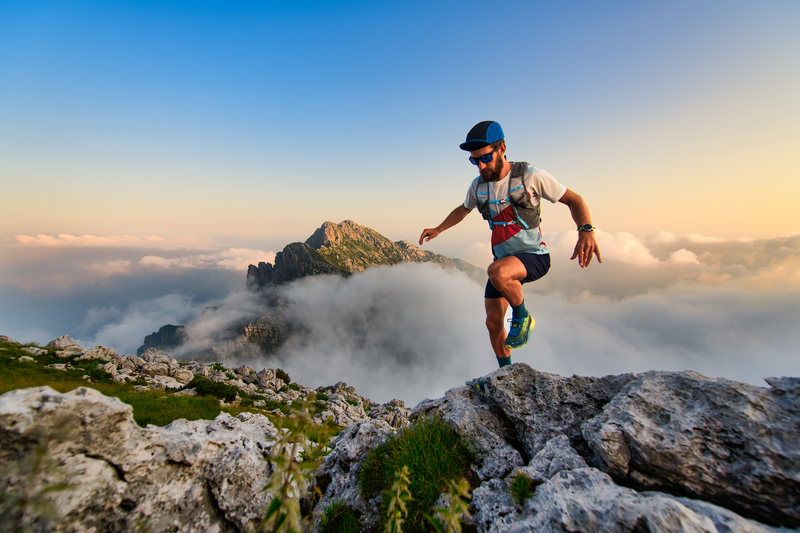
94% of researchers rate our articles as excellent or good
Learn more about the work of our research integrity team to safeguard the quality of each article we publish.
Find out more
PERSPECTIVE article
Front. Public Health , 27 August 2021
Sec. Public Mental Health
Volume 9 - 2021 | https://doi.org/10.3389/fpubh.2021.621862
This article is part of the Research Topic Early Intervention in Mood Disorders View all 9 articles
Most mental disorders emerge before the age of 25 years and, if left untreated, have the potential to lead to considerable lifetime burden of disease. Many services struggle to manage high demand and have difficulty matching individuals to timely interventions due to the heterogeneity of disorders. The technological implementation of clinical staging for youth mental health may assist the early detection and treatment of mental disorders. We describe the development of a theory-based automated protocol to facilitate the initial clinical staging process, its intended use, and strategies for protocol validation and refinement. The automated clinical staging protocol leverages the clinical validation and evidence base of the staging model to improve its standardization, scalability, and utility by deploying it using Health Information Technologies (HIT). Its use has the potential to enhance clinical decision-making and transform existing care pathways, but further validation and evaluation of the tool in real-world settings is needed.
Most mental disorders emerge before the age of 25 years and result in considerable burden of disease (1, 2). The early onset of disorders often has lifelong impacts even if the disorder is subthreshold or has remitted, so effective mental health care during this period is critical to reduce their burden (3, 4). While youth mental health services have improved access to care (5–8), many services struggle to manage high demand and have difficulty matching individuals to timely interventions due to the heterogeneity of disorders. Together these challenges perpetuate a vicious cycle between health service inefficiencies and poor treatment outcomes at high costs to the health system and society (9–12).
The increased adoption and development of HITs in mental health emphasize their growing importance in the mental health services landscape (13–15). Using HITs for assessment, triage and referral is an area of particular interest due to the scalability and standardization of technologies (16). Though, the utility of triage protocols depends on having a heuristic for allocating care appropriately, which accounts for the complexities of emerging mood and psychotic disorders among youth (17). For this the clinical staging model for youth mental health may be particularly useful as a validated and transdiagnostic framework that aims to deal with the heterogeneity of disorders based on the persistence and severity of their symptoms and syndromes (18). The application of the clinical staging model using HITs could improve its scalability and serve as a useful guiding tool for clinicians making treatment decisions and service managers deciding how to allocate resources to provide the most effective care pathways. The objective of this paper is to describe the translation of the clinical staging model into a decision support tool using HITs.
