- 1Department of Urology, National Clinical Research Center for Geriatrics, Institute of Urology, West China Hospital, Sichuan University, Chengdu, China
- 2National Clinical Research Center of Geriatrics, The Center of Gerontology and Geriatrics, West China Hospital, Sichuan University, Chengdu, China
- 3Institute of Oncology Research (IOR) and Oncology Institute of Southern Switzerland (IOSI), Bellinzona, Switzerland
Background: Previous studies have suggested that air pollution affects physiological and psychological health. Using solid fuel at home is a significant source of indoor air pollution. The associations between solid fuel use and depressive symptoms and cognitive health were unclear among older adults from low- and middle-income countries (LMICs).
Methods: To evaluate the association of solid fuel use with depressive symptoms and cognitive health among older adults, we obtained data from the Longitudinal Aging Study in India (LASI) and excluded subjects younger than 60 years and without critical data (solid fuel use, depressive symptoms, and cognitive health). The 10-item Center for Epidemiologic Studies Depression Scale (CES-D-10) was used to assess depressive symptoms, with more than ten indicative of depression. Cognitive health was assessed using measures from the Health and Retirement Study (HRS), and subjects with the lowest 10th percentile were considered to have cognitive impairment. The participants' responses defined solid fuel use. Multivariable logistic regression, linear regression, subgroup analysis, and interaction tests were performed to appraise the relationship between solid fuel use and depression and cognitive impairment.
Results: A total of 29,789 participants over 60 years old were involved in this study. Almost half of the participants (47.5%) reported using solid fuel for home cooking. Compared with clean fuel use, solid fuel use was related to an increased prevalence of depression [odds ratio (OR) 1.09, 95% CI 1.03–1.16] and higher CES-D-10 scores (β 0.23, 95% CI 0.12–0.35) after fully adjusted covariables. Using solid fuel was also related to a higher risk of cognitive impairment (OR 1.21, 95% CI 1.11–1.32) and a lower cognitive score (β −0.63, 95% CI −0.79 to −0.47) compared with those who used clean fuel. In the subgroup analysis, the prevalence of depression increased in females and non-smokers. The association of solid fuel use with depression and cognitive impairment exists in subgroups of BMI, economic status, caste, living area, education, and drinking.
Conclusions: The use of solid fuel at home was associated with an increased prevalence of depression and cognitive impairment among older adults in India.
Introduction
Older adults have received extensive attention for the decline in physical function and lifestyle changes in recent decades. Older adults suffer a higher risk of chronic diseases due to the influence of long-term unhealthy lifestyles and dysfunction (1, 2). As people get older, they are more likely to suffer from cognitive impairment owing to cerebral atrophy, which harms their quality of life (3–5). Loneliness, functional disabilities, and chronic diseases are commonly significant risk factors for depression in older adults (6–8). Depression is the most common mental disorder in older adults, and the prevalence is higher in older adults than in young and middle-aged adults (8–10). The economic costs of depression and cognitive decline in the elderly are substantial, and they will rise as the severity of their symptoms worsens due to the higher costs associated with treating their illness (11, 12). Therefore, it is vital to prevent depression and cognitive impairment. Social and environmental factors and lifestyle are also positive indicators of depression (13, 14). As reported previously, air pollution is associated with depression and cognitive impairment with dose response (15, 16).
Over the years, air pollution has been recognized as a significant threat to public health, especially in low- and middle-income countries (LMICs) (17). Previous studies have shed light on the associations between air pollution and chronic disease, as well as the fact that air pollution increases the risk of unsatisfactory healthy status (18–20). Similarly, there is more evidence that air pollution is related to the prevalence of mental disorders and is correlated with the aggravation of depressive symptoms and cognitive impairment (21, 22). Household solid fuel produces particulate matter (PM), polycyclic aromatic hydrocarbons (PAHs), nitrogen dioxide (NO2), and carbon monoxide (CO), which are primary sources of indoor pollution and can have detrimental ramifications (23–26). Evidence from previous studies has suggested that oxide nanoparticles enter the central nervous system through the alveolar epithelium; PMs reduce the cognitive learning abilities of rats (27, 28). It is worth noting that indoor air pollution was linked to depressive symptoms and cognitive impairment among elderly adults (29, 30). It is unclear whether indoor pollution is simultaneously related to depression and cognitive impairment.
In India, with the largest elderly population in the world, there will be 316 million people aged 60 years and above in 2050 (31). Approximately 9 and 13.5% of older adults live with depression and cognitive impairment in India (32, 33). More homes continue to use solid fuels because of their low cost and the lack of predictability in their revenue; only 22.5% of homes use clean fuels, and only 10% of rural homes use clean fuels (34, 35). Hence, it is essential to determine how indoor solid fuel use affects human health. Due to the widespread use of solid fuel in India, it makes sense to focus on the health outcomes of solid fuel use based on its population. A study from India suggested that solid fuel use for cooking was a risk factor for depression among premenopausal females (36). However, solid fuels have an opaque association with depression and cognitive impairment in older Indian adults. Using data from the Longitudinal Aging Study in India (LASI), the present study aimed to evaluate the association between the use of solid fuels with depression and cognitive impairment.
Methods
Participants and design of the study
We performed a cross-sectional study to evaluate the association between solid fuel use with depression and cognitive impairment. Data for the present study came from the Longitudinal Aging Study in India (LASI). Health, economic, and social data from 72,250 adults aged 45 and up across all Indian states and union territories, with the exception of Sikkim, were collected over the course of 2 years (2017 and 2018) (37). LASI aimed to provide data regarding demography, financial status, self-reported health information, family, etc. In this study, we used the data from the first wave of the LASI. Variables of household fuel information and score of the 10-item Center for Epidemiologic Studies Depression Scale (CES-D-10) and the cognitive score of method from the Health and Retirement Study (HRS) were analyzed in the present study. We excluded those under 60 years old, those who lost data on household fuel details and CES-D-10 scores, and those without information on their cognition. Finally, 29,789 participants were included in this study. The inclusion and exclusion criteria of the study population are shown in Figure 1.
Definition of solid fuel use
In the LASI study, subjects were required to answer the type of cooking fuel. Participants who reported using clean fuels such as liquefied petroleum gas, biogas, or electricity were classified as such, whereas those who reported using other fuels were classified as solid fuel users.
Assessment of depression
The LASI study used the 10-item Center for Epidemiologic Studies Depression Scale (CES-D-10) to evaluate depressive symptoms, a short version of the CES-D-20, which is widely used for screening depression. It assesses the depressive symptoms of subjects with ten items about depressive feelings and behaviors in the past week, including three items, five items, and two items about the depressive, somatic, and positive effects, respectively (38). The score range of each item is 0–3 points, corresponding to “ <1 day,” “1–2 days,” “3–4 days,” and “5–7 days.” They were measured on a Likert scale. The scores of each item are summed, and the total score of the CES-D-10 ranges from 0 to 30. The CES-D-10 has good reliability and validity in older adults (39). According to a previous study, a CES-D-10 score of more than 10 was defined as a symptom of depression (40).
