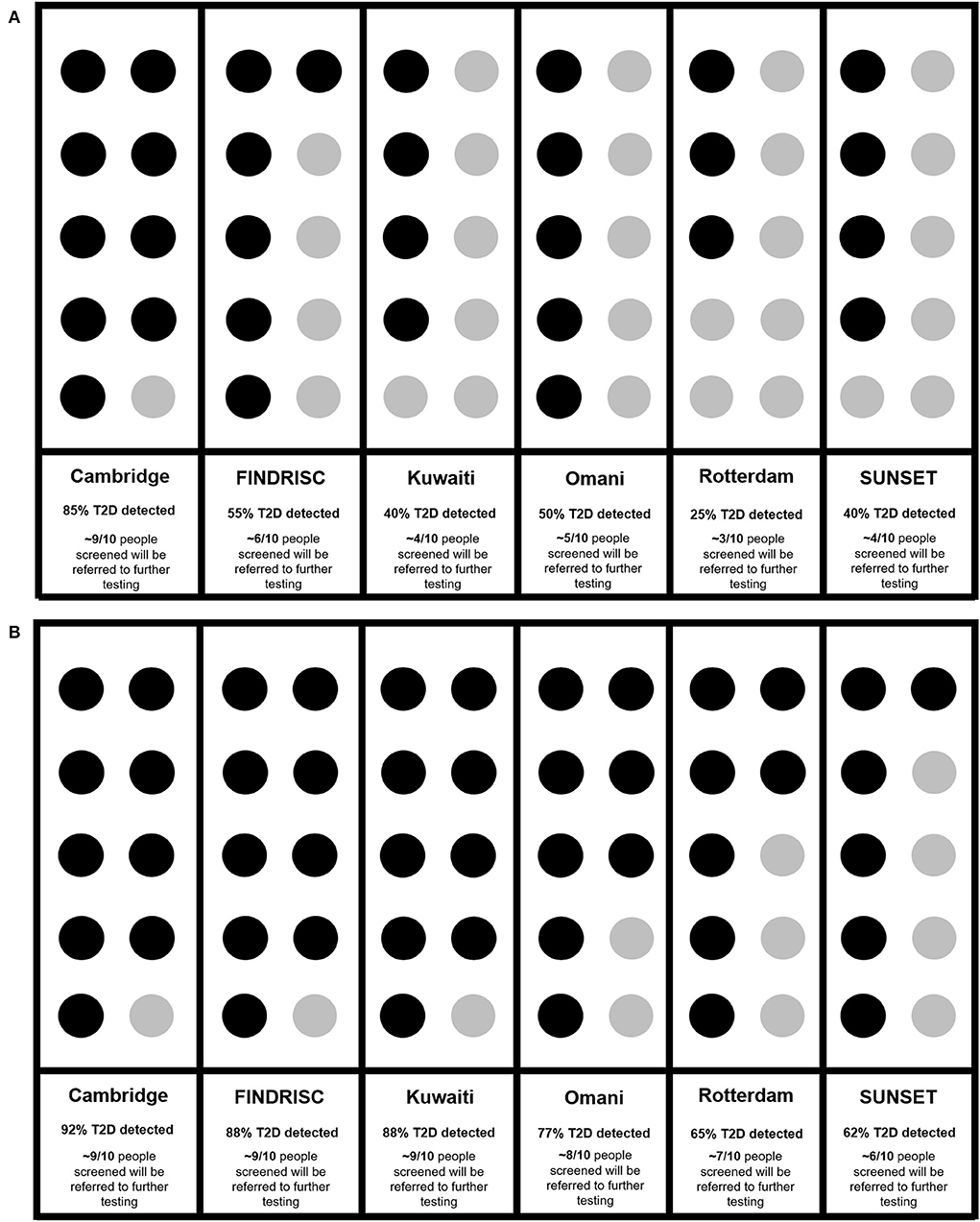
Figure 4. (A) Ability of risk scores to identify diabetes in every 10 diabetes cases due to β -Cell-Failure. (B) Ability of risk scores to identify diabetes in every 10 diabetes cases due to insulin resistance. Cambridge (18), FINDRISC (20), Kuwaiti (21), Omani (22), Rotterdam (23), SUNSET (24).
