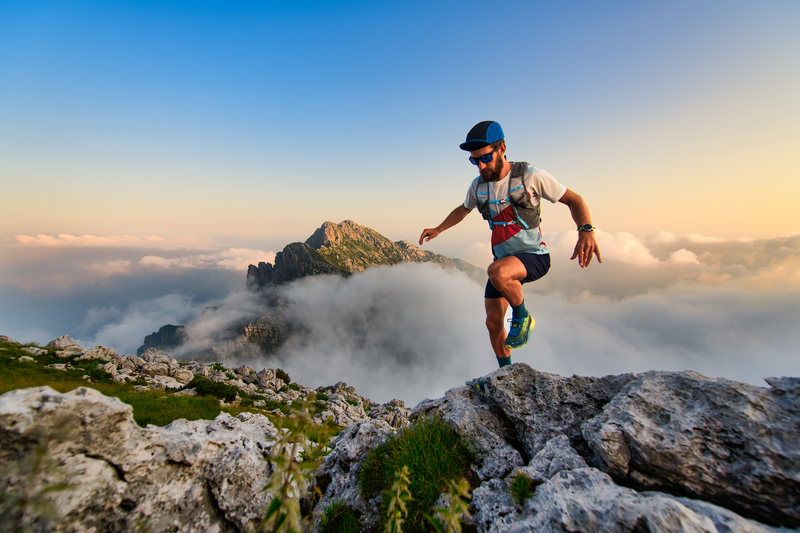
95% of researchers rate our articles as excellent or good
Learn more about the work of our research integrity team to safeguard the quality of each article we publish.
Find out more
ORIGINAL RESEARCH article
Front. Public Health , 15 June 2022
Sec. Public Health Education and Promotion
Volume 10 - 2022 | https://doi.org/10.3389/fpubh.2022.914592
This article is part of the Research Topic Myopia: Public Health challenges and interventions View all 15 articles
Purpose: To understand the latent classes and distribution of an adolescent eye care behavior, and to provide a basis for the formulation of appropriate adolescent vision health management interventions.
Methods: Information on eye behavior and eye health of primary and secondary school students in Wuhan was collected by multistage stratified cluster sampling. The latent class analysis (LCA) method was used to analyze the students' eye care behavior, and the latent class model (LCM) was built.
Results: A total of 6,130 students were enrolled in this study, of which 53.56% were males, aged from 6 to 17 years old, with an average age of 10.33 ± 2.60. The latent class results classified the adolescents' eye care behaviors into bad behaviors, moderate behaviors, and healthy behaviors. The model fitting results were as follows: Akaike Information Criterion (AIC) was 36,698.216, Bayesian Information Criterion (BIC) was 36,906.565, Adjusted Bayesian Information Criterion (aBIC) was 36,808.056, and entropy was 0.838.Compared with the healthy behaviors class, the bad behaviors class was more prevalent in high schools (p = 0.003), non-demonstration schools (p = 0.001), and most of this group had astigmatism (p = 0.002). The moderate behaviors class predominately consisted of females (p = 0.001), 15–17 years old (p = 0.005, 6~8 years old as the reference), from non-demonstration schools (p < 0.001), and most had myopia (p = 0.009).
Conclusion: There were differences in basic demographic characteristics, visual acuity development level, and family visual environment among different classes. In the management and intervention of an adolescent vision health, we should continue to promote the visual health management of adolescents based on visual monitoring and realize the early intervention and guidance of individuals in bad behaviors class.
Visual health refers to normal visual physiology and visual psychology and good visual social adaptation on the premise of not suffering from eye diseases and abnormal symptoms such as visual fatigue. An analysis of studies suggests that by 2050, nearly half of the world's population may be myopic, with up to 10% being highly myopic (1).The World Health Organization (WHO) lists myopia as one of the 5 eye diseases requiring priority elimination and improvement (2). At the same time, there are racial and ethnic differences in the levels and prevalence of myopia, both of which are higher in Asia than in other parts of the world (3).At present, the prevalence of myopia among adolescents in China is characterized by a high prevalence (4, 5), fast growth rate (6, 7), and early age onset (8). China has the most teenage myopia patients in the world (9). Some researchers predict that if no intervention measures are taken, the myopia rate of Chinese adolescents will reach 61.8% in 2030 (10).
