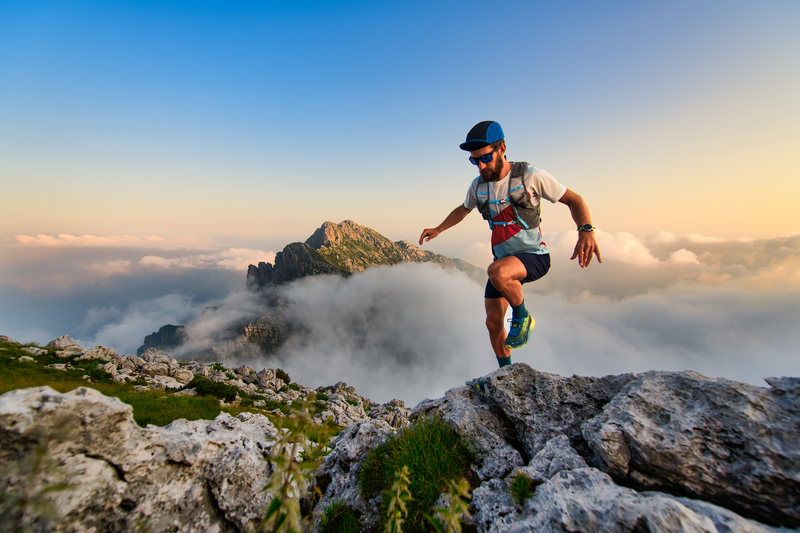
94% of researchers rate our articles as excellent or good
Learn more about the work of our research integrity team to safeguard the quality of each article we publish.
Find out more
ORIGINAL RESEARCH article
Front. Public Health , 22 June 2022
Sec. Public Health Policy
Volume 10 - 2022 | https://doi.org/10.3389/fpubh.2022.870210
This article is part of the Research Topic Realizing Universal Health Coverage in India View all 6 articles
Building good health systems is an important objective for policy makers in any country. Developing countries which are just starting out on their journeys need to do this by using their limited resources in the best way possible. The total health expenditure of a country exerts a significant influence on its health outcomes but, given the well-understood failures of price-based market-mechanisms, countries that spend the most money do not necessarily end-up building the best health systems. To help developing country policy makers gain a deeper insight into what factors matter, in this study the contribution of per-capita total, out-of-pocket, and pooled health expenditures, to the cross-country variation in Disability Adjusted Life Years lost per 100,000 population (DALY Rates), a summary measure of health outcomes, is estimated. The country-specific residuals from these analyses are then examined to understand the sources of the rest of the variation. The study finds that these measures are able to explain between 40 and 50% of the variation in the DALY Rates with percentage increases in per-capita out-of-pocket and pooled expenditures being associated with improvements in DALY Rates of about 0.06% and 0.095%, respectively. This suggests that while increases in per-capita total health expenditures do matter, moving them away from out-of-pocket to pooled has the potential to produce material improvements in DALY Rates, and that taken together these financial parameters are able to explain only about half the cross-country variation in DALY Rates. The analysis of the residuals from these regressions finds that while there may be a minimum level of per-capita total health expenditures (> $100) which needs to be crossed for a health system to perform (Bangladesh being a clear and sole exception), it is possible for countries to perform very well even at very low levels of these expenditures. Colombia, Thailand Honduras, Peru, Nicaragua, Jordan, Sri Lanka, and the Krygyz Republic, are examples of countries which have demonstrated this. It is also apparent from the analysis that while very high rates (> 75%) of pooling are essential to build truly high performing health systems (with DALYRates < 20, 000), a high level of pooling on its own is insufficient to deliver strong health outcomes, and also that even at lower levels of pooling it is possible for countries to out-perform their peers. This is apparent from the examples of Ecuador, Mexico, Honduras, Malaysia, Vietnam, Kyrgyz Republic, and Sri Lanka, which are all doing very well despite having OOP% in the region of 40–60%. The analysis of residuals also suggests that while pooling (in any form) is definitely beneficial, countries with single payer systems are perhaps more effective than those with multiple payers perhaps because, despite their best efforts, they have insufficient market power over customers and providers to adequately manage the pulls and pressures of market forces. It can also be seen that countries and regions such as Honduras, Peru, Nicaragua, Jordan, Sri Lanka, Bangladesh, Kerala, and the Kyrgyz Republic, despite their modest levels of per-capita total health expenditures have delivered attractive DALY Rates on account of their consistent prioritization of public-health interventions such as near 100% vaccine coverage levels and strong control of infectious diseases. Additionally, countries such as Turkey, Colombia, Costa Rica, Thailand, Peru, Nicaragua, and Jordan, have all delivered low DALY Rates despite modest levels of per-capita total health expenditures on account of their emphasis on primary care. While, as can be seen from the discussion, several valuable conclusions can be drawn from this kind of analysis, the evolution of health systems is a complex journey, driven by multiple local factors, and a multi-country cross-sectional study of the type attempted here runs the risk of glossing over them. The study attempts to address these limitations by being parsimonious and simple in its approach toward specifying its quantitative models, and validating its conclusions by looking deeper into country contexts.