Evaluation of cognition
The methods of evaluating cognition from the HRS were used in the present study, which includes several aspects of cognition, such as orientation, function, arithmetic, object naming, and word recall. In the LASI study, subjects were asked to perform word repetition, the orientation of time and place, backward counting, serial computation, implementation of paper folding and pentagon drawing, and object naming to measure their memory ability. During a session on word repetition, subjects immediately recited a list of words that had been shown to them before, and the procedure was to assess their memory function (score from 0 to 10). The test of the orientation of time and place made subjects identify the place, day, month, and year to measure the orientation score (from 0 to 4). The arithmetic test consisted of backward counting, serial seven, and computation, and the scores were 0–2, 0–5, and 0–2, respectively. In the implementation test, subjects were asked to fold a paper and draw scores from 0 to 3 and from 0 to 1. In addition, subjects were required to name and identify a specific object (score from 0 to 2). The scores of all domains were calculated into cognitive scores from 0 to 43 to assess older adults' cognition, and higher cognition scores indicated better cognitive health in older adults. Subjects with lower than 10% cognitive scores were identified as having cognitive impairment.
Measurement of covariates
We included the following factors as covariates: The sociodemographic information included age (continuous), gender (male, female), education (never, middle school or under, secondary or above), marital status (married or in a relationship, widowed, or other), living area (urban or rural), whether to work (yes, no), economic status (tertile group-low/middle/high), caste (scheduled caste, scheduled tribe, other backward class, no or other castes), religion (Christian, Muslim, Hindu, or other); biological behavior information included moderate physical activity (more than once a week or hardly ever), body mass index (BMI) (<18.5, ≥18.5 and <25, ≥25 and <30, ≥30), drinking (never, current, ever), smoking (never, current, ever), combined chronic diseases (CCDs) (0, 1, 2, 3, or more), sleep disorder (no, yes); house environment information included other indoor pollution (no, yes), and indicators of poor housing quality (0, 1, 2, 3, 4, 5).
Several variables need to be explained in this study. Economic status was defined by annual per capita consumption expenditure. BMI (kg/m2) is defined as the weight (kg)/square of height (m). CCDs include hypertension, diabetes, tumors, lung disease, chronic heart disease, stroke, arthritis, mental disease, Alzheimer's disease, hypercholesterolemia, asthma, congestive heart failure, heart attack, abnormal heart rate, osteoporosis, abnormal thyroid function, digestive disease, skin disease, kidney stones, presbyopia, cataracts, glaucoma, myopia, hyperopia, tooth decay, and periodontal disease. Sleep disorder was identified as any of the following five situations that subjects reported: difficulty sleeping, waking up at night, waking up early, feeling sleepy during the day, and taking medicine to help sleep. Other indoor pollution included incense sticks (agarbatti), mosquito coils, liquid vaporizers/mosquito repellents/mats, fast cards/sticks/cakes, or if the housing respondent reports that a usual member of their household smokes inside their home. The subjects' answers (no, yes) to the above substances were analyzed. Indicators of poor housing quality were used to assess the quality of houses, which consisted of five indicators: house material, sanitary facilities, electric power, water source, and crowding (41). One score was recorded for each missing indicator of the subject's house, and the total score range was 0–5. The higher the score indicates, the worse the house quality.
Statistical analysis
In the present study, we classified participants into two groups based on solid fuel use, and we presented categorical and continuous variables as percentages and means ± standard deviations. Multivariable logistic regression models evaluated the associations of solid fuel use with depressive symptoms and cognitive impairment. Multivariable linear regression models assessed the associations of solid fuel use with the CES-D-10 score and cognitive score. We used five models in each analysis: the unadjusted model, model I (adjusted for age, gender, and BMI), model II (adjusted for age, gender, and BMI, education, marriage, living area, whether someone works, caste, religion, and economic status), model III (adding indicators of drinking, smoking, sleep disorders, CCDs, and vigorous physical activity into model II), model IV (adding other indoor pollution and indicators of poor housing quality). Subgroup and interaction analyses were performed and stratified by gender, caste, living area, education, economic status, smoking, drinking, and BMI to explore potential effect modification. The sample size for each analysis is shown in the Supplementary material. The results with p < 0.05 and 95% confidence intervals (95% CI) were considered statistically significant. All analyses were performed by the statistical software package R 4.1.2 (http://www.R-project.org, The R Foundation).
Result
Characteristics of the study population
A total of 29,789 participants over 60 years old were included in this study. Of whom, there were 15,507 females and 14,282 males, and the total mean age was 68.7 years. For solid fuel, 14,203 participants reported using solid fuel at home, and 15,586 participants reported using clean fuel. Compared with those using clean fuel, solid fuel users showed a higher mean CES-D-10 score (solid fuel users: 10.2; clean fuel users: 9.5) and a higher rate of depression (solid fuel users: 49.8%; clean fuel users: 42.6%). The cognitive score of solid fuel users was 21.6, which was lower than that of clean fuel users (25.4). The incidence of cognitive impairment was 23.9% in solid fuel users, which was higher than that in clean fuel users (11.5%). Solid fuel users were more likely to have a low education level, live in the village, be unemployed, have low economic status, have a low BMI, drink alcohol, smoke, and live with other indoor pollution. However, there was no significant difference in age, gender, marriage status, or indoor pollution between clean and solid fuel users. Table 1 shows the characteristics of the study participants classified by using solid fuel.
Association of solid fuel use at home with depression and cognitive impairment
We found a positive and significant association between solid fuel use and depression in logistic regression analysis. In the unadjusted model, using solid fuel was associated with a higher risk of depression, with an OR of 1.37. In model I, adjusted for age, gender, and BMI, the relationship between solid fuel use and depression remained unchanged (OR 1.27, 95% CI 1.21–1.33). In model II, the OR of depression from solid fuel use decreased after adjusting for sociodemographic information, which still met statistical significance (OR 1.15, 95% CI 1.08–1.22). After adding covariates of biological information, solid fuel use was significantly correlated with depression (OR 1.18, 95% CI 1.11–1.25) in model III. In the fully adjusted model (model IV), the significance of the relation between solid fuel use and depression decreased, with an OR of 1.09 (95% CI 1.03–1.16). There was a trend for the OR of depression from solid fuel consumption to decrease when additional covariates were included in the analysis.
With the similarity of trend, there was a significant association between solid fuel use and cognitive impairment in all models based on the logistic regression analysis that domestic solid cooking fuel use was associated with a higher prevalence of cognitive impairment (unadjusted model, OR 2.42, 95% CI 2.27–2.57; mode l I, OR 2.14, 95% CI 1.99–2.30; model II, OR 1.29, 95% CI 1.18–1.40; model III, OR 1.27,95% CI 1.16–1.38; model IV, OR 1.21, 95% CI 1.11–1.32).
