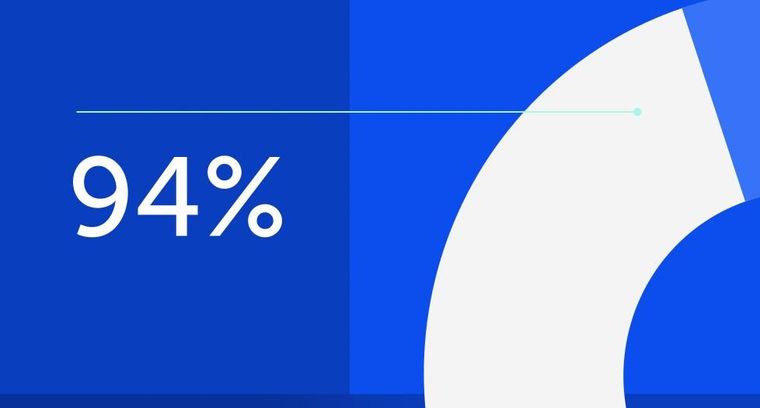
94% of researchers rate our articles as excellent or good
Learn more about the work of our research integrity team to safeguard the quality of each article we publish.
Find out more
ORIGINAL RESEARCH article
Front. Public Health, 08 September 2022
Sec. Life-Course Epidemiology and Social Inequalities in Health
Volume 10 - 2022 | https://doi.org/10.3389/fpubh.2022.930798
This article is part of the Research TopicPublic Health for Chronic Kidney Disease Prevention and CareView all 5 articles
Background: Understanding renal function state transition risk and associated factors in community residences is vital for appropriate preventive and care actions. We aim to investigate factors affecting renal function state transitions through 10-year longitudinal community screening surveys.
Methods: The prospective cohort study included participants who attended the screening program ≥2 times from 2001 to 2009 and were divided into two cohorts: those with baseline estimated glomerular filtration rate (eGFR) ≥60 (n = 46,278) and those with eGFR 59–30 mL/min/1.73 m2 (n = 4,656). We applied the illness-death model to identify associated factors with eGFR <60 and death for the cohort with baseline eGFR ≥60 and eGFR <30 and death for that with baseline eGFR ≥59–30.
Results: Among the followed-up participants, 3,018 (6.5%) in the cohort of baseline eGFR ≥60 mL/min/1.73 m2 and 322 (6.9%) in the cohort of eGFR 59–30 mL/min/1.73 m2 experienced renal function state transition during a median over 7-year follow-up. Besides eGFR and grade of proteinuria, diabetes mellitus (adding nearly 50% hazard rate) is the main factor associated with both state transitions. Other early-phase eGFR state transition risk factors were metabolic syndrome score, triglyceride, uric acid, fasting blood sugar, and high-density lipoprotein cholesterol. Males, poor hemoglobin, high triglyceride, and high low-density lipoprotein cholesterol were all linked with the late-phase eGFR state transition hazard rate.
Conclusion: The study developed the state transition functions for community participants with varying renal function levels. Further actions to develop precision screening plans and services that incorporate personal risk factors and state transition risks are necessary.
Chronic kidney disease (CKD) is a global public health problem accounting for 11.1% of the world population (1). CKD, without appropriate management, could rapidly progress to end-stage kidney disease (ESKD), requiring renal replacement therapy, which may incur substantial financial burdens to governments. According to the latest annual report of the United States Renal Data System, the annual ESKD prevalence (per million population) average yearly increased by 37.5% in reported countries from 2009 to 2018 (2); thus, seeking a sustainable development strategy to release these pressures is essential. Recent Kidney Disease: Improving Global Outcomes Controversies Conference emphasized the importance of early identifying risk stratification for renal function progression and delaying the time to advanced stages (3).
The annual average estimated glomerular filtration rate (eGFR) decline in healthy subjects is 0.97 ± 0.02 ml/min/1.73m2 per year (4) but could be more rapid when patients have different comorbidities and primary renal disorders (5). However, even for individuals with the same underlying cause of renal injury or degree of functional impairment, the eGFR declines could be highly variable. Early identification and management of people based on risks for CKD stage transition are essential worldwide. Previous epidemiological studies have reported that aristolochic acid digestion, diabetes, hypertension, proteinuria, hyperlipidemia, hyperuricemia, smoking habit, advanced age, male sex, and metabolic syndrome (MetS) were factors associated with CKD development and progression (6–9). Moreover, several CKD progression prediction models have been proposed (10–13), of which the endpoints were eGFR lower than 60 mL/min/1.73 m2, doubling of serum creatinine, 20% decrease in eGFR, and ESKD requiring renal replacement therapy. However, information on factors associated with state transition in renal function progression is quite a few especially in the community. The use of state transition as an endpoint can help healthcare providers easily communicate with patients and strengthen staged CKD prevention and management. Therefore, we analyzed longitudinal population-based community screening data to elucidate the factors influencing early- and late-phase eGFR state changes using the illness-death model.