Discussion
Non-invasive diabetes risk scores failed to detect previously undiagnosed diabetes in Africans when the etiology was β-cell-Failure, but they effectively detected diabetes due to insulin resistance. When diabetes was due to β-cell-Failure, Cambridge was somewhat effective. Yet, this should be interpreted with caution, as Cambridge also produces a high rate of false positives and therefore might divert sparse resources to unnecessarily refer more people for diagnostic diabetes testing.
Risk of diabetes-β-cell-failure without obesity
Even when validated in African-descent populations in the United Kingdom (36), South Africa (15), The Netherlands (24) and United States (16), non-invasive diabetes risk scores do not accurately identify diabetes due to β-cell-failure. In the current study, the only risk score that shows promise in detecting diabetes due to β-cell-failure, is the Cambridge score. Cambridge was able to identify 88% of all diabetes-cases screened. As Cambridge scores multiple levels of BMI, not just the presence or absence of obesity, it might retain relative accuracy (18). The Cambridge score may therefore be the most useful, specifically in populations where β-cell-failure is suspected to be the primary cause of diabetes. As diabetes due to β-cell-failure predominantly occurs in the absence of obesity, screening programs are unlikely to identify these as “high-risk” cases. Such risk-underestimation means that diabetes diagnosis occurs only after the onset of clinical complications. Nevertheless, as the modified Cambridge score was able to accurately identify previously undiagnosed diabetes due to both insulin resistance and β-cell-failure, this score appears to perform relatively well regardless of diabetes etiology. However, this must be interpreted with caution, as low specificity indicates that Cambridge over-estimates risk. Therefore, applying Cambridge in low resource settings, would divert scarce resources to unnecessary diagnostic testing of people who do not have diabetes.
Obesity-linked risk in the diabetes-insulin-resistance group
The Diabetes-Insulin-Resistance etiology group did indeed show phenotypic characteristics associated with insulin resistance: higher BMI, higher waist circumference and higher visceral adipose tissue in addition to higher triglyceride/HDL ratio. This supports the notion that non-invasive risk scores assume that the primary cause of diabetes is obesity-linked insulin resistance. The six risk scores showed better discriminatory ability to identify undiagnosed diabetes due to insulin resistance compared to β-cell-failure. In three population studies, specifically FINDRISC was linked to insulin resistance (16, 37, 38), and shown to be effective in detecting diabetes in patients with obesity. A cross-sectional validation study of the Cambridge and Rotterdam scores in an Irish population, (39) reported that anthropometric components (BMI and waist circumference) of the risk scores demonstrated the greatest discriminatory ability and largest contribution to the overall score. Our findings support this, as all six scores effectively detect diabetes in the insulin resistant group, the group that also had the highest prevalence of obesity. Body mass index and/or waist circumference are two of the strongest predictors of risk in all six scores. The value of waist circumference as a proxy for VAT and predictor of insulin resistance is illustrated by the significantly higher VAT, in the Diabetes-Insulin-Resistance group vs. the Diabetes-β-Cell-Failure group as previously shown (5, 40).
Although the six non-invasive diabetes risk scores remain valuable for the detection of undiagnosed diabetes primarily caused by insulin resistance, risk scores relying on BMI and waist circumference fail to predict diabetes due to β-cell-failure, as only 10% (n = 2) had obesity. This is of major concern, as Black people born in sub-Saharan African countries may be at greater risk of developing diabetes at a lean-to-normal BMI and have β-cell-failure or secretory dysfunction, rather than peripheral insulin resistance (3–6, 41, 42).
Family history
Family history of diabetes has been shown to be a strong, independent predictor of diabetes risk (43, 44). Yet, in populations originating from low- and middle-income countries, family history might be underreported because family medical history is unknown, or family members live or have lived with undisclosed diabetes (43). Although, in our population, family history of diabetes did not significantly differ between diabetes etiology groups, we recognize that family history could be a powerful predictor of diabetes risk.