Building good health systems in an efficient way is an important objective for policy makers in any country, but in particular for those in developing countries which are just starting out on their journeys, and need to use their limited resources in the best way possible. An important framework that is used to guide policy formulation is the Control Knobs Framework shown in Figure 1. As can be seen from the figure, the framework takes the view that there are multiple Control Knobs that can be dialled up or down by policy makers to reach their desired health systems goals. One of the key Control Knobs is that of financing which refers to, among other things, the total quantum of funds that are spent on healthcare in a county, and manner in which they are spent (1). These questions are often starting points for any analysis of health systems.
Figure 1. Control Knobs Framework (1).
The per-capita health expenditure of a country is determined by its per-capita income and by the proportion of that income that is allocated toward healthcare by its citizens and governments, and has a significant influence on health outcomes. However, given the well-understood failures of price-based market-mechanisms to build good health systems (2), countries that spend the most money do not necessarily end-up building the best health systems. Within the financing domain, one such set of added factors which are considered to be important (3, 4) relate to the extent to which the health expenditures in a country are pooled, and how much citizens are required to spend on an out-of-pocket basis, when they seek healthcare. That all of these factors matter is a widely accepted view, however, from a policy perspective it would be important get a more precise estimate of the extent of their importance. Additionally, once a careful determination has been made of the extent to which these two aspects of financing matter, it then becomes important to understand what role, if any, the other Control Knobs have in influencing the performance of health systems. The study therefore attempts to explore three broad questions:
1. To what extent are countries that have higher per-capita health expenditures able to generate better health outcomes?
2. To what extent is pooling of these expenditures important?
3. Are there factors, other than per-capita health expenditures and the extent of pooling, which have an impact on health outcomes?
To carry out these analyses, data are needed for health systems performance, total heath expenditures, out-of-pocket expenditures, and pooled expenditures, for countries and regions around the world. All of the data used in this study have been reported in Tables 11–15.
In this study Disability Adjusted Life Years lost (DALYs), as defined by the Institute for Health Metrics and Evaluation, (5), per 100,000 population, referred to as the DALY Rate, is used as the measure of health status (Figure 1).1 For countries data on DALY Rates have been obtained from the Institute for Health Metrics and Evaluation (7). In addition, given their sheer sizes, the wide variations between them in both inputs and outputs, and the constitutional authority and resources possessed by them to manage their own health systems, Indian states, for which data are available, are treated on par with independent countries, and India as a country is omitted from the data set. The DALY Rates for Indian states have been obtained from the statistical appendix to the Global Burden of Disease Study for Indian states (8, 9).
It is important to note that the DALY Rates used in this study are not age-standardized. This is because population-age distributions vary widely between countries, as exemplified by the variation in the proportion of 0–14 year olds across countries (10). These variations, in a manner similar to other environmental variables such as distance from the equator (11), have a material impact how much countries spend on their health systems, how they design them, as well as on the performance of these health systems. The question being asked in this paper is, given the reality in which countries find themselves, how well have they responded through their health systems. Age standardizing only the DALY Rates and not the inputs into the health system, would end-up systematically biasing the results in favor of (against) health systems of the more developed countries (less developed countries) which have a higher (lower) proportion of older individuals.
It is also important to bear in mind that the use of DALY Rate to characterize the overall performance of health systems, does not directly assess the performance of these systems on other factors listed in Figure 1, such as the degree of financial protection offered, equity of access, and degree of responsiveness. While, if poor performance of the health system on any of these factors is sufficiently large it is possible that it would be reflected in the DALY Rate, a proper study of what drives the performance of health systems on these factors would need an entirely different approach—a cross-sectional analysis of the type undertaken here may not be best suited to study the drivers of performance of health systems on these dimensions.