A prospective cohort design was developed for participants aged ≥20 years, using a community-based multiple screening program in Keelung, the northernmost city of Taiwan. This screening program, the Keelung Community-based Integrated Screening (KCIS) program, was implemented from 1999 to 2009. The study used data from the screening results from 2001 to 2009 due to the lack of urine protein tests in the early 2 years. Details regarding the study design and implementation of this program prevalence have been reported elsewhere (14, 15). The CKD incidence and prevalence detected by the annual screening program have also been broadly noted (16). In brief, adults residing in Keelung and eligible for the KCIS program were invited annually for screening non-communicable diseases, such as cancers, diabetes, hypertension, dyslipidemia, and renal disease. However, participants may reattend the screening program at different year intervals. This program was governed by the Health Bureau of Keelung City, Taiwan, and approved by the local ethical committee of the Health Bureau of Keelung City. Written informed consent was received from all participants in each screening activity.
In the KCIS program, information was collected at each screening round, including data from a series of medical tests for blood, urine, body measurements, functional assessments, and medical history (questionnaire). The data also included anthropometric measures at the baseline, such as body mass index and waist circumference; biochemical variables, such as spot urine analysis using the dipstick method and serum creatinine, low-density lipoprotein cholesterol (LDL), high-density lipoprotein cholesterol (HDL), albumin, hemoglobin, uric acid, and fasting blood sugar levels; and lifestyle factors, such as alcohol intake, smoking, betel nut chewing, and physical activity. Participants were classified as drinkers and non-drinkers of alcohol, smokers and non-smokers, betel nut chewers and non-chewers, and regularly performing and not performing exercise according to drinking, smoking, betel nut chewing, and physical activity. Individual comorbidity included the presence of diabetes mellitus (DM), hypertension, and coronary artery disease (CAD). Moreover, proteinuria severity according to the spot urine dipstick analysis was recorded as follows: grade 0, absent; grade 1 (trace), 15–30 mg/dL; grade 2 (1+), 30–100 mg/dL; grade 3 (2+), 100–300 mg/dL; grade 4 (3+), 300–1,000 mg/dL; and grade 5 (4+), >1,000 mg/dL.
MetS contains five components. It is defined according to the National Cholesterol Education Program Adult Treatment Panel III criteria (Expert Panel on Detection Evaluation and Treatment of High Blood Cholesterol In Adults, 2001) (17). MetS was defined when a participant exhibited more than three components which included the following: central obesity (waist circumference ≥80 cm for women and ≥90 cm for men), elevated blood pressure (systolic blood pressure ≥130 mmHg or diastolic blood pressure ≥85 mmHg), hyperglycemia (fasting glucose level ≥100 mg/dL), hypertriglyceridemia (serum triglyceride level ≥150 mg/dL), and low HDL (serum HDL level <50 mg/dL for women and <40 mg/dL for men). The MetS score was calculated as the sum of the scores for the presence of metabolic components.
The eGFR values were calculated by age, sex, and serum creatinine through CKD-EPI equation (18). Participants who attended the KCIS program ≥2 times from 2001 to 2009 were categorized into the groups of eGFR ≥60 mL/min/1.73 m2 and eGFR 59–30 mL/min/1.73 m2 based on their values at the first screening. We defined the appearance of eGFR <60 as early-phase transition and <30 mL/min/1.73 m2 as late-phase transition at consequent screening visits for the groups of eGFR ≥60 mL/min/1.73 m2 and eGFR 59–30 mL/min/1.73 m2, retrospectively. Death between 2001 and 2010, ascertained by the National Death Registry of Taiwan, was considered an absorbed state.
