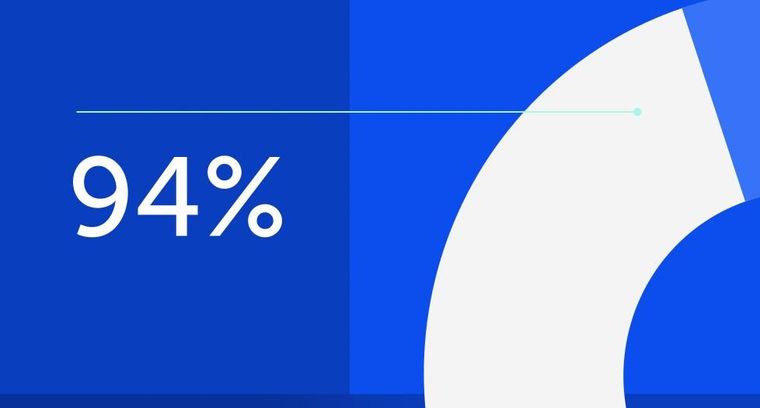
94% of researchers rate our articles as excellent or good
Learn more about the work of our research integrity team to safeguard the quality of each article we publish.
Find out more
ORIGINAL RESEARCH article
Front. Public Health, 07 April 2022
Sec. Children and Health
Volume 10 - 2022 | https://doi.org/10.3389/fpubh.2022.829840
Background: Wilms tumor (WT) is the most common tumor in children. We aim to construct a nomogram to predict the cancer-specific survival (CSS) of WT in children and externally validate in China.
Methods: We downloaded the clinicopathological data of children with WT from 2004 to 2018 in the SEER database. At the same time, we used the clinicopathological data collected previously for all children with WT between 2013 and 2018 at Children's Hospital of Chongqing Medical University (Chongqing, China). We analyzed the difference in survival between the patients in the SEER database and our hospital. Cox regression analysis was used to screen for significant risk factors. Based on these factors, a nomogram was constructed to predict the CSS of children with WT. Calibration curve, concordance index (C-index), the area under the receiver operating curve (AUC) and decision curve analysis (DCA) was used to evaluate the accuracy and reliability of the model.
Results: We included 1,045 children with WT in the SEER database. At the same time, we collected 112 children with WT in our hospital. The Kaplan-Meier curve suggested that children in China with WT had a higher mortality rate than those in the United States. Cox regression analysis revealed that age, lymph node density (LND), and tumor stage were significant prognostic factors for the patients in the SEER database. However, the patients in our hospital only confirmed that the tumor stage and the number of positive regional lymph nodes were significant factors. The prediction model established by the SEER database had been validated internally and externally to prove that it had good accuracy and reliability.
Conclusion: We have constructed a survival prognosis prediction model for children with WT, which has been validated internally and externally to prove accuracy and reliability.
Wilms tumor (WT) is derived from embryonic kidney tissue and is the most common kidney tumor in children, accounting for about 90% of kidney tumors (1). At present, in high-income countries, with modern multidisciplinary comprehensive treatment, the survival rate of children with WT can reach more than 90% (2–4). The International Society of Pediatric Oncology Renal Tumor Study Group (SIOP-RTSG) and Children's Oncology Group Renal Tumor Committee (COG-RTC) of the United States and Canada have been committed to improving the survival rate of tumors in children (5, 6). The difference is that COG-RTC advocates surgical resection first, while SIOP-RTSG advocates chemotherapy first. However, in underdeveloped countries, the late diagnosis of tumors in children and the higher recurrence rate are important factors for high child mortality (7). In China, the incidence of WT in children is about 330 per million (8). However, the incidence of WT in children in the United States is 7-10 per million (9). Therefore, the morbidity and mortality of WT in children in China are particularly cruel.
A previous study had developed and validated a nomogram for predicting the CSS of patients with WT, showing that age, tumor size, and the number of lymph nodes (LNs) examined were essential factors for the prognosis (10). In addition, some studies have also proved that age, tumor size, and tumor stage were factors influencing the forecast of WT (11, 12). Lymph node density (LND) has been proven to have significant prognostic value in colon cancer and rectal cancer (13). A study showed that the overall survival rate of children with WT was closely related to LND (14).