We considered the CES-D-10 score a continuous variable in linear regression analysis, and the results were similar to those from logistic regression analysis. In the unadjusted model, solid fuel users had a higher CES-D-10 score than clean fuel users (β 0.77, 95% CI 0.67–0.86). In mode I, those using solid fuel were associated with a 0.60 higher CES-D-10 score than clean fuel users. The result stating solid fuel use was related to a higher CES-D-10 score remained unchanged in model II (β 0.38, 95% CI 0.27–0.49). After adjusting for biological information, the CES-D-10 score of solid fuel users was 0.39 points higher than that of clean fuel users (95% CI, 0.27–0.50). In the fully adjusted model, the difference between solid and clean fuel users decreased but met significance (β 0.23, 95% CI 0.12–0.35). Solid fuel use was negatively related to cognitive scores. Compared with those who used clean fuel, the cognitive score decreased by 3.89 in those who used solid fuel in the unadjusted model (95% CI, −3.96 to −3.65). With more covariates included in analyses, the OR-value of the cognitive score between solid fuel users and clean fuel users decreased. In the fully adjusted model, the relation between solid fuel use and the cognitive score remained unchanged (model I, β −2.91, 95% CI −3.07 to −2.76; mode lII, β −0.78,95% CI −0.93 to −0.62; model III, β −0.75, 95% CI −0.91 to −0.60; model IV, β −0.63, 95% CI −0.79 to −0.47). Table 2 presented the association between solid fuel use and depression and cognitive impairment by logistic regression and linear regression analysis.
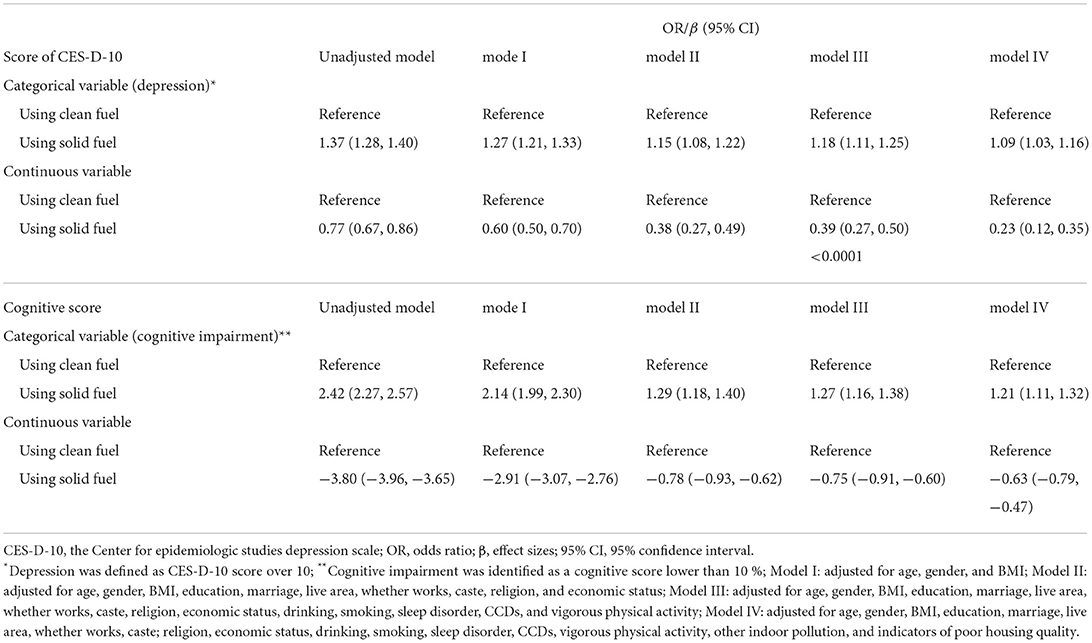
Table 2. Association of using solid fuel with symptoms of depression, CES-D-10 score, cognitive impairment, and cognitive scores.
Subgroup analyses and interaction analyses
In this present study, subgroup analyses were stratified by gender (female, male), education (never, middle school or under, and secondary or above), living area (urban, rural), caste (scheduled caste, scheduled tribe, other backward class, no or other castes), and economic status (tertile group), BMI (< 18.5, ≥18.5 and <25, ≥25 and <30, ≥30), smoking (never, current, ever), and drinking (never, current, ever). We observed significant interactions in the association between solid fuel use and depression on the relation of solid fuel with depression, in different gender and smoking (p for interaction: gender 0.009, smoking 0.002). After classifying participants by gender, the association between solid fuel use and depression still existed among females (OR 1.18, 95% CI 1.08–1.28), while the relationship between the two did not have statistical differences in males (OR 1.01, 95% CI 0.92–1.10). Those who never smoked were more likely to suffer from depression due to solid fuel use (OR 1.14, 95% CI 1.07–1.23). The correlation of solid fuel use with depression disappeared among those who ever smoked or currently smoked. We did not observe a significant interaction of BMI, economic status, caste, living area, education, or drinking on the association between solid fuel use and depression.
After adjusting for all confounders, no significant interaction of gender, education, living area, caste, BMI, smoking, or drinking on the correlation between solid fuel use and cognitive impairment was observed. We did not obtain any evidence to prove the existence of differences in the relation of solid fuel use with cognitive impairment in different subgroups. Subgroup analysis is presented in Figure 2.
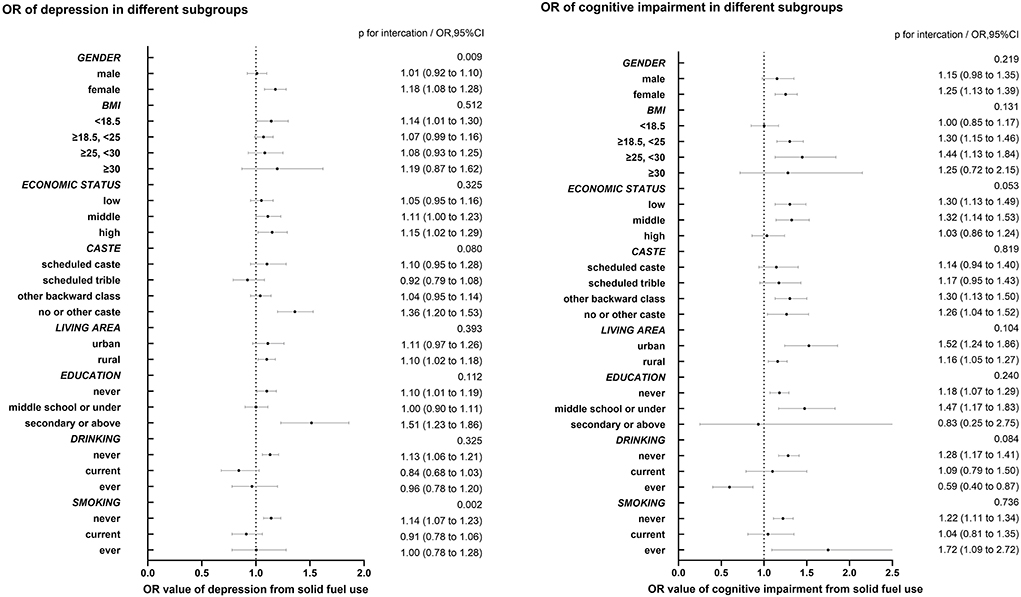
Figure 2. Subgroup analysis in the association of solid fuel use with depression and cognitive impairment. Subgroup analysis were adjusted by age, gender, BMI, education, marriage, live age, whether works, caste, religion, economic status, drinking, smoking, sleep disorder, CCDs, vigorous physical activity, other indoor pollution, indicators of poor housing quality.