Baseline data are described using mean ± standard deviation or median with interquartile range (IQR) as appropriate for continuous variables and proportions for categorical variables. Because most of the exact time of eGFR state transition is unknown, we recorded age at the first screening visit, age at screening visit before eGFR state transition, age at screening visit after eGFR state transition, and age at death or the latest screening visit for both cohorts. The timing of any eGFR state transition between two subsequent screenings is usually unknown in almost all patients, and we considered the time to transition as interval-censored. Patients with CKD may be more likely to suffer from death than the eGFR stage transitions; we adopted a competing risk analysis with the illness-death model to fit the Weibull distribution. The illness-death model simultaneously estimates three-state (eGFR ≥60, eGFR <60, and death; eGFR 59–30, eGFR <30, and death) transition intensities by fitting Weibull regression functions using the Levenberg–Marquardt's algorithm to maximize the (penalized) likelihood (19, 20). This model firstly produces estimates for intensity and regression parameters. Then, through continuous estimates of intensity by the model could obtain relevant quantities, such as transition probabilities, cumulative probability, and life expectancies (21). To explore the relationship between continuous biochemical data and each eGFR transition, we categorized them by quartile and put the least quartile group as the reference group. All covariates besides age were forced entered to model development except the variable of betel nut chewing because of its small portion in the cohort with eGFR 59–30 mL/min/1.73 m2. We displayed model results by hazard ratio (HR) with 95% confidence intervals (CIs). Based on the developed models, we could apply the model to understand the risk of eGFR state transition based on participants' characteristics. The models predicted consequent annual transition probabilities by changing selecting participants' characteristics (male with DM, male without DM, female with DM, and female without DM) in a free participant for the defined state at 45 years old. The transition probability curves of the selecting participant's characteristics were compared to understand the participant's transition risks. A two-tailed p of <0.05 was considered statistically significant. All analyses were performed using R Studio (version 1.2.5042) with “SmoothHazard” packages (22) and SAS (version 9.4; SAS Institute Inc., Cary, NC, USA).
This study included 46,278 participants with eGFR ≥60 mL/min/1.73 m2 and 4,656 participants with eGFR 59–30 mL/min/1.73 m2. Of the observed participants, 3,018 (6.1%) in the group of eGFR ≥60 mL/min/1.73 m2 and 322 (6.9%) in the group of eGFR 59–30 mL/min/1.73 m2 experienced eGFR state transition during the follow-up period (Figure 1). The study population was slightly older and more female than the overall Keelung population (data not shown). The participant characteristics of our study cohorts are summarized in Table 1. Participants with eGFR 59–30 mL/min/1.73 m2 were significantly older (mean age, 68.2 ± 10.1 vs. 47.4 ± 13.5 years), more likely to be men (46.8 vs. 35.0%), and had DM (12.9 vs. 4.0%), hypertension (37.5 vs. 11.3%), and CAD (17.7 vs. 4.9%) than those with eGFR ≥60 mL/min/1.73 m2.
In the participants with eGFR ≥60 mL/min/1.73 m2, the model identified the curve relationship between baseline transition intensities and age (Figure 2A). The transition intensities of eGFR <60 are higher than the transition intensities of death across observed ages. The differences between transition intensities of eGFR <60 and death gradually increase with rising age, especially after 60 years. On the contrary, the age-specific baseline transition intensities of eGFR <30 in the participants with eGFR 59–30 are higher than death only before age 65 years (Figure 2B). The annual transition intensities of death directly from the baseline state rapidly raised in the cohort of eGFR 59–30 compared to eGFR ≥60, causing more than six times (19.4 vs. 3.0%) immediate risk for death at age 85 years.
Figure 2. Estimated baseline transition intensity by illness-death model for (A) estimated glomerular filtration rate (eGFR) <60 state and death transitions in the cohort with baseline eGFR ≥60 ml/min/1.73 m2 and (B) eGFR <30 state and death transitions in the cohort with baseline eGFR 59–30 ml/min/1.73 m2. Transition 01 represents the transition intensity from the baseline state to the selected state. Transition 02 exhibits the transition intensity from the baseline state to death. Transition 12 displays the transition to death after transiting the selected state from the baseline state.
The cohort with eGFR ≥60 mL/min/1.73 m2 was followed for a median time of 7.2 years (IQR, 5.3–8.3) and contributed a median-censored time of 4.0 years (IQR, 2.4–6.0). The significant factors associated with increased risk of early-phase eGFR state transition (transition to eGFR <60 mL/min/1.73 m2) were DM, MetS, rising quartile levels in triglyceride, uric acid, grad of proteinuria, and reducing quartile levels in fasting blood sugar, HDL, and eGFR (Table 2). In addition, being male sex, with CAD, a smoker, rising quartile levels in triglyceride, reduced quartile levels in hemoglobin, total cholesterol, triglyceride, and LDL were associated with an increased risk of death direct from eGFR ≥60 mL/min/1.73 m2.
