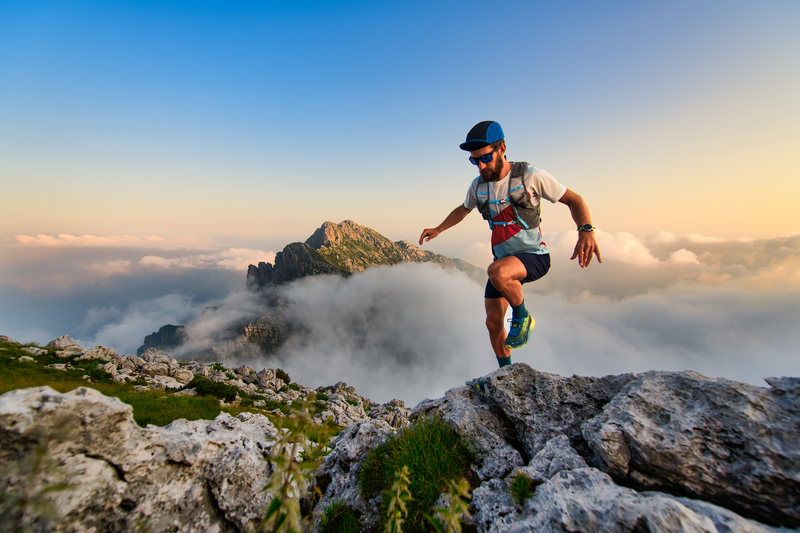
95% of researchers rate our articles as excellent or good
Learn more about the work of our research integrity team to safeguard the quality of each article we publish.
Find out more
ORIGINAL RESEARCH article
Front. Public Health , 17 October 2022
Sec. Infectious Diseases: Epidemiology and Prevention
Volume 10 - 2022 | https://doi.org/10.3389/fpubh.2022.1015714
Background: Trachoma, caused by ocular infection with Chlamydia trachomatis, is a neglected tropical disease that can lead to blinding pathology. Current trachoma control programmes have successfully used mass drug administration (MDA) with azithromycin to clear C. trachomatis infection and reduce transmission, alongside promoting facial cleanliness for better personal hygiene and environmental improvement. In areas of low-trachoma endemicity, the relationship between C. trachomatis infection and trachomatous disease weakens, and non-chlamydial bacteria have been associated with disease signs.
Methods: We enrolled a cohort of children aged 6–10 years from three adjacent trachoma endemic villages in Kilimanjaro and Arusha regions, Northern Tanzania. Children were divided into four clinical groups based on the presence or absence of ocular C. trachomatis infection and clinical signs of trachomatous papillary inflammation (TP). To determine the impact of treatment on the ocular microbiome in these clinical groups, we performed V4-16S rRNA sequencing of conjunctival DNA from children 3–9 months pre-MDA (n = 269) and 3 months post-MDA (n = 79).
Results: Chlamydia trachomatis PCR-negative, no TP children had the highest pre-MDA ocular microbiome alpha diversity, which was reduced in C. trachomatis infected children and further decreased in those with TP. Pre-MDA, Haemophilus and Staphylococcus were associated with C. trachomatis infection with and without concurrent TP, while Helicobacter was increased in those with TP in the absence of current C. trachomatis infection. Post-MDA, none of the studied children had ocular C. trachomatis infection or TP. MDA increased ocular microbiome diversity in all clinical groups, the change was of greater magnitude in children with pre-MDA TP. MDA effectively reduced the prevalence of disease causing pathogenic non-chlamydial bacteria, and promoted restoration of a normal, healthy conjunctival microbiome.
Conclusion: We identified Helicobacter as a non-chlamydial bacterium associated with the clinical signs of TP. Further investigation to determine its relevance in other low-endemicity communities is required. MDA was shown to be effective at clearing C. trachomatis infection and other non-chlamydial ocular pathogens, without any detrimental longitudinal effects on the ocular microbiome. These findings suggest that azithromycin MDA may be valuable in trachoma control even in populations where the relationship between clinical signs of trachoma and the prevalence of current ocular C. trachomatis infection has become dissociated.
Trachoma is a neglected tropical disease caused by ocular infection with Chlamydia trachomatis. The WHO-endorsed SAFE (surgery, antibiotics, facial cleanliness, and environmental improvement) strategy (1) for trachoma control has been implemented across the globe for 20 years, significantly reducing trachoma endemicity in many countries by stopping, or limiting, transmission of C. trachomatis.
Numerous studies have shown a strong association between detection of C. trachomatis and active trachoma disease signs, however the relationship between them is consistently weaker in settings of lower trachoma endemicity (2–5). While in some scenarios this is likely in part due to lower bacterial loads, there is considerable evidence of non-chlamydial bacteria being associated with trachomatous disease (2, 3, 6), particularly in the presence of the longer-term conjunctival scarring sequelae that lead to blindness (7, 8). In support of this, multiple studies have identified trachomatous papillary inflammation (TP) as a driver of incident and progressive conjunctival scarring, independent of C. trachomatis infection (9, 10).