Data on per-capita total health expenditures (THE) for countries have been obtained from the data sets published by the World Bank (12). For the Indian states THE data have been obtained from the 2015-16 National Health Accounts estimates published by the National Health Systems Resource Centre (13). Sufficient data could not be obtained for a number of smaller countries, including American Samoa, Aruba, Bermuda, British Virgin Islands, Greenland, Guam, North Korea, Kosovo, Libya, and Hong Kong, and within India, for the state of West Bengal. All of these countries and regions have therefore been omitted from the analysis. The THE data have all been expressed in US Dollars, measured using Purchasing Power Parity (PPP) exchange rates (14). An exchange rate of Rs. 18.55 per US$ has been used in the study to convert state level rupee expenditures into PPP US Dollar numbers for the Indian states.
Using 2016 data, Figure 2 graphs the relationship between the DALY Rates and THE for all the countries (and Indian states) in the data-set. It can be seen from the graph that, while at lower levels of THE even small increases are associated with large reductions in the DALY Rate, the benefits that accrue from additional increases in THE appear to decline at an exponential rate.
The 2016 data on per-capita out-of-pocket expenditures (OOP) for countries have been obtained from the data sets published by the World Bank (15). For the Indian states the 2016 OOP data have been obtained from the 2015-16 National Health Accounts estimates published by the National Health Systems Resource Centre (13). Per-capita Pooled Expenditures (POOL) for each country (i), are computed simply as:
While population data are not used directly in any of the quantitative analyses in the study, they are included in the tables to provide an assessment of the overall size of the country. For countries population numbers have been obtained from data published by the World Bank (16). For Indian States the population numbers, given the recent separation of the state of Telangana from the state of Andhra Pradesh, the only source from which 2016 population data could be obtained was the website IndiaPopulation2019 (17).
The study attempts to quantify the impact of THE, OOP, and POOL on the DALY Rate using multi-country cross-sectional analysis. Multi-country cross-sectional analysis of this sort is fraught with a number of difficulties and has been famously compared by Joan Robinson to looking for a black cat in a dark room where no cat exists (18). There are also a number of continuing questions about the methodology behind estimating DALYs which limit the value of attempting to obtain more precise and detailed conclusions from further cross-country quantitative data-analysis (19–21). Despite these concerns, given the salience of these variables, there is value in examining the degree to which they have explanatory power, and what lessons they hold for policy makers. However, given these issues, once the analysis of the relationship between THE, OOP, POOL, and DALY Rates is complete, instead of attempting further quantitative analyses to explain the rest of the variation in DALY Rates, a comparison of the predicted values of health outcomes with actuals, is used in the study to identify positive and negative outlier countries. The unusual performance of these outliers is then explored further, to see if there are additional lessons to be learnt from them for wider application.
The exponential relationship between the DALY Rate and THE is apparent from Figure 2 and suggests that a simple Cobb-Douglas production function (22) as shown in Equation (2), could be used to explore the relationships between these variables for each country i, and to understand how much of the variation in the DALY Rates they are able to explain—the variable labeled DALY in the equations refers to the DALY Rate.
with γ being the elasticity of percentage change in the DALY Rate for every percentage change in THE and ln Qi being the portion not explainable by the changes in THE.
For estimation purposes this equation may be rewritten as:
where, ηi is the residual or the error term for each country i, which captures the unexplained part of the performance of each health system, with ln Q0 being the constant term (i.e., ln Qi = ln Q0 + ln ηi).
THE can be further divided into Pooled Expenditures (POOL) and Out-of-Pocket Expenditures (OOP) incurred by the consumers of healthcare services. Since POOL and OOP evolve independently in any health system, using these variables, Equation (2) may be rewritten as:
where, α and β are the elasticities associated, respectively, with POOL and OOP.
Just as has been done in Equation (4), the associated regression equation may be written as:
Having estimated these elasticities, the residual associated with each country i, is estimated as:
where, , , and are the estimated values from Equation (6).
These residuals (ϵi), reported in Tables 11–15, which represent the extent to which the health system is an outlier (i.e, has aspects of its performance that are not explainable by POOL and OOP) are then subjected to further examination to ascertain if they offer any lessons for developing country policy makers.