Table 2. Factors associated with transiting to estimated glomerular filtration rate (eGFR) <60 mL/min/1.73 m2 or death in the cohort with baseline eGFR ≥60 mL/min/1.73 m2.
Patients with eGFR 59–30 mL/min/1.73 m2 were followed for an average of 7.7 years (IQR, 5.6–8.4), and a median-censored time of 3.9 years was recorded (IQR, 2.1–5.8). The significant independent factors for late-phase CKD state transition were male, DM, quartile of hemoglobin, quartile of fasting blood sugar, quartile of triglyceride, quartile of LDL, grade of proteinuria, and eGFR quartile (Table 3). However, only being a smoker, a low quartile of albumin, and a high grade of proteinuria were significantly associated with an excessive risk of direct transiting to death in patients with eGFR 59–30 mL/min/1.73 m2. The hazard functions for eGFR state and death transitions that developed based on selected covariates are displayed in Supplementary material.
Table 3. Factors associated with transiting to estimated glomerular filtration rate (eGFR) <30 mL/min/1.73 m2 or death in the cohort with baseline eGFR 59–30 mL/min/1.73 m2.
The developed model predicted the risks of defined eGFR state transition at age 46–65 in participants aged 45 and free for the state. The early-phase eGFR state transition model represents that regardless of which sex, DM compared with non-DM added a 0.3-time risk to transit to eGFR <60 in the next 20 years (Figure 3A). However, both sex and DM impact eGFR <30 transition risks. The late-phase eGFR state transition model displays that males with DM show a substantially doubled eGFR <30 transition risk in the next 20 years than females without DM (Figure 3B).
Figure 3. The model predicted age 46–65 CKD state transition risks at age 45 by sex and diabetes mellitus. (A) Predicted eGFR <60 transition risk and (B) predicted eGFR <30 transition risk. We set the reference values for factors other than sex and diabetes in the prediction models, said without coronary artery disease, selected life habitats, metabolic syndrome, and the lowest quartile of all baseline laboratory data. Despite the large study sample size and a median 7-year follow-up, the predicted risk may lose accuracy when it is extrapolated for a long period of time.
This community-based study is one of the few studies that quantified transition intensity for participants with different eGFR states and clarified factors influencing state transitions after accounting for competing risks of death. Our findings revealed that DM, fasting blood sugar, triglyceride, proteinuria grade, and eGFR were associated with early- and late-phase eGFR state transitions. However, uric acid and HDL were only linked to the early-phase eGFR state transition, whereas male, hemoglobin, and LDL were only related to the late-phase eGFR state transition. In addition, the developed models provide precisely eGFR state risk quantification at various ages by inputting participants' characteristics.
The primary tasks advocated by the Kidney Disease Outcomes Quality Initiative clinical guidelines are slow progression and avoiding immature death (23). Although the factors affecting clinical renal function deterioration have been identified during past decades, only a few pieces of evidence from community residents are available (24). The results of the current study show varying age-specific baseline hazard rates of the eGFR state and death transitions in community residences. The findings could be used to arrange different CKD screening activities for risky populations, such as shortening screening intervals and adopting more accurate tests (e.g., cystatin c and urine albumin creatine ratio). Further actions are needed to develop more precise CKD screening and referral policies based on the age-specific transition risks.
DM in a community is the main attributor for both eGFR state transitions. Routinely performing screenings by urinary and serum creatinine tests is the standard procedure for detecting early CKD transition. Although a recent study reported a dramatically improving trend in annual proportion of ≥ one-time serum creatinine or microalbuminuria tests in DM care (25), optimal screening intervals of renal function are still unknown and worthy of further study. Previous studies strongly support the finding that MetS is a crucial risk factor only for the early phase of renal function decline. Metabolic disorders are prevalent globally because of unhealthy lifestyles, such as increasing fast-food consumption and physical inactivity (26). Further research exploring the effects of correcting unhealthy lifestyles on the risk of proteinuria and early-phase renal function progression is wanted.