Azithromycin MDA is effective at reducing prevalence of ocular C. trachomatis infection (1, 11) without giving rise to antibiotic-resistant C. trachomatis (12–15). However, it can drive antibiotic resistance in other bacteria, both in the nasopharynx and the gut (16–20). As well as promoting acquisition of antibiotic resistance, azithromycin MDA has consistently been shown to lead to short-term changes in the overall composition of the gut microbiota (18, 21), with variable evidence of longer-term perturbations (22, 23). As yet, no studies have explored the impact of azithromycin MDA for trachoma on the ocular microbiome.
In this study, to identify non-chlamydial bacteria associated with TP and how they're impacted by azithromycin MDA, we profiled the ocular microbiome of children from three trachoma-endemic villages in Kilimanjaro and Arusha regions of northern Tanzania 3 to 9 months pre-MDA and again 3 months post-MDA.
This study was reviewed and approved by the Tanzanian National Institute for Medical Research Ethics Committee, the Kilimanjaro Christian Medical Centre Ethics Committee, and the London School of Hygiene & Tropical Medicine Ethics Committee. It adhered to the tenets of the Declaration of Helsinki.
The details of the study design and population have been described previously (24). Briefly, the study was conducted in three adjacent trachoma endemic villages in Kilimanjaro and Arusha regions, Northern Tanzania. In January 2012, we recruited a cohort of children aged 6–10 years from these villages. The cohort was subsequently followed-up every 3 months for 4 years to investigate the pathogenesis of conjunctival scarring. At each visit, the left eye of each child was graded for trachoma by an ophthalmic nurse using the 1981 WHO Detailed Trachoma Grading System (FPC) (25), and a photograph was taken of the conjunctiva. For the purpose of this study, we consider that papillary hypertrophy scores of both two (P2) and three (P3) represent clinically significant papillary inflammation and refer to this as “TP.” Progressive scarring was subsequently determined from photographs by an ophthalmologist experienced in using a detailed scarring grading system (7), as described previously (10). Two conjunctival swabs were collected and placed into a dry tube and one containing RNAlater (Life Technologies). Air control swabs were collected after every 50 samples by passing a swab 10 cm from a participant's everted eye, these were labeled and processed identically to participant samples. All residents of the three villages received three rounds of annual mass antibiotic treatment with oral azithromycin (and topical tetracycline ointment for infants under 6 months and pregnant women), during the course of the longitudinal study.
DNA was extracted from the conjunctival swab stored in RNALater using a DNA/RNA Purification Kit (Norgen Biotek Corp), following the manufacturer's instructions. Extracted DNA was quantified on a Qubit 2.0 Fluorometer (Invitrogen) with high-sensitivity reagents (Life Technologies), following the manufacturer's instructions.
The details of 16S rRNA amplification and sequencing have been described previously (26). Briefly, DNA libraries were prepared by amplifying a ~390 bp V4 region of the 16S rRNA gene in genomic DNA. Each PCR run included a mock bacterial community (composed of DNA from Haemophilus influenza, Moraxella catarrhalis and Staphylococcus epidermis), which acted as a positive control, and a no-template control (molecular-grade water). Purified amplicons were subjected to a second, barcoding PCR to enable downstream multiplexing. The barcoded amplicons were again purified using AMPure XP beads (Beckman Coulter) and then quantified on a Qubit 2.0 Fluorometer (Invitrogen) with high-sensitivity reagents (Life Technologies) and thereafter pooled in equimolar amounts. A PhiX-spiked DNA library was then assayed by 2 × 300 bp paired-end sequencing on the Illumina MiSeq platform for a total of 600 cycles. Anonymized V4-16S sequencing data are available from the Sequence Read Archive (SRA) at the National Center for Biotechnology Information (NCBI) under accession number PRJNA815053.
Raw reads generated by MiSeq were error-corrected and filtered using DADA2 (27) through QIIME2 (28). Filtered reads were clustered de novo into Operational Taxonomic Units (OTUs) at 97% sequence similarity. OTUs were then assigned taxonomy using a Naive Bayes classifier trained on the SILVA 16S database. Both processes were performed with QIIME2. Manual filtering of classified OTUs was performed using R as described below. OTUs were retained if they had been classified as bacteria, had a genus-level classification and constituted >0.005% of the total number of reads. Ralstonia and Nocardioides were removed because they had an average abundance of >1,000 reads in the no-template (water) and air controls, respectively. Samples with < 1,000 reads were excluded. Final read counts were rarefied to 10,000 reads per sample using the R package vegan. Alpha diversity (Shannon's H) and beta diversity (weighted UniFrac distance) were calculated using the R package vegan.
