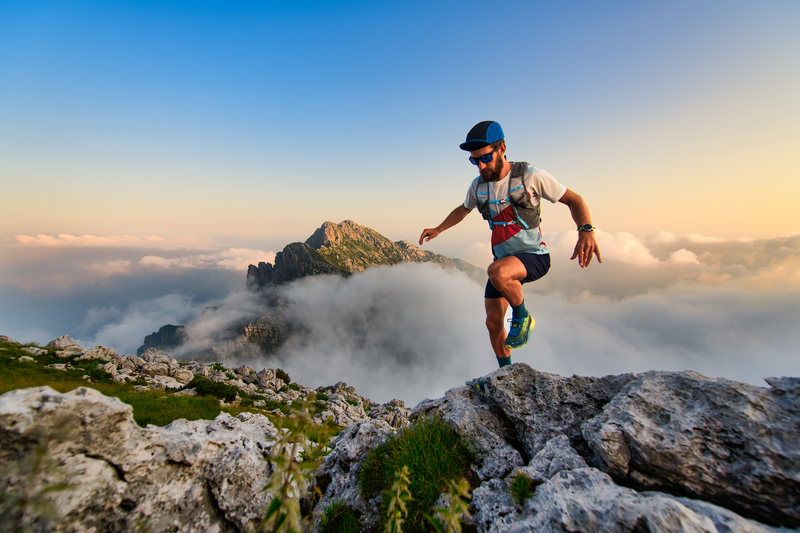
94% of researchers rate our articles as excellent or good
Learn more about the work of our research integrity team to safeguard the quality of each article we publish.
Find out more
ORIGINAL RESEARCH article
Front. Public Health , 02 February 2022
Sec. Health Economics
Volume 10 - 2022 | https://doi.org/10.3389/fpubh.2022.787929
This article is part of the Research Topic Addressing the Effects of COVID-19 on Rural Areas in Low and Middle Income Countries View all 12 articles
COVID-19 is an unprecedented challenge for public health worldwide. Reducing the incidence of the disease requires protective measures to prevent virus transmission. Understanding those factors influencing preventive behavior is the first step in preventing the spread of the disease. This study investigates factors affecting youth intention and preventive behaviors in the face of COVID-19 through the health belief model by using a cross-sectional survey collected through an online questionnaire. The sample comprises 304 rural youth in South Iran who were selected through a random sampling technique. The results reveal that perceived severity, perceived benefits, public health beliefs, perceived self-efficacy, and the cue to act positively and significantly affect preventive behaviors. The model explains 59% of variance changes in rural youth preventive behaviors during COVID-19. Cue to action is the strongest and self-efficacy was the weakest determinant of youth's preventive behavior. This study confirms that the HBM framework has appropriate predictive power and is an effective tool for investigating preventive behaviors during COVID-19. These results provide important policy implications for the development of policies that aim to avoid the further spread of COVID-19 between young citizens.
On January 30, 2020, the World Health Organization (WHO) issued a statement declaring the outbreak of the new coronavirus to be a public health emergency and a threat to the entire world (1). Due to its contagious nature and rapid spread around the world, on March 11, 2020, the World Health Organization confirmed it as a pandemic (2). The disease severely disrupted the daily activities of more than half of the world's population. The movement of people and many goods from different regions stopped completely (3). The coronavirus not only severely affected people's health, it also affected the world economy (4). The increasing outbreak of COVID-19 cases and deaths has also increased the fears of household vulnerability to food insecurity worldwide (5).
To counteract the rapid spread of the disease, key action areas were promoted by many governments, seeking to minimize virus transmission by promoting preventive behaviors that would flatten the peak of the disease and reduce its impact on health care, allowing doctors to treat severe cases while also reducing overall mortality (6). Existing research shows that the rapid spread of COVID-19 is strongly associated with the displacement of people with no-to-mild symptoms; i.e., those who are unaware they are infected (7). Therefore, non-medical practices, such as promoting protective behaviors like staying at home (home quarantine and reducing human interactions), increasing social distance, avoiding travels, and following personal hygiene tips (washing hands regularly and using masks when going to public places and crowded places) (8, 9), are used to control the spread of the disease (10). Promoting these preventive behaviors is essential for slowing the spread of COVID-19 (11, 12).
Despite the availability of COVID-19 prevention recommendations, people's adoption of behaviors that prevent the spread of COVID-19 differs between groups, generally leaving room for improvement. Monitoring people's perceptions and behavioral responses to COVID-19 is necessary to improve health risk communications and achieve successful changes in people's behaviors. However, globally, there is little information about people's perceptions and preventive behaviors regarding COVID-19.
Protective measures to prevent the transmission of the disease largely depend on rapid changes in the behavior of communities, thus relying on the ability of individuals to understand the risks associated with the virus and adapt their behaviors accordingly (13). For example, Wise et al. (13) show that understanding higher personal risk predicts preventive behaviors like hand washing and social distance. Akter (6) show that people who perceive a threat from COVID-19 collect medical and cleaning equipment, while those who do not take the disease seriously are careless, for example, moving around freely, traveling, gathering together, and not engaging in any preventive behaviors.