Comprehensive eye care (CEC) aims to ensure that people have access to the ophthalmic health services that meet the needs of each stage of their lives, which includes visual loss prevention, due to poor eye care habits and behavior (11, 12). Although a small percentage of myopia is inherited, much more is simply caused by poor eye care habits and behavior (13–16). A large number of studies have shown that near work, incorrect reading, and writing posture, and prolonged use of electronic devices can lead to visual fatigue, altered refractive state, and myopia (17–20). In terms of daily life, sleep deprivation is a risk factor for the development of myopia in teenagers (21). A diet high in sugar and cholesterol can also contribute to myopia (22, 23). There is a wealth of epidemiological evidence about the amount of time spent outdoors, indicating that adequate time spent outdoors is one of the most important factors in protecting visual health (24–26), which may be due to vitamin D and dopamine (27–29). Given the close relationship between behavior and visual health (30), it is necessary to effectively identify the accumulation of vision-related risks in adolescents by exploring and studying heterogeneous subsets of related behaviors.
Although most studies have shown that visual health development is significantly correlated with behavior, most of these studies have grouped adolescents according to gender, age, and other conditions for analysis, and it is impossible to judge whether subgroups can be defined only by significant variables. To explore the visual health and behavioral development of adolescents, Wuhan city has carried out visual health management and monitoring for primary and secondary school students. In this study, a latent class model was established to determine the class attributes of adolescents' eye health behaviors and analyze their distribution characteristics, providing a scientific basis for understanding the relationship between adolescents' eye care changes and visual health development.
Data were collected from the vision prevention and treatment project for adolescents in Wuhan, which was reviewed and approved by the Ethics Committee of the School of Public Health, Wuhan University. This study was conducted in 2019 and used a multistage stratified cluster sampling approach to recruit participants. According to the basic information released by the Wuhan Education Bureau in 2017, there are 735,799 students in Wuhan. The sample size of the sample survey is calculated as follows:
N is the total sample number, and P is denoted as 0.50. If the sample population is large, the sampling size formula can be written as:
In general, α is denoted as 0.05 and K as 1.96. According to the statistical formula, it was estimated that 385 participants in each group were required. Considering grade differences, the sample size of this study is n≥12(grade)*385 ≈ 4,620, which means that the sample size needs to be >4,620 people.
Schools are divided by the Wuhan Education Bureau into vision health management demonstration schools and non-demonstration schools, and the classification standards are as follows: (1) Whether to carry out regular visual health management; (2) Whether to successfully apply for a municipal demonstration school; if both standards are met, the school will be regarded as a demonstration school of visual health. In consideration of geographical location (urban/rural region), whether it is a demonstration school or not, and the key age of myopia prevention and control, 140 schools in 14 districts (such as primary school, junior high school, and senior high school) were selected for this study, and a total of 6,130 students were enrolled.
This study adopted a self-made questionnaire as a survey tool, which development took reference from the Questionnaire of Vision Care Related Behavior for Students (AQVCRBS) (31). The results were filled in by students through the “Internet +” vision monitoring management application platform. The survey content included general demographic characteristics (sex, age, residence, education stage, school type);and eye care behaviors (near work, reading posture, time of electronics use, duration of sleep, eating habits, supplementation of vitamin A, outdoor exercise, eye exercises, non-sports training courses, eye muscle exercises). Each respondent completed a self-report questionnaire independently, and both the respondent and guardian signed informed consent forms.
The inclusion criteria for participants were as follows: (1) Students aged 6–17 years. (2) The legal guardian signed the informed consent. (3) Students without congenital eye diseases, such as congenital brain damage and visual impairment. (4)No neurological disorders, such as severe cognitive impairment.
The exclusion criteria for participants were as follows: (1) The legal guardian did not consent to participate in the vision test or related investigation. (2) Students with an incomplete investigation.
The results of vision monitoring will be reported by each school through the “Internet +” vision monitoring management platform and sent to the Wuhan Visual Prevention and Control Center. Visual acuity assessment uses the flat vision examination instrument, which has passed the approval and detection of relevant departments. Refractive inspection was performed according to the recommended desktop automatic computer optometry, and optometry equipment by the standard (ISO10342ophthalmic instrument-optometry) provisions. All physicians or investigators will be trained to independently perform standard ophthalmic examinations.
