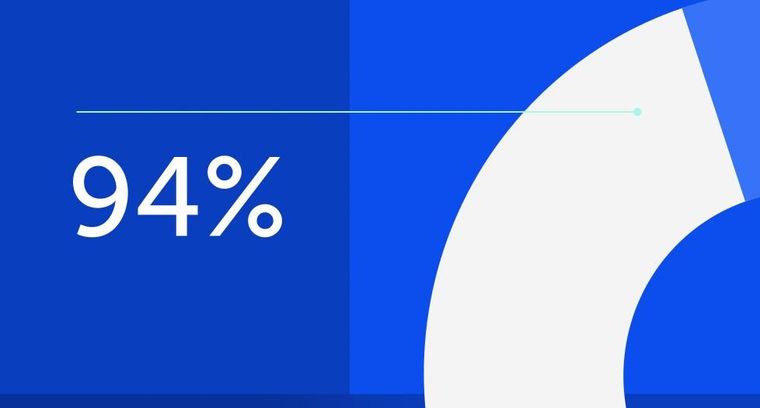
94% of researchers rate our articles as excellent or good
Learn more about the work of our research integrity team to safeguard the quality of each article we publish.
Find out more
ORIGINAL RESEARCH article
Front. Public Health, 17 March 2022
Sec. Environmental Health and Exposome
Volume 10 - 2022 | https://doi.org/10.3389/fpubh.2022.776850
This article is part of the Research TopicOne Health, Environmental Health, Global Health, and Inclusive Governance: What can we do?View all 12 articles
Objective: The purpose of this study is to empirically examine the impact of environmental information disclosure on the health of middle-aged and old residents and investigate whether such disclosure can improve the health of middle-aged and old residents.
Methods: This study matches the data of the Pollution Information Transparency Index (PITI) and China Health and Retirement Longitudinal Study in 2018 and uses the ordered logistic regression model to assess the impact of environmental information disclosure on the health of middle-aged and old residents. Furthermore, stepwise regression, ordinary least square, and ordered probit regression models are used for robustness tests. The IV-Ordered probit regression model solves the endogenous problem.
Results: Environmental information disclosure has a significant positive correlation with the health level of middle-aged and old residents. After the robustness test and endogenous problem handling, this conclusion still holds. Estimation results show that when PITI increases by 1 unit, the probability of improving the self-reported health level and actual health level of middle-aged and old residents increases by 1 and 0.87%, respectively. The impact of environmental information disclosure on the health of middle-aged and old residents also has significant regional heterogeneity. Specifically, the impact is mainly reflected in the central region of China.
Conclusion: Environmental information disclosure can improve the health of middle-aged and old residents. To improve the health of middle-aged and old residents, it is necessary to implement and enhance the environmental information disclosure system continuously. The anti-driving effect of environmental information disclosure on the treatment of environmental pollution must be intensified further, particularly focusing on the central region of China, where is more polluted and more concentrated than other regions.
Population aging is an important issue currently facing China (1–3). According to the results of China's seventh national census (4), as of November 2020, China has a population of 264,018,766 people aged 60 and over, accounting for 18.7% of the total population and far exceeding the 10% specified in internationally accepted aging standards. There are 190,635,280 people aged 65 and over, accounting for 13.50% of the total population and far more than the 7% stipulated in international standards. Furthermore, China's aging population is increasing. Compared with the sixth national census in 2010, the proportion of people aged 15–59 in the seventh national census in 2020 dropped by 6.79%, but the proportion of the population aged 60 and 65 years and above increased by 5.44 and 4.63%, respectively (5). In addition to the ever-increasing total number of middle-aged and old people, China's middle-aged and old residents are also plagued by chronic diseases (6, 7). According to official Chinese statistics (8), in 2018, the prevalence of chronic diseases among residents over 45 years old in China was 47.32%, while the prevalence of chronic diseases among residents aged 65 and over was as high as 62.33%. The high proportion of the middle-aged and old population and the high prevalence of chronic diseases, coupled with China's low-level doctor-patient ratio (9), have further aggravated medical pressure in the country and hindered its social and economic development (10, 11).
Numerous studies have confirmed the close relationship between the ecological environment and the health of residents (12–14), especially in terms of middle-aged and old residents (15–17). China's rapid economic development has significantly improved the living standards of such residents. However, China's economic growth has been characterized by high energy consumption, high pollution, and high emissions (18) since the reform and opening-up, which has brought various ecological and environmental issues such as smog pollution, which is well-known to the public (19), industrial pollution (20), water pollution (21), and offshore pollution (22). These environmental pollution problems have caused great harm to the health of residents. Kampa and Castanas (23) believes that air pollution has both acute and chronic effects on human health, affecting a significant number of different systems and organs, including heart disease, lung cancer, acute respiratory infections in children and chronic bronchitis in adults. Mannucci and Franchini (24) indicates that at least seven million deaths globally are attributable to the effects of air pollution annually. Ashbolt (25) and Schwarzenbach et al. (26) believe that drinking water is a major source of microbial pathogens in developing regions. Wang and Yang (27) studies the relationship between health and water pollution using the random-effects and random effects logit models. He demonstrates that the negative health effects of water pollution remain a major source of morbidity and mortality in China.