Discussion
This cross-sectional study assessed the associations of solid fuel use with depression and cognitive impairment. Solid fuel use was associated with a 37% higher prevalence of depression and a 142% higher prevalence of cognitive impairment than clean fuel use in the unadjusted model. After adjusting biological and social demographic covariates, the association of solid fuel use with depression and cognitive impairment remained unchanged. After adding house quality and indoor pollution as covariates into the analysis, the value of solid fuel use on depression and cognitive impairment decreased. However, the results still showed a significant difference. In the subgroup analysis, we found that females and non-smokers suffered a higher prevalence of depression related to solid fuel use. The relationship between solid fuel and cognitive impairment was stable in different subgroups.
We analyzed the association of solid fuel use with depression and cognitive impairment among older adults based on a population sample from representable LMICs. At the same time, the current study included the largest and latest sample size among similar studies (29,789). The prevalence of depression and cognitive impairment in LMICs is increasing, especially in the elderly population, but the relevant research has been insufficient in recent years. For the extensive research on the adverse health effects of air pollution, reducing the use of solid fuels has been considered a way to improve indoor pollution (42, 43). Previous studies have also demonstrated the enormous economic burden of indoor solid fuel use (44). Our research shows that the adverse effects on health caused by solid fuels still exist based on the latest data from LASI Wave 1, even though such negative consequences had been reported beforehand. As noted previously, in the different subgroups of gender, education, BMI, smoking, or drinking, there are different susceptibilities to depression, so it is necessary to determine the association of solid fuel use with depression and cognitive impairment in those subgroups (45–54). In India, populations with different economic statuses, living areas, and castes used different ratios of solid fuel. According to our findings, subgroup analyses based on economic status, living area, and castes used different ratios of solid fuel, according to our findings of subgroup analyses.
The associations between the use of solid fuel and depression and cognitive impairment have been provided by previous studies, but the underlying molecular mechanism for the effects of air pollution on depression and cognitive impairment is not clear (30, 36, 55–60). Several explanations show possible links. First, solid fuel increases oxidative stress (OS) in the human body through a high concentration of PM and chemical substances, which promotes the progression of depression and cognitive impairment. OS plays a major role in neurodegeneration, which involves depressive pathogenesis (61, 62). Animal experiments have shown that PM2.5 promotes oxidative stress through the Nrf2/NF-κB pathway and increases the level of inflammatory cytokines (63). PM was also related to the activation of the nuclear transcription factors Nrf-2 and NF-κB in male rats, and PM may lead to physiological changes in the central nervous system (64). A study on females with solid fuel use suggested that solid fuel users had 32% more leukocytes in circulation, and reactive oxygen species (ROS) were generated at higher levels in neutrophils, lymphocytes, eosinophils, and alveolar macrophages (65). Second, metabolic alterations resulting from PM and chemical substances may lead to depression. After short- and long-term exposure to PM, triglyceride levels increased; after long-term exposure to PM, free fatty acid levels also increased (66). PM also hurts glucose metabolism: exposure to PM2.5 increases insulin resistance (67). PAHs, NO2, and CO are related to biological toxicity (68–70). Similarly, higher levels of triglycerides and free fatty acids were observed among those living with depression, and it was reported that higher glucose concentrations of the pregenual anterior cingulate were associated with major depressive disorder (71–73). In a study from China, hyperlipidemia and hyperglycemia were related to cognitive impairment (53). Finally, solid fuel use was related to depression and cognitive impairment, which may be linked to chronic disease. Solid fuel users suffer a higher risk of chronic disease. A meta-analysis suggested that multimorbidity was related to depression and cognitive impairment, which means that chronic diseases resulting from solid fuel led to the emergence of depression and cognitive impairment (26, 53, 74, 75).
As reported by previous studies, using solid fuel was related to depression, which is consistent with the results of our study (36, 55–59). The adjusted OR and 95% CI of solid fuel use for depression were OR 1.09 and 95% CI 1.03–1.16 in our study, respectively. The value differed from previous studies due to the difference in study populations and definitions of solid fuel use and depression. In the subgroup analysis, we found that females and non-smokers suffer a higher OR from solid fuel use for depression, and there was no interaction on economic status, which was different from the findings in previous studies. Contrary to our findings, research from China found no connection between gender and smoking solid fuel and depression (59). The India Human Development Survey study found that in 98% of households, females cooked, which seems to explain why females have a higher OR of solid fuel use for depression than males in the Indian population due to the higher exposure to pollutants from solid fuel (34). Secondhand smoke exposure is related to depressive symptoms among those who never smoke (76). People who are not exposed to tobacco appear to be more sensitive to air pollution, and nonsmokers who suffer a higher OR from solid fuel use for depression need further study. In a previous study, associations between using solid fuel and depression were generally higher in females and those with low household economic levels among older Chinese adults (55). In our study, low household economic levels were not related to a higher risk of depression, which may be associated with the broader use of solid fuel in India. In the subgroup of caste, different relations of solid fuel to depression were observed for the differences in health and lifestyle (77).
The association between solid fuel use and cognitive impairment has been frequently determined in China, while the evidence from other areas is limited (30, 60, 78). A study from Mexico suggested that solid cooking fuels may represent a risk factor for cognitive decline (79). Additionally, the association between solid fuel and depressive symptoms for ten years depended on the WHO Study on Global AGEing and Adult Health (SAGE) (80). A meta-analysis suggested that a 24% higher risk of depression was related to solid fuel use, while the OR of solid fuel use for depression was 1.08, according to our findings (81). Compared with another study based on the LASI study, we excluded those younger than 60 and involved indicators of poor housing quality as covariants to accurately evaluate the relationship between solid fuel use and cognitive impairment among older adults (82). Evidence from a study from northern China suggested that domestic solid fuel consumption was a dose-dependent risk factor for cognitive impairment (83). Although we did not find a significant p for interaction in subgroups between solid fuel use and cognitive impairment, there were still subgroups suffering from the different effects of solid fuel on cognitive impairment. Among males, we found a negative relationship between solid fuel and cognitive impairment compared with females because of their lower exposure to pollutants (34). There was no significant association between solid fuel use and cognitive impairment in subgroups of high economic status and high educational status, which indicates the protective effect of the two (51, 84). In our study, the correlations between solid fuel use and cognitive impairment were stable and consistent with the findings from a previous study (82).