Young people experiencing mental illness vary along a continuum by factors including severity, duration of symptoms, and illness course (e.g., first episode vs. recurrent illness). Clinical staging is a framework which deals with clinical heterogeneity by using these factors to distinguish between young people in the early subthreshold phases of illness from those who have reached full threshold for major, discrete, and persistent or recurrent disorders. The clinical staging model is summarized in Table 1, and a detailed description of its development, validity and utility can be found in previous publications (18–22).
Table 1. Clinical stages for youth mental health (18).
The clinical staging model is supported by a range of clinical and neurobiological validation studies. The construct validity of the model is supported by the high rates of agreement for classifying clinical stage across independent expert raters (18), and longitudinal work supports the differential rates of progression from earlier to later stages of anxiety, mood, psychotic, or comorbid disorders (23)—a key assumption of the model. Clinical stage has also been associated with neurobiological change [e.g., white brain matter; (24), differences in neuropsychological performance (25), and sleep disruption (26)]. These objective features characterize major demarcation points in adolescent-onset mood and psychotic syndromes, which are consistent with the clinical staging models assumptions about illness progression and severity (Figure 1).
Figure 1. Transdiagnostic framework of clinical stages overlapping pathophysiological domains, and theorized magnitude of illness impacts (19).
The clinical staging model lends itself to allocating different levels of care and providing early intervention to slow or prevent the emergence and recurrence of these syndromes (27). Young people at later clinical stages will typically (though not always) require more intensive mental health care (19). While stages 1a and 1b represent subthreshold syndromes, and if left untreated, they have the potential to progress to more debilitating and persistent mental illness. Stage 1b in particular describes a relatively more severe state of attenuated syndrome that is associated with a higher risk of progressing to the development of more persistent and crystallized symptom clusters characteristic of discrete disorders (23). Young people at stage 1b are therefore recommended for more frequent clinical follow-up compared to those at stage 1a, as well as lengthier monitoring. This translates to a more intensive allocation of service resources (17). Therefore, identifying whether young people presenting to mental health services are at stage 1a, stage 1b, or beyond (Stage 2+) is of paramount importance for effective early intervention and secondary prevention.
Importantly, and as is the case with other clinical staging models (e.g., cancer), once placed on the mental illness continuum and assigned a clinical stage, young people cannot move back to an earlier stage, even in case of remission. As guidelines and standards of care are developed around the various clinical stages, this may provide a more accessible frame of reference for mental health consumers to quickly develop a shared understanding of their mental health with their clinician as well as an idea of what to expect in treatment. Ultimately, this framework has the potential to assist mental health services in resource (particularly human resource) management and in ensuring that all young people presenting to mental health services receive the most appropriate care for their personal circumstances that minimizes their risk of illness progression: “right care, first time.”
This paper extends previous work on developing a clinical decision-making protocol based on the clinical staging framework (18, 23), by presenting the basic structure of an automated version of this protocol (Algorithms 1 and 2). This automated protocol was developed as a feature of a HIT that aimed to support mental health services by (among other things) automating intake processes, including conducting a multidimensional initial assessment by collecting demographic data, administering psychometric scales, and providing real-time feedback about results. This HIT is currently used in multiple youth mental health services across Australia (28).
The multidimensional initial assessment is the first step of a proposed model for youth mental health service delivery that aims to deliver highly personalized and measurement-based care (19, 29). In this step, a comprehensive assessment spanning a wide variety of biopsychosocial health domains is conducted in order to gather enough information to allocate the appropriate intensity and type (e.g., online CBT, clinician-delivered CBT, “non-mental health” interventions focusing on returning to school) of care (27, 29). By using clinical staging principles to distill a young person's scores on key domains into a suggested clinical stage, this automated protocol aims to provide another source of information for clinicians to consider when making key treatment decisions (including shared decision-making with the young person).