Measuring insulin resistance not degree of glycemia
Adding HOMA-IR and HOMA-β did not increase FINDRISC's discriminatory accuracy (45, 46). Our findings show that insulin resistance status plays a key role in all scores, except Cambridge. This is also supported by findings from the San Antonio Heart study showing that by adding HOMA-IR or HOMA-β to the predictive model the discriminatory capability of the FINDRISC model significantly improves (47). We found that not only FINDRISC, but also Rotterdam, Kuwaiti, Omani, and SUNSET's detection abilities were greatly influenced by insulin resistance status.
Furthermore, markers of glycemia, fasting plasma glucose, 2-h glucose and HbA1c did not differ between diabetes etiology groups. However, the oral disposition index differed significantly. Compared to the Diabetes-β-Cell-Failure group, the Diabetes-Insulin-Resistance group had considerable insulin resistance and also a lower oral disposition index. This might be explained by the lower insulin concentrations in the Diabetes-β-Cell-Failure group compared to the Diabetes-Absent group. In addition, the Diabetes-β-Cell-Failure group had minimal insulin resistance (Matsuda > 2.04). This observation of higher oral disposition index in β-cell-failure is supported by observations in members of the Luo and Kamba tribes in Kenya (48). This indicates that these non-invasive diabetes scores predict diabetes due to degree of insulin resistance, and not based on the level of hyperglycemia. This trend is supported by other studies (16, 37), where particularly FINDRISC effectively detected diabetes due to insulin resistance in populations at risk of diabetes. Tracking with insulin resistance and not the degree of glycemia make these scores inherently biased toward diabetes due to insulin resistance.
Future directions
Participants in our study that were diagnosed with type 2 diabetes, were newly diagnosed by OGTT and thus previously undiagnosed. According to the IDF (1), 56% of people living with type 2 diabetes in Africa are undiagnosed—and our study showed that the current, non-invasive equations applied, do not detect them. Thus, patients are not receiving the benefit of early intervention to prevent possible type 2 diabetes related complications. Therefore, we advocate for modifying and optimizing these non-invasive diabetes risk scores for Africans, to identify existing diabetes.
Using Cambridge, FINDRISC, Kuwaiti, Omani, Rotterdam, and SUNSET as initial screening tools, is justified only when diabetes etiology is insulin resistance. The only diabetes risk score that may show some promise irrespective of diabetes etiology, is the Cambridge score. Previous findings from our lab suggest that a single invasive marker such as random or fasting plasma glucose rather than a battery of non-invasive variables would ensure more accurate detection of diabetes regardless of etiology. However, diabetes screening in sub-Saharan Africa is particularly challenging as diabetes screening and diagnostic tools such as random glucose, fasting plasma glucose, HbA1c, and the gold standard oral glucose tolerance test (OGTT) are either costly, invasive, inconvenient or do not perform optimally in African-born populations. A prior study from the Africans in America cohort showed that fasting plasma glucose ≥100 mg/dL alone was equivalent to the nine-variable ARIC + HbA1c prediction equation (49) despite the inclusion of BMI, age, Triglycerides, LDL, HDL and HbA1c. However, relying on plasma glucose may present logistical challenges in low- and middle-income settings where test strips and glucometers may not always be available, calibrated, or functional.
Risk scores which do not require invasive blood tests and rely on commonly collected clinical information are easy to use as screening tools in low resource and community settings. However, as diabetes due to β-cell-failure was not identified by five of the six risk scores, future risk tools for diabetes screening must account for this etiology. Early detection of diabetes due to β-cell-failure is crucial as these patients may only be identified after the onset of clinical complications such as retinopathy, renal failure, diabetic ulcers, and amputations. Furthermore, risk scores with high sensitivity and low specificity may unnecessarily increase referrals for costly diagnostic testing. Refining existing risk scores or creating new scores that either distinguish between body size categories more accurately, or do not only rely on characteristics associated with insulin resistance is required to more accurately detect diabetes due to β-cell-failure.
Strengths and limitations
The strengths of this study are fourfold. First, this study provides glucose and insulin levels from the OGTT. This allowed us to measure estimate insulin resistance by both Matsuda index and HOMA-IR to define diabetes etiologies. These tests differentiated between and categorized diabetes etiology groups similarly, which supports the use of HOMA-IR in low resource settings as it relies on a single fasting blood sample. Second, we demonstrated that non-invasive diabetes risk scores perform well when insulin resistance is the cause of diabetes, and that score performance was comparable to other studies in the sub-Saharan African region (20).