To control environmental pollution, the Chinese government has issued a large number of policies, including environmental information disclosure. Environmental information disclosure is an environmental governance policy based on information disclosure, which is regarded as a supplement to administrative and market means (28–30). Since the “Environmental Protection Law of the People's Republic of China” put forward the concept of environmental information disclosure in 1989, such disclosure in China has experienced more than 30 years of practice and development, and the related policy has been gradually improved through continuous adjustments and changes (31). If we advance the time to before the reform and opening up, China's environmental information disclosure system has even gone through about 70 years of construction and exploration, forming a circular circle from system formulation, implementation to amendment. Before the reform and opening up, environmental information in China was an implicit disclosure. After the 1990s, China's environmental information entered a formal disclosure phase. After entering the 21st century, environmental information began to be disclosed centrally and a series of supporting policies began to be released, including the “Measures for Disclosure of Environmental Information,” “Application for Disclosure of Environmental Information According to Law,” “Measures for Reporting Environmental Emergency Information,” and “Measures for Disclosure of Environmental Information by Enterprises and Institutions.” As a policy tool, environmental information disclosure has had a significant positive impact on improving China's ecological environment (32–35).
At present, scholars have studied the relationship between environmental pollution and the health of middle-aged and old residents as well as the relationship between environmental information disclosure and environmental pollution. However, they rarely combine the two to study the impact of environmental information disclosure on the health of middle-aged and old residents. In terms of macro policy, the Chinese government has also placed greater emphasis on environmental and health issues. In the 14th Five-Year Plan released in March 2021, it is proposed to comprehensively promote the construction of “Healthy China,” and set a goal to increase life expectancy per capita by 1 year during the 14th Five-Year Plan. At the 75th session of the United Nations General Assembly, Chinese President Xi Jinping directly announced China's “dual carbon” goal, which is to peak CO2 emissions by 2030 and work toward achieving carbon neutrality by 2060. In conclusion, it is of great theoretical and practical significance to study the relationship between environmental information disclosure and the health level of middle-aged and old residents.
This study matches the environmental information disclosure indicators at the urban level in China with the micro-survey data of middle-aged and old residents. It uses the ordered logistic regression model to study the impact of environmental information disclosure on the health of middle-aged and old residents. We find that environmental information disclosure can improve the health level of middle-aged and old residents. After the robustness test and endogenous problem handling, this conclusion still holds. We also find significant regional differences in the impact of environmental information disclosure on the health of middle-aged and old residents, and this impact is mainly reflected in the central region of China. The marginal contribution of this study is that we provide a new perspective for studying the impact of environmental policies on health, that is, environmental information disclosure system. From the perspective of environment and health, the implementation of the environmental information disclosure system is not only conducive to solving the problem of environmental pollution, but also has a positive impact on the improvement of residents' health. These results provide more evidence to government to development and implement evidence-based strategies and policies to improve the residents' health.
The remainder of this study is organized as follows. Section Materials and Methods introduces the materials and methods, including data sources, specification of variables and ordered logistic regression model. Section Results presents the results of the research, which used Stata, including tests of parallel regression assumption, benchmark regression, robustness test, endogenous problem handling, and regional differences. Section Discussion discusses the empirical results based on previous studies. Finally, Section Conclusion provides a summary of the results and puts forward some policy recommendations and the direction of further research.
The data source of this article includes two parts, with the most important one being the China Health and Retirement Longitudinal Study in 2018 (CHARLS-2018) hosted by the National School of Development of Beijing University. CHARLS is a longitudinal survey that aims to be representative of the residents in mainland China aged 45 and older, with no upper age limit. It attempts to set up a high-quality public micro-database that can provide a wide range of information from socioeconomic status to health conditions to serve scientific research needs or the elderly (36). Currently, CHARLS is widely used in the study of the health of middle-aged and old people in China (37–40). The national baseline survey was conducted in 2011, with wave 2 in 2013, wave 3 in 2015, and wave 4 in 2018, respectively. To ensure sample representativeness, the CHARLS baseline survey covered 28 provinces, 150 countries/districts, and 450 villages/urban communities across the country, reflecting the middle-aged and older Chinese population collectively. The sample of CHARLS-2018 involved ~19,000 individuals in 12,400 households. Data of CHARLS-2018 are available at http://charls.pku.edu.cn/.
Another source of data is the Pollution Information Transparency Index (PITI), which is co-developed by the Institute of Public and Environmental Affair (IPE) and The Natural Resources Defense Council. At present, many studies use PITI to represent the environmental information disclosure of the region (41–43). Matching with CHARLS-2018, this article selects the PITI of 2018, covering 29 provinces and 120 cities in China. Data and more information about PITI are available at http://www.ipe.org.cn/.
The explained variable of this article is the health level of middle-aged and old residents, which is divided into self-reported health level (self_HL) and actual health level (actual_HL). Self-reported health level is an individual's subjective judgment of their health. Using it to characterize health status can meet the needs of analyzing the relationship between environment and health to a large extent (44). Self-reported health level can be obtained through a question in the CHARLS-2018 questionnaire:
• What do you think of your health?
In the questionnaire, the answer to this question is divided into five levels: 1 = very good, 2 = good, 3 = fair, 4 = poor, and 5 = very poor. To be consistent with the direction of the explanatory variables (the larger the value, the better), we re-encoded the level of this question to 1 = very poor, 2 = poor, 3 = fair, 4 = good, and 5 = very good.
The actual health level needs to be determined through a series of tests. This article selects a series of physical functional limitations in the questionnaire to be investigated. These questions include:
• Do you have any difficulty with running or jogging about 1 km?
• Do you have difficulty with getting up from a chair after sitting for a long period?
• Do you have difficulty with climbing several flights of stairs without resting?
• Do you have difficulty with stooping, kneeling, or crouching?
• Do you have difficulty with reaching or extending your arms above shoulder level?