Reports indicate that rural communities perform fewer preventive health behaviors than urban communities. Villagers are not just more likely to have a negative attitude toward the effectiveness of preventive behaviors but also have a lower level of information about COVID-19 and its prevention measures (14). Studies also show that rural communities face health inequalities due to numerous barriers, including a lack of health care infrastructure (comprising transportation, health insurance, providers, and facilities), geographical distance, and lower socioeconomic status (15–17). The lack of these resources, services, and support put these populations at a higher risk and vulnerability (17). Public health researchers are concerned that rural communities may experience a worse situation in relation to the COVID-19 pandemic, for example, higher mortality rates, than their urban and suburban counterparts due to rural/urban health inequalities (18, 19).
In addition, reports indicate that rural communities, especially rural youth, are not taking the risk of the disease seriously and are continuing their daily activities. Further, people are usually reluctant to employ preventive behaviors due to physical discomfort, cost, and inconvenience (20). The results of an Italian study by Ceccato et al. (21) show that younger people are less confident in the information received about COVID-19 and are less interested in restrictive measures. They think that most deaths are related to the elderly or people with underlying diseases.
According to FAO reports (20), rural youth suffer disproportionately from the ubiquity of COVID-19 and its consequences because they are among the most vulnerable groups during the twenty-first century. Facing high unemployment rates, not only do rural youth have very little financial strength and income, but they also lack health insurance coverage and other social supports (20). Young villagers are vital stakeholders because they are in direct contact with food resources in supply chains. Their travel to urban areas and remaining there is in opposition to health advice and can increase the spread of the disease. For this reason, the protection of this group and the encouragement of healthy behaviors within it are of major importance. The study of health protection measures in Iran is important because it was the first low- or middle-income country to suffer a major outbreak including rural areas and learning from Iran's experience will help all low- and middle-income countries (22). Certainly in developing countries, including Iran, the shock of COVID-19 will be much more severe because most farmers in rural areas in these countries are not just prone to poverty and extreme vulnerability but they also have limited access to formal mechanisms to deal with these shocks (23).
Following these findings, this study investigates why the adoption of preventive behavior by youth differs from other age groups? This research seeks to answer this question among rural youth in Bushehr province of southern Iran. Iran and its southern region are chosen because of its severe experience with COVID-19 (5, 17, 24, 25). According to statistics, as of August 10, 2021, COVID-19 has resulted in 94,603 deaths in Iran, with numbers continuing to increase (26). The existing literature shows that various factors affect the preventive behavior of people with respect to infectious diseases. Further, the behaviors of people in potentially high-risk groups can play an important role in preventing and controlling infectious diseases (27). Thus, it is important to examine why some people, especially rural youth, participate in the prevention, screening, and control of health behaviors regarding COVID-19, while others do not. However, little information exists on the reasons for the participation or non-participation of rural youth in Iran with respect to preventive behaviors for COVID-19 and the evaluation of the factors affecting this behavior.
Given the significance of psychological and behavioral factors in the management of severe global pandemics, like COVID-19, it is essential to assess behavioral and psychological responses to the situation and to determine the relationship between perceived risk and participation in engaging in protective behaviors to facilitate disease minimization strategies (13). Studies use various theories and models to investigate the factors influencing preventive behaviors for various diseases, including COVID-19. For example, the health belief model to predict the use of personal protective equipment in SARS outbreaks (28), unified theory of acceptance and use of technology to predict the willingness to wear a mask (29), the theory of protection motivation against COVID-19 (22, 30), as well as knowledge, attitudes, and practices theory to investigate preventive behaviors against COVID-19 (27). In this regard, Mukhtar (31) recommends using the Health Beliefs Model (HBM) for recognizing preventive behaviors during the COVID-19. Since, HBM is one of the most functional theories related to behavioral change and preventive health behaviors (32), this theory is used in this study.
In addition, recent studies on the use of psychological structures and models in predicting COVID-19 prevention behaviors have focused on different groups of people. For example, study Irigoyen-Camacho et al. (33) in Mexocity on the behavior of staying at home among a group of adults, Roberts and David (34) on the general acceptance of preventive behaviors by undergraduate students in the United States, Šurina et al. (35) on compliance with health recommendations and social distance among the Latvian general population, and the study Lin et al. (36) on the acceptance of preventive behaviors by the people of Iran. However, in most of these studies, the rural youth group is neglected. Therefore, this study is innovative in two ways: first, an HBM theory has been used and second, it has considered rural youth. Therefore, in this study, the HBM framework, one of the most popular health research frameworks, is used to understand why rural youth take or do not take action for personal or community health in the face of COVID-19.