Third, the use of CT scans to obtain accurate estimation of VAT also supported the higher BMI and waist circumference as correlates of insulin resistance. Fourth, all socio-behavioral questionnaires, and risk score variables were administered, measured, and scored by research staff, and clinical variables were measured by clinical staff which increases completeness and reliability of collected data.
The study has two main limitations. The study was cross sectional and therefore identifies current and not future diabetes risk, although, these scores have been applied to detect diabetes incidence (15). Additionally, this is a convenience sample representing Black immigrants from sub-Saharan countries living in the Washington DC metropolitan area who chose to participate in the study. Nevertheless, the characteristics of our study population are consistent with known demographics of sub-Saharan African immigrants in the US, including a majority being male and 50% originating from West Africa (46). Furthermore, our findings are similar to previous studies of newly diagnosed diabetes among Sub-Saharan Africans living in Canada (6%) (50) and urban Sub-Saharan African populations(8.4%) (51). Nevertheless, we demonstrated that current diabetes prediction equations fail when β-cell-failure is the cause yet perform well when insulin resistance is the cause of diabetes.
As a limitation, we did not have access to islet-cell antibody testing to rule out latent autoimmune diabetes in adults (LADA) (52) or genetic tests to rule out maturity-onset diabetes of the young (MODY) (53). However, mean age for newly diagnosed diabetes was 43y in the β-Cell-Failure group and 45y in the Insulin-Resistance group, thus MODY is unlikely. In addition, although participants with β-cell failure had below normal insulin secretion, insulin secretion was still present, and no study participants reported a current or past history of diabetic ketoacidosis. Therefore, LADA was also unlikely to be the cause of diabetes in these participants.
Conclusion
At a prevalence of 43%, β-cell-failure accounted for nearly half of the cases of diabetes. All six diabetes risk scores failed to detect previously undiagnosed diabetes due to β-cell-failure while effectively identifying diabetes when the etiology was insulin resistance. Diabetes risk scores which correctly classify diabetes due to β-cell-failure are urgently needed.
Data availability statement
The raw data supporting the conclusions of this article will be made available by the authors, without undue reservation.
Ethics statement
The studies involving human participants were reviewed and approved by the Institutional Review Boards of the National Institutes of Health. The patients/participants provided their written informed consent to participate in this study.
Author contributions
Conceptualization, writing—original draft preparation, and writing—review and editing: AW, AES, and MFH-R. Methodology and investigation: AW, ACP, ZCW, BRS, MGD, CWD, MFH-R, and AES. Formal analysis: AW, ACP, BRS, MFH-R, and AES. Resources: AES. Data curation: ZCW and AES. Visualization: AW, ACP, MFH-R, and AES. Supervision: AW, CWD, and AES. Project administration: AW, ACP, ZCW, BRS, CWD, MFH-R, and AES. Funding acquisition: AW and AES. All authors have read and agreed to the published version of the manuscript.
Funding
AW was supported by the South African Medical Research Council, Unit for Hypertension and Cardiovascular Disease, North-West University, South Africa. AW, ACP, MGD, ZCW, BRS, CWD, and AES were supported by the intramural program of NIDDK. AW, MGD, and AES were also supported by the intramural program of NIMHD. AW also received support from the NIMHD William G. Coleman Minority Health and Health Disparities Research Innovation Award. MGD was also supported by Institute of Global Health Equity Research, University of Global Health Equity, Rwanda. MFH-R was supported by City University of New York, New York.
Conflict of interest
The authors declare that the research was conducted in the absence of any commercial or financial relationships that could be construed as a potential conflict of interest.
Publisher's note
All claims expressed in this article are solely those of the authors and do not necessarily represent those of their affiliated organizations, or those of the publisher, the editors and the reviewers. Any product that may be evaluated in this article, or claim that may be made by its manufacturer, is not guaranteed or endorsed by the publisher.