In this study, the spherical equivalent (SE) was calculated as the dioptric powers of the sphere and half of the cylinder (sphere+0.5 cylinders). Myopia was defined as SE of <0.5 diopter (D) and visual acuity <5.0. Astigmatism is the diopter difference between 2 main diameters of the same eye (absolute diopter value of the column mirror) above 0.50D.
In this study, each item of students' eye care behaviors in 2019 was parameterized by latent class analysis (LCA), and the latent class model (LCM) was constructed, which is a statistical analysis that addresses the relationship between types of latent variables. The optimal model is determined by the following criteria: Akaike information criterion (AIC), Bayesian information criterion (BIC), sample-size adjusted Bayesian information criterion (aBIC), Bootstrap likelihood ratio test (BLRT), and adjusted Lo-Mendell-Rubin likelihood ratio test (aLMR). After identifying the latent classes, the regression mixture modeling (RMM) was used to analyze the sociodemographic characteristics and visual health levels of different behavioral groups. SPSS 25.0 and Mplus 7.4 statistical software were used to analyze the data, and p < 0.05 was taken as the criterion of significance.
A total of 7,840 primary and secondary school students were recruited for this study, among which 1.710 were excluded (21.81%) due to transfer to other schools, incomplete questionnaires, and other reasons. Table 1 contains basic demographic characteristics. Among the participants, 6,130 were included in the final analysis, of which 3,283 (53.56%) were men and 2,847 (46.44%) were women. The sample population was 6–17 years old, with an average age of 10.33±2.60.Respondents aged 6–8 years accounted for the highest proportion (37.63%), and those aged 15–17 years accounted for the lowest proportion (10.73%). In terms of education, primary school students accounted for the highest proportion (56.51%), and the proportion of high school students was the lowest at 13.16%. The number of myopic students in the sample population was 3,067, accounting for 50.03%. The prevalence of myopia increased as age increased (Figure 1). There were statistically significant differences in the prevalence rate of myopia among students with different behaviors (Table 2).
Table 3 shows that the information criteria indices AIC, BIC, and aBIC decreased with the increase in the number of latent classes, and reached the maximum value in model 5. From the likelihood ratio test statistics, the entropy value reached 0.838 in model 3, indicating that the model was the most accurate for sample classification when there were 3 latent classes. Based on the model fitting evaluation results and conditional probability distribution of the latent class, the latent class of adolescent visual behavior was finally divided into 3 classes: class 1, class 2, and class 3.
Table 4 and Figure 2 show the conditional probability of the latent class of adolescent eye care behavior. In class 1, the item probability of frequent use of the eyes for more than 40 min (78.2%) was the highest, and the item probability of holding a pen, reading, and writing correctly (1.2%) was the lowest. In class 3, the item probability of regularly using electronic devices for more than 30 min (15.8%) was the lowest, but outdoor exercise time reached up to 2 h a day (93.8%), and eye exercises were performed every day (93.0%). Compared with the other 2 classes, the conditional probability of class 2 tends to be in the middle. Therefore, class 1 was named the “bad behaviors class,” class 2 was the “moderate behaviors class,” and class 3 was the “healthy behaviors class.”
Figure 2. Line chart of latent classes of adolescent eye care behavior. *Q1: Frequent close eye contact for more than 40 min; Q2: Hold a pen, read, and write correctly; Q3: Regularly use electronic devices for more than 30 min; Q4: Get enough sleep each day; Q5: Poor eating habits; Q6: Pay attention to supplement foods rich in vitamin A; Q7: Outdoor exercise time up to 2 h a day; Q8: Do eye exercises every day; Q9: Attend non-sports training courses regularly; Q10: Exercise or train eye muscles regularly. Q1, Q3, Q5 and Q9 were reverse scored.
Table 5 shows the influence of basic demographic characteristics and visual development on the distribution of latent classes of adolescent eye care behaviors.
Of the sociodemographic characteristics, the distribution of gender (p = 0.016), age (p < 0.001), education stage (p < 0.001), type of school (p < 0.001), and urban/rural region (p = 0.030) differed among the classes of students. In addition, the distributions of myopia (p = 0.009) and wearing glasses (p < 0.001) were also significantly different among the 3 classes of students.
The results of multicollinearity diagnosis showed that the tolerance between the respective variables was >0.1, and the variance inflation factor (VIF) was <10 (Table 6).The results of the parallel line test showed that X2 = 210.070, p < 0.05. These results showed that polynomial logistic regression can be used.