AI is already widely used in health care. Awais et al. (15) used texture analysis to classify the abnormal areas caused by oral cancer. Ghayvat et al. (16) apply blockchain to the management of medical big data. Mishra et al. (17) applied intelligence drive to multilevel assessment of psychological disorders. The nomogram is a numerical estimation of various clinicopathological factors to predict the occurrence of an outcome event. At present, the nomogram has been widely used to indicate the survival and prognosis of multiple cancers (18, 19). Although Tang et al. (20) have constructed a nomogram for CSS prediction in patients with WT, there is no study have been conducted for external validation.
Our purpose is to compare children's mortality and prognostic factors with WT between the United States and China. Then, the cases in the SEER database were used to construct a predictive prediction model for children with WT, and the patients from a children's hospital in China were used for external validation.
We collected clinicopathological data of all children under 18 with WT from the Surveillance, Epidemiology, and End Results (SEER) database. The SEER database is a cancer database in the United States, including 18 cancer registries and covering 28% of the US population (21). At the same time, we collected the clinicopathological information of all children with WT in Children's Hospital of Chongqing Medical University(CHCMU, Chongqing, China) from 2013 to 2018. The Ethics Committee approved our study of Children's Hospital of Chongqing Medical University, and the patient's guardian provided written informed consent.
Patients' clinicopathological data include age, sex, laterality, tumor stage, tumor size, number of lymph nodes (LNs) examined, number of positive lymph nodes, LND, surgery, and chemotherapy. The selection criteria are (1) Age ≤18 years; (2) International Classification of Oncological Diseases (ICD-O-3), code 8960; (3) Unilateral renal cancer. The exclusion criteria are (1) incomplete survival data; (2) the tumor size was unknown; (3) LNs examined and positive LNs were unknown; (4) Bilateral tumors; (5) the survival time was <1 month; (6) Unknown SEER stage. The patient screening process in the SEER database is shown in Figure 1.
LND is the number of positive regional LNs divided by the number of regional LNs examined. Age, tumor size, number of positive LNs, number of LNs examined, LND were continuous variables, expressed in median and inter-quartile ranges. The SEER stage was divided into three stages: local, regional and distant.
We followed up the children with WT in our hospital through outpatient clinic, hospitalization, telephone and letter. The start date of the follow-up was the first day after surgery and ended on March 1, 2019. The follow-up index was cancer-specific survival time, and the outcome event was death.
We randomly divided the 1045 children collected in the SEER database into a training set of 70% (n = 742) and a validation set of 30% (n = 303). Univariate and multivariate Cox proportional hazard risk models were used to evaluate independent risk factors for children with WT, and the hazard ratio (H.R.) and 95% confidence interval (CI) were recorded simultaneously.
The independent risk factors identified by cox regression analysis were used to construct a nomogram. When constructing the multivariate Cox regression, we obtained the regression coefficient β (coef) for each variable; Nomogram would normalize the regression coefficients and display them as risk scores on a number line.
The concordance index (C-index), the area under the receiver operating characteristics curve (AUC), and calibration curve were used to evaluate the accuracy and discrimination ability of the nomogram. Decision curve analysis (DCA) is a method for assessing the value of a model based on the net benefit under the estimated risk threshold (22). We used DCA to evaluate the clinical application value of the nomogram.
C-index, AUC and DCA were used for external validation to identify the accuracy and discrimination ability of the nomogram. In addition, we divided all patients into high-risk and low-risk groups according to their risk values on the nomogram. We used the Kaplan-Meier curve and log-rank test to test the difference in survival between the two groups.
For children in our hospital, the Kaplan-Meier method was used to estimate CSS. CSS was defined as the death of patients due to cancer, including metastasis, recurrence, or any related factors. Continuous variables were represented by median and inter-quartile range, and many-Whitney U-test was used to compare differences between groups. The comparison of categorical variables used the Pearson X2 test. All statistical analyses used R software (version 3.4.1; http://www.Rproject.org) and SPSS 24.0 (IBM Corp, Armonk, New York, USA). A P-value < 0.05 was considered statistically significant.