Supplementary material
The Supplementary Material for this article can be found online at: https://www.frontiersin.org/articles/10.3389/fpubh.2022.941086/full#supplementary-material
References
1. International Diabetes Federation. Diabetes Atlas. 10th ed. Brussels: International Diabetes Federation (2021).
2. Wagner R, Heni M, Tabák AG, Machann J, Schick F, Randrianarisoa E, et al. Pathophysiology-based subphenotyping of individuals at elevated risk for type 2 diabetes. Nat Med. (2021) 27:49–57. doi: 10.1038/s41591-020-1116-9
3. Kibirige D, Lumu W, Jones AG, Smeeth L, Hattersley AT, Nyirenda MJ. Understanding the manifestation of diabetes in sub Saharan Africa to inform therapeutic approaches and preventive strategies: a narrative review. Clin Diabetes Endocrinol. (2019) 5:2. doi: 10.1186/s40842-019-0077-8
4. Amoah ASD, Gaillard T, Osei K. Insulin resistance, beta cell function and cardiovascular risk factors in Ghanaians with varying degrees of glucose tolerance. Ethn Dis. (2002) 12:10–7.
5. Ishimwe MCS, Wentzel A, Shoup EM, Osei-Tutu NH, Hormenu T, Patterson AC, et al. Beta-cell failure rather than insulin resistance is the major cause of abnormal glucose tolerance in Africans: insight from the Africans in America study. BMJ Open Diabetes Res Care. (2021) 9:e002447. doi: 10.1136/bmjdrc-2021-002447
6. Gill GEP, Dedicoat M, Tesfaye S. The variable African diabetic phenotype: tales from the north and the south. African J Diabetes. (2010) 18:12–4.
7. Ji T, Zhang L, Tang Z, Sun F, Li Y, Ma L. Prevalence of normal-weight obesity in community-dwelling Chinese older adults: results from the Beijing longitudinal study of aging. Diabetes Metab Syndr Obes. (2020) 13:1611–7. doi: 10.2147/DMSO.S246884
8. Kim MK, Han K, Kwon HS, Song KH, Yim HW, Lee WC, et al. Normal weight obesity in Korean adults. Clin Endocrinol. (2014) 80:214–20. doi: 10.1111/cen.12162
9. Kapoor N, Lotfaliany M, Sathish T, Thankappan KR, Thomas N, Furler J, et al. Prevalence of normal weight obesity and its associated cardio-metabolic risk factors - Results from the baseline data of the Kerala Diabetes Prevention Program (KDPP). PLoS ONE. (2020) 15:e0237974. doi: 10.1371/journal.pone.0237974
10. Marques-Vidal P, Pécoud A, Hayoz D, Paccaud F, Mooser V, Waeber G, et al. Normal weight obesity: relationship with lipids, glycaemic status, liver enzymes and inflammation. Nutr Metab Cardiovasc Dis. (2010) 20:669–75. doi: 10.1016/j.numecd.2009.06.001
11. Madeira FB, Silva AA, Veloso HF, Goldani MZ, Kac G, Cardoso VC, et al. Normal weight obesity is associated with metabolic syndrome and insulin resistance in young adults from a middle-income country. PLoS One. (2013) 8:e60673. doi: 10.1371/journal.pone.0060673
12. Romero-Corral A, Somers VK, Sierra-Johnson J, Korenfeld Y, Boarin S, Korinek J, et al. Normal weight obesity: a risk factor for cardiometabolic dysregulation and cardiovascular mortality. Eur Heart J. (2010) 31:737–46. doi: 10.1093/eurheartj/ehp487
13. Ramsaran C, Maharaj RG. Normal weight obesity among young adults in Trinidad and Tobago: prevalence and associated factors. Int J Adolesc Med Health. (2017) 29. doi: 10.1515/ijamh-2015-0042
14. Dagenais GR, Gerstein HC, Zhang X, McQueen M, Lear S, Lopez-Jaramillo P, et al. Variations in diabetes prevalence in low-, middle-, and high-income countries: results from the prospective urban and rural epidemiological study. Diabetes Care. (2016) 39:780–7. doi: 10.2337/dc15-2338