Using this data, Equation (4) is estimated using Ordinary Least Squares (OLS). The results of this regression are given in Table 1. These results suggest that with an elasticity γ = −0.1557 (p << 0.01%) applied to THE, it is possible to explain 49.43% of the variation in DALY Rates, leaving fairly large unexplained residuals. The large value of lnQ0 can perhaps be seen as the centrifugal force (23) pulling country health systems toward a baseline level of low-performance.2 The large value of lnQ0 implies that , a DALY Rate comparable to that of the Central African Republic (see Table 8). The value of γ = −0.1557 also indicates that countries and regions desirous of bringing down DALY Rates by 15.57% would need to double their THE and that a halving of the DALY Rate would need a tripling of total health expenditures. It is clear from the analysis that THE is an important driver of DALYs but increases of the magnitude required would necessarily have to follow the natural growth curve of the per-capita incomes in these economies which, in turn, would need them to allocate increased amounts in the development of their own Human Capital (25), money that they may not necessarily be able to find, at least in the near term. These limitations make it important to examine if, even within the current levels of THE there are other opportunities that developing country policy makers have, to generate a positive impact on DALY Rates.
As a next step, using 2016 data, when Equation (6) is estimated using Ordinary Least Squares (OLS) the results given in Table 2 are obtained.
These results suggest that with an elasticity of −0.0941 applied to POOL and −0.0584 applied to OOP, it is possible to explain 43.22% of the variation in the DALY Rates leaving, once again, fairly large unexplained residuals. It can be seen from the estimated values of α and β that, as expected, pooled expenditures have a far greater impact on reducing DALYs than do out-of-pocket expenditures. This differential in elasticities suggests a potentially additional pathway toward improving the performance of health systems, and one that is much more directly in the hands of the government than is increasing THE, i.e., increasing the quantum of pooled expenditures and reducing the amounts being spent on an out-of-pocket basis. Given the relative values of α and β, for a region (like the Indian state of Kerala, for example) where the OOP% ≈ 70%, a 10% reduction in the level of OOP would improve the DALY Rate by 0.584%. However, if that reduction in OOP is entirely reallocated to pooled expenditures, it would result in a 21% increase in POOL and consequently a 1.98% reduction in DALYs. This represents a net improvement of 1.4% in the DALY Rate without any increase in THE itself. These reallocations are hard to accomplish but are likely to be easier than pushing per-capita growth rates in the entire economy and THE to higher levels.
Driven by this insight [using OOP% data from (26)], and other considerations relating to financial protection and equity (Figure 1), it can be seen from Table 3 that many countries, have reduced the OOP% between the years 2016 and 2000, in order to improve the performance of their health systems.
Based on the elasticities arrived at in Table 2 residuals () are estimated for each country using Equation (7) and are listed in Tables 11–15 for all the countries and regions in the data set. Figure 3 graphs the value of these residuals against THE. It can be seen from the graph that at each and every level of THE there are both positive and negative outliers. It is important to note though that at lower levels of THE the range of over/under-performance is far greater than at higher level of THE. This suggests that there may be useful additional lessons to gained from a careful analysis of these residuals, particularly for low-income countries, which would result in a large improvement in their health outcomes.
In order to aid in the analysis of these residuals, the health systems of countries are classified in this paper into different categories based on the criteria listed below (determined by the author based on where the natural breakpoints in health outcomes appeared to exist):
1. Extent of Pooling: Pooled Dominant if OOP < 25%; Market Significant if 25% < OOP < 50%; and Market Dominant if OOP> 50%.
2. Health System Performance: High Performance if DALY < 25, 000; Medium Performance if 25, 000 < DALY < 30, 000; and Low Performance if DALY> 30, 000.
3. Extent of Outperformance: Positive Outlier if Residual < −5, 000; Negative Outlier if Residual > 5000; and Neutral if −5000 < Residual < 5000.
4. Size of Country: Small if Population < 1 million; Large if Population > 1 million.
Using these and other categorizations, the interest is in studying countries and regions that operate in different environments, to understand what additional steps larger countries with high and medium performance health systems have taken to become Positive Outliers and what challenges are being experienced by the Negative Outliers. It is clear from the Tables 4–10 that outlier countries can be drawn from any mix of pooled and out-of-pocket expenditures and THE levels.
Table 4 lists large, high and medium performance countries which are Pooled Dominant (i.e., OOP < 25%). While each of these countries is worthy of careful study, of most immediate relevance in a developing country context would perhaps be Colombia (shaded in gray) which has been able to generate a DALY Rate of 21,613 against an expected rate, given its low THE level of $830, of 30,766, giving a high residual of -9,153. Amongst these high performing outliers, with a residual of −5,306 at a THE of only $635, while Thailand's achievements are indeed notable, its DALY Rate, at 27,412, still remains considerably above 20,000, suggesting that it still has long way to go before it can catch-up with the truly high performing countries list in the Table 4.