HBM was introduced in the early 1950s by social psychologists in the United States (37). HBM assumes that safe actions originate from two main mechanisms: One's attitude toward danger (threat perception) and individual assessment of preventive behavior (behavioral evaluation). Perception of threat includes two sub-components or social psychological beliefs: perceived susceptibility (PSU) and perceived severity (PSE). The behavioral assessment comprises two sub-components of perceived benefits (PBE) and perceived barriers (PBR) (38, 39). PSU means people's beliefs regarding the probability of getting an illness (32). PSE means the belief that a severe health problem can lead to death or other serious consequences (40–42). The PBE refers to a person's assessment of the worth or effectiveness of performing health-oriented behaviors to reduce the risk of disease (37). Perceived barriers refer to a person's estimation of challenges to behavior change (43). HBM assumes that people engage in preventative behaviors if they (i) believe that they are more likely to get a disease (perceived susceptibility); (ii) understand the depth of the risk and its severity (perceived severity); (iii) perceive the benefits by adopting preventive health behaviors; and (iv) recognize that there is an imperfect obstacle to the implementation of preventive behaviors (44, 45) (see Figure 1).
In addition to these variables, HBM includes other variables that predict behavior, including self-efficacy, cue to action, and general health beliefs (46). Self-efficacy originates from Bandura's known theory (Social Cognitive Theory), which refers to the confidence and belief that the person can perform a specific behavior (47). HBM also states that a trigger is necessary to stimulate the conduct of health behaviors. Cues to action remind someone to engage in a particular behavior (48). Cue to action may be internal (physical symptoms of pain, fever, cough) or external (friends, health care providers, media, and social media) (49). In HBM, general health beliefs refer to the person's disposition or routine regarding health seeking behavior in general. It is not related to the expected concerns of healthcare behavior; instead, it is a person's general response predisposition (50).
Therefore, the present study examines seven following hypotheses.
H1) Perceived Susceptibility positively affects people's preventive behavior.
H2) Perceived severity positively affects people's preventive behavior.
H3) Perceived benefits positively affect people's preventive behavior.
H4) Perceived barriers negatively affect people's preventive behavior.
H5) Perceived self-efficacy positively affects people's preventive behavior.
H6) Cue to action positively affects people's preventive behavior.
H7) General health beliefs directly affect people's preventive behavior.
The present study is applied research in terms of purpose, non-experimental in terms of control variables, and a field survey in terms of data collection. This study is quantitative research carried out through a sectional online survey. Data were collected in August 2020 through an online platform; all data were collected in the Persian language. The population of concentration (statistical population) of this study comprises rural youth (between 18 and 30 years) of Dashtestan county in Bushehr province in southern Iran (N = 1,500). Based on (51) table and using random sampling, 304 rural youths were selected as the study sample. The mean age of respondents was 24.79 years. In terms of gender, 125 people (41%) were male, and 180 were female (59%). The average household size was 4.68, with a standard deviation of 1.67.
The data collection tool in this study was an online questionnaire. It consisted of two sections, including socio-economic characteristics and the HBM variables. HBM variables measured in the form of a spectrum of a 5-point Likert scale: “very low (1)“ to ”very high (5)" that were extracted from previous studies. Faculty members confirmed the facial and content validity and psychometric properties of the questionnaire. The internal validity of each variable examined using Cronbach's alpha. As shown in Table 1, all scales had good to excellent validity, generally between 0.78 and 0.93 (Table 1). SPSSversion24 and AMOSversion20 software used to analyze the data.
The Pearson correlation test is applied to examine the correlation among HBM constructs (Table 2). The results show that there is a positive and significant correlation between preventive behavior and perceived severity, PSU, PBE, self-efficacy, general health beliefs, and cue to action. The PBR is not meaningfully correlated to preventive behavior.
Structural equation modeling is used to investigate the explanatory power of HBM in predicting preventive behaviors. The two stages of structural equation modeling include Confirmatory Factor Analysis (CFA) to evaluate the suitability of the measurement model and structural equation modeling (53).
To estimate the measurement models of the components affecting preventive behavior, the data collected using AMOS 20 was analyzed using CFA. The results show the measurement model has an acceptable fit. (RMSEA = 0.038; CMIN = 796.705, DF = 550, p = 0.000; CMIN/DF = 1.449; CFI = 0.957; GFI = 0.878; AGFI = 0.853; IFI = 0.957; and NFI = 0.874). The acceptable level of CFI, IFI, GFI, and AGFI is generally equal to, or greater than, 0.9. Values from 0.8 to 0.9 are considered marginal. RMSEA is acceptable between 0.03 and 0.08 and relative Chi-square (CMIN/DF) equal to, or less than, three is associated with a better fit (54). Thus, the results of our measurement model commonly show an adequate fit.
To confirm convergent validity, the average variance extracted (AVE) and composite reliability (CR) were calculated. AVE results show that the value of this index for all variables studied is more than 0.5. Except for perceived severity, perceived benefits, and behavior, the composite reliability calculated for the model variables was >0.7 (Table 2). The acceptable value for AVE and CR is 0.5 and 0.7, respectively (54). However, according to (55), if the composite reliability is >0.6, an AVE <0.5 is acceptable. Therefore, the instrument has an acceptable convergent validity.