To detect C. trachomatis, we extracted DNA from conjunctival swab samples stored in dry tubes using the PowerSoil DNA Isolation Kit (Mo Bio Laboratories), according to the manufacturer's instructions. The cells attached to the swabs were initially disrupted by bead beating to release their contents. C. trachomatis DNA was detected using a previously described droplet digital PCR assay (29). All samples were tested for the C. trachomatis plasmid and the human gene RPP30.
For all downstream comparisons, samples were defined as “Ct–/TP–” (C. trachomatis PCR-negative, no TP), “Ct+/TP–” (C. trachomatis PCR-positive, no TP), “Ct–/TP+” (C. trachomatis PCR-negative, signs of TP) or “Ct+/TP+” (C. trachomatis PCR-positive, signs of TP). Alpha diversities were compared by linear regression, adjusting for age. Beta diversities were compared by PERMANOVA with 1,000 permutations using the R package vegan. Prevalence (read count >0) and rarefied abundances were compared between clinical groups by binomial logistic regression. P-values were corrected for multiplicity of testing using the Benjamini-Hochberg procedure.
From the complete cohort of 666 children, 633 had DNA available for sequencing prior to MDA. Based on clinical trachoma grading and C. trachomatis PCR testing, children were classified as “Ct–/TP–,” “Ct+/TP–,” “Ct–/TP+” or “Ct+/TP+.” We selected all available children with Ct+TP– (n = 48), the smallest clinical group, then selected age, sex, and village-matched children from the remaining three clinical groups. After removing samples with low quantity or quality of DNA, and post-sequencing quality control, we studied ocular bacterial microbiome profiles of 269 children prior to azithromycin MDA by V4-16S rRNA sequencing (Table 1). C. trachomatis plasmid load, unquantifiable in Ct-/TP- and Ct-/TP+ groups, was significantly higher in Ct+/TP+ compared with Ct+/TP–. Conjunctival scarring grades were higher in Ct–/TP+ and Ct+/TP+.
Visual inspection of genera with ≥1% group-level abundance (Figure 1A) showed Ct+/TP– and Ct+/TP+ groups were similar but were separated from the Ct–/TP– groups by the presence of Chlamydia and increased abundance of Mycoplasma. The CT–/TP+ group had increased abundance of Helicobacter and a reduced abundance of “Other” genera (“Other” constituting a group consisting of each genera < 1% overall abundance). All children in which the genera Chlamydia was detected by V4-16S rRNA amplicon sequencing were C. trachomatis PCR-positive (n = 57), however, a further 24 Ct PCR-positive children had zero-read counts for Chlamydia by 16S rRNA-amplicon sequencing (Figure 1B). C. trachomatis plasmid load was significantly higher in 16S rRNA-positive individuals (p = 0.009).
Figure 1. Pre-MDA ocular microbiome by Ct and TP status. (A) Genera with ≥1% relative abundance, all genera below < 1% are grouped as “Other.” (B) Ct plasmid load, quantified by PCR, in children who had detectable Chlamydia reads by 16S rRNA PCR (+) or not (–). (C) Alpha diversity, measured by Shannon's H, by group. (D) PCA of beta diversity, measured by weighted uniFrac distance. (E) Logistic regression comparing genera abundance between indicated clinical groups, odds ratio (color of points; red >1, blue < 1) and p-value (size of points). (F) Logistic regression comparing genera prevalence (read count >0) between indicated clinical groups.
Alpha diversity, quantified by Shannon's H, was highest in Ct–/TP– children (Figure 1C), this was also true for Simpson's D. This difference was significant for Ct–/TP+ [p = 0.009, scarring-grade adjusted p (adj.p) =0.011] but not Ct+/TP– (p = 0.482, adj.p = 0.552) or Ct+/TP+ (p = 0.325, adj.p = 0.398). Ct–/TP+ alpha diversity was non-significantly lower compared with Ct+/TP– (p = 0.0790, adj.p = 0.080) and Ct+/TP+ (p = 0.113, adj.p = 0.114). Beta diversity, quantified by weighted UniFrac distance, was clearly different in Ct-/TP+ and to a lesser extent Ct+/TP+ (Figure 1D). PERMANOVA analysis found the differences in beta diversity to be significant (p = 0.001, adj.p = 0.001). Ct–/TP– and Ct+/TP– were not different.