There were several limitations to this study. First, the present study is cross-sectional, making it difficult to identify the causal relationship between solid fuel use and depression or cognitive impairment. Second, our study's definitions of depression and cognitive impairment were based on screening tools (the CES-D-10 and HRS) but not diagnostic criteria. The CES-D-10 was primarily used for assessing depression symptom severity (40). Although the HRS cognitive assessment method is widely used in various studies as a face-to-face interview-based test, there are still some limitations (85). Third, the patient's answer determined whether the subjects used solid fuel, which may not have been objectively observed and have certain recall biases. Fourth, due to the lack of relevant data, we did not evaluate the impact of the total time of solid fuel use every day and the total time of solid fuel use on the association between solid fuel use and depressive symptoms. Fifth, because of the lack of kitchen ventilation, we used indicators of poor housing quality to assess the quality of the house. Indicators of poor housing quality helped us reduce the statistical bias caused by the lack of kitchen ventilation information in a previous study (41). Sixth, due to the low concentration of air pollution to which the subjects were exposed, we did not analyze the dose relationship of air pollutant concentrations with depression and cognitive impairment. Seventh, the population we studied was comprised of elderly individuals over the age of 60 and did not include people under 60. The relationship of solid fuel use with depression and cognitive impairment in these populations needs further verification.
Although evidence from a 10-year study suggested a link between solid fuel use and depression, solid fuel use was also related to depression in our research, indicating that solid fuel's effect still existed. Therefore, more government measures to improve indoor pollution are needed (59, 80). Older adults should use clean fuel at home for cooking, which is beneficial for their mental health and cognition. Our study first provided the association of solid fuel use with depression and depression at the same time among older adults, and more policies on reducing the use of solid fuels, promoting clean fuels, and improving air quality are needed.
Conclusion
Domestic solid cooking fuel use was associated with the increased prevalence of depression and cognitive impairment among older adults in India.
Data availability statement
The datasets presented in this study can be found in online repositories. The names of the repository/repositories and accession number(s) can be found at: https://doi.org/10.25549/h-lasi.
Ethics statement
The studies involving human participants were reviewed and approved by the Indian Council of Medical Research. The patients/participants provided their written informed consent to participate in this study.
Author contributions
SQ, XZ, and QW were involved in the conception and design of the work. YJ, LD, and XX contributed to the acquisition, analysis, and interpretation of the data. YJ and YL finished the first draft. SQ and BD revised the manuscript for important intellectual content. All authors reviewed and approved the final version.
Funding
This work was supported by programs from the Science and Technology Department of Sichuan Province (2021YJ0462), the 1.3.5 Project for Disciplines of Excellence at West China Hospital, Sichuan University (ZYGD20010), the Health and Family Planning Commission of Sichuan Province (20PJ039), the China Postdoctoral Science Foundation (2020M670057ZX), a postdoctoral research project at West China Hospital, Sichuan University (2019HXBH092), and the Chengdu Science and Technology Bureau Major Science and Technology Application Demonstration Project (2019YF0900083SN).
Acknowledgments
This analysis uses data or information from the Harmonized LASI dataset and Codebook, Version A.2 as of October 2021, developed by the Gateway to Global Aging Data (DOI: https://doi.org/10.25549/h-lasi). The development of the Harmonized LASI was funded by the National Institute on Aging (R01 AG042778, 2R01 AG030153, and 2R01 AG051125). For more information about the Harmonization Project, please refer to https://g2aging.org/.
Conflict of interest
The authors declare that the research was conducted in the absence of any commercial or financial relationships that could be construed as a potential conflict of interest.
Publisher's note
All claims expressed in this article are solely those of the authors and do not necessarily represent those of their affiliated organizations, or those of the publisher, the editors and the reviewers. Any product that may be evaluated in this article, or claim that may be made by its manufacturer, is not guaranteed or endorsed by the publisher.
Supplementary material
The Supplementary Material for this article can be found online at: https://www.frontiersin.org/articles/10.3389/fpubh.2022.1038573/full#supplementary-material
References
1. Arena R, Mcneil A, Sagner M, Hills AP. The current global state of key lifestyle characteristics: health and economic implications. Prog Cardiovasc Dis. (2017) 59:422–9. doi: 10.1016/j.pcad.2017.02.002
2. Yuan Y, Lapane KL, Tjia J, Baek J, Liu SH, Ulbricht CM. Physical frailty and cognitive impairment in older nursing home residents: a latent class analysis. BMC Geriatr. (2021) 21:487. doi: 10.1186/s12877-021-02433-1
3. Zhang PD, Lv YB, Li ZH, Yin ZX, Li FR, Wang JN, et al. Age, period, and cohort effects on activities of daily living, physical performance, and cognitive functioning impairment among the oldest-old in China. J Gerontol A Biol Sci Med Sci. (2020) 75:1214–21. doi: 10.1093/gerona/glz196
4. Abrahamson K, Clark D, Perkins A, Arling G. Does cognitive impairment influence the quality of life among nursing home residents? Gerontologist. (2012) 52:632–40. doi: 10.1093/geront/gnr137
5. Jokinen H, Koikkalainen J, Laakso HM, Melkas S, Nieminen T, Brander A, et al. Global burden of small vessel disease-related brain changes on MRI predicts cognitive and functional decline. Stroke. (2020) 51:170–8. doi: 10.1161/STROKEAHA.119.026170
6. Domènech-Abella J, Lara E, Rubio-Valera M, Olaya B, Moneta MV, Rico-Uribe LA, et al. Loneliness and depression in the elderly: the role of social network. Soc Psychiatry Psychiatr Epidemiol. (2017) 52:381–90. doi: 10.1007/s00127-017-1339-3