The current study showed the dose-response association between uric acid levels and risks of CKD state transition, especially in early CKD transition. It is worthy to notice that even high uric acid levels under therapeutic criteria could elevate the risk of CKD transition. High uric acid may result in arteriolosclerosis, glomerular injury, and tubulointerstitial fibrosis, either directly triggering urate crystals or indirectly causing vascular injuries (27). One systemic review study supported that improving uric acid abnormality by uric acid-lowering therapy would prevent kidney function decline in patients with CKD (28). In addition, our findings put insights into whether maintaining uric acid lower than the therapeutic standard would delay CKD progression.
It is not surprising that subjects with more severe proteinuria and lower eGFR levels at baseline were associated with high-risk CKD state transition. Our observations suggest that subjects maintain a higher level of eGFR, and controlling urine protein in a normal range is more critical in the late- than early-phase CKD. Although the mechanisms are unknown, they may associate with a non-linear decline of the renal reserve capacity from healthy to different CKD states (29). Contrary to intuition, we found that higher eGFR levels were associated with a higher hazard of directly transiting to death from eGFR ≥60 mL/min/1.73 m2. The findings may be attributed to comparing the transition hazard to eGFR <60 under the competing risk model structure, which could not be directly explained as an overall mortality hazard. However, it also substantially reflects the importance of applying a competing risk model structure rather than standard survival models when one study would explore factors influencing early-phase CKD transition. Male and low hemoglobin levels were two determinants for increasing state transition intensities only in patients whose eGFR was 59–30 ml/min/1.73 m2. Anemia is one of the primary complications in patients with late CKD stage (30). Recent international real-world evidence supports our findings that a higher hemoglobin level could benefit renal function progression and survival (31). Notably, our study repeals that sex is not associated with early-phase CKD transition, which was different from most previous observations but similar to one study that was analyzed using the illness-death model (32, 33). One possible explanation is the illness-death model incorporates interval-censored information, and death after CKD state transition makes our estimation less-biased. Our results of further analysis by the cause-specific hazard model, males had both higher hazards transiting to eGFR <60 (HR: 1.14, 95% CI: 1.02–1.28) and death (HR: 1.85, 95% CI: 1.47–2.34) than the females (data not shown), support this viewpoint. However, the mechanisms of sex disparity in different phases of CKD progression and therapy are complex and usually involved in biological, cultural, and socioeconomic dimensions (33), which are beyond the scope of our study. Therefore, more research is needed to explore the effects of sex-specific hormones, behaviors, and social–cultural on these discrepancies.
The roles of lipid profiles on CKD progression have not been well-established. Similar to the conclusion from a recent review, we did not find a consistent relationship between cholesterol on risks of transition in different CKD states (34). In this study, HDL plays a more significant role in early CKD state transition, whereas higher LDL levels were associated with a more transition risk to the advanced CKD stage. Given that both cholesterols have several hundred specials and may carry different proteins, the only quantifying cholesterol might not reflect the variety of biological effects of heterogeneous HDL and LDL on CKD progression (35). Therefore, exploring functional components of both cholesterols on CKD progression in great detail and evaluating their usability in the future are suggested.
This study had several strengths. First, it examined the changes in CKD states in a large cohort of community individuals over a long period, which assessed a sufficient number of desired events. Second, a multistate transition model can offer more holistic details about the influence of risk factors on renal function decline state. Third, using an illness-death model with Weibull distribution can estimate the absolute risk of CKD state transition. However, some limitations should be appropriately declared. First, we used only one laboratory result to determine the participant's eGFR state, which may not be accurate. Then, this study did not collect medications and behaviors, which may affect the effect estimation of laboratory levels. Third, the dynamic and multidimensional observed states make it challenging to evaluate the prediction performance of the developed models. However, all confidence regions for the model parameters generated by a simulation-based approach are located within a reasonable range, which reflects that our results should meet in line with practical reliability. Fourth, although our parametric model makes it possible to do any prediction even beyond the following-up time, one may apply it in line with caution. Finally, current findings were observed in the Asia population, limiting the generalization to other races.
We documented independent factors of renal progression in a three-state transition model to classify the risks for community patients with different levels of eGFR. Moreover, we successfully developed two absolute risk functions for predicting renal function state transition. This information is beneficial for arranging more appropriate screening actions for participants with different risk characteristics of renal function state transitions and alerting physicians to early transfer the risky patients to receive more appropriate CKD management and care. The predicted probabilities by the developed models were particularly useful for high-risk screening attendees by arranging optimal screening intervals, avoiding substances-induced renal damage, and managing their chronic personal conditions.