In the bad behaviors class (reference: health behavior class), in terms of visual acuity development status, those with astigmatism was 1.26 (p = 0.002) times more likely to be found in the bad behaviors class than in the healthy behaviors class, and those with glasses were 1.90 times more likely to be found in the bad behaviors class than the healthy behaviors class (p < 0.001). In addition, the probability of the high school group being distributed in the bad behaviors class was 2.44 (1/0.41, p = 0.003) times that of the primary school group, and the probability of a non-demonstration school population in the bad behaviors class was 1.27 times higher than that in the healthy behaviors class (1/0.79, p = 0.001).
In the moderate behaviors class (reference: health behavior class), those with myopia were 1.83 times more likely to be found in the moderate behaviors class than in the non-myopic group (p = 0.009).In terms of gender, females were 1.35 times more likely to be in the moderate behaviors class than males (1/0.74, p = 0.001).Compared with those aged 6–8 years old, those aged 15–17 years old were 5.26 (1/0.19, p = 0.005) times more likely to be found in the moderate behaviors class than the healthy behaviors class. Regarding the type of school, people from non-demonstration schools were 2.08 (1/0.48, p < 0.001) times more likely to be found in the moderate behaviors class than those from demonstration schools. These results are shown in Table 7.
First, there were 3 subgroups among adolescent eye care behaviors, such as the bad behaviors class, moderate behaviors class, and health behaviors class. Second, the results of regression mixed analysis showed that those from the lower grade group, the demonstration school, and those with good vision were more likely to be distributed in the healthy behavior group.
The latent classes of adolescent behavior vary according to the findings of different researchers. As a survey on adolescent health risks in China in 2020, the Health Risk Behavior Assessment Questionnaire (HRBAQ) was used to analyze the latent class of 22,628 middle school students in China, and found 4 latent classes, such as low-risk classes (64.0%), medium risk class 1 (4.5%), medium risk class 2 (28.8%), and high-risk class (2.7%) (32).Our results were similar. According to the results of the Australian student health behavior survey in 2019, 1,965 students in Australia were divided into 3 latent classes, namely unhealthy class (11.2%), moderate class (40.2%), and healthy class (48.6%), based on their diet, exercise, and sleep habits. This study explored the latent categories of adolescents from the perspective of eye care behavior, which enriches the existing literature on adolescent behavior (33).
In the 3 latent classes of adolescents in Wuhan, class 1 had the highest conditional probability in the items of “Frequent near work” and “Regularly use electronic devices.” Multiple studies have reported that near work and prolonged use of electronic devices significantly increase the risk of myopia (34–36). Therefore, class 1 was named the bad behaviors class because of its weak visual health management ability and high probability of bad behavior. Class 3 was named the healthy behaviors class because it had a higher conditional probability of the positive items. However, the healthy behaviors class was slightly higher than the moderate behaviors class in terms of the measurement items of “Regularly participating in non-sports excellent training courses,” which may indicate that on the one hand, adolescents in the healthy behaviors class attach more importance to the cultivation of healthy behavior and can consciously manage vision health. On the other hand, it also indicates that adolescents are still under great pressure from extracurricular tutoring. Although the adolescents in this class consciously carried out self-vision management, they still had some negative eye care behaviors due to academic pressure.
There were significant differences in basic demographic characteristics among the different classes. Compared with the healthy behaviors class, the bad behaviors class was more distributed in high school and non-demonstration schools, while the moderate behavior group was more distributed in female, 15–17 years old, and non-demonstration schools. These findings suggested that with the increase in age and academic pressure, adolescents in high school may have to reduce the time for outdoor exercise and sleep and increase the time for near work, resulting in the heterogeneity of eye care behavior. Moreover, the results also suggested that there were significant differences in visual acuity development between the classes, with the prevalence of myopia and the number of people wearing glasses being higher in the bad and moderate behaviors class than in the healthy behaviors class. This finding is consistent with previous research. In 2014, the survey results showed that the ametropia of students in Beijing was significantly related to lower level activities (37). In 2021, Dutch researchers surveyed 525 teenagers' smartphone use, which also showed that refractive errors were significantly correlated with behaviors (38). Orlansky's findings suggest that poor vision affects a wide range of areas, such as reading, writing, posture, and movement, which may increase the likelihood of bad behavior in adolescents with poor vision (39). By identifying the characteristics of different latent behavior classes, students can be guided in a targeted way to protect their visual health.