7. Ouyang Z, Chong AM, Ng TK, Liu S. Leisure, functional disability and depression among older Chinese living in residential care homes. Aging Ment Health. (2015) 19:723–30. doi: 10.1080/13607863.2014.962009
8. Moussavi S, Chatterji S, Verdes E, Tandon A, Patel V, Ustun B. Depression, chronic diseases, and decrements in health: results from the world health surveys. Lancet. (2007) 370:851–8. doi: 10.1016/S0140-6736(07)61415-9
9. Fugger G, Waldhör T, Hinterbuchinger B, Pruckner N, König D, Gmeiner A, et al. Pattern of inpatient care for depression: an analysis of 232,289 admissions. BMC Psychiatry. (2020) 20:375. doi: 10.1186/s12888-020-02781-z
10. Luppa M, Sikorski C, Luck T, Ehreke L, Konnopka A, Wiese B, et al. Age- and gender-specific prevalence of depression in latest-life–systematic review and meta-analysis. J Affect Disord. (2012) 136:212–21. doi: 10.1016/j.jad.2010.11.033
11. Jonsson U, Bertilsson G, Allard P, Gyllensvärd H, Söderlund A, Tham A, et al. Psychological treatment of depression in people aged 65 years and over: a systematic review of efficacy, safety, and cost-effectiveness. PLoS ONE. (2016) 11:e0160859. doi: 10.1371/journal.pone.0160859
12. Deardorff WJ, Liu PL, Sloane R, Van Houtven C, Pieper CF, Hastings SN, et al. Association of sensory and cognitive impairment with healthcare utilization and cost in older adults. J Am Geriatr Soc. (2019) 67:1617–24. doi: 10.1111/jgs.15891
13. Stahl ST, Beach SR, Musa D, Schulz R. Living alone and depression: the modifying role of the perceived neighborhood environment. Aging Ment Health. (2017) 21:1065–71. doi: 10.1080/13607863.2016.1191060
14. Tolahunase MR, Sagar R, Faiq M, Dada R. Yoga- and meditation-based lifestyle intervention increases neuroplasticity and reduces severity of major depressive disorder: a randomized controlled trial. Restor Neurol Neurosci. (2018) 36:423–42. doi: 10.3233/RNN-170810
15. Braithwaite I, Zhang S, Kirkbride JB, Osborn DPJ, Hayes JF. Air pollution (particulate matter) exposure and associations with depression, anxiety, bipolar, psychosis and suicide risk: a systematic review and meta-analysis. Environ Health Perspect. (2019) 127:126002. doi: 10.1289/EHP4595
16. Yu X, Zheng L, Jiang W, Zhang D. Exposure to air pollution and cognitive impairment risk: a meta-analysis of longitudinal cohort studies with dose-response analysis. J Glob Health. (2020) 10:010417. doi: 10.7189/jogh.10.010417
17. Ostro B, Spadaro JV, Gumy S, Mudu P, Awe Y, Forastiere F, et al. Assessing the recent estimates of the global burden of disease for ambient air pollution: methodological changes and implications for low- and middle-income countries. Environ Res. (2018) 166:713–25. doi: 10.1016/j.envres.2018.03.001
18. Guan WJ, Zheng XY, Chung KF, Zhong NS. Impact of air pollution on the burden of chronic respiratory diseases in China: time for urgent action. Lancet. (2016) 388:1939–51. doi: 10.1016/S0140-6736(16)31597-5
19. Al-Aly Z, Bowe B. Air pollution and kidney disease. Clin J Am Soc Nephrol. (2020) 15:301–3. doi: 10.2215/CJN.16031219
20. Kim SY, Kim SH, Wee JH, Min C, Han SM, Kim S, et al. Short and long term exposure to air pollution increases the risk of ischemic heart disease. Sci Rep. (2021) 11:5108. doi: 10.1038/s41598-021-84587-x
21. Buoli M, Grassi S, Caldiroli A, Carnevali GS, Mucci F, Iodice S, et al. Is there a link between air pollution and mental disorders? Environ Int. (2018) 118:154–68. doi: 10.1016/j.envint.2018.05.044
22. Griffiths CJ, Mudway IS. Air pollution and cognition. BMJ. (2018) 363:k4904. doi: 10.1136/bmj.k4904
23. Deng M, Li P, Ma R, Shan M, Yang X. Air pollutant emission factors of solid fuel stoves and estimated emission amounts in rural Beijing. Environ Int. (2020) 138:105608. doi: 10.1016/j.envint.2020.105608
24. Du W, Zhuo S, Wang J, Luo Z, Chen Y, Wang Z, et al. Substantial leakage into indoor air from on-site solid fuel combustion in chimney stoves. Environ Pollut. (2021) 291:118138. doi: 10.1016/j.envpol.2021.118138
25. Lee KK, Bing R, Kiang J, Bashir S, Spath N, Stelzle D, et al. Adverse health effects associated with household air pollution: a systematic review, meta-analysis, and burden estimation study. Lancet Glob Health. (2020) 8:e1427–34. doi: 10.1016/S2214-109X(20)30343-0
26. Balmes JR. Household air pollution from domestic combustion of solid fuels and health. J Allergy Clin Immunol. (2019) 143:1979–87. doi: 10.1016/j.jaci.2019.04.016
27. Sutunkova MP, Katsnelson BA, Privalova LI, Gurvich VB, Konysheva LK, Shur VY, et al. On the contribution of the phagocytosis and the solubilization to the iron oxide nanoparticles retention in and elimination from lungs under long-term inhalation exposure. Toxicology. (2016) 363–4:19–28. doi: 10.1016/j.tox.2016.07.006
28. Li Q, Zheng J, Xu S, Zhang J, Cao Y, Qin Z, et al. The neurotoxicity induced by PM(2.5) might be strongly related to changes of the hippocampal tissue structure and neurotransmitter levels. Toxicol Res. (2018) 7:1144–52. doi: 10.1039/C8TX00093J
29. Li C, Zhou Y, Ding L. Effects of long-term household air pollution exposure from solid fuel use on depression: Evidence from national longitudinal surveys from 2011 to (2018). Environ Pollut. (2021) 283:117350. doi: 10.1016/j.envpol.2021.117350
30. Luo Y, Zhong Y, Pang L, Zhao Y, Liang R, Zheng X. The effects of indoor air pollution from solid fuel use on cognitive function among middle-aged and older population in China. Sci Total Environ. (2021) 754:142460. doi: 10.1016/j.scitotenv.2020.142460
31. Ravindranath V, Sundarakumar JS. Changing demography and the challenge of dementia in India. Nat Rev Neurol. (2021) 17:747–58. doi: 10.1038/s41582-021-00565-x
32. Srivastava S, Debnath P, Shri N, Muhammad T. The association of widowhood and living alone with depression among older adults in India. Sci Rep. (2021) 11:21641. doi: 10.1038/s41598-021-01238-x
33. Muhammad T, Meher T. Association of late-life depression with cognitive impairment: evidence from a cross-sectional study among older adults in India. BMC Geriatr. (2021) 21:364. doi: 10.1186/s12877-021-02314-7
34. Choudhuri P, Desai S. Gender inequalities and household fuel choice in India. J Clean Prod. (2020) 265:121487. doi: 10.1016/j.jclepro.2020.121487