We included a total of 1,045 children with WT from the SEER database. The clinicopathological characteristics of the training set and the validation set are shown in Table 1. The median age of the patient was 3 years old (quartile, 1–5 years old). The median size of the children's tumor was 110 mm (quartile, 83–135 mm). The median number of regional LNs examined in the patient was 5 (quartile, 3 to 9). Among them, there were fewer males (47.46%), most patients had undergone chemotherapy (91.39%), lymph node-positive patients accounted for only 19.6%, and SEER stage was as follows: local accounted for 41.63%, regional accounted for 36.27%, and distant accounted for 22.11%. There was no significant difference between the patients in the training set and the validation set.
We collected a total of 112 children with WT in our hospital. The median age of all children was 3 years old(quartile, 1–5 years old), and the median follow-up time was 22 months (quartile, 12 to 42.5 months). Among them, most patients had undergone chemotherapy (99.1%), the number of positive LNs accounted for 18.8%, and the median number of LNs examined was 2 (quartile, 0–5). The SEER stage of patients was as follows: the localized accounted for 60.71%, the region accounts for 20.54%, and the foreign accounts for 18.75%. The clinicopathological characteristics of the SEER cohort and the CHCMU cohort are shown in Table 2.
We performed univariate and multivariate Cox regression analysis on patients in the SEER database and revealed age (HR 1.119, 95%CI: 1.039–1.206), LND (HR 4.465, 95%CI: 1.99–10.017), and stage (HR 1.673, 95%CI: 0.661–4.239; HR 3.603, 95%CI: 1.455–8.923) were independent risk factors for the prognosis of children with WT (Table 3). However, we performed univariate and multivariate Cox regression analysis on the patients collected in our hospital, suggesting that positive LNs (HR 2.958, 95%CI: 1.803–4.851), stage (HR 1.731, 95%CI: 0.359–8.345; HR 15.151, 95%CI: 4.744–48.39;) were independent risk factors for prognosis of children with WT (Table 4).
We used the independent risk factors identified by patients in the SEER database to construct a nomogram (Figure 2). The nomogram indicated that age and LND were the most significant risk factors affecting patient survival, followed by stage. The calibration curves of the training set and the validation set indicated that the predicted value was consistent with the observed value, which suggested that the nomogram has good accuracy (Figures 3A–F). The C-index of the training set and the validation set were 0.811 (95% CI, 0.738–0.884) and 0.937 (95% CI, 0.884–0.990), respectively. The C-index of the external validation set was 0.902 (95% CI, 0.837–0.967). The AUC of the training set, validation set (Figures 4A,B) and external validation (Figure 5) set showed similar results, suggesting that the nomogram has good accuracy and discrimination. The calibration curves of the training set and the validation set indicated that the predicted value was consistent with the observed value, which suggested that the nomogram has good accuracy.
Figure 3. Calibration plots of a nomogram for 3-, 5-, and 8-year CSS in training cohort (A–C) and validation cohort (D–F).
The DCA of the training set and the validation set indicated that the nomogram had an excellent clinical value (Figures 6A,B), and the DCA of the external validation set also proved the potential clinical value of the model (Figure 7). According to the risk value in the nomogram, we divided the patients into low-risk (≤60.2) and high-risk (>60.2) groups. The Kaplan-Meier curve and log-rank test showed that the high-risk group had a lower survival rate in the training and validation sets (Figures 8A,B). Similarly, the overall cohort and external validation set suggested that the high-risk group had a lower survival rate (Figures 9A,B). It proved that the nomogram had good discrimination. In addition, the Kaplan-Meier curve suggested that the survival rate of children in CHCMU was significantly lower than that of children in the SEER database (Figure 10).