15. Masconi K, Matsha TE, Erasmus RT, Kengne AP. Independent external validation and comparison of prevalent diabetes risk prediction models in a mixed-ancestry population of South Africa. Diabetol Metab Syndr. (2015) 7:42. doi: 10.1186/s13098-015-0039-y
16. Zhang L, Zhang Z, Zhang Y, Hu G, Chen L. Evaluation of Finnish Diabetes Risk Score in screening undiagnosed diabetes and prediabetes among U.S. adults by gender and race: NHANES 1999-2010. PLoS ONE. (2014) 9:e97865. doi: 10.1371/journal.pone.0097865
17. Staimez LR DM, Ali MK, Mohan V, Hanson RL, Narayan V. Tale of two Indians: Heterogenenity in type 2 diabetes pathophysiology. Diabetes Metab Res Rev. (2019) 35:e3192. doi: 10.1002/dmrr.3192
18. Griffin SJ, Little PS, Hales CN, Kinmonth AL, Wareham NJ. Diabetes risk score: towards earlier detection of type 2 diabetes in general practice. Diabetes Metab Res Rev. (2000) 16:164–71. doi: 10.1002/1520-7560(200005/06)16:3<164::aid-dmrr103>3.0.co;2-r
19. Bergmann A, Li J, Wang L, Schulze J, Bornstein SR, Schwarz PE, et al. simplified Finnish diabetes risk score to predict type 2 diabetes risk and disease evolution in a German population. Horm Metab Res. (2007) 39:677–82. doi: 10.1055/s-2007-985353
20. Kulkarni M, Foraker RE, McNeill AM, Girman C, Golden SH, Rosamond WD, et al. Evaluation of the modified FINDRISC to identify individuals at high risk for diabetes among middle-aged white and black ARIC study participants. Diabetes Obes Metab. (2017) 19:1260–6. doi: 10.1111/dom.12949
21. Al Khalaf MM, Eid MM, Najjar HA, Alhajry KM, Doi SA, Thalib L. Screening for diabetes in Kuwait and evaluation of risk scores. East Mediterr Health J. (2010) 16:725–31. doi: 10.26719/2010.16.7.725
22. Al-Lawati JA, Tuomilehto J. Diabetes risk score in Oman: a tool to identify prevalent type 2 diabetes among Arabs of the Middle East. Diabetes Res Clin Pract. (2007) 77:438–44. doi: 10.1016/j.diabres.2007.01.013
23. Baan CA, Ruige JB, Stolk RP, Witteman JC, Dekker JM, Heine RJ, et al. Performance of a predictive model to identify undiagnosed diabetes in a health care setting. Diabetes Care. (1999) 22:213–9. doi: 10.2337/diacare.22.2.213
24. Bindraban NR, van Valkengoed IG, Mairuhu G, Holleman F, Hoekstra JB, Michels BP, et al. Prevalence of diabetes mellitus and the performance of a risk score among Hindustani Surinamese, African Surinamese and ethnic Dutch: a cross-sectional population-based study. BMC Public Health. (2008) 8:271. doi: 10.1186/1471-2458-8-271
25. Mbanya JC, Motala AA, Sobngwi E, Assah FK, Enoru ST. Diabetes in sub-Saharan Africa. Lancet. (2010) 375:2254–66. doi: 10.1016/S0140-6736(10)60550-8
26. Hobabagabo AF, Osei-Tutu NH, Hormenu T, Shoup EM, DuBose CW, Mabundo LS, et al. Improved detection of abnormal glucose tolerance in Africans: the value of combining hemoglobin A(1c) with glycated albumin. Diabetes Care. (2020) 43:2607–13. doi: 10.2337/dc20-1119
27. Hormenu T, Shoup EM, Osei-Tutu NH, Hobabagabo AF, DuBose CW, Mabundo LS, et al. Stress measured by allostatic load varies by reason for immigration, age at immigration, and number of children: the Africans in America Study. Int J Environ Res Public Health. (2020) 17:4533. doi: 10.3390/ijerph17124533
28. Jagannathan R, DuBose CW, Mabundo LS, Chung ST, Ha J, Sherman A, et al. The OGTT is highly reproducible in Africans for the diagnosis of diabetes: Implications for treatment and protocol design. Diabetes Res Clin Pract. (2020) 170:108523. doi: 10.1016/j.diabres.2020.108523
29. Obesity and Overweight [Fact Sheet]. World Health Organization. (2020). Available online at: https://www.who.int/news-room/fact-sheets/detail/obesity-and-overweight (accessed July 13, 2020).