• Do you have difficulty with lifting or carrying weights over 5 kg, such as a heavy bag of groceries?
• Do you have difficulty with picking up a small coin from a table?
In the questionnaire, these seven questions adopt a four-level evaluation standard: 1 = No, I don't have any difficulty, 2 = I have difficulty but can still do it, 3 = Yes, I have difficulty and need help, and 4 = I can't do it. We add up the total scores of these seven questions and measure the actual health level of residents according to Table 1. The higher the total score, the lower the actual health level. As with the self-rated health level, we also coded these levels as follows: 1 = very poor, 2 = poor, 3 = fair, and 4 = good.
The explanatory variable of this article is the level of environmental information disclosure, measured by PITI. The full score of PITI is 100. The higher the score, the higher the level of environmental information disclosure in the region. PITI's accounting is divided into five dimensions: regulatory information, self-monitoring, interactive response, emissions data, and environmental impact assessment (EIA) information, including eight indicators. Table 2 shows the specific description and weight of each indicator.
To improve the accuracy of the model estimation results, we refer to some literature that studies the factors affecting the health of middle-aged and old residents. Based on the availability of data, we selected some factors as control variables and put them in the model. There are five dimensions of control variables: (1) Demographic characteristics, including gender (gender), age (age), education level (edu), and marriage status (marriage); (2) Living habits, including sleep status (sleep), whether the individual smokes (smoke), frequency of drinking (drinking), and whether physical activity is performed (physic); (3) Medical behaviors, including whether to participate in medical insurance (insurance) and medical and fitness expenditures (health_ep); (4) Health level in childhood (health_ch), that is, the health status before the age of 15; and (5) Urban or rural residents (urban). It is worth noting that unhealthy diet is also an important risk factor affecting the health level of middle-aged and old residents. However, there was no survey related to dietary habits in the CHARLS-2018 questionnaire; therefore, the control variables in the model do not include this factor. Table 3 shows the specific description and value meaning of all variables.
Table 4 shows the descriptive statistical results of all variables. The second column indicates the number of observations (Obs) for each variable. PITI matches approximately half of the CHARLS-2018 sample. The mean, standard deviation (SD), minimum (Min), and maximum (Max) can reflect the measures of dispersion of the sample. To test whether multicollinearity exists between variables, we calculate the variance inflation factor (VIF) of the explanatory variable and all control variables. According to commonly used judgment standards (19, 45), these VIFs are significantly <10, thereby indicating that the variables selected in this article do not have multicollinearity.
The explained variable in this paper is an ordinal variable, so it is not accurate enough to estimate with the traditional ordinary least square (OLS) regression model (46–48). The ordered logistic (Ologit) regression model is more suitable for dealing with such problems (49). It estimates the effects of a set of independent variables (numerical or categorical) on the logarithm of the probability that the dependent variable assumes low values rather than high values (50). The benchmark model constructed in this article is as follows:
where HLi is the health level of i, PITIi is the PITI of the city where i is located, Controli refers to all control variables, and εi is the random error term. Assuming that εi follows the logistic distribution, and the ordered explained variable has j distinct values, the relationship with theXk explanatory variables for j varying between 1 and J-1 can be expressed through the following formula:
where αj is the intercept and indicates the probability that the explained variable assumes low values rather than high values in case of nullity of all the explanatory variables. βk represents the log(ODDS) change corresponding to a unitary increase of the Xk variables. Positive values of the βk coefficients correspond to higher probabilities that the explained variable assumes high values, and vice versa (51).
Ologit regression model is performed under the assumption of cumulative logit parallelity (52). Therefore, we need to test the parallel regression assumption of the model first, that is, to test whether the influence of each value level of the explanatory variable on the explained variable is the same in each regression equation. Table 5 shows the results of five test methods. The data in the table indicates that whether self_HL or actual_HL is used as the explained variable, and the P-value of the parallel hypothesis test obtained by all methods is >0.1, indicating that all the probabilities show none significance. In particular, the parallel regression assumption holds, and we can use the Ologit regression model for research.
Table 6 shows the benchmark regression results of the model. Since the information reflected by the coefficients in the Ologit regression model is limited, we further calculate the odds ratio [odds ratio = exp(coefficient)] of each variable to make it easier to understand the regression results.
From the results in Table 6, we can conclude the following:
(1) Whether self_HL or actual_HL as the explained variable, the estimated coefficients of PITI are significantly positive, thereby indicating that environmental information disclosure has a significant positive correlation with the health level of middle-aged and old residents. When self_HL is used as the explained variable, the odds ratios of PITI is 1.0100, and when actual_HL is used as the explained variable, the odds ratios of PITI is 1.0087. These results indicate that when environmental information disclosure level increases by 1 unit, the probability of improving the self-reported health level and actual health level of middle-aged and old residents increases by 1 and 0.87%, respectively.
(2) In terms of the influence of the control variables on the explained variables, we choose a model with a better fitting effect for interpretation, that is, taking actual_HL as the explained variable. On the basis of the regression results, we can divide these control variables into three categories. The first type is that the regression coefficient is significantly positive, including edu, marriage, sleep, physic, health_ch and urban. Combined with Table 3, the outcome reveals that middle-aged and old residents with higher education and adequate sleep have better health conditions. Physical activity also has a significant positive impact on the health of middle-aged and old residents. Compared with rural areas, urban middle-aged and old residents have a higher level of health. The second type of control variable is that the regression coefficient is significantly negative, including gender, age, smoke, drinking, and health_ep. These results indicate that among middle-aged and old residents, the health of females is worse than that of males. In addition, the older the age, the higher the expenditure on medical and fitness, and the middle-aged and old residents who have smoking and drinking habits have worse health. The third type is the control variable with an insignificant regression coefficient, which is only insurance. The reason may be that this control variable in the sample does not have a significant binary classification. According to statistics, the medical insurance participation rate of Chinese residents in 2018 exceeded 95%.