Genera relative abundance (Figure 1E) and prevalence (Figure 1F) in Ct+/TP–, Ct–/TP+ and Ct+/TP+ were compared with the Ct–/TP– group. Additionally, we compared Ct+/TP– with Ct–/TP+. Ct+/TP– had significantly increased abundance (p = 0.034) and prevalence (p = 0.042) of Thermus, increased prevalence of Staphylococcus (p = 0.044) and decreased abundance of Corynebacterium (p = 0.040). Ct+/TP+ also had significantly increased Thermus (abundance p = 0.017, prevalence p = 0.015), as well as Haemophilus abundance (p = 0.018), with decreased abundance of multiple genera, most significantly Acinetobacter (p = 0.005), Micrococcus (p = 0.001) and Streptococcus (p = 0.016). Ct–/TP+ only had significantly increased abundance (p = 0.393 × 10−5) and prevalence of Helicobacter (p = 0.001), but had decreased abundance and prevalence of multiple genera, most significantly Acinetobacter (abundance p = 0.019) and Sphingomonas (abundance p = 0.208 × 10−3, prevalence p = 0.608 × 10−4). Comparison of Ct+/TP– and Ct–/TP+ reinforced these differences, highlighted by increased Helicobacter in Ct-/TP+ (abundance p = 0.704 × 10−3, prevalence p = 0.011) and reduced Sphingomonas (abundance p = 0.001, prevalence p = 0.004).
Seventy-nine of 269 children profiled pre-MDA had DNA available to profile their ocular microbiome 3 months post-MDA. All 79 children were C. trachomatis PCR-negative and had no evidence of clinical signs of TP post-MDA, therefore we compared post-MDA changes by pre-MDA clinical group. The four clinical groups as defined by their pre-MDA status, were still equivalent in age, sex and resident village (Table 2). There was marginal evidence of higher conjunctival scarring grade in Ct+/TP+. All 79 children received azithromycin, while community-wide treatment coverage was 68.7% (30).
Visual inspection of genera with ≥1% group-level abundance showed no clear differences between the groups at the post-MDA timepoint (Figure 2A). Alpha diversity, quantified by Shannon's H, significantly increased from pre- to post-MDA for all four groups (Figure 2B), this was also true for Simpson's D. The greatest magnitude of change was in Ct–/TP+ (linear regression coefficient [coef] = 0.602, standard error [SE] = 0.153), Ct+/TP– (coef = 0.323, SE = 0.119) and Ct+/TP+ (coef = 0.287, SE = 0.106) were similar, with the smallest increase for Ct–/TP– (coef = 0.170, SE = 0.061).
Figure 2. Post-MDA ocular microbiome by pre-MDA Ct and TP status. (A) Genera with ≥1% relative abundance, all genera below < 1% are grouped as “Other.” (B) Change in Shannon's H from pre- to post-MDA. (C) Alpha diversity, measured by Shannon's H, by group. (D) PCA of beta diversity, measured by weighted uniFrac distance. (E) Logistic regression comparing genera abundance from pre- to post-MDA for indicated clinical groups, odds ratio (color of points; red >1, blue < 1) and p-value (size of points). (F) Logistic regression comparing genera prevalence (read count >0) from pre- to post-MDA for indicated clinical groups.
Alpha diversity post-MDA was not significantly different between any of the groups (Figure 2C). Although a subset of Ct–/TP+ children still had lower alpha diversity, the same was true to a lesser extent for Ct+/TP+. Beta diversity post-MDA, quantified by weighted UniFrac distance, was similar between groups (Figure 2D). PERMANOVA analysis found slight differences in beta diversity (p = 0.033), appearing to be driven primarily by Ct+/TP+.
To identify any genera driving the increase in diversity seen after MDA, we compared relative abundance (Figure 2E) and prevalence (Figure 2F) of genera between pre- and post-MDA for each of the clinical groups. The Ct-/TP- group had significant changes in many genera post-MDA, most notably increased Acinetobacter (abundance p = 0.206 × 10−3, prevalence p = 0.006), Corynebacterium (abundance p = 0.871 × 10−3, prevalence p = 0.004) and Micrococcus (abundance p = 0.435 × 10−3, prevalence p = 0.010), with decreased Haemophilus (abundance p = 0.043, prevalence p = 0.009), Moraxella (abundance p = 0.011, prevalence p = 0.931 × 10−3) and Sphingomonas (abundance p = 0.005). Both Ct+/TP– and Ct+/TP+ had increased abundance of Corynebacterium (Ct+/TP– p = 0.038, prevalence p = 0.017), with additionally increased prevalence of Cloacibacterium (p = 0.009) in Ct+/TP–, and Micrococcus (abundance p = 0.028) and Kocuria (abundance p = 0.030, prevalence p = 0.007) in Ct+/TP+. Ct+/TP– also had decreased prevalence of Haemophilus post-MDA (p = 0.016). Sphingomonas abundance was increased in Ct–/TP+ (p = 0.035). Since increased Corynebacterium abundance has previously been found in scarring trachoma, we tested for association between 4 year scarring progression and Corynebacterium abundance. Neither change in abundance of Corynebacterium from pre- to post-MDA (p = 0.652) nor abundance post-MDA (p = 0.392) were associated with progressive scarring trachoma (Table 3).