35. Mani S, Jain A, Tripathi S, Gould CF. The drivers of sustained use of liquified petroleum gas in India. Nat Energy. (2020) 5:450–7. doi: 10.1038/s41560-020-0596-7
36. Banerjee M, Siddique S, Dutta A, Mukherjee B, Ranjan Ray M. Cooking with biomass increases the risk of depression in pre-menopausal women in India. Soc Sci Med. (2012) 75:565–72. doi: 10.1016/j.socscimed.2012.03.021
37. Nagarkar A, Kulkarni S. Association between daily activities and fall in older adults: an analysis of longitudinal ageing study in India (2017-18). BMC Geriatr. (2022) 22:203. doi: 10.1186/s12877-022-02879-x
38. James C, Powell M, Seixas A, Bateman A, Pengpid S, Peltzer K. Exploring the psychometric properties of the CES-D-10 and its practicality in detecting depressive symptomatology in 27 low- and middle-income countries. Int J Psychol. (2020) 55:435–45. doi: 10.1002/ijop.12613
39. Irwin M, Artin KH, Oxman MN. Screening for depression in the older adult: criterion validity of the 10-item center for epidemiological studies depression scale (CES-D). Arch Intern Med. (1999) 159:1701–4. doi: 10.1001/archinte.159.15.1701
40. Björgvinsson T, Kertz SJ, Bigda-Peyton JS, Mccoy KL, Aderka IM. Psychometric properties of the CES-D-10 in a psychiatric sample. Assessment. (2013) 20:429–36. doi: 10.1177/1073191113481998
41. Saenz JL, Adar SD, Zhang YS, Wilkens J, Chattopadhyay A, Lee J, et al. Household use of polluting cooking fuels and late-life cognitive function: a harmonized analysis of India, Mexico, and China. Environ Int. (2021) 156:106722. doi: 10.1016/j.envint.2021.106722
42. Lin HH, Murray M, Cohen T, Colijn C, Ezzati M. Effects of smoking and solid-fuel use on COPD lung cancer, and tuberculosis in China: a time-based, multiple risk factor, modelling study. Lancet. (2008) 372:1473–83. doi: 10.1016/S0140-6736(08)61345-8
43. Gordon SB, Bruce NG, Grigg J, Hibberd PL, Kurmi OP, Lam KB, et al. Respiratory risks from household air pollution in low and middle income countries. Lancet Respir Med. (2014) 2:823–60. doi: 10.1016/S2213-2600(14)70168-7
44. Balakrishnan K, Ghosh S, Ganguli B, Sambandam S, Bruce N, Barnes DF, et al. State and national household concentrations of PM2.5 from solid cookfuel use: results from measurements and modeling in India for estimation of the global burden of disease. Environ Health. (2013) 12:77. doi: 10.1186/1476-069X-12-77
45. Jantaratnotai N, Mosikanon K, Lee Y, Mcintyre RS. The interface of depression and obesity. Obes Res Clin Pract. (2017) 11:1–10. doi: 10.1016/j.orcp.2016.07.003
46. Salk RH, Hyde JS, Abramson LY. Gender differences in depression in representative national samples: meta-analyses of diagnoses and symptoms. Psychol Bull. (2017) 143:783–822. doi: 10.1037/bul0000102
47. Nguyen AW, Walton QL, Thomas C, Mouzon DM, Taylor HO. Social support from friends and depression among African Americans: the moderating influence of education. J Affect Disord. (2019) 253:1–7. doi: 10.1016/j.jad.2019.04.013
48. Fluharty M, Taylor AE, Grabski M, Munafò MR. The association of cigarette smoking with depression and anxiety: a systematic review. Nicotine Tob Res. (2017) 19:3–13. doi: 10.1093/ntr/ntw140
49. Lee SB, Chung S, Lee H, Seo JS. The mutual relationship between men's drinking and depression: a 4-year longitudinal analysis. Alcohol Alcohol. (2018) 53:597–602. doi: 10.1093/alcalc/agy003
50. Overton M, Pihlsgård M, Elmståhl S. Prevalence and incidence of mild cognitive impairment across subtypes, age, and sex. Dement Geriatr Cogn Disord. (2019) 47:219–32. doi: 10.1159/000499763
51. Lövdén M, Fratiglioni L, Glymour MM, Lindenberger U, Tucker-Drob EM. Education and cognitive functioning across the life span. Psychol Sci Public Interest. (2020) 21:6–41. doi: 10.1177/1529100620920576
52. Hou Q, Guan Y, Yu W, Liu X, Wu L, Xiao M, et al. Associations between obesity and cognitive impairment in the Chinese elderly: an observational study. Clin Interv Aging. (2019) 14:367–73. doi: 10.2147/CIA.S192050
53. Jia L, Du Y, Chu L, Zhang Z, Li F, Lyu D, et al. Prevalence, risk factors, and management of dementia and mild cognitive impairment in adults aged 60 years or older in China: a cross-sectional study. Lancet Public Health. (2020) 5:e661–71. doi: 10.1016/S2468-2667(20)30185-7
54. Topiwala A, Ebmeier KP. Effects of drinking on late-life brain and cognition. Evid Based Ment Health. (2018) 21:12–5. doi: 10.1136/eb-2017-102820
55. Liu Y, Chen X, Yan Z. Depression in the house: the effects of household air pollution from solid fuel use among the middle-aged and older population in China. Sci Total Environ. (2020) 703:134706. doi: 10.1016/j.scitotenv.2019.134706
56. Shao J, Ge T, Liu Y, Zhao Z, Xia Y. Longitudinal associations between household solid fuel use and depression in middle-aged and older Chinese population: a cohort study. Ecotoxicol Environ Saf. (2021) 209:111833. doi: 10.1016/j.ecoenv.2020.111833
57. Zhang H, Xia Y, Cao L, Chang Q, Zhao Y. Associations between long term exposures to outdoor air pollution and indoor solid fuel use and depression in China. J Environ Manage. (2022) 302:113982. doi: 10.1016/j.jenvman.2021.113982
58. Liao W, Liu X, Kang N, Song Y, Li R, Song X, et al. Effect modification of kitchen ventilation on the associations of solid fuel use and long-duration cooking with the increased prevalence of depressive and anxiety symptoms: the Henan rural cohort study. Indoor Air. (2022) 32:e13016. doi: 10.1111/ina.13016
59. Deng Y, Zhao H, Liu Y, Liu H, Shi J, Zhao C, et al. Association of using biomass fuel for cooking with depression and anxiety symptoms in older Chinese adults. Sci Total Environ. (2022) 811:152256. doi: 10.1016/j.scitotenv.2021.152256
60. Cao L, Zhao Z, Ji C, Xia Y. Association between solid fuel use and cognitive impairment: a cross-sectional and follow-up study in a middle-aged and older Chinese population. Environ Int. (2021) 146:106251. doi: 10.1016/j.envint.2020.106251
61. Bhatt S, Nagappa AN, Patil CR. Role of oxidative stress in depression. Drug Discov Today. (2020) 25:1270–6. doi: 10.1016/j.drudis.2020.05.001
62. Yan N, Xu Z, Qu C, Zhang J. Dimethyl fumarate improves cognitive deficits in chronic cerebral hypoperfusion rats by alleviating inflammation, oxidative stress, and ferroptosis via NRF2/ARE/NF-κB signal pathway. Int Immunopharmacol. (2021) 98:107844. doi: 10.1016/j.intimp.2021.107844