The datasets presented in this article are not readily available because these data only could be analyzed under the government's regulations. Requests to access the datasets should be directed to https://chmuseum.klchb.gov.tw/default_2019.aspx.
The studies involving human participants were reviewed and approved by Ethical Committee of Health Bureau of Keelung City. The participants provided their written informed consent to participate in this study. All study procedures followed the principles of the Declaration of Helsinki.
M-HT and M-YL: manuscript drafting, had full access to all data in the study and take responsibility for data integrity and accuracy of the data analysis, and administrative, technical, or material support. C-YH, AY, TC, and SC: concept and design. M-HT, C-YH, AY, TC, and SC: acquisition, analysis, or interpretation of data. TC and S-JH: critical revision of the manuscript for important intellectual content. M-YL: statistical analysis. All authors contributed to the article and approved the submitted version.
We would like to thank the Taiwan Ministry of Science and Technology (Grant Number: MOST-108-2314-B-037-110) for supporting this work. The funders had no role in the design and conduct of the study, collection, management, analysis, and interpretation of the data, and preparation, review, or approval of the manuscript.
We thank the participants for contributing the required information to this study and Wallace Academic Editing for editing this manuscript. In addition, many thanks for the great technique supported by the team member in developing SmoothHazard R packages (Thomas Alexander Gerds, Célia Touraine, and Pierre Joly) and Yihuang Kang from National Sun Yat-sen University.
The authors declare that the research was conducted in the absence of any commercial or financial relationships that could be construed as a potential conflict of interest.
All claims expressed in this article are solely those of the authors and do not necessarily represent those of their affiliated organizations, or those of the publisher, the editors and the reviewers. Any product that may be evaluated in this article, or claim that may be made by its manufacturer, is not guaranteed or endorsed by the publisher.
The Supplementary Material for this article can be found online at: https://www.frontiersin.org/articles/10.3389/fpubh.2022.930798/full#supplementary-material
CKD, chronic kidney disease; CAD, coronary artery disease; CIs, confidence intervals; DM, diabetes mellitus; eGFR, estimated glomerular filtration rate; ESKD, end-stage kidney disease; HDL, high-density lipoprotein cholesterol; HR, hazard ratio; IQR, interquartile range; KCIS, Keelung Community-based Integrated Screening; LDL, low-density lipoprotein cholesterol; MetS, metabolic syndrome.
1. Jager KJ, Kovesdy C, Langham R, Rosenberg M, Jha V, Zoccali C. A Single Number for Advocacy and Communication—Worldwide More Than 850 Million Individuals Have Kidney Diseases. Oxford: Oxford University Press (2019). p. 1803–5. doi: 10.1093/ndt/gfz174
2. System USRD. 2020USRDS Annual Data Report: Epidemiology of kidney disease in the United States. National Institutes of Health, National Institute of Diabetes and Digestive and Kidney Diseases, Bethesda, MD (2020).
3. Shlipak MG, Tummalapalli SL, Boulware LE, Grams ME, Ix JH, Jha V, et al. The case for early identification and intervention of chronic kidney disease: conclusions from a Kidney Disease: improving global outcomes (KDIGO) controversies conference. Kidney Int. (2021) 99:34–47. doi: 10.1016/j.kint.2020.10.012
4. Cohen E, Nardi Y, Krause I, Goldberg E, Milo G, Garty M, et al. A longitudinal assessment of the natural rate of decline in renal function with age. J Nephrol. (2014) 27:635–41. doi: 10.1007/s40620-014-0077-9