The process of validation and the structural equation model reveal sturdiness for the practical data, thus meeting the necessities of convinced indexes. The goodness-of-fit indices suggested that the HBM model has a suitable fit (RMSEA = 0.038; CMIN = 796.705, DF = 550, p = 0.000; CMIN/DF = 1.449; CFI = 0.957; GFI = 0.878; AGFI = 0.853; IFI = 0.957; and NFI = 0.874). In Table 3, it is shown that 5 out of 7 hypotheses are confirmed: Hypotheses H1 and H4 are not empirically confirmed. In the HBM model, the variables of self-efficacy (β = 0.141, p < 0.05), general health beliefs (β = 0.248, p < 0.0001), PSE (β = 0.177, p < 0.05), PBE (β = 0.142, p < 0.05), and cue to action (β = 0.347, p < 0.0001) have a significant impact on behavior and are able to predict 59% of behavioral changes (Figure 2).
Figure 2. The results of the structural equation modeling (own design; factor loading of each items placed in the small boxes).
Effective suppression of COVID-19 requires nontrivial efforts by a substantial portion of the world's population, including social distancing and preventing unnecessary meetings with others. No matter how strongly these actions suggested, some individuals may not trust that they can or should follow these recommendations (8). For effective policymaking to prevent the spread of the disease, it is crucial to know how beliefs affect the adoption of preventive measures. Using HBM, an approach used to exam behaviors related to disease prevention or reduction this study answers this question. Here, the relationship between health beliefs and preventive behaviors is investigated using HBM.
Results show that HBM predicts a significant percentage (59%) of preventive behavior, which is high in the psychological and behavioral literature. The results of the study also show that the cue to action, general health beliefs, PSE, PBE, and self-efficacy can predict preventive behaviors. However, PSU and PBR do not have a significant impact on preventive behavior.
According to the findings, among the variables of threat assessment, the perceived severity variable is a positive predictor of protective behaviors (Hypothesis H1). If a person feels that getting COVID-19 disease will profoundly affect their life, they are more likely to engage in protective behaviors. Akter (6) points out that some people do not take the COVID-19 pandemic seriously. Whether or not people engage in preventive activities depends on how seriously they take the consequences of the disease. The results are consistent with existing studies (13, 56–58).
Based on the findings, understanding the benefits of performing preventive behavior significantly affects that behavior (Hypothesis H3). People do not change their behavior until they receive something in return for doing so (6).
The more respondents feel that the recommended behaviors (home quarantine and the use of safeguards) effectively prevent coronavirus infection, the more likely they are to implement the behaviors. In other words, if a person believes in the benefits of preventive activities to reduce the risks, they are more willing to engage in them. This result is constant with the existing literature (44, 45, 56, 57).
Perceived self-efficacy is another predictor of protective behaviors (Hypothesis H5). Self-efficacy is an individual's belief in his or her capability to change performance (47); here, the extent to which a person feels that he or she can apply protective methods. If a person believes in their ability to access and use protective equipment, they are likely to engage in protective behavior. This result is consistent with previous studies (22, 44, 45, 57, 59). The cue to action is the most important predictor of people's protective behavior (hypothesis H6). Cue to action is a stimulus that motivates people to take action to prevent the transmission of the disease. These stimuli can be clips or news about the death of people due to COVID-19 or the effects and consequences of this disease on the lives of people, whether via mass media or social networks (6). Seeing or hearing about any of these (cues to action) can encourage people to take protective measures. These findings are consistent with previous studies (56, 57, 60). The present study provides evidence of the effectiveness of public health beliefs in performing preventive behaviors (Hypothesis H7). Public health beliefs refer to a person's values, beliefs, concerns, and readiness for health issues in daily activities. People who are aware of health issues and choose a wellness-oriented routine are more likely to engage in preventative actions than people who are unaware of health issues (61). In this study, young people who have a higher level of awareness about health measures and their impact on health are more likely to engage in preventive behaviors. The effect of PSU and PBR are not significant in the preventive behaviors of rural youth (Hypothesis H2, H4).
The present study provides important results for the development of policies and guidelines to avoid the further spread of COVID-19. Officials should inform rural youth of the severe consequences of the disease through enhanced news or the provision of educational materials (guidelines and recommendations). Young people need to know that, although the disease is incurable, they can prevent the severe consequences of the disease and reduce its damage. Policies and programs encouraging rural youth to engage in preventive behaviors should include education through mass media, social media, posters, and other advertisements.
Given the importance of self-efficacy, agricultural promotion experts, local associations, councils, and local trustees can talk to rural youth about the ease with which preventative behaviors can be practiced, thus building the confidence and beliefs of rural youth that they can effectively implement preventive measures to prevent disease. Given the vital role of perceived benefits in implementing preventive behavior, the extension efforts should highlight the benefits of preventive behavior, such as protecting not just their own lives but also their loved ones. To improve the efficacy of prevention efforts, extension experts and health officials need to strengthen public health beliefs regarding COVID-19 through information and awareness campaigns that use publicity and educational materials on preventive activities. Finally, we believe that COVID-19 will create a new role for agricultural extension services in developing countries like Iran. Most variables affecting preventive behavior are triggered by education and awareness; thus, training and awareness about the depth of risk and seriousness of the disease, public health beliefs, stimulants (cues/trigger), and the benefits of preventive behaviors along with the ability of young people to perform these actions are fundamental and necessary. As extension agents have been working in rural areas for years, educating and working with villagers, the simplest and cheapest way to achieve broad outreach in rural areas is to equip extension agents with the materials needed to teach villagers how to deal with COVID-19.