This study profiled the ocular microbiome of children from three trachoma-endemic villages in Kilimanjaro and Arusha regions of northern Tanzania pre- and post-MDA with azithromycin. Ocular C. trachomatis infection and TP, both alone and in combination (Ct+/TP+), were associated with reduced alpha diversity and changes in individual genera. Notably Acinetobacter and Sphingomonas were reduced compared with Ct–/TP- children, with increased Thermus in both Ct+/TP– and Ct+/TP+, and increased Helicobacter in Ct–/TP+. Post-MDA there was no C. trachomatis infection or clinical signs of TP, alpha diversity was increased in all groups and ocular microbial profiles were equivalent. MDA was associated with consistent increases in Corynebacterium, Kocuria, and Micrococcus regardless of pre-MDA clinical group, as well as decreased abundance of Helicobacter and Haemophilus. Notably, MDA was associated with decreased prevalence of Sphingomonas in Ct–/TP– children post-MDA but increased in those with Ct–/TP+.
This study found that TP in the absence of C. trachomatis infection, had a greater effect on the ocular microbiome than infection alone or in combination with TP. This may be in part due to timing of sampling since TP is known to persist after clearance of infection (4). C. trachomatis-induced changes in the ocular microbiome may continue to develop, or at least be maintained, after clearance. In support of this, Ct+/TP–, Ct+/TP+, and Ct–/TP+ had similar profiles for several genera. Specifically, Acinetobacter was decreased in both Ct+/TP+ and Ct–/TP+, while Thermus was increased in both Ct+/TP– and Ct+/TP+. Alternatively, there may be non-chlamydial causes of ocular inflammation, multiple studies have reported such associations, most frequently for Streptococcus, Haemophilus, and Corynebacterium (2, 3, 6–8). We found, Ct+/TP- and Ct+/TP+ groups had increased Staphylococcus and Haemophilus, respectively, while Ct-/TP+ had increased Helicobacter. There is a growing literature of Helicobacter pylori infection associated with chronic eye diseases (31), particularly diseases in which oxidative stress is an important factor, but the direction of causation remains unknown. This is also true in our study, Helicobacter infection may be driven or facilitated by changes to the conjunctiva following C. trachomatis infection and/or TP, or longitudinal changes due to scarring trachoma, alternatively, it may itself be causing inflammation. Given that TP is consistently found to be associated with both new and increasing conjunctival scarring (9, 10), it is plausible that continued presence of non-C. trachomatis pathogens such as Helicobacter in the conjunctiva, in the absence of treatment, may drive persistent immune responses leading to scarring pathology.
Importantly, azithromycin MDA effectively cleared Helicobacter from the studied population, despite high prevalence pre-MDA. Similarly, Ct+/TP+-associated Haemophilus was decreased post-MDA, as well as Moraxella which is known to cause conjunctival inflammation (32, 33). None of the studied children had ocular C. trachomatis infection or clinical signs of TP 3 months post-MDA in addition to similar microbiome profiles, in terms of alpha and beta diversity, across all children following treatment regardless of pre-MDA clinical status. MDA also increased microbial diversity in all children, with the greatest magnitude observed in Ct-/TP+. Azithromycin MDA has been proven to be effective at clearing ocular C. trachomatis infection (11) and is clearly shown in this data and the larger study cohort, where C. trachomatis infection prevalence decreased from 11.6% pre-MDA to 1.3% post-MDA (30). However, this is the first study to determine the effects of MDA on non-chlamydial bacteria and the broader conjunctival microbiome. These results show that MDA decreases the abundance and/or prevalence of potentially pathogenic bacteria and restores a healthy microbiome in children with C. trachomatis and/or TP, without causing detrimental changes in Ct-/TP- children. MDA was associated with consistent increases in Corynebacterium and Micrococcus, both of which are primarily commensal residents of the skin and conjunctiva (34, 35). Notably, multiple studies have found an association between Corynebacterium and scarring trachoma (6, 36), with links to gene expression patterns involved in responses to the microbiota, including mucins (6). These studies were not designed to demonstrate whether increased Corynebacterium abundance was a result or cause of scarring trachoma. The finding that MDA consistently increases the abundance of Corynebacterium, regardless of pre-treatment clinical status, would suggest it is unlikely to be driving pathological changes to the conjunctiva in this population, supported by the lack of association between abundance of Corynebacterium post-MDA and progressive scarring trachoma.