Figure 6. Decision curves of the nomogram predicting CSS in the training cohort (A) and the validation cohort (B). The x-axis is the threshold probability, and the y-axis is the net income. The green line indicates that no patients have died, and the dark green line indicates that all patients have been killed. When the threshold probability is between 5% and 40%, the net benefit of the model exceeds all deaths or no deaths.
Figure 8. Kaplan–Meier curves of CSS for children in the low- and high-risk groups in the training set (A) and validation set (B).
Figure 9. Kaplan–Meier curves of CSS for children in the low- and high-risk groups in SEER (A) and CHCMU cohort (B).
For childhood kidney tumors, COG-RTC and SIOP-RTSG are the two most authoritative academic organizations for clinical research. Although the two adopted different treatment strategies for children with WT (surgery first vs. chemotherapy first), the therapeutic effects obtained were very close, and the long-term survival rate of patients was over 90% (23, 24). However, the total treatment capacity of Chinese children with WT is still insufficient, and only in some children's cancer centers, the 5-year survival rate of children reach 80% (25, 26). Although multidisciplinary combined treatment has dramatically increased the survival rate of children with WT, some high-risk children have low survival rates, especially in developing countries. Studies had shown that tumor rupture, no lymph node examined, insufficient chemotherapy intensity, insensitivity to chemotherapy were high-risk factors for the prognosis (27, 28). At present, although there was a nomogram to predict the CSS of patients with WT (10), there was no special nomogram for the survival prognosis of WT in children. Therefore, we used SEER database patients to screen independent risk factors and established a nomogram to predict the prognosis in children.
This study found that age was an independent risk factor for WT in children, and older age meant higher mortality, similar to previous studies (29). In addition, LND was also an independent risk factor in the study, and the previous research had also proved that LND was an important factor (30). SEER stage was also a risk factor, and patients with distant metastases had a worse prognosis. According to the stratification of risk value, the Kaplan-Meier curve and log-rank test proved that the nomogram had an excellent discriminating ability. Multivariate Cox regression analysis suggested that the risk factors of children in our hospital were significantly different from those in the SEER database. Race may be a potential factor, even though the race had never been a risk factor in previous studies (30, 31). In addition, our follow-up time was significantly shorter, which was also one of the reasons for the deviation of the results.
In the cases in our hospital, we found that the children were younger than patients in the SEER database, which may be related to the high incidence of WT in China (8). In addition, the number of LNs examined in our hospital was lower than that in the SEER database. The children in our hospital were young, and the lymph nodes were challenging to identify during the operation, resulting in a significant decrease in the number of LNs examined. Recent studies have shown that failure to sample lymph nodes during tumor resection was associated with increased tumor recurrence rates (31, 32). Due to the occult lymph node metastasis in some low-stage WT, these children have not been appropriately staged, resulting in insufficient treatment intensity. This was also a reason for the low survival rate of WT in Chinese children.
The construction of a nomogram requires a large number of patients to achieve sure accuracy and reliability. There were only a small number of patients with WT in our hospital, which cannot meet the needs of nomogram construction. The SEER database is a cancer database covering 28% of the US population, with many patients (21). So, the structure of the nomogram was based on patients from the SEER database in the United States. Due to ethnic differences and the baseline differences between the children in our hospital and the SEER database. However, on the contrary, external validation showed that this prediction model still had good accuracy and discrimination. The clinicopathological variables included in this nomogram were easy to obtain and were friendly to both doctors and patients.
Our study still has many limitations and deficiencies. First of all, there are still many variables in the SEER database that are not available, such as 1p and 16q gene mutation factors, which are essential gene variants that affect the prognosis of WT (33). A better model can be constructed if variables with genetic mutations are added. Secondly, because our study is a retrospective study, selection variants are difficult to avoid, such as the lack of tumor size in many patients, which results in a certain degree of bias. However, a previous study suggested that tumor size has a negligible effect on the prognosis (10). Finally, although we conducted external validation, the children's follow-up time was short, making it impossible to validate the long-term prognosis. Long-term prognostic prediction validation and even prospective clinical trials are necessary. With the improvement of medical technology, the prediction model will have some deviation. The prediction model we built needs to be constantly updated in the future to improve accuracy.