The adoption of preventive measures varies across regions, socio-economic status, and age of people. Understanding behavioral differences is crucial for better targeting education, campaigns, and policies toward different groups. Only if the needs of different groups are properly accounted for will adoption rates be enhanced and the spread of diseases such as COVID-19 limited. In addition, people's perceptions are very influential in a rapid change in their behavior toward COVID-19. This study investigates the factors affecting the preventive behavior of rural youth in relation to the COVID-19 pandemic using HBM. The results using structural equations show good explanatory power for self-reported behavior. The HBM model predicts 59% of the variance of preventive behavior against COVID-19 outbreaks, such as wearing gloves and masks, regular hand washing, and regular hand disinfection. All of HBM's independent constructs, except perceived susceptibility and perceived barriers affect preventive behavior. The results show that cue to action is the strongest predictor of behavior.
This study adds new and important knowledge to existing information on the effectiveness of HBM in predicting the preventive behaviors of rural youth against COVID-19. The results of this study are useful for designing better policies and public information campaigns on measures to contain the spread of health diseases such as COVID-19. Further, the results of this study can be used as a basis for further studies on the COVID-19 pandemic. This theory could also be a roadmap for modifying adaptive behaviors to help effectively manage unknown pandemics in the future.
While this study is valuable information to the body of research on COVID-19, it is not without its limitations. This study is a cross-sectional study and its data was collected in August 2020 in Iran, so caution should be exercised in generalizing the results to other regions and times. Another potential limitation of this study was that the questionnaire was online and as a result, the respondents were the only people who had access to the Internet. While not all villagers have high access to the Internet. Therefore, it is possible that some villagers are less likely to participate in data collection. Another limitation is that behaviors are self-reported. The self-report does not allow the assessment of actual behavior (35). It is suggested that in future studies, the actual behaviors of individuals be examined. In addition, the paradigm of this study is quantitative. It is suggested that combined quantitative-qualitative methods be used in future studies to obtain more accurate results.
The raw data supporting the conclusions of this article will be made available by the authors, without undue reservation.
Ethical review and approval was not required for the study on human participants in accordance with the local legislation and institutional requirements. The patients/participants provided their written informed consent to participate in this study.
MT, TZ, KL, and SS contributed to conception and design of the study. MT and TZ conducted the data collection, performed the statistical analysis, and wrote the first draft of the manuscript. KL and SS wrote sections of the manuscript. All authors contributed to manuscript revision, read, and approved the submitted version.
The authors declare that the research was conducted in the absence of any commercial or financial relationships that could be construed as a potential conflict of interest.
All claims expressed in this article are solely those of the authors and do not necessarily represent those of their affiliated organizations, or those of the publisher, the editors and the reviewers. Any product that may be evaluated in this article, or claim that may be made by its manufacturer, is not guaranteed or endorsed by the publisher.
We dedicate our work to the nurses and physicians worldwide who are bravely and tirelessly fighting COVID-19.
1. Zhu N, Zhang D, Wang W, Li X, Yang B, Song J, et al. A novel coronavirus from patients with pneumonia in China, 2019. New Engl J Med. (2020) 382:727–33. doi: 10.1056/NEJMoa2001017
2. World Health Organization. WHO Director-General's Opening Remarks at the Media Briefing on COVID-19-11 March 2020. (2020). Geneva, Switzerland: World Health Organization. Available on: https://www.who.int/director-general/speeches/detail/who-director-general-s-opening-remarks-at-the-media-briefing-on-covid-19—11-march-2020 (accesesed July 12, 2021).
3. Iqbal N, Fareed Z, Shahzad F, He X, Shahzad U, Lina M. Nexus between COVID-19, temperature and exchange rate in Wuhan City: New findings from Partial and Multiple Wavelet Coherence. Scie Total Environ. (2020) 729:138916 doi: 10.1016/j.scitotenv.2020.138916
4. Baker SR, Bloom N, Davis SJ, Kost K, Sammon M, Viratyosin T. The unprecedented stock market reaction to COVID-19. Covid Econ Vetted Real-Time Papers. (2020) 1. doi: 10.3386/w26945
5. Pakravan-Charvadeh MR, Savari M, Khan HA, Gholamrezai S, Flora C. Determinants of household vulnerability to food insecurity during COVID-19 lockdown in a mid-term period in Iran. Public Health Nutr. (2021) 24:1619–28. doi: 10.1017/S1368980021000318
6. Akter J,. Human Behavior Toward COVID-19. (2020). Available on the https://www.researchgate.net/publication/340066427_Title_Human_Behavior_toward_COVID-19 (accesesed July 10, 2021).