5. Gansevoort RT, Correa-Rotter R, Hemmelgarn BR, Jafar TH, Heerspink HJ, Mann JF, et al. Chronic kidney disease and cardiovascular risk: epidemiology, mechanisms, and prevention. Lancet. (2013) 382:339–52. doi: 10.1016/S0140-6736(13)60595-4
6. Tsai WC, Wu HY, Peng YS, Ko MJ, Wu MS, Hung KY, et al. Risk factors for development and progression of chronic kidney disease: a systematic review and exploratory meta-analysis. Medicine. (2016) 95:e3013. doi: 10.1097/MD.0000000000003013
7. Staples AO, Greenbaum LA, Smith JM, Gipson DS, Filler G, Warady BA, et al. Association between clinical risk factors and progression of chronic kidney disease in children. Clin J Am Soc Nephrol. (2010) 5:2172–9. doi: 10.2215/CJN.07851109
8. McClellan WM, Flanders WD. Risk factors for progressive chronic kidney disease. J Am Soc Nephrol. (2003) 14(7 Suppl. 2):S65–70. doi: 10.1097/01.ASN.0000070147.10399.9E
9. Yang HY, Chen PC, Wang JD. Chinese herbs containing aristolochic acid associated with renal failure and urothelial carcinoma: a review from epidemiologic observations to causal inference. Biomed Res Int. (2014) 2014:569325. doi: 10.1155/2014/569325
10. Echouffo-Tcheugui JB, Kengne AP. Risk models to predict chronic kidney disease and its progression: a systematic review. PLoS Med. (2012) 9:e1001344. doi: 10.1371/journal.pmed.1001344
11. Taal MW, Brenner BM. Predicting initiation and progression of chronic kidney disease: developing renal risk scores. Kidney Int. (2006) 70:1694–705. doi: 10.1038/sj.ki.5001794
12. Tangri N, Stevens LA, Griffith J, Tighiouart H, Djurdjev O, Naimark D, Levin A, Levey AS. A predictive model for progression of chronic kidney disease to kidney failure. JAMA. (2011) 305:1553–9. doi: 10.1001/jama.2011.451
13. Johnson ES, Thorp ML, Platt RW, Smith DH. Predicting the risk of dialysis and transplant among patients with CKD: a retrospective cohort study. Am J Kidney Dis. (2008) 52:653–60. doi: 10.1053/j.ajkd.2008.04.026
14. Chen TH, Chiu YH, Luh DL, Yen MF, Wu HM, Chen LS, et al. Community-based multiple screening model: design, implementation, and analysis of 42,387 participants. Cancer. (2004) 100:1734–43. doi: 10.1002/cncr.20171
15. Chiu YH, Wu SC, Tseng CD, Yen MF, Chen TH. Progression of pre-hypertension, stage 1 and 2 hypertension (JNC 7): a population-based study in Keelung, Taiwan (Keelung Community-based Integrated Screening No. 9). J Hypertens. (2006) 24:821–8. doi: 10.1097/01.hjh.0000222750.82820.19
16. Tsai M-H, Hsu C-Y, Lin M-Y, Yen M-F, Chen H-H, Chiu Y-H, et al. Incidence, prevalence, and duration of chronic kidney disease in Taiwan: results from a community-based screening program of 106,094 individuals. Nephron. (2018). 140:175–84. doi: 10.1159/000491708
17. Kassi E, Pervanidou P, Kaltsas G, Chrousos G. Metabolic syndrome: definitions and controversies. BMC Med. (2011) 9:48. doi: 10.1186/1741-7015-9-48
18. Levey AS, Stevens LA, Schmid CH, Zhang YL, Castro AF III, Feldman HI, et al. A new equation to estimate glomerular filtration rate. Ann Intern Med. (2009) 150:604–12. doi: 10.7326/0003-4819-150-9-200905050-00006
19. Fachinotti V, Anca A, Cardona A. A method for the solution of certain problems in least squares. Int J Numer Method Biomed Eng. (2011) 27:595–607. doi: 10.1002/cnm.1324
20. Marquardt DW. An algorithm for least-squares estimation of nonlinear parameters. J Soc Industr Appl Math. (1963) 11:431–41. doi: 10.1137/0111030
21. Touraine C, Helmer C, Joly P. Predictions in an illness-death model. Stat Methods Med Res. (2016) 25:1452–70. doi: 10.1177/0962280213489234
22. Touraine C, Gerds TA, Joly P. The SmoothHazard Package for R: Fitting Regression Models to Interval-Censored Observations of Illness-Death Models. Copenhagen: University of Copenhagen, Department of Biostatistics (Research Report 13/12) (2013).
23. Levey AS, Coresh J, Bolton K, Culleton B, Harvey KS, Ikizler TA, et al. K/DOQI clinical practice guidelines for chronic kidney disease: evaluation, classification, and stratification. Am J Kidney Dis. (2002) 39(2 Suppl. 1):S1–266.