Table 5 lists large, high and medium performance countries which are Market Significant (i.e., 25% < OOP < 50%). Of particular interest in this list are Honduras, Peru, Nicaragua, and Jordan. Amongst these countries, Peru, Nicaragua, and Jordan (all shaded in gray), have DALY Rates close to or below 20,000 despite low THE levels and OOP > 25%. Honduras (shaded in gray) has a THE of only $400 and an OOP of 45% but has nevertheless been able to deliver a DALY Rate of <25,000.
Table 6 lists large, high and medium performance countries which are Market Dominant (i.e., OOP> 50%). All the countries / regions in this list are interesting, but in particular Sri Lanka and the Kyrgyz Republic (both shaded in gray). Sri Lanka is the only country with an OOP level that exceeds 50% that has been able to reduce its DALY Rate to <25,000. The Kyrgyz Republic spends only $240, with close to 60% of it being out-of-pocket, both numbers very similar to those of the Indian State of Tamil Nadu (Table 10), but, at 26,864, has been able to deliver a DALY Rate of close to 25,000, while, at 33,527, Tamil Nadu is well above 30,000.
In Table 7 are countries that have high THE but all are doing more poorly that would have been expected, given their high THE > $1,000 and relatively low OOP levels. In this list while the presence of United States (shaded in gray), with its extraordinarily high THE = $9,870 is not entirely surprising, the fact that Germany (shaded in gray) should have a DALY Rate 30,000 when, given its THE = $5,463 and OOP = 12%, it was expected to have a DALY Rate of <25,000 with a very high residual of 7,283, does invite special attention. France, a very similar country, by contrast (Table 9) with a lower THE = $4,782 and OOP = 10% has a much lower DALY Rate of 25,328.
Table 8 lists countries with THE < $100. All of them, with the exception of Bangladesh (shaded in gray) and Ethiopia, have a DALY Rate that exceeds 40,000. This is not in and of itself surprising but what is noteworthy is that, with the exception of Bangladesh, Ethiopia, and Gambia, they are all under-performing even relative to the low levels expected of them given their low THE and high OOP% levels.
In Table 9, all of the countries with THE > $2,500 are listed. As a group it is clear from the table that they are perhaps either not getting the value from all their expenditures or there are factors within their economies, such as a rapidly aging population, that needs them to spend much more than they are currently doing to get better DALY Rates. However, in this group, France, Australia, Singapore, Spain, Saudi Arabia, Israel, South Korea, and United Arab Emirates (all shaded in gray) stand-out because either they are staying close to what is expected of them or doing much better, despite having problems comparable to those of other developed countries. France and Spain stand out as the only large European countries in the list, and South Korea as being perhaps one which is getting the most value for the relatively low THE = $2, 712 that it is spending. The countries of the middle-east, as a group, appear to be outperforming the other developed nations.
It is interesting to note from Table 10 that the Indian states as a group are broadly doing as well as can be expected, but given their very low THE levels (Neutral Performance with −5, 000 < Residual < 5, 000) and very high OOP% levels they have DALY Rates well above 30,000. The only two exceptions being the states of Kerala and Himachal Pradesh (both shaded in gray along with India). Kerala in particular is note worthy because while it has a low THE = $386, and a verylarge OOP% = 71%, it nevertheless appears to have been able to harness market forces to deliver a globally respectable DALY Rate of 27,301.
Policy makers in developing countries have to work within severe resource constraints and need to deploy them with care in order to achieve their multiple policy goals. Improving the performance of their health systems is an urgent imperative for them and any systematic insights that they can gather from the experiences of other countries, both high and low performing, are likely to be of great value. This study analyses the performance of health systems around the world to understand more precisely the respective roles of total health expenditures, pooling of these expenditures, and multiple other factors, in shaping the behavior of health systems.
It is already well-known from the literature that total health expenditures and the extent of pooling matters (3, 27) for developing country health systems. From this study we learn additionally that while total expenditure on health does indeed matter, beyond a minimum level, it is neither necessary nor sufficient and can perhaps be excessive as well. From Table 1, it can be seen that there is a robust estimate of elasticity (γ) of −0.1557 associated with THE. This can also be seen from Figure 2 which indicates that there is a clear negative association between THE and the DALY Rate. However, from both Table 1 and Figure 2, it can clearly be seen that there are many countries with both high and low THE levels that are doing much worse and much better than others in their cohort. Tables 4–10 also bear this out and suggest that, while there may be a minimum level of THE > $100 which may need to be crossed for a health system to perform (Bangladesh being a clear and sole exception), it is possible for both countries and states with low levels of THE to perform very well (such as Colombia, Thailand Honduras, Peru, Nicaragua, Jordan, Sri Lanka, and the Krygyz Republic), and for those with high levels of THE to under-perform (such as United States and Germany).