In summary, the results of the present study offer support for the notion that there is a diversity of eye care behaviors among adolescents. These subgroups also illustrate differential profiles in basic demographic characteristics and visual acuity development. In the future, a short and valid instrument may be developed accordingly to quickly screen and classify these subgroups. Eventually, we could expect an efficient and precise group intervention for students in different latent classes.
The raw data supporting the conclusions of this article will be made available by the authors, without undue reservation.
This study was reviewed and approved by the Ethics Committee of the School of Public Health, Wuhan University. Written informed consent was obtained from the minor(s)' legal guardian/next of kin for the publication of any potentially identifiable images or data included in this article.
ML and BZ are responsible for manuscript writing and WW is responsible for data analysis. XT reviewed the manuscript. All the authors made substantial contributions to the completion of this manuscript, final approval of the version to be published, and agreed to be accountable for all aspects of the work.
The authors declare that the research was conducted in the absence of any commercial or financial relationships that could be construed as a potential conflict of interest.
All claims expressed in this article are solely those of the authors and do not necessarily represent those of their affiliated organizations, or those of the publisher, the editors and the reviewers. Any product that may be evaluated in this article, or claim that may be made by its manufacturer, is not guaranteed or endorsed by the publisher.
1. Holden BA, Fricke TR, Wilson DA, Jong M, Naidoo KS, Sankaridurg P, et al. Global Prevalence of myopia and high myopia and temporal trends from 2000 through 2050. Ophthalmology. (2016) 123:1036–42. doi: 10.1016/j.ophtha.2016.01.006
2. Bourne RRA. Vision 2020: where are we? Curr Opin Ophthalmol. (2020) 31:81–4. doi: 10.1097/ICU.0000000000000647
3. Walline JJ, Lindsley KB, Vedula SS, Cotter SA, Mutti DO, Ng SM, et al. Interventions to slow the progression of myopia in children. Cochrane Database Syst Rev. (2020) 1:CD004916. doi: 10.1002/14651858.CD004916.pub4
4. Jin JX, Hua WJ, Jiang X, Wu XY, Yang J, et al. Effect of outdoor activity on myopia onset and progression in school-aged children in northeast China: the Sujiatun Eye Care Study. BMC Ophthalmol. (2015) 15:73. doi: 10.1186/s12886-015-0052-9
5. Wang J, Ying GS, Fu X, Zhang R, Meng J, Gu F, et al. Prevalence of myopia and vision impairment in school students in Eastern China. BMC ophthalmology. (2020) 20:2. doi: 10.1186/s12886-019-1281-0
6. Xiong F, Mao T, Liao H, Hu X, Shang L, Yu L, et al. Orthokeratology and low-intensity laser therapy for slowing the progression of myopia in children. BioMed Res Int. (2021) 2021:8915867. doi: 10.1155/2021/8915867
7. Liang Y, Kee CS. Risk Factors for Myopia in 2 Hong Kong School Systems: a pilot study. Asia-Pac J Ophthalmol. (2022) 11:19–26. doi: 10.1097/APO.0000000000000486
8. Dong L, Kang YK, Li Y, Wei WB, Jonas JB. Prevalence and Time Trends of Myopia in Children and Adolescents in China: A Systemic Review and Meta-Analysis. Retina. (2020) 40:399–411. doi: 10.1097/IAE.0000000000002590
9. Jan C, Li L, Keay L, Stafford RS, Congdon N, Morgan I. Prevention of myopia, China. Bull World Health Organ. (2020) 98:435–7. doi: 10.2471/BLT.19.240903
10. Chen J, He X, Wang J, Xie H, Du L, Yang J, et al. Forcasting the prevalence of myopia among students aged 6-18 years in China from 2021 to 2030. Zhonghua yan ke za Zhi. (2021) 57:261–7. doi: 10.3760/cma.j.cn112142-20201228-000851