The process of stepwise regression is to first regress the explanatory variable to the explained variable separately and then gradually add the control variables and observe whether the sign and significance of the coefficient of the explanatory variable have changed to judge the robustness of the result. Tables 7, 8, respectively, show the stepwise regression results with self_HL and actual_HL as the explained variables. Among them, Column 1 presents the regression result without adding any control variables, and Columns 2–6 show the regression results after gradually adding the control variables. Owing to space limitations, we only list the coefficients, robust standard errors, and odds ratio of the explained variables in the text. The results in Tables 7, 8 show that whether self_HL or actual_HL is used as the explained variable, in all cases, the coefficient of the core explanatory variable PITI is significantly positive at the 1% statistical level, and the odds ratio is basically maintained at about 1%.
Table 9 reports the estimated results using OLS and ordered probit regression models. We can find that after changing the estimation method, the regression coefficients of the core explanatory variable PITI to the explained variables self_HL and actual_HL are still significantly positive. This result confirms the robustness of the conclusions drawn in this article once more.
There may be a reciprocal causation relationship between environmental information disclosure and the health of middle-aged and old residents. On the one hand, environmental information disclosure has an impact on the health of middle-aged and old residents, which is the causal relationship that this article focuses on. On the other hand, the poor health of middle-aged and old residents may force companies and governments to strengthen environmental information disclosure to promote environmental improvement. In addition, although we have added a large number of control variables to the model to minimize the impact of missing variables on the model estimation results, due to the limitations of the survey data and the complexity of the factors affecting the health of middle-aged and old residents, there may still be the problem of missing variables. All these will lead to endogenous problems in the model.
To solve the endogenous problem of the model, we choose the IV-Ordered probit regression model. With reference to Zhao (53), we select the lag period of PITI as an instrumental variable, including one lag period (PITIt−1) and three lag periods (PITIt−3). On the one hand, the level of public environmental information in cities generally has “inertial” characteristics, so the PITI in the lag period is significantly related to the PITI in the current period. On the other hand, the PITI in the lag period is difficult to directly impact the health of the current middle-aged and old residents.
Table 10 reports the regression results after dealing with the endogenous problem. From this table, we find that the Wald test of the two instrumental variables passed the 1% significance test, thereby rejecting the null hypothesis of weak instrumental variables. After the instrumental variables are added, the estimated coefficients of the core explanatory variable PITI for the explained variables self_HL and actual_HL are still significantly positive, reconfirming the conclusion that there is a significant positive relationship between environmental information disclosure and the health of middle-aged and old residents.
To study whether there are regional differences in the impact of environmental information disclosure on the health of middle-aged and old residents, we divided all samples into three categories: eastern, central, and western. The eastern region includes Beijing, Tianjin, Hebei, Liaoning, Shanghai, Jiangsu, Zhejiang, Fujian, Shandong, Guangdong and Hainan. The central region includes Heilongjiang, Jilin, Shanxi, Anhui, Jiangxi, Henan, Hubei and Hunan. The western region includes Guizhou, Yunnan, Shaanxi, Inner Mongolia, Guangxi, Chongqing, Sichuan, Qinghai, Gansu, Ningxia and Xinjiang.
Table 11 shows the Ologit regression results of different regions. We select the explained variable actual_HL with better fitting effect. The results in Table 11 show that similar to the estimated results of the total sample, the estimated coefficients of PITI in different regions are all positive. However, only the estimated coefficients in the central region are significant, and the estimated coefficients in the eastern and western regions have not passed the significance test. This outcome shows that the impact of environmental information disclosure on the health of middle-aged and old residents is mainly manifested in the central region of China.
(1) Forcing local governments to strengthen environmental management and improve the ecological environment is one of the roles of environmental information disclosure, which is conducive to improving the health of middle-aged and old residents. For a long time, China has been regarded as “information-poor”, and the country has strong control over economic development and information dissemination (54, 55). In the past 10 years, China's control of information and information processes has undergone major changes, especially in the field of environmental governance (56). The central government allows or even actively promotes the disclosure of environmental information and media reports to fight against powerful local polluters—usually some high-polluting manufacturing companies (34). However, local authorities generally do not support this environmental transparency because manufacturing companies are often an important source of tax revenue for local governments (57). With the openness of environmental information and the freedom of more media to report environmental disasters and protests, it is possible to resist the distortion of environmental information by local and regional authorities and force local governments to conduct environmental governance. The central government is likewise paying more and more attention to ecological and environmental issues in assessing the work and promotion of local officials, and the level of local environmental information disclosure is one of them (58). In the international community, The Chinese government has been calling on all countries to pursue innovative, coordinated, green and open development for all. The Chinese government has also been making its own contribution to controlling carbon emissions and addressing global climate change. Therefore, in the face of inspections and assessments from the central government and public supervision, local governments also form an accountability mechanism for environmental governance similar to the reverse effect (59). By increasing the transparency of environmental information, the central government, the general public, and the news media can strengthen the multiple accountabilities of local governments, thereby forcing them to strengthen environmental governance.