30. Kabakambira JD, Baker RL Jr, Briker SM, Courville AB, Mabundo LS, DuBose CW, et al. Do current guidelines for waist circumference apply to black Africans? Prediction of insulin resistance by waist circumference among Africans living in America BMJ Glob Health. (2018) 3:e001057. doi: 10.1136/bmjgh-2018-001057
31. O'Connor MY, Thoreson CK, Ricks M, Courville AB, Thomas F, Yao J, et al. Worse cardiometabolic health in African immigrant men than African American men: Reconsideration of the healthy immigrant effect. Metab Syndr Relat Disord. (2014) 12:347–53. doi: 10.1089/met.2014.0026
32. Association AD. Standards of medical care in diabetes - 2022. Diabetes Care. (2022) 45:S1–2. doi: 10.2337/dc22-Sint
33. Matsuda M DR. Insulin sensitivity indices obtained from oral glucose tolerance testing: comparison with the euglycemic insulin clamp. Diabetes Care. (1999) 22:1462–70. doi: 10.2337/diacare.22.9.1462
34. Friedewald WT LR, Fredrickson DS. Estimation of the concentration of low-density lipoprotein cholesterol in plasma, without use of the preparative ultracentrifuge. Clin Chem. (1972) 18:499–502. doi: 10.1093/clinchem/18.6.499
35. Mugeni R, Aduwo JY, Briker SM, Hormenu T, Sumner AE, Horlyck-Romanovsky MF, et al. Review of diabetes prediction equations in African descent populations. Front Endocrinol. (2019) 10:663. doi: 10.3389/fendo.2019.00663
36. Spijkerman AMW YM, Griffin SJ, Dekker JM, Nijpels G, Wareham NJ. The performance of a risk score as a screening test for undiagnosed hyperglycemia in ethnic minority groups: data from the 1999 health survey for England. Diabetes Care. (2004) 27:116–22. doi: 10.2337/diacare.27.1.116
37. Schwarz PEH LJ, Reimann M, Schutte AE, Bergmann A, Hanefeld M, Bornstein SR, et al. The finnish diabetes risk score is associated with insulin resistance and progression towards type 2 diabetes. JCEM. (2009) 94:920–6. doi: 10.1210/jc.2007-2427
38. Meijnikman AS, De Block CEM, Verrijken A, Mertens I, Van Gaal LF. Predicting type 2 diabetes mellitus: a comparison between the FINDRISC score and the metabolic syndrome. Diabetol Metab Syndr. (2018) 10:12. doi: 10.1186/s13098-018-0310-0
39. Flynn S MS, Buckley C, Junker K, Phillips C, Harrington J. Comparing non-invasive diabetes risk scores for detecting patients in clinical practice: a cross-sectional validation study. HRB Open Res. (2021) 4:70. doi: 10.12688/hrbopenres.13254.1
40. Force USPST, Davidson KW, Barry MJ, Mangione CM, Cabana M, Caughey AB, et al. Screening for prediabetes and type 2 diabetes: US preventive services task force recommendation statement. JAMA. (2021) 326:736–43. doi: 10.1001/jama.2021.12531
41. Ephraim RKD, Owusu VB, Asiamah J, Mills A, Abaka-Yawson A, Kpene GE, et al. Predicting type 2 diabetes mellitus among fishermen in Cape Coast: a comparison between the FINDRISC score and the metabolic syndrome. J Diabetes Metab Disord. (2020) 19:1317–24. doi: 10.1007/s40200-020-00650-w
42. Joffe B PV, Wing R, Raal F, Seftel H. Pathogenesis of non-insulindependent diabetes mellitus in the black population of southern Africa. Lancet. (1992) 340:460–2. doi: 10.1016/0140-6736(92)91777-6
43. Hariri S YP, Qureshi N, Valdez R, Scheuner MT, Khoury MJ. Family history of type 2 diabetes: a population-based screening tool for prevention? Genet Med. (2006) 8:102–8. doi: 10.1097/01.gim.0000200949.52795.df
44. InterAct Consortium SR, Langenberg C, Sharp SJ, Franks PW, Rolandsson O, Drogan D, et al. The link between family history and risk of type 2 diabetes is not explained by anthropometric, lifestyle or genetic risk factors: the EPIC-InterAct study. Diabetologia. (2013) 56:60–9. doi: 10.1007/s00125-012-2715-x
45. Wilson PW MJ, Sullivan L, Fox CS, Nathan DM, D'Agostino RB Sr. Prediction of incident diabetes mellitus in middle-aged adults: the Framingham Offspring Study. Arch Intern Med. (2007) 167:1068–74. doi: 10.1001/archinte.167.10.1068
46. Sub-Saharan African Immigrants in the United States: Migration Policy Institute 2019. (2019). Available online at: https://www.migrationpolicy.org/article/sub-saharanafrican-immigrants-united-states-2019. (accessed May 11, 2022).