11. Cicinelli MV, Marmamula S, Khanna RC. Comprehensive eye care-Issues, challenges, and way forward. Indian J Ophthalmol. (2019) 68:316–23. doi: 10.4103/ijo.IJO_17_19
12. Rao G, Athota SM, Rani PK, Khanna R, Rajshekar V. Integrated model of primary and secondary eye care for underserved rural areas: The L V Prasad Eye Institute experience. Indian J Ophthalmol. (2012) 60:396–400. doi: 10.4103/0301-4738.100533
13. Baird PN, Saw SM, Lanca C, Guggenheim JA, Smith EL III, Zhou X, et al. Myopia. Nat Rev Dis Primers. (2020) 6:1–20. doi: 10.1038/s41572-020-00231-4
14. Tedja MS, Haarman AE, Meester-Smoor MA, Kaprio J, Mackey DA, Guggenheim JA, et al. IMI–myopia genetics report. Invest Ophthalmol Vis Sci. (2019) 60:M89–M105. doi: 10.1167/iovs.18-25965
15. Cooper J, Tkatchenko AV. A review of current concepts of the etiology and treatment of myopia. Eye Contact Lens. (2018) 44:231. doi: 10.1097/ICL.0000000000000499
16. Fabian ID, Abdallah E, Abdullahi SU, Abdulqader RA, Boubacar SA, Ademola-Popoola DS, et al. Global retinoblastoma presentation and analysis by national income level. JAMA Oncol. (2020) 6:685–95. doi: 10.1001/jamaoncol.2019.6716
17. Williams R, Bakshi S, Ostrin EJ, Ostrin LA. Continuous objective assessment of near work. Sci Rep. (2019) 9:1–10. doi: 10.1038/s41598-019-43408-y
18. Bhandari KR, Ostrin LA. Validation of the Clouclip and utility in measuring viewing distance in adults. Ophthalmic Physiol Opt. (2020) 40:801–14. doi: 10.1111/opo.12735
19. Dirani M, Crowston JG, Wong TY. From reading books to increased smart device screen time. Br J Ophthalmol. (2019) 103:1–2. doi: 10.1136/bjophthalmol-2018-313295
20. Tsai TH, Liu YL, Ma IH, Su CC, Lin CW, Lin LLK, et al. Evolution of the prevalence of myopia among Taiwanese schoolchildren: a review of survey data from 1983 through 2017. Ophthalmology. (2021) 128:290–301. doi: 10.1016/j.ophtha.2020.07.017
21. Liu XN, Naduvilath TJ, Wang J, Xiong S, He X, Xu X, et al. Sleeping late is a risk factor for myopia development amongst school-aged children in China. Sci Rep. (2020) 10:1–11. doi: 10.1038/s41598-020-74348-7
22. Berticat C, Mamouni S, Ciais A, Villain M, Raymond M, Daien V. Probability of myopia in children with high refined carbohydrates consumption in France. BMC Ophthalmol. (2020) 20:1–8. doi: 10.1186/s12886-020-01602-x
23. Lim LS, Gazzard G, Low YL, Choo R, Tan DT, Tong L, et al. Dietary factors, myopia, and axial dimensions in children. Ophthalmology. (2010) 117:993–7. e4. doi: 10.1016/j.ophtha.2009.10.003
24. Jonas JB, Ang M, Cho P, Guggenheim JA, He MG, Jong M, et al. IMI prevention of myopia and its progression. Invest Ophthalmol Vis Sci. (2021) 62:6. doi: 10.1167/iovs.62.5.6
25. Xiong S, Sankaridurg P, Naduvilath T, Zang J, Zou H, Zhu J, et al. Time spent in outdoor activities in relation to myopia prevention and control: a meta-analysis and systematic review. Acta ophthalmol. (2017) 95:551–66. doi: 10.1111/aos.13403
26. Lingham G, Lucas RM, Milne E, Hewitt AW, Hammond CJ, MacGregor S, et al. Time spent outdoors in childhood is associated with reduced risk of myopia as an adult. Sci Rep. (2021) 11:1–11. doi: 10.1038/s41598-021-85825-y
27. Smith EL, Hung L-F, Arumugam B, Huang J. Negative lens–induced myopia in infant monkeys: effects of high ambient lighting. Invest Ophthalmol Vis Sci. (2013) 54:2959–69. doi: 10.1167/iovs.13-11713
28. Zhang LM, Lu Y, Gong L. Pterygium is related to short axial length. Cornea. (2020) 39:140. doi: 10.1097/ICO.0000000000002200
29. Sun HP, Lin Y, Pan CW. Iris color and associated pathological ocular complications: a review of epidemiologic studies. Int J Ophthalmol. (2014) 7:872. doi: 10.3980/j.issn.2222-3959.2014.05.25