We constructed a nomogram to predict the prognosis of children with WT and We found that age, LND, and stage were independent risk factors for the prediction in children. Internal validation and external validation had been conducted to prove the nomogram has good accuracy and reliability. However, we need to further collect patients to improve the accuracy of the model in the future.
The original contributions presented in the study are included in the article/supplementary material, further inquiries can be directed to the corresponding author.
XT and JW: conceptualization. JW: methodology, investigation, and supervision. JT: software and formal analysis. XT, ML, and ZZ: validation. DH: resources. LJ: data curation. XT: writing—original draft preparation. ML: essay—review and editing. ZZ: visualization. DH: project administration and funding acquisition. All authors have read and agreed to the published version of the manuscript. All authors contributed to the article and approved the submitted version.
This research was funded by the Special Key Project of Chongqing Technology Innovation and Application Development (No. Cstc2019jscx-tjsbX0003).
The authors declare that the research was conducted in the absence of any commercial or financial relationships that could be construed as a potential conflict of interest.
All claims expressed in this article are solely those of the authors and do not necessarily represent those of their affiliated organizations, or those of the publisher, the editors and the reviewers. Any product that may be evaluated in this article, or claim that may be made by its manufacturer, is not guaranteed or endorsed by the publisher.
1. Davidoff AM. Wilms' tumor. Curr Opin Pediatr. (2009) 21:357–64. doi: 10.1097/MOP.0b013e32832b323a
2. Koshinaga T, Takimoto T, Oue T, Okita H, Tanaka Y, Nozaki M, et al. Outcome of renal tumors registered in Japan Wilms Tumor Study-2 (JWiTS-2): A report from the Japan Children's Cancer Group (JCCG). Pediatr Blood Cancer. (2018) 65:e27056. doi: 10.1002/pbc.27056
3. Spreafico F, Biasoni D, Lo Vullo S, Gandola L, D'Angelo P, Terenziani M, et al. AIEOP Wilms Tumor Working Group. Results of the Third AIEOP Cooperative Protocol on Wilms Tumor (TW2003) and Related Considerations. J Urol. (2017) 198:1138–45. doi: 10.1016/j.juro.2017.06.081
4. Termuhlen AM, Tersak JM, Liu Q, Yasui Y, Stovall M, Weathers R, et al. Twenty-five year follow-up of childhood Wilms tumor: a report from the Childhood Cancer Survivor Study. Pediatr Blood Cancer. (2011) 57:1210–6. doi: 10.1002/pbc.23090
5. Grundy P, Perlman E, Rosen NS, Warwick AB, Glade Bender J, Ehrlich P, et al. Current issues in Wilms tumor management. Curr Probl Cancer. (2005) 29:221–60. doi: 10.1016/j.currproblcancer.2005.08.002
6. D'Angio GJ, Evans AE, Breslow N, Beckwith B, Bishop H, Feigl P, et al. The treatment of Wilms' tumor: Results of the national Wilms' tumor study. Cancer. (1976) 38:633–46. doi: 10.1002/1097-0142(197608)38:2<633::aid-cncr2820380203>3.0.co;2-s
7. Phelps HM, Al-Jadiry MF, Corbitt NM, Pierce JM, Li B, Wei Q, et al. Molecular and epidemiologic characterization of Wilms tumor from Baghdad, Iraq. World J Pediatr. (2018) 14:585–93. doi: 10.1007/s12519-018-0181-3
8. Bao PP, Li K, Wu CX, Huang ZZ, Wang CF, Xiang YM, et al. Recent incidences and trends of childhood malignant solid tumors in Shanghai, 2002-2010. Zhonghua Er Ke Za Zhi. (2013) 51:288–94.