We present this automated protocol in algorithm format (in pseudocode) for ease of understanding and to illustrate how the clinical staging model has been translated. The translation of this model was led by FI, SC, and IH. The process involved collating all previously published works on clinical staging criteria and supporting evidence and identifying the critical differentiating features from these publications. These differentiating features were best matched to the self-report items available in the HIT. This process involved wider consultation with youth mental health clinicians practicing in the application of clinical staging for young people, until the algorithm could be refined to maximize face validity.
We focused on distinguishing between young people at stages 1a, 1b, and 2+ at this initial phase of development as these clinical stages are the most relevant for early intervention and prevention. The protocol aims to automate two critical decision points associated with clinical staging (19, 23) and is presented in Table 2. The first is to determine whether there is any clear evidence of at least one full-threshold, major, discrete, and persistent or recurrent syndrome. This decision aims to distinguish between stage 2+ disorders and stage 1 (1a or 1b) disorders. The second is to then determine, of those among the stage 1 group, whether the syndrome is non-specific or attenuated. This decision aims to separate stage 1a and stage 1b syndromes.
Each of the evaluation criterion (text in italics) found in the algorithm 1 are underpinned by a secondary algorithm which evaluates the raw data to ascertain a result. Table 3 presents an example of a secondary algorithm used to evaluate a young person's depression results to determine the appropriate flag for algorithm 1. The examples in this table were chosen to show the versatility with which these secondary algorithms can feed into algorithm 1, and how the clinical staging model has been operationalized using scale thresholds, individual items and symptom severity in other mental health domains (e.g., mania-like experiences and psychotic-like experiences).
Multiple scales measuring the same construct, such as the Quick Inventory of Depressive Symptomatology [QIDS; (30)], or the Patient Health Questionnaire-9 [PHQ-9; (31)], which measure depressive symptoms can be added as conditions. This increases the versatility of the protocol (and the HIT) since it can be simultaneously used by multiple services employing different psychometric scales. Should they fit clinical staging criteria, responses to individual scale items can also be added to the secondary algorithms. For example, the QIDS items “Feeling slowed down” and “Feeling restless” (30) are conceptually similar to the PHQ-9 item “Moving or speaking so slowly that other people could have noticed. Or, the opposite—being so fidgety or restless that you have been moving around a lot more than usual” (31). As endorsements of these items can indicate specific functional or circadian disruptions differentially associated with clinical staging (compared to other QIDS and PHQ-9 items or total scores), adding these as flag conditions can allow more targeted identification of young people at higher risk of worsening mental health outcomes. Specifying the exact degree of endorsement (e.g., a response of “2” or “3” on a 4-point Likert scale ranging from “0” to “3” where “0” indicates no impairment) could further increase precision.
While the secondary algorithm allows for the incorporation of multiple scales measuring the same construct, they cannot be assumed to be psychometrically equivalent to each other. For example, someone who scores “Severe” on the QIDS may not score “Severe” on the PHQ-9. This problem could perhaps be solved by using Item Response Theory to identify how scores for frequently used scales measuring each biopsychosocial domain would map onto a common metric (32). This would enable the conversion of scores between psychometric scales, yet would require further validation.
Clinical staging is not meant to replace diagnosis but instead act as an adjunct to it. This means that the automated clinical staging protocol presented here, should not (and is not intended to) be taken as the sole indicator of a young person's mental health. Instead, the application of the clinical staging model using HITs provides the opportunity for it to be used as a standardized and scalable decision support tool in services. In tandem with the multidimensional intake assessment administered by the HIT, the automated clinical staging protocol provides a useful heuristic for distilling the complex assessment results into a clinical stage that can be used to help clinicians decide about the best care plan and pathway for a young person.