30. Leo SW. Current approaches to myopia control. Curr Opin Ophthalmol. (2017) 28:267–75. doi: 10.1097/ICU.0000000000000367
31. Gao GP, Tan H, Zhang XJ. Development of the Assessment Questionnaire of Vision Care Related Behavior for Students(AQVCRBS) and its evaluation on reliability and validity. Chin J Scl Health. (2013) 34:1456–9. doi: 10.16835/j.cnki.1000-9817.2013.11.007
32. Song B, Hu W, Hu W, Yang R, Li D, Guo C, et al. Physical disorders are associated with health risk behaviors in chinese adolescents: a latent class analysis. Int J Environ Res Public Health. (2020) 17:2139. doi: 10.3390/ijerph17062139
33. Straker L, Smith A, Hands B, Olds T, Abbott R. Screen-based media use clusters are related to other activity behaviours and health indicators in adolescents. BMC Public Health. (2013) 13:1–12. doi: 10.1186/1471-2458-13-1174
34. Grzybowski A, Kanclerz P, Tsubota K, Lanca C, Saw SM. A review on the epidemiology of myopia in school children worldwide. BMC Ophthalmol. (2020) 20:1–11. doi: 10.1186/s12886-019-1220-0
35. Lin Z, Vasudevan B, Mao GY, Ciuffreda KJ, Jhanji V, Li XX, et al. The influence of near work on myopic refractive change in urban students in Beijing: a three-year follow-up report. Graefe Arch Clin Exp Ophthalmol. (2016) 254:2247–55. doi: 10.1007/s00417-016-3440-9
36. Huang PC, Hsiao YC, Tsai CY, Tsai DC, Chen CW, Hsu CC, et al. Protective behaviours of near work and time outdoors in myopia prevalence and progression in myopic children: a 2-year prospective population study. Br J Ophthalmol. (2020) 104:956–61. doi: 10.1136/bjophthalmol-2019-314101
37. Lin Z, Vasudevan B, Jhanji V, Mao GY, Gao TY, Wang FH, et al. Near work, outdoor activity, and their association with refractive error. Optom Vis Sci. (2014) 91:376–82. doi: 10.1097/OPX.0000000000000219
38. Enthoven CA, Polling JR, Verzijden T, Tideman JWL, Al-Jaffar N, Jansen PW, et al. Smartphone use associated with refractive error in teenagers: the myopia app study. Ophthalmology. (2021) 128:1681–8. doi: 10.1016/j.ophtha.2021.06.016
Keywords: adolescent, latent class analysis, visual health management, myopia, eye care
Citation: Li M, Wang W, Zhu B and Tan X (2022) A Latent Class Analysis of Student Eye Care Behavior: Evidence From a Sample of 6–17 Years Old in China. Front. Public Health 10:914592. doi: 10.3389/fpubh.2022.914592
Received: 07 April 2022; Accepted: 16 May 2022;
Published: 15 June 2022.
Edited by:
Carla Costa Lanca, Escola Superior de Tecnologia da Saúde de Lisboa (ESTeSL), PortugalReviewed by:
Maria Barata, Centro Hospitalar Universitário Lisboa Central (CHULC), PortugalCopyright © 2022 Li, Wang, Zhu and Tan. This is an open-access article distributed under the terms of the Creative Commons Attribution License (CC BY). The use, distribution or reproduction in other forums is permitted, provided the original author(s) and the copyright owner(s) are credited and that the original publication in this journal is cited, in accordance with accepted academic practice. No use, distribution or reproduction is permitted which does not comply with these terms.
*Correspondence: Xiaodong Tan, MDAzMDA0NjlAd2h1LmVkdS5jbg==
†These authors have contributed equally to this work and share first authorship
Disclaimer: All claims expressed in this article are solely those of the authors and do not necessarily represent those of their affiliated organizations, or those of the publisher, the editors and the reviewers. Any product that may be evaluated in this article or claim that may be made by its manufacturer is not guaranteed or endorsed by the publisher.
Research integrity at Frontiers
Learn more about the work of our research integrity team to safeguard the quality of each article we publish.