63. Piao CH, Fan Y, Nguyen TV, Shin HS, Kim HT, Song CH, et al. PM(2.5) exacerbates oxidative stress and inflammatory response through the Nrf2/NF-κB signaling pathway in OVA-induced allergic rhinitis mouse model. Int J Mol Sci. (2021) 22:8173. doi: 10.3390/ijms22158173
64. Guerra R, Vera-Aguilar E, Uribe-Ramirez M, Gookin G, Camacho J, Osornio-Vargas AR, et al. Exposure to inhaled particulate matter activates early markers of oxidative stress, inflammation and unfolded protein response in rat striatum. Toxicol Lett. (2013) 222:146–54. doi: 10.1016/j.toxlet.2013.07.012
65. Mondal NK, Saha H, Mukherjee B, Tyagi N, Ray MR. Inflammation, oxidative stress, and higher expression levels of Nrf2 and NQO1 proteins in the airways of women chronically exposed to biomass fuel smoke. Mol Cell Biochem. (2018) 447:63–76. doi: 10.1007/s11010-018-3293-0
66. Yang S, Chen R, Zhang L, Sun Q, Li R, Gu W, et al. Lipid metabolic adaption to long-term ambient PM(2.5) exposure in mice. Environ Pollut. (2021) 269:116193. doi: 10.1016/j.envpol.2020.116193
67. Rajagopalan S, Park B, Palanivel R, Vinayachandran V, Deiuliis JA, Gangwar RS, et al. Metabolic effects of air pollution exposure and reversibility. J Clin Invest. (2020) 130:6034–40. doi: 10.1172/JCI137315
68. Stading R, Gastelum G, Chu C, Jiang W, Moorthy B. Molecular mechanisms of pulmonary carcinogenesis by polycyclic aromatic hydrocarbons (PAHs): Implications for human lung cancer. Semin Cancer Biol. (2021) 76:3–16. doi: 10.1016/j.semcancer.2021.07.001
69. González-Santiago AE, Zúñiga-González GM, Gómez-Meda BC, Gutiérrez-Corral FJ, Zamora-Perez AL, Sánchez-Parada MG. Cytogenotoxicity evaluation of young adults exposed to high levels of air pollution in a mexican metropolitan zone using buccal micronucleus cytome assay. Biomed Res Int. (2021) 2021:6630861. doi: 10.1155/2021/6630861
70. Tian X, Guan T, Guo Y, Zhang G, Kong J. Selective susceptibility of oligodendrocytes to carbon monoxide poisoning: implication for delayed neurologic sequelae (DNS). Front Psychiatry. (2020) 11:815. doi: 10.3389/fpsyt.2020.00815
71. Zhang M, Chen J, Yin Z, Wang L, Peng L. The association between depression and metabolic syndrome and its components: a bidirectional two-sample mendelian randomization study. Transl Psychiatry. (2021) 11:633. doi: 10.1038/s41398-021-01759-z
72. Ter Horst DM, Schene AH, Figueroa CA, Assies J, Lok A, Bockting CLH, et al. Cortisol, dehydroepiandrosterone sulfate, fatty acids, and their relation in recurrent depression. Psychoneuroendocrinology. (2019) 100:203–12. doi: 10.1016/j.psyneuen.2018.10.012
73. Ernst J, Hock A, Henning A, Seifritz E, Boeker H, Grimm S. Increased pregenual anterior cingulate glucose and lactate concentrations in major depressive disorder. Mol Psychiatry. (2017) 22:113–9. doi: 10.1038/mp.2016.73
74. Liu J, Hou B, Ma XW, Liao H. Solid fuel use for cooking and its health effects on the elderly in rural China. Environ Sci Pollut Res Int. (2018) 25:3669–80. doi: 10.1007/s11356-017-0720-9
75. Read JR, Sharpe L, Modini M, Dear BF. Multimorbidity and depression: a systematic review and meta-analysis. J Affect Disord. (2017) 221:36–46. doi: 10.1016/j.jad.2017.06.009
76. Bandiera FC, Arheart KL, Caban-Martinez AJ, Fleming LE, Mccollister K, Dietz NA, et al. Secondhand smoke exposure and depressive symptoms. Psychosom Med. (2010) 72:68–72. doi: 10.1097/PSY.0b013e3181c6c8b5
77. Bawaskar HS, Bawaskar PH, Bawaskar PH. Health and the Indian caste system. Lancet. (2015) 385:416. doi: 10.1016/S0140-6736(15)60149-0
78. Chen H, Chen L, Hao G. Sex difference in the association between solid fuel use and cognitive function in rural China. Environ Res. (2021) 195:110820. doi: 10.1016/j.envres.2021.110820
79. Saenz JL. Solid cooking fuel use and cognitive decline among older Mexican adults. Indoor Air. (2021) 31:1522–32. doi: 10.1111/ina.12844
80. Li X, Guo Y, Xiao J, Liu T, Zeng W, Hu J, et al. The effect of polluting cooking fuels on depression among older adults in six low- and middle-income countries. Sci Total Environ. (2022) 838:155690. doi: 10.1016/j.scitotenv.2022.155690
81. Li N, Song Q, Su W, Guo X, Wang H, Liang Q, et al. Exposure to indoor air pollution from solid fuel and its effect on depression: a systematic review and meta-analysis. Environ Sci Pollut Res Int. (2022) 29:49553–67. doi: 10.1007/s11356-022-20841-7
82. Jana A, Varghese JS, Naik G. Household air pollution and cognitive health among Indian older adults: evidence from LASI. Environ Res. (2022) 214:113880. doi: 10.1016/j.envres.2022.113880
83. Tseng TJ, Carter E, Yan L, Chan Q, Elliott P, Ezzati M, et al. Household air pollution from solid fuel use as a dose-dependent risk factor for cognitive impairment in northern China. Sci Rep. (2022) 12:6187. doi: 10.1038/s41598-022-10074-6
84. Basta NE, Matthews FE, Chatfield MD, Brayne C. Community-level socio-economic status and cognitive and functional impairment in the older population. Eur J Public Health. (2008) 18:48–54. doi: 10.1093/eurpub/ckm076
Keywords: indoor air pollution, solid fuel, depression, older adults, cognitive impairment
Citation: Jin Y, Zhou X, Deng L, Xiong X, Li Y, Wei Q, Dong B and Qiu S (2022) Association between the domestic use of solid cooking fuel and increased prevalence of depression and cognitive impairment in a big developing country: A large-scale population-based study. Front. Public Health 10:1038573. doi: 10.3389/fpubh.2022.1038573
Received: 07 September 2022; Accepted: 04 November 2022;
Published: 24 November 2022.
Edited by:
Zhiming Yang, University of Science and Technology Beijing, ChinaReviewed by:
Dorina Cadar, Brighton and Sussex Medical School, United KingdomRobert Bradley, Arizona State University, United States
Copyright © 2022 Jin, Zhou, Deng, Xiong, Li, Wei, Dong and Qiu. This is an open-access article distributed under the terms of the Creative Commons Attribution License (CC BY). The use, distribution or reproduction in other forums is permitted, provided the original author(s) and the copyright owner(s) are credited and that the original publication in this journal is cited, in accordance with accepted academic practice. No use, distribution or reproduction is permitted which does not comply with these terms.
*Correspondence: Shi Qiu, cWl1c2hpQHNjdS5lZHUuY24=; Birong Dong, Ymlyb25nZG9uZzEyM0BvdXRsb29rLmNvbQ==
†These authors have contributed equally to this work and share first authorship