Tables 4–6 reaffirm the insight that the extent of pooling matters, but go on to make the point that very high rates (> 75%) of pooling are essential to building truly high performing health systems (with DALYRates < 20, 000). It is also apparent from the tables that merely having a high level of pooling on its own is insufficient to deliver strong health outcomes, and also that even at lower levels of pooling it is possible to out-perform one's peers using other Control Knobs (Figure 1). From Table 2 it can be seen that the elasticity associated with pooled expenditures (α = −0.0941) is almost double that of the one associated with out-of-pocket expenditures (β = −0.0584), with both having p-values that are well below the 1% level. So clearly the more the level of pooling, on average, the better the outcomes but from Figure 3 it can be seen that there are a number of countries that are doing much better than their level of pooling would imply. This can be seen more clearly from Tables 5–7 where, while many countries with high levels of pooling (and high levels of THE) are doing poorly, others such as the Ecuador, Mexico, Honduras, Malaysia, Vietnam, Kyrgyz Republic, and Sri Lanka are doing very well despite having out-of-pocket expenditures in the region of 40–60%. While a measure of pooling (in any form) is beneficial, the manner in which pooling and associated purchasing arrangements are setup does matter a great deal to get high performance. A review of the performance of the countries listed in Tables 4–9, such as Colombia, Costa Rica, Israel, Thailand, United States, Germany, France, Australia, and Spain suggests that while pooling (in any form) is definitely beneficial, countries with single payer systems are perhaps more effective than those with multiple payers perhaps because, despite their best efforts, the multi-payer countries have insufficient market power over customers and providers to adequately manage pulls and pressures of market forces. This hypothesis is also consistent with the arguments made in (28) regarding inflation rates associated with different health system arrangements.
From the list of Positive Outliers, it can also be gathered that, consistent with existing insights (29), an emphasis on strong provision of essential public health services by the government can result in low DALY Rates even at low THE. Countries and regions such as Honduras, Peru, Nicaragua, Jordan, Sri Lanka, Bangladesh, Kerala, and the Kyrgyz Republic (Tables 5, 6) despite modest levels of THE have delivered attractive DALY Rates on account of their consistent prioritization of public-health interventions such as near 100% vaccine coverage levels and strong control of infectious diseases. From the examples of Turkey, Colombia, Costa Rica, Thailand, Peru, Nicaragua, and Jordan in Tables 4, 5, which have all delivered low DALY Rates despite modest levels of THE, it can also be seen that an emphasis on primary care, another well known insight (30, 31), can result in low DALY Rates even at low THE.
While, as can be seen from the discussion, several valuable conclusions can be drawn from this kind of analysis, the evolution of health systems is a complex journey, driven by multiple local factors, and a multi-country cross-sectional study of the type attempted here runs the risk of glossing over them (18). There are also multiple concerns about the methodologies associated with the computation of DALYs (19–21). The study attempts to address these limitations by being parsimonious and simple in its approach toward specifying its quantitative models and validating its conclusions by looking deeper into country contexts. However, another, related, limitation of the study is that while it has indeed made an attempt to examine the experiences of outlier countries, it has not done so at the level of depth that would be needed—additional research to address this shortcoming could yield powerful insights. Policy makers and researchers interested in these insights would do well to keep these limitations of the study in mind while reviewing the conclusions presented here (Tables 11–15).
Table 15. Estimated residuals for each country (Trinidad and Tobago to Zimbabwe; India and Indian States).
There is a generally accepted view that higher levels of total health expenditure (THE) in a country lead to better health outcomes, particularly if spent using pooled instead of out-of-pocket expenditures. In this paper, using DALY Rates as an outcome indicator, the effects of THE, and pooled (POOL) and out-of-pocket expenditures (OOP) are examined using simple Cobb-Douglas health-production functions. Consistent with the accepted view, this analysis indicates that for every 1% increase in THE, DALY Rates fall by 0.15% and that a 1% increase in pooled expenditures reduces DALY Rates by 0.095% while a similar increase in out-of-pocket expenditure, at 0.06%, leads to a much lower quantum of reduction in DALY Rates.