9. Breslow N, Olshan A, Beckwith JB, Green DM. Epidemiology of Wilms tumor. Med Pediatr Oncol. (1993) 21:172–81. doi: 10.1002/mpo.2950210305
10. Pan Z, You H, Bu Q, Feng X, Zhao F, Li Y, et al. Development and validation of a nomogram for predicting cancer-specific survival in patients with Wilms' tumor. J Cancer. (2019) 10:5299–305. doi: 10.7150/jca.32741
11. Sredni ST, Gadd S, Huang CC, Breslow N, Grundy P, Green DM, et al. Renal Tumor Committee of the Children's Oncology Group. Subsets of very low risk Wilms tumor show distinctive gene expression, histologic, and clinical features. Clin Cancer Res. (2009) 15:6800–9. doi: 10.1158/1078-0432.CCR-09-0312
12. Gratias EJ, Dome JS. Current and emerging chemotherapy treatment strategies for Wilms tumor in North America. Paediatr Drugs. (2008) 10:115–24. doi: 10.2165/00148581-200810020-00006
13. Park IJ, Choi GS, Jun SH. Nodal stage of stage III colon cancer: the impact of metastatic lymph node ratio. J Surg Oncol. (2009) 100:240–3. doi: 10.1002/jso.21273
14. Saltzman AF, Carrasco A Jr, Amini A, Aldrink JH, Dasgupta R, Gow KW, et al. Patterns of lymph node sampling and the impact of lymph node density in favorable histology Wilms tumor: An analysis of the national cancer database. J Pediatr Urol. (2018) 14:161.e1–161.e8. doi: 10.1016/j.jpurol.2017.09.025
15. Awais M, Ghayvat H, Krishnan Pandarathodiyil A, Nabillah Ghani WM, Ramanathan A, et al. Healthcare Professional in the Loop (HPIL): Classification of Standard and Oral Cancer-Causing Anomalous Regions of Oral Cavity Using Textural Analysis Technique in Autofluorescence Imaging. Sensors (Basel). (2020) 20:5780. doi: 10.3390/s20205780
16. Ghayvat H, Pandya SN, Bhattacharya P, Zuhair M, Rashid M, Hakak S, et al. CP-BDHCA: Blockchain-based Confidentiality-Privacy preserving Big Data scheme for healthcare clouds and applications. IEEE J Biomed Health Inform. (2021). doi: 10.1109/JBHI.2021.3097237
17. Mishra S, Tripathy HK, Kumar Thakkar H, Garg D, Kotecha K, Pandya S. An Explainable Intelligence Driven Query Prioritization Using Balanced Decision Tree Approach for Multi-Level Psychological Disorders Assessment. Front Public Health. (2021) 9:795007. doi: 10.3389/fpubh.2021.795007
18. Yan Y, Liu H, Mao K, Zhang M, Zhou Q, Yu W, et al. Novel nomograms to predict lymph node metastasis and liver metastasis in patients with early colon carcinoma. J Transl Med. (2019) 17:193. doi: 10.1186/s12967-019-1940-1
19. Zhang Z, Xie H, Chen P, Cao P. Development and Identification of a Nomogram Prognostic Model for Patients with Primary Clear Cell Carcinoma of the Liver. Med Sci Monit. (2020) 26:e919789. doi: 10.12659/MSM.919789
20. Cronin KA, Ries LA, Edwards BK. The Surveillance, Epidemiology, and End Results (SEER) Program of the National Cancer Institute. Cancer. (2014) 120 Suppl 23:3755–7.