7. Liu X, Zhang D, Sun T, Li X, Zhang H. Containing COVID-19 in rural and remote areas: experiences from China. J Travel Med. (2020) 27:taaa060 doi: 10.1093/jtm/taaa060
8. Carico RR, Sheppard J, Thomas CB. Community pharmacists and communication in the time of COVID-19: Applying the health belief model. Res Social Adm Pharm. (2020). In Press. doi: 10.1016/j.sapharm.2020.03.017
9. Yang L, Ren Y. Moral obligation, public leadership, and collective action for epidemic prevention and control: evidence from the corona virus disease 2019 (COVID-19) emergency. Int J Environ Res Public Health. (2020) 17:2731. doi: 10.3390/ijerph17082731
10. van der Weerd W, Timmermans DR, Beaujean DJ, Oudhoff J, van Steenbergen JE. Monitoring the level of government trust, risk perception and intention of the general public to adopt protective measures during the influenza A (H1N1) pandemic in the Netherlands. BMC Public Health. (2011) 11:575. doi: 10.1186/1471-2458-11-575
11. Prusaczyk B. Strategies for disseminating and implementing COVID-19 public health prevention practices in rural areas. J Rural Health. (2020) 37:142–4. doi: 10.1111/jrh.12432
12. Van den Broucke S. Why health promotionmatters to the COVID-19 pandemic, and vice versa. Health Promot Int. (2020) 35:181–6. doi: 10.1093/heapro/daaa042
13. Wise T, Zbozinek TD, Michelini G, Hagan CC. Changes in risk perception and protective behavior during the first week of the COVID-19 pandemic in the United States (2020). doi: 10.31234/osf.io/dz428
14. Chen X, Chen H. Differences in preventive behaviors of COVID-19 between urban and rural residents: lessons learned from a cross-sectional study in China. Int J Environ Res Public Health. (2020) 17:4437. doi: 10.3390/ijerph17124437
15. Sparks PJ. Rural health disparities. In: Kulcsár LJ, Curtis KJ, editors. International Handbook of Rural Demography. Springer: Amsterdam, The Netherlands. (2012). p. 255–71. doi: 10.1007/978-94-007-1842-5_18
16. Thomas TL, DiClemente R, Snell S. Overcoming the triad of rural health disparities: How local culture, lack of economic opportunity, and geographic location instigate health disparities. Health Educ J. (2014) 73:285–94. doi: 10.1177/0017896912471049
17. Yazdanpanah M, Tajeri Moghadam M, Savari M, Zobeidi T, Sieber S, Löhr K. The impact of livelihood assets on the food security of farmers in Southern Iran during the COVID-19 pandemic. Int J Environ Res Public Health. (2021) 18:5310. doi: 10.3390/ijerph18105310
18. Curley C,. Rural America Could Be the Region Hardest Hit by the COVID-19 Outbreak. (2020). Available online: https://www.healthline.com/health-news/rural-america-hardest-hit-by-covid-19-outbreak (accessed on April 21, 2020).
19. Henning-Smith C, Tuttle M, Kozhimannil KB. Unequal distribution of COVID-19 risk among rural residents by race and ethnicity. J Rural Health. (2021) 37:224–6. doi: 10.1111/jrh.12463
20. FAO. Coping with COVID-19: Voices of Young Agripreneurs. (2020). Available on: http://www.fao.org/rural-employment/work-areas/youth-employment/ica-programme/uganda/en/ (accesesed July 24, 2021).
21. Ceccato I, Palumbo R, Di Crosta A, La Malva P, Marchetti D, Maiella R, et al. Age-related differences in the perception of COVID-19 emergency during the Italian outbreak. Aging Mental Health. (2021) 25:1305–13. doi: 10.1080/13607863.2020.1856781
22. Yazdanpanah M, Abadi B, Komendantova N, Zobeidi T, Sieber S. Some at risk for COVID-19 are reluctant to take precautions, but others are not: A case from rural in Southern Iran. Front Public Health. (2020) 8:678. doi: 10.3389/fpubh.2020.562300
23. Shehu A, Sidique SF. The effect of shocks on household consumption in rural Nigeria. J Develop Areas. (2015) 353–64. doi: 10.1353/jda.2015.0163
24. CNN. 1 Iranian Dies from Coronavirus Every 10 Minutes, Health Ministry Says. (2020). Available on the https://edition.cnn.com/world/live-news/coronavirus-outbreak-03-19-20-intl-hnk/h_7449e0f9d299c2a4205f5e20ceeef577 (accesesed July 12, 2021).
25. Yazdanpanah M, Zobeidi T, Tajeri Moghadam M, Komendantova N, Löhr K, Sieber S. Cognitive theory of stress and farmers' responses to the COVID 19 shock; a model to assess coping behaviors with stress among farmers in southern Iran. Int J Disaster Risk Reduct. (2021) 64:102513. doi: 10.1016/j.ijdrr.2021.102513
26. Worldmeters. Total Coronavirus Cases in Iran. (2021). Available on the https://www.worldometers.info/coronavirus/country/iran/ (accesesed August 10, 2021).