(2) Promoting companies to reduce pollution emissions and improve the ecological environment is another role of environmental information disclosure, which is also conducive to improving the health of middle-aged and old residents. Such disclosure can increase the environmental awareness of corporate management and stimulate changes in the production process (60). According to the theory of organizational legitimacy (61), pollutant companies are subject to pressure from regulatory agencies and public opinion when facing environmental information disclosure. If corporate behavior deviates from social values, then corporate legitimacy will be threatened and public policies that are unfavorable to corporate development will be incurred. To avoid these situations, companies will further strengthen technological upgrading and information disclosure, bear their due economic and social costs, reduce pollutant emissions, and improve their environmental efficiency. In addition to regulatory pressures, the need to improve financial performance also forces companies to take environmental protection actions (62). Environmental information disclosure can reduce information asymmetry between companies and investors, increase investors' trust in companies, and build social confidence (63, 64). It can also improve the company's green reputation (65). A good reputation can improve the company's relationship with stakeholders and attract more consumers (66), which will positively impact its financial performance.
(3) Environmental information disclosure is also an important driver of health behavior and decision-making changes among middle-aged and older residents. The first is by avoiding health risks. Passively perceiving changes in environmental pollution and strengthening environmental information disclosure can play certain roles in early warning. Since the physical health of middle-aged and old residents is more susceptible to threats than that of young people, as a rational individual, the former will quickly take measures against environmental pollution. For example, timely disclosure of air pollution information can enable middle-aged and old residents to reasonably plan outdoor activities based on environmental pollution state. Smog alarms issued through media channels, such as television, radio, and newspapers, can significantly reduce their daily attendance and outdoor activities (67, 68). The second is by actively participating in environmental protection. Public participation in environmental governance must be based on a certain amount of environmental information; however, the public will pay a lot of private costs, such as time and money, to collect relevant environmental information (69). Environmental information disclosure can allow middle-aged and old residents to understand the surrounding environmental conditions and existing problems, point out the direction for participating in environmental governance, and reduce the difficulty of their participation in environmental governance. Such disclosure can improve the scientific understanding of environmental issues and ecological protection awareness of middle-aged and old residents (70). At the same time, it helps them increase their trust in the government, thereby increasing their enthusiasm to participate in environmental governance. Furthermore, in the field of public health, non-government actors such as private sectors, social society, philanthropy, academia play their role to improve the health of all. The implementation of the environmental information disclosure system also helps to enhance their motivation and convenience to participate in environmental governance. The third is by rising health care expenditures. A study based on data from Indian households shows that households who are told their drinking water may be contaminated will increase their spending on water purification (71). The environmental information disclosure system can also bring the same effect. It can inform middle-aged and old residents whether the surrounding environmental pollution is severe. When environmental pollution is serious, middle-aged and old residents increase their health care expenditures to protect their health, such as purchasing protective equipment, sports equipment, and health care drugs.
(4) The impact of environmental information disclosure on the health of middle-aged and old residents also has significant regional heterogeneity. Specifically, the impact is mainly reflected in the central region of China. The reason for this result may be derived from the high pollution in central China. Compared with the eastern and western regions, the central region of China has a large labor force and low labor costs. Meanwhile, the cost of land in the central region is lower, and there are more relaxed environmental regulations. Enterprises with high pollution are more willing to build factories in the central region. Therefore, the central region has become the most concentrated area of environmental pollution in China and is facing more severe environmental pollution challenges. Official statistics from the Chinese government show that in terms of waste water, waste gas and industrial solid waste, the central region has significantly higher emissions than the eastern and western regions, with Shanxi and Anhui provinces experiencing particularly severe pollution. Enhancing the disclosure of environmental information can restrain the pollutant discharge behavior of polluting enterprises and promote the process of environmental pollution control in the central region. Therefore, the health level of middle-aged and old residents in the central region is more prominently affected by environmental information disclosure.
Based on the data of PITI and CHARLS in China, this study uses the Ologit regression model to explore the impact of environmental information disclosure on the health of middle-aged and old residents. Results show that environmental information disclosure can improve the health of middle-aged and old residents. After the robustness test and endogenous problem handling, this conclusion still holds. Estimation results of the Ologit regression model show that when PITI increases by 1 unit, the probability of improving the self-reported health level and actual health level of middle-aged and old residents increases by 1 and 0.87%, respectively. In addition, due to different levels of environmental pollution, the impact of environmental information disclosure on the health of middle-aged and old residents has significant regional heterogeneity. Specifically, the impact is mainly reflected in the central region of China.
In order to improve the health level of middle-aged and old residents, we suggest that the Chinese government should further improve the environmental information disclosure system, and take the reception rate and satisfaction rate of residents as important indicators to evaluate the implementation effect of the environmental information disclosure system. Meanwhile, the government should timely adjust the content and form of environmental information disclosure according to the needs of residents. In addition, the government also needs to formulate different environmental information disclosure goals according to the actual characteristics of environmental pollution in different regions.
According to the classification of the three actors of government, enterprises, and individuals, we discuss the specific mechanism of this effect and present in three paths: forcing local governments to govern the environment, promoting enterprises to reduce pollution, and influencing individuals to make behavioral decisions. However, we did not test these mechanisms with mathematical models, which is also the specific direction for our future research.
Publicly available datasets were analyzed in this study. This data can be found here: Data of CHARLS-2018 are available at http://charls.pku.edu.cn/, and data of PITI are available at http://www.ipe.org.cn/.
XY and WS: conceptualization and data collection. XY: analyzed data and writing—original draft preparation. XY and SL: writing—review and editing. All authors contributed to the article and approved the submitted version.
This research was funded by the Natural Science Foundation of Zhejiang (No. LQ22G030002), the National Social Science Fund of China, (No. 19AZD004), the National Natural Science Foundation of China (No. 71874092), and K.C. Wong Magna Fund in Ningbo University.