In conclusion, C. trachomatis and TP alone or in combination, were both associated with pre-MDA perturbations in the ocular microbiome. Helicobacter was identified as a novel non-chlamydial correlate of TP, alongside previously defined pathogens such as Haemophilus and Staphylococcus. Azithromycin MDA cleared ocular C. trachomatis infection from the studied individuals, as well as TP. Furthermore, MDA cleared or reduced the prevalence of numerous potential pathogens, including those highlighted above, while restoring individual genera indicative of a normal, healthy ocular microbiota.
The datasets presented in this study can be found in online repositories. The names of the repository/repositories and accession number(s) can be found at: https://www.ebi.ac.uk/ena/browser/view/PRJEB46956?show=reads, PRJEB46956.
The studies involving human participants were reviewed and approved by Tanzanian National Institute for Medical Research Ethics Committee Kilimanjaro Christian Medical Centre Ethics Committee London School of Hygiene & Tropical Medicine Ethics Committee. Written informed consent to participate in this study was provided by the participants' legal guardian/next of kin.
AR, RB, DM, MB, and MH contributed to study design. HP, AR, PM, EM, AM, KM, WM, TM, TD, JH, MB, and MH contributed to data collection. HP, MB, and MH contributed to data analysis. All authors interpreted the findings, contributed to writing the manuscript, and approved the final version for publication.
This work was supported by the Wellcome Trust, grant numbers: 098481/Z/12/Z to MB and 093368/Z/10/Z to MB and MH.
We would like to acknowledge the contributions of field staff at Kilimanjaro Christian Medical Centre, lab staff at Kilimanjaro Clinical Research Institute, Moshi, Tanzania and the study participants.
The authors declare that the research was conducted in the absence of any commercial or financial relationships that could be construed as a potential conflict of interest.
All claims expressed in this article are solely those of the authors and do not necessarily represent those of their affiliated organizations, or those of the publisher, the editors and the reviewers. Any product that may be evaluated in this article, or claim that may be made by its manufacturer, is not guaranteed or endorsed by the publisher.
1. Solomon AW, Burton MJ, Gower EW, Harding-Esch EM, Oldenburg CE, Taylor HR, et al. Trachoma. Nat Rev Dis Prim. (2022) 8:32. doi: 10.1038/s41572-022-00359-5
2. Burton MJ, Hu VH, Massae P, Burr SE, Chevallier C, Afwamba IA, et al. What Is causing active trachoma? The role of nonchlamydial bacterial pathogens in a low prevalence setting. Investig Ophthalmol Vis Sci. (2011) 52:6012–7. doi: 10.1167/iovs.11-7326
3. Burr SE, Hart JD, Edwards T, Baldeh I, Bojang E, Harding-Esch EM, et al. Association between ocular bacterial carriage and follicular trachoma following mass azithromycin distribution in the Gambia. PLoS Negl Trop Dis. (2013) 7:e2347. doi: 10.1371/journal.pntd.0002347
4. Ramadhani AM, Derrick T, Macleod D, Holland MJ, Burton MJ. The relationship between active trachoma and ocular Chlamydia trachomatis infection before and after mass antibiotic treatment. PLoS Negl Trop Dis. (2016) 10:e0005080. doi: 10.1371/journal.pntd.0005080
5. Butcher RMR, Sokana O, Jack K, Macleod CK, Marks ME, Kalae E, et al. Low prevalence of conjunctival infection with Chlamydia trachomatis in a treatment-naïve trachoma-endemic region of the Solomon Islands. PLoS Negl Trop Dis. (2016) 10:e0005051. doi: 10.1371/journal.pntd.0005051
6. Pickering H, Palmer CD, Houghton J, Makalo P, Joof H, Derrick T, et al. Conjunctival microbiome-host responses are associated with impaired epithelial cell health in both early and late stages of trachoma. Front Cell Infect Microbiol. (2019) 9:297. doi: 10.1101/670711
7. Hu VH, Massae P, Weiss HA, Chevallier C, Onyango JJ, Afwamba IA, et al. Bacterial infection in scarring trachoma. Investig Ophthalmol Vis Sci. (2011) 52:2181–6. doi: 10.1167/iovs.10-5829
8. Hu VH, Macleod D, Massae P, Afwamba I, Weiss HA, Mabey DCW, et al. Non-chlamydial bacterial infection and progression of conjunctival scarring in trachoma. Investig Ophthalmol Vis Sci. (2018) 59:2339–44. doi: 10.1167/iovs.17-23381