47. Stern MP WK, Haffner SM. Identification of persons at high risk for type 2 diabetes mellitus: do we need the oral glucose tolerance test? Ann Intern Med. (2002) 136:575–81. doi: 10.7326/0003-4819-136-8-200204160-00006
48. Christensen DL, Faurholt-Jepsen D, Faerch K, Mwaniki DL, Boit MK, Kilonzo B, et al. Insulin resistance and beta-cell function in different ethnic groups in Kenya: the role of abdominal fat distribution. Acta Diabetol. (2014) 51:53–60. doi: 10.1007/s00592-013-0474-x
49. Mugeni R, Hormenu T, Hobabagabo A, Shoup EM, DuBose CW, Sumner AE, et al. Identifying Africans with undiagnosed diabetes: Fasting plasma glucose is similar to the hemoglobin A1C updated Atherosclerosis Risk in Communities diabetes prediction equation. Prim Care Diabetes. (2020) 14:501–7. doi: 10.1016/j.pcd.2020.02.007
50. Creatore MI, Moineddin R, Booth G, Manuel DH, DesMeules M, McDermott S, et al. Age- and sex-related prevalence of diabetes mellitus among immigrants to Ontario, Canada. Cmaj. (2010) 182:781–9. doi: 10.1503/cmaj.091551
51. Y ADA. The burden of undiagnosed diabetes mellitus in adult African population: a systematic review and meta-analysis. J Diabetes Res. (2019) 2019:4134937. doi: 10.1155/2019/4134937
52. Muazu SB, Okpe I, Anumah F. The prevalence and characteristics of latent autoimmune diabetes in adults subset among type two diabetes mellitus patients in Northern Nigeria. Ann Afr Med. (2016) 15:163–70. doi: 10.4103/1596-3519.194277
Keywords: type 2 diabetes, risk score, African (Black) diaspora, β-cell failure, insulin resistance, diabetes screening
Citation: Wentzel A, Patterson AC, Duhuze Karera MG, Waldman ZC, Schenk BR, DuBose CW, Sumner AE and Horlyck-Romanovsky MF (2022) Non-invasive type 2 diabetes risk scores do not identify diabetes when the cause is β-cell failure: The Africans in America study. Front. Public Health 10:941086. doi: 10.3389/fpubh.2022.941086
Received: 11 May 2022; Accepted: 19 August 2022;
Published: 23 September 2022.
Edited by:
Paolo Vineis, Imperial College London, United KingdomReviewed by:
Devjit Tripathy, The University of Texas Health Science Center at San Antonio, United StatesRashmi Prasad, Lund University, Sweden
Copyright © 2022 Wentzel, Patterson, Duhuze Karera, Waldman, Schenk, DuBose, Sumner and Horlyck-Romanovsky. This is an open-access article distributed under the terms of the Creative Commons Attribution License (CC BY). The use, distribution or reproduction in other forums is permitted, provided the original author(s) and the copyright owner(s) are credited and that the original publication in this journal is cited, in accordance with accepted academic practice. No use, distribution or reproduction is permitted which does not comply with these terms.
*Correspondence: Annemarie Wentzel, annemarie.wentzel@nwu.ac.za; Margrethe F. Horlyck-Romanovsky, margrethehr@brooklyn.cuny.edu