The authors declare that the research was conducted in the absence of any commercial or financial relationships that could be construed as a potential conflict of interest.
All claims expressed in this article are solely those of the authors and do not necessarily represent those of their affiliated organizations, or those of the publisher, the editors and the reviewers. Any product that may be evaluated in this article, or claim that may be made by its manufacturer, is not guaranteed or endorsed by the publisher.
1. Li M. The impact of China's aging population. SERI Q. (2011) 4:25–33. Available online at: https://www.proquest.com/docview/898962342?pq-origsite=gscholar&fromopenview=true
2. Dong B, Ding Q. Aging in China: a challenge or an opportunity? J Am Med Dir Assoc. (2009) 10:456–58. doi: 10.1016/j.jamda.2009.06.007
3. Guangzhou W, Jun W. Economic and social impact of China's aging population and public policy response. China Econ. (2021) 16:78–107. doi: 10.19602/j.chinaeconomist.2021.01.05
4. Zheng Z. From the past to the future: what we learn from China's 2020 Census. China Popul Dev Stud. (2021) 5:101–106. doi: 10.1007/s42379-021-00084-8
5. National Bureau of Statistics. Bulletin of the Seventh National Census. (2021). Available online at: http://www.stats.gov.cn/tjsj/ (accessed August 20, 2021).
6. Liu Q, Cai H, Yang LH, Xiang Y, Yang G, Li H, et al. Depressive symptoms and their association with social determinants and chronic diseases in middle-aged and elderly Chinese people. Sci Rep. (2018) 8:1–11. doi: 10.1038/s41598-018-22175-2
7. Yao S, Cao G, Han L, Chen Z, Huang Z, Gong P, et al. Prevalence and patterns of multimorbidity in a nationally representative sample of older Chinese: results from the China Health and Retirement Longitudinal Study. J Gerontol Ser A. (2020) 75:1974–80. doi: 10.1093/gerona/glz185
9. Zhang P, Wang F, Cheng Y, Zhang LY, Ye BZ, Jiang HW, et al. Impact of organizational and individual factors on patient-provider relationships: a national survey of doctors, nurses and patients in China. PLoS ONE. (2017) 12:e0181396. doi: 10.1371/journal.pone.0181396
10. Bai C, Lei X. New trends in population aging and challenges for China's sustainable development. China Econ J. (2020) 13:3–23. doi: 10.1080/17538963.2019.1700608
11. Banister J, Bloom DE, Rosenberg L. Population Aging and Economic Growth in China. The Chinese Economy. London: Springer (2012). p. 114–49.
12. Pope Iii CA, Burnett RT, Thun MJ, Calle EE, Krewski D, Ito K, et al. Lung cancer, cardiopulmonary mortality, and long-term exposure to fine particulate air pollution. JAMA. (2002) 287:1132–41. doi: 10.1001/jama.287.9.1132
13. Briggs D. Environmental pollution and the global burden of disease. Br Med Bull. (2003) 68:1–24. doi: 10.1093/bmb/ldg019
14. Joumard I, André C, Nicq C, Chatal O. Health status determinants: lifestyle, environment, health care resources and efficiency. In: Environment, Health Care Resources and Efficiency (May 27, 2010) OECD Economics Department Working Paper, Paris. (2010).
15. Liu S, Yan Z, Liu Y, Yin Q, Kuang L. Association between air pollution and chronic diseases among the elderly in China. Nat Hazards. (2017) 89:79–91. doi: 10.1007/s11069-017-2955-7
16. Fang L, Lu N. Can the middle-aged and the elderly achieve their health improvement from domestic energy consumption structure optimization in rural China. Chin J Popul Resour Environ. (2019) 29:40–9. doi: 10.12062/cpre.20190126
17. Yuan K-S, Wu T-J. Environmental stressors and well-being on middle-aged and elderly people: the mediating role of outdoor leisure behaviour and place attachment. Environ Sci Pollut Res. (2021) 1:1–10. doi: 10.1007/s11356-021-13244-7
18. Ding L, Liu C, Chen K, Huang Y, Diao B. Atmospheric pollution reduction effect and regional predicament: an empirical analysis based on the Chinese provincial NOx emissions. J Environ Manage. (2017) 196:178–87. doi: 10.1016/j.jenvman.2017.03.016
19. Yu X, Shen M, Shen W, Zhang X. Effects of land urbanization on smog pollution in China: estimation of spatial autoregressive panel data models. Land. (2020) 9:337. doi: 10.3390/land9090337
20. Cheng J, Dai S, Ye X. Spatiotemporal heterogeneity of industrial pollution in China. China Econ Rev. (2016) 40:179–91. doi: 10.1016/j.chieco.2016.07.001
21. Wu C, Maurer C, Wang Y, Xue S, Davis DL. Water pollution and human health in China. Environ Health Perspect. (1999) 107:251–56. doi: 10.1289/ehp.99107251
22. Wang Z, Zhao L, Wang Y. An empirical correlation mechanism of economic growth and marine pollution: a case study of 11 coastal provinces and cities in China. Ocean Coast Manag. (2020) 198:105380. doi: 10.1016/j.ocecoaman.2020.105380
23. Kampa M, Castanas E. Human health effects of air pollution. Environ Pollut. (2008) 151:362–67. doi: 10.1016/j.envpol.2007.06.012
24. Mannucci PM, Franchini M. Health effects of ambient air pollution in developing countries. Int J Environ Res Public Health. (2017) 14:1048. doi: 10.3390/ijerph14091048