However, the analysis also indicates that these variables are able to explain less than 50% of the variation in DALY Rates, leaving fairly large unexplained residuals. An analysis of these residuals suggests several interesting insights, which bear further scrutiny. The analysis, for example, clearly indicates that developing countries which are able to spend in excess of ≈$100 per-capita can aspire to good health outcomes for their citizens and do not necessarily need to wait for several decades for national per-capita income to grow to a level that allows them to considerably increase their aggregate spending on health as a country. However, there are several other steps that they would need to take to produce good health from their current levels of health expenditures. These include making an effort to increase the level of pooling to > 75%, and moving in a direction such that a single payer is responsible for purchasing healthcare with these pooled resources. Additionally, all countries, including those with low levels of total health expenditure, need to ensure that their governments first properly complete the task of providing public-health public/merit-goods such as vaccinations and infectious disease control if they wish to have good health outcomes. And, whether using pooled funds or out-of-pocket expenditures, they need to be aware that a strong emphasis on comprehensive primary care can result in low DALY Rates even at low levels of total health expenditures.
Good health outcomes are obtainable both with private provision and through the use of government-owned providers. However, even with high levels of total health expenditures combined with high levels of pooling, unless carefully designed purchasing arrangements are put in place, it is possible to deliver relatively poor health outcomes, and, conversely even a small amount of pooled resources, if spent in a catalytic manner, can help deliver strong outcomes in the overall health system by ensuring that citizens get better value for their out-of-pocket expenditures.
The original contributions presented in the study are included in the article/supplementary material, further inquiries can be directed to the corresponding author/s.
The author confirms being the sole contributor of this work and has approved it for publication.
The author declares that the research was conducted in the absence of any commercial or financial relationships that could be construed as a potential conflict of interest.
All claims expressed in this article are solely those of the authors and do not necessarily represent those of their affiliated organizations, or those of the publisher, the editors and the reviewers. Any product that may be evaluated in this article, or claim that may be made by its manufacturer, is not guaranteed or endorsed by the publisher.
1. ^See (6) for a more detailed discussion on the computation of DALYs.
2. ^These forces are similar to those found in physical systems and are referred to as the ones increasing the total entropy (23) of the system. This is an apt analogy for health systems where the laissez-faire approach toward the functioning of the system found in many developing country situations, with the government almost exclusively focused only on the providers and facilities it manages and owns, has led market forces to having free rein and producing highly distorted outcomes (24).
1. Roberts MJ, Hsiao W, Berman P, Reich MR. Getting Health Reform Right: A Guide to Improving Performance and Equity. Oxford; New York, NY: Oxford University Press (2004).
3. Kutzin J. Health financing for universal coverage and health system performance: concepts and implications for policy. Bull World Health Organ. (2013) 91:602–11. doi: 10.2471/BLT.12.113985
5. IHME. What Is a DALY? (2019). Available online at: http://www.healthdata.org/gbd/faq#WhatisaDALY?
6. Devleesschauwer B, Havelaar AH, de Noordhout CM, Haagsma JA, Praet N, Dorny P, et al. Calculating disability-adjusted life years to quantify burden of disease. International Journal of Public Health. (2014) 59:565–9. doi: 10.1007/s00038-014-0552-z
7. IHME. Global Burden of Disease Study 2017 (GBD 2017) Results. (2018). Available online at: http://ghdx.healthdata.org/gbd-results-tool
8. Dandona L, Dandona R, Kumar GA, Shukla DK, Paul VK, Balakrishnan K, et al. Nations within a nation: variations in epidemiological transition across the states of India, 1990-2016 in the Global Burden of Disease Study. Lancet. (2017) 390:2437–60. doi: 10.1016/S0140-6736(17)32804-0
9. Dandona L, Dandona R, Kumar GA, Shukla DK, Paul VK, Balakrishnan K, et al. Statistical Appendix to Nations within a nation: variations in epidemiological transition across the states of India, 1990-2016 in the Global Burden of Disease Study. The Lancet. (2017) 390:2437–60.