27. Gao H, Hu R, Yin L, Yuan X, Tang H, Luo L, et al. Knowledge, attitudes and practices of the Chinese public with respect to coronavirus disease (COVID-19): an online cross-sectional survey. BMC Public Health. (2020) 20:1–8. doi: 10.1186/s12889-020-09961-2
28. Tan NC, Goh LG, Lee SS. Family physicians' experiences, behaviour, and use of personal protection equipment during the SARS outbreak in Singapore: do they fit the Becker Health Belief Model? Asia Pacific J Public Health. (2006) 18:49–56. doi: 10.1177/10105395060180030901
29. Zhou M, Long P, Kong N, Campy KS. Characterizing Wuhan residents' mask-wearing intention at early stages of the COVID-19 pandemic. Patient Educ Couns. (2021) 104:1868–77. doi: 10.1016/j.pec.2020.12.020
30. Al-Rasheed M. Protective behavior against COVID-19 among the public in Kuwait: An examination of the protection motivation theory, trust in government, and sociodemographic factors. Soc Work Public Health. (2020) 35:546–56. doi: 10.1080/19371918.2020.1806171
31. Mukhtar S. Mental health and emotional impact of COVID-19: Applying Health Belief Model for medical staff to general public of Pakistan. Brain Behav Immun. (2020) 87:28–9. doi: 10.1016/j.bbi.2020.04.012
32. Champion VL, Skinner CS. The health belief model. Health Behav Health Educ Theor Res Pract. (2008) 4:45–65.
33. Irigoyen-Camacho ME, Velazquez-Alva MC, Zepeda-Zepeda MA, Cabrer-Rosales MF, Lazarevich I, Castaño-Seiquer A. Effect of income level and perception of susceptibility and severity of COVID-19 on stay-at-home preventive behavior in a group of older adults in Mexico City. Int J Environ Res Public Health. (2020) 17:7418. doi: 10.3390/ijerph17207418
34. Roberts JA, David ME. Improving predictions of COVID-19 preventive behavior: development of a sequential mediation model. J Med Internet Res. (2021) 23:e23218. doi: 10.2196/23218
35. Šurina S, Martinsone K, Perepjolkina V, Kolesnikova J, Vainik U, RuŽa A, et al. Factors related to COVID-19 preventive behaviors: a structural equation model. Front Psychol. (2021) 12. doi: 10.3389/fpsyg.2021.676521
36. Lin CY, Imani V, Majd NR, Ghasemi Z, Griffiths MD, Hamilton K, et al. Using an integrated social cognition model to predict COVID-19 preventive behaviours. Br J Health Psychol. (2020) 25:981–1005. doi: 10.1111/bjhp.12465
37. Janz NK, Becker MH. The health belief model: a decade later. Health Educ Q. (1984) 11:1–47. doi: 10.1177/109019818401100101
38. Simşekoglu Ö, Lajunen T. Social psychology of seat belt use: a comparison of theory of planned behavior and health belief model. Transp Res Part F: Traffic Psychol Behav. (2008) 11:181–91. doi: 10.1016/j.trf.2007.10.001
39. Yazdanpanah M, Komendantova N, Shirazi ZN, Linnerooth-Bayer J. Green or in between? Examining youth perceptions of renewable energy in Iran. Energy Res Social Sci. (2015) 8:78–85. doi: 10.1016/j.erss.2015.04.011
40. Yazdanpanah M, Forouzani M, Hojjati M. Willingness of Iranian young adults to eat organic foods: application of the Health Belief Model. Food Qual Prefer. (2015) 41:75–83. doi: 10.1016/j.foodqual.2014.11.012
41. Bakhtiyari Z, Yazdanpanah M, Forouzani M, Kazemi N. Intention of agricultural professionals toward biofuels in Iran: Implications for energy security, society, and policy. Renew Sustain Energy Rev. (2017) 69:341–9. doi: 10.1016/j.rser.2016.11.165
42. Tajeri moghadam M, Raheli H, Zarifian S, Yazdanpanah M. The power of the health belief model (HBM) to predict water demand management: A case study of farmers' water conservation in Iran. J Environ Manage. (2020) 263:110388. doi: 10.1016/j.jenvman.2020.110388
43. Orji R, Vassileva J, Mandryk R. Toward an effective health interventions design: an extension of the health belief model. Online J Public Health Inform. (2012) 4. doi: 10.5210/ojphi.v4i3.4321
44. Huang X, Dai Sh, Xu H. Predicting tourists' health risk preventative behaviour and travelling satisfaction in Tibet: combining the theory of planned behaviour and health belief model. Tourism Manage Perspect. (2020) 33:100589. doi: 10.1016/j.tmp.2019.100589
45. Huang C, Wang Y, Li X, Ren L, Zhao J, Hu Y, et al. Clinical features of patients infected with 2019 novel coronavirus in Wuhan, China. Lancet. (2020) 395:497–506. doi: 10.1016/S0140-6736(20)30183-5
46. Becker MH, Rosenstock IM. Comparing social learning theory and the health belief model. Adv Health Educ Promot. (1987) 2:245–9.