25. Ashbolt NJ. Microbial contamination of drinking water and disease outcomes in developing regions. Toxicology. (2004) 198:229–38. doi: 10.1016/j.tox.2004.01.030
26. Schwarzenbach RP, Egli T, Hofstetter TB, Von Gunten U, Wehrli B. Global water pollution and human health. Annu Rev Environ Resour. (2010) 35:109–36. doi: 10.1146/annurev-environ-100809-125342
27. Wang Q, Yang Z. Industrial water pollution, water environment treatment, and health risks in China. Environ Pollut. (2016) 218:358–65. doi: 10.1016/j.envpol.2016.07.011
28. Stephan M. Environmental information disclosure programs: they work, but why? Soc Sci Q. (2002) 83:190–205. doi: 10.1111/1540-6237.00078
29. Esty DC. Environmental protection in the information age. NY Univ Law Rev. (2004) 79:115. doi: 10.2139/ssrn.429580
30. Mol AP. Environmental governance in the Information Age: the emergence of informational governance. Environ Plann C Govern Policy. (2006) 24:497–514. doi: 10.1068/c0508j
31. Sun Y, Liu H, Li P. Policy change of China's environmental information disclosure: path and logical explanation. China Popul Resour Environ. (2018) 28:168–76. doi: 10.12062/cpre.20170704
32. Huang R, Chen D. Does environmental information disclosure benefit waste discharge reduction? Evid China J Bus Ethics. (2015) 129:535–52. doi: 10.1007/s10551-014-2173-0
33. Yu Y, Huang J, Luo N. Can more environmental information disclosure lead to higher eco-efficiency? Evid China Sustain. (2018) 10:528. doi: 10.3390/su10020528
34. Zhang L, Mol AP, He G. Transparency and information disclosure in China's environmental governance. Curr Opin Environ Sustain. (2016) 18:17–24. doi: 10.1016/j.cosust.2015.03.009
35. Zhong S, Li J, Zhao R. Does environmental information disclosure promote sulfur dioxide (SO2) remove? New evidence from 113 cities in China. J Clean Prod. (2021) 299:126906. doi: 10.1016/j.jclepro.2021.126906
36. Zhao Y, Hu Y, Smith JP, Strauss J, Yang G. Cohort profile: the China health and retirement longitudinal study (CHARLS). Int J Epidemiol. (2014) 43:61–8. doi: 10.1093/ije/dys203
37. Tang S, Xu Y, Li Z, Yang T, Qian D. Does economic support have an impact on the health status of elderly patients with chronic diseases in China?Based on CHARLS (2018) data research. Front Public Health. (2021) 9:336. doi: 10.3389/fpubh.2021.658830
38. Pan G, Li S, Geng Z, Zhan K. Do social pension schemes promote the mental health of rural middle-aged and old residents? Evidence from China. Front Public Health. (2021) 9:128. doi: 10.3389/fpubh.2021.710128
39. Yang L, Peng W. Prevalence and factors associated with body pain: results of a nationally representative survey of 9,586 Chinese adults aged 60 and over. Front Public Health. (2021) 9:634123. doi: 10.3389/fpubh.2021.634123
40. Strauss J, Lei X, Park A, Shen Y, Smith JP, Yang Z, et al. Health outcomes and socio-economic status among the elderly in China: evidence from the CHARLS Pilot. J Popul Ageing. (2010) 3:111–42. doi: 10.1007/s12062-011-9033-9
41. Pien C-P. Local environmental information disclosure and environmental non-governmental organizations in Chinese prefecture-level cities. J Environ Manag. (2020) 275:111225. doi: 10.1016/j.jenvman.2020.111225
42. Pan D, Fan W. Benefits of environmental information disclosure in managing water pollution: evidence from a quasi-natural experiment in China. Environ Sci Pollut Res. (2021) 28:14764–781. doi: 10.1007/s11356-020-11659-2
43. Feng Y, Wang X, Liang Z. How does environmental information disclosure affect economic development and haze pollution in Chinese cities? The mediating role of green technology innovation. Sci Total Environ. (2021) 775:145811. doi: 10.1016/j.scitotenv.2021.145811
44. Qi Y, Lu H. Pollution, health and inequality: Overcoming the trap of “environmental health poverty”. Manage World. (2015) 9:32–51. doi: 10.19744/j.cnki.11-1235/f.2015.09.004
45. Pan Y, Jackson RT. Insights into the ethnic differences in serum ferritin between black and white US adult men. Am J Hum Biol. (2008) 20:406–16. doi: 10.1002/ajhb.20745
46. Winship C, Mare RD. Regression models with ordinal variables. Am Sociol Rev. (1984) 512–25. doi: 10.2307/2095465
47. Calvin JA. Regression models for categorical and limited dependent variables. Technometrics. (1998) 40:80–81. doi: 10.1080/00401706.1998.10485496
48. McCullagh P. Regression models for ordinal data. J R Stat Soc Ser B. (1980) 42:109–27. doi: 10.1111/j.2517-6161.1980.tb01109.x
49. Das S, Rahman RM. Application of ordinal logistic regression analysis in determining risk factors of child malnutrition in Bangladesh. Nutr J. (2011) 10:1–11. doi: 10.1186/1475-2891-10-124
50. Cainarca GC, Sgobbi F. Educational mismatch e skill mismatch: un'indagine empirica sui lavoratori italiani. Quaderni Economia Lavoro. (2005) 1:83–84. Available online at: https://www.researchgate.net/publication/228379451_Educational_mismatch_e_skill_mismatch_un%27indagine_empirica_sui_lavoratori_italiani.