10. World Bank. Population Ages 0-14 (% of Total Population). (2019). Available online at: https://data.worldbank.org/indicator/SP.POP.0014.TO.ZS
11. Acemoglu D, Johnson S, Robinson JA. The colonial origins of comparative development: an empirical investigation. Am Econ Rev. (2001) 91:1369–401. doi: 10.1257/aer.91.5.1369
12. World Bank. Current Health Expenditure Per Capita, PPP (Current International $) (2019). Available online at: https://data.worldbank.org/indicator/SH.XPD.CHEX.PP.CD
13. NHSRC. National Health Accounts Estimates for India (2015-16). New Delhi, Ministry of Health and Family Welfare, Government of India (2018). Available online at: https://nhsrcindia.org/sites/default/files/2021-06/NHAEstimatesReport-2015-16.pdf
14. IMF. Implied PPP Conversion Rate. Washington, DC: IMF (2019). Available online at: https://www.imf.org/external/datamapper/PPPEX@WEO/OEMDC/ADVEC/WEOWORLD
15. World Bank. Out-of-Pocket Expenditure Per Capita, PPP (Current International $) (2019). Available online at: https://data.worldbank.org/indicator/SH.XPD.OOPC.PP.CD
16. World Bank. Total Population. (2019). Available online at: https://data.worldbank.org/indicator/sp.pop.totl
17. IndiaPopulation. Population of India 2019. (2019). Available online at: https://indiapopulation2019.com/
18. Maddala GS. On the use of panel data methods with cross-country data. Ann Econ Stat. (1999) 55–56. doi: 10.2307/20076206
19. McQueston K. The Known Unknown: Estimating the Global Burden of Disease. (2014). Available online at: https://www.cgdev.org/blog/known-unknown-estimating-global-burden-disease
20. Kariuki JK, Stuart-Shor EM, Leveille SG, Hayman LL. Methodological challenges in estimating trends and burden of cardiovascular disease in Sub-Saharan Africa. Cardiol Res Pract. (2015) 2015:1–6. doi: 10.1155/2015/921021
21. McLain AC, Frongillo EA, Hess SY, Piwoz EG. Comparison of methods used to estimate the global burden of disease related to undernutrition and suboptimal breastfeeding. Adv Nutr. (2019) 10:380–90. doi: 10.1093/advances/nmy094
24. Mor N, Dhar D, Venkateswaran S. Healthcare in India: a fork in the road. In: Mohan R, editor. India Transformed : 25 Years of Economic Reforms. Gurgaon: Penguin Random House (2017). p. 384–403.
25. Mor N. Exploring the Role of Human Capital in Economic Growth using a Systems Dynamics Approach. (2019).
26. World Bank. Out-of-Pocket Expenditure (% of Current Health Expenditure). (2019). Available online at: https://data.worldbank.org/indicator/SH.XPD.OOPC.CH.ZS
27. Cashin C. Health Financing Policy: The Macroeconomic, Fiscal, and Public Finance Context. World Bank, Washington, DC (2016).
28. Hsiao WC. Why is a systemic view of health financing necessary? Health Affairs. (2007) 26:950–61. doi: 10.1377/hlthaff.26.4.950
29. Filmer D, Hammer JS, Pritchett LH. Weak links in the chain II: a prescription for health policy in poor countries. World Bank Res Observer. (2002). 17:47–66. doi: 10.1093/wbro/17.1.47
30. Starfield B, Shi L, Macinko J. Contribution of primary care to health systems and health. Milbank Q. (2005) 83:457–502. doi: 10.1111/j.1468-0009.2005.00409.x
Keywords: out-of-pocket expenditures (OOPE), Disability Adjusted Life Year (DALY), financial protection, universal health care (UHC), total health expenditure
Citation: Mor, N (2022) Lessons for Developing Countries From Outlier Country Health Systems. Front. Public Health 10:870210. doi: 10.3389/fpubh.2022.870210
Received: 06 February 2022; Accepted: 30 May 2022;
Published: 22 June 2022.
Edited by:
Simon Grima, University of Malta, MaltaReviewed by:
Aviad Tur-Sinai, Max Stern Academic College of Emek Yezreel, IsraelCopyright © 2022 Mor. This is an open-access article distributed under the terms of the Creative Commons Attribution License (CC BY). The use, distribution or reproduction in other forums is permitted, provided the original author(s) and the copyright owner(s) are credited and that the original publication in this journal is cited, in accordance with accepted academic practice. No use, distribution or reproduction is permitted which does not comply with these terms.
*Correspondence: Nachiket Mor, bmFjaGlrZXRAbmFjaGlrZXRtb3IubmV0
Disclaimer: All claims expressed in this article are solely those of the authors and do not necessarily represent those of their affiliated organizations, or those of the publisher, the editors and the reviewers. Any product that may be evaluated in this article or claim that may be made by its manufacturer is not guaranteed or endorsed by the publisher.
Research integrity at Frontiers
Learn more about the work of our research integrity team to safeguard the quality of each article we publish.