24. Janmaat CJ, van Diepen M, van Hagen CC, Rotmans JI, Dekker FW, Dekkers OM. Decline of kidney function during the pre-dialysis period in chronic kidney disease patients: a systematic review and meta-analysis. Clin Epidemiol. (2018) 10:613. doi: 10.2147/CLEP.S153367
25. Wang C-Y, Wu Y-L, Sheu WH-H, Tu S-T, Hsu C-C, Tai T-Y. Accountability and utilization of diabetes care from 2005 to 2014 in Taiwan. J Formosan Med Assoc. (2019) 118:S111–21. doi: 10.1016/j.jfma.2019.08.010
26. Saklayen MG. The global epidemic of the metabolic syndrome. Curr Hypertens Rep. (2018) 20:1–8. doi: 10.1007/s11906-018-0812-z
27. Johnson RJ, Nakagawa T, Jalal D, Sánchez-Lozada LG, Kang D-H, Ritz E. Uric acid and chronic kidney disease: which is chasing which? Nephrol Dial Transpl. (2013) 28:2221–8. doi: 10.1093/ndt/gft029
28. Tsukamoto S, Okami N, Yamada T, Azushima K, Yamaji T, Kinguchi S, et al. Prevention of kidney function decline using uric acid-lowering therapy in chronic kidney disease patients: a systematic review and network meta-analysis. Clin Rheumatol. (2021) 41:1–9. doi: 10.1007/s10067-021-05956-5
29. Barai S, Gambhir S, Prasad N, Sharma RK, Ora M. Functional renal reserve capacity in different stages of chronic kidney disease. Nephrology. (2010) 15:350–3. doi: 10.1111/j.1440-1797.2010.01291.x
30. Babitt JL, Lin HY. Mechanisms of anemia in CKD. J Am Soc Nephrol. (2012) 23:1631–34. doi: 10.1681/ASN.2011111078
31. Hoshino J, Muenz D, Zee J, Sukul N, Speyer E, Guedes M, et al. Associations of hemoglobin levels with health-related quality of life, physical activity, and clinical outcomes in persons with stage 3-5 nondialysis CKD. J Renal Nutr. (2020) 30:404–14. doi: 10.1053/j.jrn.2019.11.003
32. Boucquemont J, Metzger M, Combe C, Stengel B, Leffondre K, Group NS. Should we use standard survival models or the illness-death model for interval-censored data to investigate risk factors of chronic kidney disease progression? PLoS ONE. (2014) 9:e114839. doi: 10.1371/journal.pone.0114839
33. Neugarten J, Golestaneh L. Influence of sex on the progression of chronic kidney disease. In: Mayo Clinic Proceedings. vol. 94. Rochester, NY: Elsevier (2019). p. 1339–56.doi: 10.1016/j.mayocp.2018.12.024
34. Mathew RO, Rosenson RS, Lyubarova R, Chaudhry R, Costa SP, Bangalore S, et al. Concepts and controversies: lipid management in patients with chronic kidney disease. Cardiovasc Drugs Ther. (2021) 35:479–89. doi: 10.1007/s10557-020-07020-x
Keywords: chronic kidney disease, disease state, risk factor, illness-death model, risk prediction
Citation: Tsai M-H, Lin M-Y, Hsu C-Y, Yen AM-F, Chen TH-H, Chiu SY-H and Hwang S-J (2022) Factors associated with renal function state transitions: A population-based community survey in Taiwan. Front. Public Health 10:930798. doi: 10.3389/fpubh.2022.930798
Received: 28 April 2022; Accepted: 27 July 2022;
Published: 08 September 2022.
Edited by:
Boris Bikbov, Mario Negri Pharmacological Research Institute (IRCCS), ItalyReviewed by:
Cécile Couchoud, Agence de la Biomédecine, FranceCopyright © 2022 Tsai, Lin, Hsu, Yen, Chen, Chiu and Hwang. This is an open-access article distributed under the terms of the Creative Commons Attribution License (CC BY). The use, distribution or reproduction in other forums is permitted, provided the original author(s) and the copyright owner(s) are credited and that the original publication in this journal is cited, in accordance with accepted academic practice. No use, distribution or reproduction is permitted which does not comply with these terms.
*Correspondence: Shang-Jyh Hwang, c2pod2FuZ0BrbXUuZWR1LnR3
†These authors have contributed equally to this work
Disclaimer: All claims expressed in this article are solely those of the authors and do not necessarily represent those of their affiliated organizations, or those of the publisher, the editors and the reviewers. Any product that may be evaluated in this article or claim that may be made by its manufacturer is not guaranteed or endorsed by the publisher.
Research integrity at Frontiers
Learn more about the work of our research integrity team to safeguard the quality of each article we publish.