51. Eboli L, Mazzulla G. An ordinal logistic regression model for analysing airport passenger satisfaction. EuroMed J Bus. (2009) 4:40–57. doi: 10.1108/14502190910956684
52. Erkan A, Yildiz Z. Parallel lines assumption in ordinal logistic regression and analysis approaches. Int Interdiscip J Sci Res. (2014) 1:8–23. Available online at: https://www.researchgate.net/publication/311903359_PARALEL_LINES_ASSUMPTION_IN_ORDINAL_LOGISIC_REGRESSION_AND_ANALYSES_APPROACHES
53. Zhao W. The effect of air pollution on personal health care expenditure of elderly people: evidence from CHARLS data. Popul Econ. (2020) 238:75–88. doi: 10.3969/j.issn.1000-4149.2020.00.005
54. Wang H, Greer L, Lin X. Practice and inspiration of environmental information disclosure. World Environ. (2008) 5:24–6.
55. Mol AP. Environmental governance through information: China and Vietnam. Singap J Trop Geogr. (2009) 30:114–29. doi: 10.1111/j.1467-9493.2008.00358.x
57. Chen Z, He Y, Liu Z, Serrato JCS, Xu DY. The Structure of Business Taxation in China. Tax Policy Econ. (2021) 35:131–77. doi: 10.1086/713495
58. Zhou M, Deng LY editors. Officials' turnover, promotion incentives and environmental quality: Evidence from provincial government leaders in China. In: Proceedings of the 4th Annual International Conference on Social Science and Contemporary Humanity Development (Wuhan: SSCHD 2018) (2019).
59. Carter NT, Mol AP. China and the environment: domestic and transnational dynamics of a future hegemon. Env Polit. (2006) 15:330–44. doi: 10.1080/09644010600562294
60. Lee E. Information disclosure and environmental regulation: green lights and gray areas. Regul Govern. (2010) 4:303–28. doi: 10.1111/j.1748-5991.2010.01087.x
61. Dowling J, Pfeffer J. Organizational legitimacy: social values and organizational behavior. Pac Sociol Rev. (1975) 18:122–36. doi: 10.2307/1388226
62. Fan L, Yang K, Liu L. New media environment, environmental information disclosure and firm valuation: evidence from high-polluting enterprises in China. J Clean Prod. (2020) 277:123253. doi: 10.1016/j.jclepro.2020.123253
63. Clarkson PM Li Y, Richardson GD, Vasvari FP. Revisiting the relation between environmental performance and environmental disclosure: an empirical analysis. Account Org Soc. (2008) 33:303–27. doi: 10.1016/j.aos.2007.05.003
64. Wang P, Wang F, Hu N. The effect of ultimate ownership on the disclosure of environmental information. Aust Account Rev. (2018) 28:186–98. doi: 10.1111/auar.12166
65. Branco MC, Rodrigues LL. Corporate social responsibility and resource-based perspectives. J Bus Ethics. (2006) 69:111–32. doi: 10.1007/s10551-006-9071-z
66. Öberseder M, Schlegelmilch BB. Gruber V. “Why don't consumers care about CSR?”: A qualitative study exploring the role of CSR in consumption decisions. J Bus Ethics. (2011) 104:449–60. doi: 10.1007/s10551-011-0925-7
67. Graff Zivin J, Neidell M. The impact of pollution on worker productivity. Am Econ Rev. (2012) 102:3652–673. doi: 10.1257/aer.102.7.3652
68. Zivin JG, Neidell M. Days of haze: Environmental information disclosure and intertemporal avoidance behavior. J Environ Econ Manage. (2009) 58:119–28. doi: 10.1016/j.jeem.2009.03.001
69. Ané DP. Public participation, good environmental governance and fulfilment of environmental rights. Potchefstroom Electron Law J. (2008) 11:1–34. doi: 10.4314/pelj.v11i2.42232
70. Tu Z, Hu T, Shen R. Evaluating public participation impact on environmental protection and ecological efficiency in China: Evidence from PITI disclosure. China Econ Rev. (2019) 55:111–23. doi: 10.1016/j.chieco.2019.03.010
Keywords: environmental information disclosure, health level, middle-aged and old residents, impact analysis, empirical research
Citation: Yu X, Shen W and Lin S (2022) Does Environmental Information Disclosure Improve the Health Level of Middle-Aged and Old Residents? Evidence From China. Front. Public Health 10:776850. doi: 10.3389/fpubh.2022.776850
Received: 14 September 2021; Accepted: 21 February 2022;
Published: 17 March 2022.
Edited by:
Ulrich Laaser, Bielefeld University, GermanyReviewed by:
Jing Han, Jinan Municipal Center for Disease Control and Prevention, ChinaCopyright © 2022 Yu, Shen and Lin. This is an open-access article distributed under the terms of the Creative Commons Attribution License (CC BY). The use, distribution or reproduction in other forums is permitted, provided the original author(s) and the copyright owner(s) are credited and that the original publication in this journal is cited, in accordance with accepted academic practice. No use, distribution or reproduction is permitted which does not comply with these terms.
*Correspondence: Xuan Yu, eXV4dWFuQG5idS5lZHUuY24=
Disclaimer: All claims expressed in this article are solely those of the authors and do not necessarily represent those of their affiliated organizations, or those of the publisher, the editors and the reviewers. Any product that may be evaluated in this article or claim that may be made by its manufacturer is not guaranteed or endorsed by the publisher.
Research integrity at Frontiers
Learn more about the work of our research integrity team to safeguard the quality of each article we publish.