47. Groenewold G, Bruijn B, Bilsborrow R. Psychosocial factors of migration: Adaptation and application of the health belief model. Int Migr. (2012) 50:211–31. doi: 10.1111/j.1468-2435.2012.00781.x
48. Beaujean DJMA, Bults M, van Steenbergen JE, Voeten HACM. Study on public perceptions and protective behaviors regarding Lyme disease among the general public in the Netherlands: implications for prevention programs. BMC Public Health. (2013) 13:225. doi: 10.1186/1471-2458-13-225
49. Carpenter CJ. A meta-analysis of the effectiveness of health belief model variables in predicting behavior. Health Commun. (2010) 25:661–9. doi: 10.1080/10410236.2010.521906
50. Ng BY, Kankanhalli A, Xu YC. Studying users' computer security behavior: A health belief perspective. Decis Support Syst. (2009) 46:815–25. doi: 10.1016/j.dss.2008.11.010
51. Krejcie RV, Morgan DW. Determining sample size for research activities. Educ Psychol Meas. (1970) 30:607–10.
52. Boazar M, Abdeshahi A, Yazdanpanah M. Changing rice cropping patterns among farmers as a preventive policy to protect water resources. J Environ Plann Manage. (2020) 1–17. doi: 10.1080/09640568.2020.1729705
53. Anderson JC, Gerbing DW. Structural equation modeling in practice: A review and recommended two-step approach. Psychol Bull. (1988) 103:411–23.
54. Hair JF, Black WC, Babin BJ, Anderson RE, Tatham RL. Multivariate Data Analysis. (2010). Prentice Hall, Upper Saddle River, NJ.
55. Fornell C, Larcker DF. Structural equation models with unobservable variables and measurement error: algebra and statistics. J Mark Res. (1981) 18:382–88.
56. Ayosanmi OS, Oden L, Ayosanmi T, Alli B, Wen M, Johnson J. The role of health belief model in HIV screening decision among international students in the United States: a pilot study. Int J Transl Med Res Public Health. (2020) 4:4–12. doi: 10.21106/ijtmrph.99
57. Jeihooni AK, Rakhshani T. The effect of educational intervention based on health belief model and social support on promoting skin cancer preventive behaviors in a sample of Iranian farmers. J Cancer Educ. (2019) 34:392–401. doi: 10.1007/s13187-017-1317-1
58. Luquis RR, Kensinger WS. Applying the Health Belief Model to assess prevention services among young adults. Int J Health Promotion Educ. (2019) 57:37–47. doi: 10.1080/14635240.2018.1549958
59. Bates BR, Villacís AG, Mendez-Trivino A, Mendoza LE, Grijalva MJ. Determinants of intentions to prevent triatomine infestation based on the health belief model: an application in rural southern Ecuador. PLoS Negl Trop Dis. (2020) 14:e0007987. doi: 10.1371/journal.pntd.0007987
60. Yazdanpanah M, Tajeri Moghadam M, Zobeidi T, Turetta A, Eufemia L, Sieber S. What factors contributed to convert towards organic farming? Considerate health belief model to uptake organic farming by Iranian farmers. J Environ Plann Manag. (2020) 1–23. doi: 10.1080/09640568.2021.1917348
Keywords: COVID-19, preventive behavior, rural youth, health belief model, adoption
Citation: Tajeri Moghadam M, Zobeidi T, Sieber S and Löhr K (2022) Investigating the Adoption of Precautionary Behaviors Among Young Rural Adults in South Iran During COVID-19. Front. Public Health 10:787929. doi: 10.3389/fpubh.2022.787929
Received: 01 October 2021; Accepted: 07 January 2022;
Published: 02 February 2022.
Edited by:
Masoud Yazdanpanah, Agricultural Sciences and Natural Resources University of Khuzestan, IranReviewed by:
Paschal Mugabe, University of Dar es Salaam, TanzaniaCopyright © 2022 Tajeri Moghadam, Zobeidi, Sieber and Löhr. This is an open-access article distributed under the terms of the Creative Commons Attribution License (CC BY). The use, distribution or reproduction in other forums is permitted, provided the original author(s) and the copyright owner(s) are credited and that the original publication in this journal is cited, in accordance with accepted academic practice. No use, distribution or reproduction is permitted which does not comply with these terms.
*Correspondence: Katharina Löhr, a2F0aGFyaW5hLmxvZWhyQHphbGYuZGU=
Disclaimer: All claims expressed in this article are solely those of the authors and do not necessarily represent those of their affiliated organizations, or those of the publisher, the editors and the reviewers. Any product that may be evaluated in this article or claim that may be made by its manufacturer is not guaranteed or endorsed by the publisher.
Research integrity at Frontiers
Learn more about the work of our research integrity team to safeguard the quality of each article we publish.