9. Burton MJ, Rajak SN, Hu VH, Ramadhani A, Habtamu E, Massae P, et al. Pathogenesis of progressive scarring trachoma in Ethiopia and Tanzania and its implications for disease control: two cohort studies. PLoS Negl Trop Dis. (2015) 9:e0003763. doi: 10.1371/journal.pntd.0003763
10. Ramadhani AM, Derrick T, Macleod D, Massae P, Mafuru E, Malisa A, et al. Progression of scarring trachoma in Tanzanian children: a four-year cohort study. PLoS Negl Trop Dis. (2019) 13:e0007638. doi: 10.1371/journal.pntd.0007638
11. Bailey RL, Arullendran P, Whittle HC, Mabey DC. Randomised controlled trial of single-dose azithromycin in treatment of trachoma. Lancet. (1993) 342:453–6. doi: 10.1016/0140-6736(93)91591-9
12. Hong KC, Schachter J, Moncada J, Zhou Z, House J, Lietman TM. Lack of macrolide resistance in Chlamydia trachomatis after mass azithromycin distributions for trachoma. Emerg Infect Dis. (2009) 15:1088–90. doi: 10.3201/eid1507.081563
13. O'Brien KS, Emerson P, Hooper PJ, Reingold AL, Dennis EG, Keenan JD, et al. Antimicrobial resistance following mass azithromycin distribution for trachoma: a systematic review. Lancet Infect Dis. (2019) 19:e14–25. doi: 10.1016/S1473-3099(18)30444-4
14. Alkhidir AAI, Holland MJ, Elhag WI, Williams CA, Breuer J, Elemam AE, et al. Whole-genome sequencing of ocular Chlamydia trachomatis isolates from Gadarif State, Sudan. Parasites Vectors. (2019) 12:518. doi: 10.1186/s13071-019-3770-7
15. Pickering H, Chernet A, Sata E, Zerihun M, Williams CA, Breuer J, et al. Genomics of ocular Chlamydia trachomatis after 5 years of SAFE interventions for trachoma in Amhara, Ethiopia. J Infect Dis. (2020) 225:994–1004. doi: 10.1101/2020.06.07.138982
16. Bojang E, Jafali J, Perreten V, Hart J, Harding-Esch EM, Sillah A, et al. Short-term increase in prevalence of nasopharyngeal carriage of macrolide-resistant Staphylococcus aureus following mass drug administration with azithromycin for trachoma control. BMC Microbiol. (2017) 17:75. doi: 10.1186/s12866-017-0982-x
17. Coles CL, Mabula K, Seidman JC, Levens J, Mkocha H, Munoz B, et al. Mass distribution of azithromycin for trachoma control is associated with increased risk of azithromycin-resistant streptococcus pneumoniae carriage in young children 6 months after treatment. Clin Infect Dis. (2013) 56:1519–26. doi: 10.1093/cid/cit137
18. Parker EPK, Praharaj I, John J, Kaliappan SP, Kampmann B, Kang G, et al. Changes in the intestinal microbiota following the administration of azithromycin in a randomised placebo-controlled trial among infants in south India. Sci Rep. (2017) 7:9168. doi: 10.1038/s41598-017-06862-0
19. Doan T, Hinterwirth A, Worden L, Arzika AM, Maliki R, Abdou A, et al. Gut microbiome alteration in MORDOR I: a community-randomized trial of mass azithromycin distribution. Nat Med. (2019) 25:1370–6. doi: 10.1038/s41591-019-0533-0
20. Seidman J., Coles C., Levens J, Mkocha H, Munoz B, West SK. Increased resistance to azithromycin in E. coli following mass treatment for trachoma control in rural Tanzania. Am J Trop Med Hyg. (2012) 87:178.
21. Abeles SR, Jones MB, Santiago-Rodriguez TM, Ly M, Klitgord N, Yooseph S, et al. Microbial diversity in individuals and their household contacts following typical antibiotic courses. Microbiome. (2016) 4:39. doi: 10.1186/s40168-016-0187-9
22. Korpela K, Salonen A, Virta LJ, Kekkonen RA, Forslund K, Bork P, et al. Intestinal microbiome is related to lifetime antibiotic use in Finnish pre-school children. Nat Commun. (2016) 7:10410. doi: 10.1038/ncomms10410
23. Wei S, Mortensen MS, Stokholm J, Brejnrod AD, Thorsen J, Rasmussen MA, et al. Short- and long-term impacts of azithromycin treatment on the gut microbiota in children: a double-blind, randomized, placebo-controlled trial. EBioMedicine. (2018) 38:265–72. doi: 10.1016/j.ebiom.2018.11.035
24. Ramadhani AM, Derrick T, Macleod D, Massae P, Mtuy T, Jeffries D, et al. Immunofibrogenic gene expression patterns in Tanzanian children with ocular Chlamydia trachomatis infection, active trachoma and scarring: baseline results of a 4-year longitudinal study. Front Cell Infect Microbiol. (2017) 7:406. doi: 10.3389/fcimb.2017.00406
25. Dawson CR, Jones BR, Tarizzo ML, World Health Organization. Guide to Trachoma Control in Programmes for the Prevention of Blindness. World Health Organization (1981).