21. Vickers AJ, Cronin AM, Elkin EB, Gonen M. Extensions to decision curve analysis, a novel method for evaluating diagnostic tests, prediction models and molecular markers. BMC Med Inform Decis Mak. (2008) 8:53. doi: 10.1186/1472-6947-8-53
22. Tang F, Zhang H, Lu Z, Wang J, He C, He Z. Prognostic Factors and Nomograms to Predict Overall and Cancer-Specific Survival for Children with Wilms' Tumor. Dis Markers. (2019) 2019:1092769. doi: 10.1155/2019/1092769
23. Smith MA, Altekruse SF, Adamson PC, Reaman GH, Seibel NL. Declining childhood and adolescent cancer mortality. Cancer. (2014) 120:2497–506. doi: 10.1002/cncr.28748
24. Dome JS, Graf N, Geller JI, Fernandez CV, Mullen EA, Spreafico F, et al. Advances in Wilms Tumor Treatment and Biology: Progress Through International Collaboration. J Clin Oncol. (2015) 33:2999–3007. doi: 10.1200/JCO.2015.62.1888
25. Yao W, Li K, Xiao X, Gao J, Dong K, Xiao X, et al. Outcomes of Wilms' tumor in eastern China: 10 years of experience at a single center. J Invest Surg. (2012) 25:181–5. doi: 10.3109/08941939.2011.615893
26. Pan C, Cai JY, Xu M, Ye QD, Zhou M, Yin MZ, et al. Renal tumor in developing countries: 142 cases from a single institution at Shanghai, China. World J Pediatr. (2015) 11:326–30. doi: 10.1007/s12519-015-0041-3
27. Grundy P, Breslow N, Green DM, Sharples K, Evans A, D'Angio GJ. Prognostic factors for children with recurrent Wilms' tumor: results from the Second and Third National Wilms' Tumor Study. J Clin Oncol. (1989) 7:638–47. doi: 10.1200/JCO.1989.7.5.638
28. Aoba T, Urushihara N, Fukumoto K, Furuta S, Fukuzawa H, Mitsunaga M, et al. Relapse of unilateral favorable histology Wilms' tumor: significant clinicopathological factors. J Pediatr Surg. (2012) 47:2210–5. doi: 10.1016/j.jpedsurg.2012.09.010
29. Metzger ML, Dome JS. Current therapy for Wilms' tumor. Oncologist. (2005) 10:815–26. doi: 10.1634/theoncologist.10-10-815
30. You H, Yang J, Liu Q, Tang L, Bu Q, Pan Z, et al. The impact of the lymph node density on overall survival in patients with Wilms' tumor: a SEER analysis. Cancer Manag Res. (2018) 10:671–7. doi: 10.2147/CMAR.S163514
31. Zhuge Y, Cheung MC, Yang R, Koniaris LG, Neville HL, Sola JE. Improved survival with lymph node sampling in Wilms tumor. J Surg Res. (2011) 167:e199–203. doi: 10.1016/j.jss.2010.12.026
32. Raval MV, Bilimoria KY, Bentrem DJ, Stewart AK, Winchester DP, Ko CY, et al. Nodal evaluation in Wilms' tumors: analysis of the national cancer data base. Ann Surg. (2010) 251:559–65. doi: 10.1097/SLA.0b013e3181cc95d7
Keywords: nomogram, cancer-specific survival, Wilms tumor, children, SEER
Citation: Tan X, Wang J, Tang J, Tian X, Jin L, Li M, Zhang Z and He D (2022) A Nomogram for Predicting Cancer-Specific Survival in Children With Wilms Tumor: A Study Based on SEER Database and External Validation in China. Front. Public Health 10:829840. doi: 10.3389/fpubh.2022.829840
Received: 06 December 2021; Accepted: 28 February 2022;
Published: 07 April 2022.
Edited by:
Thippa Reddy Gadekallu, VIT University, IndiaReviewed by:
Sharnil Pandya, Symbiosis International University, IndiaCopyright © 2022 Tan, Wang, Tang, Tian, Jin, Li, Zhang and He. This is an open-access article distributed under the terms of the Creative Commons Attribution License (CC BY). The use, distribution or reproduction in other forums is permitted, provided the original author(s) and the copyright owner(s) are credited and that the original publication in this journal is cited, in accordance with accepted academic practice. No use, distribution or reproduction is permitted which does not comply with these terms.
*Correspondence: Dawei He, aGVkYXdlaUBob3NwaXRhbC5jcW11LmVkdS5jbg==
Disclaimer: All claims expressed in this article are solely those of the authors and do not necessarily represent those of their affiliated organizations, or those of the publisher, the editors and the reviewers. Any product that may be evaluated in this article or claim that may be made by its manufacturer is not guaranteed or endorsed by the publisher.
Research integrity at Frontiers
Learn more about the work of our research integrity team to safeguard the quality of each article we publish.