26. Chaima D, Pickering H, Hart JD, Burr SE, Houghton J, Maleta K, et al. Four biannual administrations of mass azithromycin treatment are associated with modest changes in the gut microbiota of rural Malawian Children. Preprints. (2020) 2020:2020100138. doi: 10.20944/preprints202010.0138.v1
27. Callahan BJ, McMurdie PJ, Rosen MJ, Han AW, Johnson AJA, Holmes SP. DADA2: high-resolution sample inference from Illumina amplicon data. Nat Methods. (2016) 13:581–3. doi: 10.1038/nmeth.3869
28. Bolyen E, Rideout JR, Dillon MR, Bokulich NA, Abnet CC, Al-Ghalith GA, et al. Reproducible, interactive, scalable and extensible microbiome data science using QIIME 2. Nat Biotechnol. (2019) 37:852–7. doi: 10.1038/s41587-019-0209-9
29. Roberts CH, Last A, Molina-Gonzalez S, Cassama E, Butcher R, Nabicassa M, et al. Development and evaluation of a next-generation digital PCR diagnostic assay for ocular Chlamydia trachomatis infections. J Clin Microbiol. (2013) 51:2195–203. doi: 10.1128/JCM.00622-13
30. Ramadhani AM, Derrick T, Macleod D, Massae P, Malisa A, Mbuya K, et al. Ocular immune responses, Chlamydia trachomatis infection and clinical signs of trachoma before and after azithromycin mass drug administration in a treatment naïve trachoma-endemic Tanzanian community. PLoS Negl Trop Dis. (2019) 13:e0007559. doi: 10.1371/journal.pntd.0007559
31. Saccà SC, Vagge A, Pulliero A, Izzotti A. Helicobacter pylori infection and eye diseases: a systematic review. Medicine. (2014) 93:e216. doi: 10.1097/MD.0000000000000216
32. Kowalski RP, Harwick JC. Incidence of Moraxella conjunctival infection. Am J Ophthalmol. (1986) 101:437–40. doi: 10.1016/0002-9394(86)90643-4
33. LaCroce SJ, Wilson MN, Romanowski JE, Newman JD, Jhanji V, Shanks RMQ, et al. Moraxella nonliquefaciens and M. osloensis are important Moraxella species that cause ocular infections. Microorganisms. (2019) 7:163. doi: 10.3390/microorganisms7060163
34. Willcox MDP. Characterization of the normal microbiota of the ocular surface. Exp Eye Res. (2013) 117:99–105. doi: 10.1016/j.exer.2013.06.003
35. Huang Y, Yang B, Li W. Defining the normal core microbiome of conjunctival microbial communities. Clin Microbiol Infect. (2016) 22:643.e7-12. doi: 10.1016/j.cmi.2016.04.008
Keywords: trachoma, Chlamydia trachomatis, azithromycin, mass drug administration, microbiome, ocular, bacteria
Citation: Pickering H, Ramadhani AM, Massae P, Mafuru E, Malisa A, Mbuya K, Makupa W, Mtuy T, Derrick T, Houghton J, Bailey RL, Mabey DCW, Burton MJ and Holland MJ (2022) The conjunctival microbiome before and after azithromycin mass drug administration for trachoma control in a cohort of Tanzanian children. Front. Public Health 10:1015714. doi: 10.3389/fpubh.2022.1015714
Received: 10 August 2022; Accepted: 30 September 2022;
Published: 17 October 2022.
Edited by:
Adwoa Asante-Poku, University of Ghana, GhanaReviewed by:
Aleksandra Inic-Kanada, Medical University of Vienna, AustriaCopyright © 2022 Pickering, Ramadhani, Massae, Mafuru, Malisa, Mbuya, Makupa, Mtuy, Derrick, Houghton, Bailey, Mabey, Burton and Holland. This is an open-access article distributed under the terms of the Creative Commons Attribution License (CC BY). The use, distribution or reproduction in other forums is permitted, provided the original author(s) and the copyright owner(s) are credited and that the original publication in this journal is cited, in accordance with accepted academic practice. No use, distribution or reproduction is permitted which does not comply with these terms.
*Correspondence: Harry Pickering, aGFycnljcGlja2VyaW5nQGdtYWlsLmNvbQ==
Disclaimer: All claims expressed in this article are solely those of the authors and do not necessarily represent those of their affiliated organizations, or those of the publisher, the editors and the reviewers. Any product that may be evaluated in this article or claim that may be made by its manufacturer is not guaranteed or endorsed by the publisher.
Research integrity at Frontiers
Learn more about the work of our research integrity team to safeguard the quality of each article we publish.