The ability to assess and process this information prior to the first appointment could expedite the intake process and improve service efficiencies, such as wait times for assessment and treatment, by shifting initial assessment to HITs. Thus, the first application of this tool may be to help differentiate between those who would be suitable for self-directed or low intensity services (stage 1a) from those who require higher intensity services (stage 1b and 2+). Young people at stage 1b or stage 2+ typically require more complex interventions (17) and further assessment (including neuropsychological and circadian), however young people at stage 1a, typically experience milder symptoms and have a lower risk of progressing to a discrete disorder (23). This provides services with an opportunity to direct these young people to suitable care faster by leveraging HITs and the widespread availability of brief online mental health interventions such as Internet-delivered cognitive behavioral therapy (CBT) and other app-based programs (17, 29). This has the dual benefit of ensuring that limited mental health specialist resources directly assist those most at need while also ensuring that those with a relatively lower risk of illness progression receive the appropriate supports for recovery. Importantly, these young people (stage 1a) would not be sent away from the service, but rather HITs are used here to keep the young people in contact with the service so that their progress can be monitored, and if required, they can be directed to higher intensity interventions if they do not respond to the initial treatment options.
As clinical staging operates on a consensus model, a clinical stage suggested by this protocol represents one source of information that the clinician could use when considering a young person's case. For those at higher clinical stages or not accessing self-directed or low intensity services, their cases would ideally be reviewed at a full multidisciplinary team meeting or by a highly skilled clinician to determine the right care plan and pathway (29). To facilitate this staging process, the HIT was designed to collate much of the relevant information in one place and provide an overview of the factors driving the clinical staging decision. With this information, decisions can be made about the need for further assessment, the care team required to address their needs and the overall intensity of services (i.e., type of service and treatments, minimum duration of care required, treatment frequency). This ensures that young people receive the right level of care for an appropriate amount of time to optimize treatment and prevention outcomes (27).
Ideally, in all cases, after the young person completes the multidimensional assessment a clinician would review their full intake data [including demographic information, assessment responses via a “dashboard” (28), and other contextual information collected during intake] alongside the suggested clinical stage, and record whether they agree with the automated protocols' recommendation (Figure 2). Any cases of disagreement should be referred to a multidisciplinary team for review and care allocation.
Figure 2. A (fictional) young person's suggested clinical stage from our automated protocol, and the clinician's initial assessment of clinical stage (in this case disagreeing with the protocol).
In practice, the application of this protocol extends beyond the initial care allocation process and can be applied dynamically over the course of a person's care. A young person's clinical stage should be reviewed periodically over time and HITs can be used to automatically schedule these according to a young person's clinical stage (i.e., stage 1b after 1 month). These reviews integrate further information, including continual multidimensional data from the young person (which would provide clinically valuable information on longitudinal trends) and results from further assessment (e.g., neuropsychological or circadian) if required. These results can then be used to increase confidence in the clinical stage result or identify what pieces of information are required to reduce uncertainty in the result. The dynamic use of this protocol ensures that an individual's care plan is adjusted over time to match any changes in their clinical stage or care needs.
The demand and need for youth mental health care continues to increase, which puts pressure on an under resourced system, and impacts the overall quality of care a young person receives (33). Innovations to the way services assess, treat and monitor youth mental health problems are critical to improve service efficiencies and clinical outcomes.
Young people presenting for youth mental health care typically vary in terms of the type, severity, and complexity of illness. This makes the initial assessment and care allocation process difficult (34), particularly when greater demand forces services to place individuals on waitlists before initial and standard consultations (35). The use of HITs facilitates the initial assessment of a young person's needs prior to face-to-face contact, yet a current limitation of these HITs is being able to distill a comprehensive assessment into useful information for clinical decision making. Current solutions typically heavily rely on scoring of validated measures to identify a young person's symptom severity or need for care; however these approaches do not properly account for the complexity of youth mental health.
In contrast, the automated clinical staging protocol developed and presented here extracts critical clinical information from the multidimensional assessment to provide clinically meaningful evaluation and interpretation of a young person's current mental health illness trajectory. Its proposed use to identify young people in the very early stages of illness (stage 1a) and direct them to the relevant online services for treatment, has the potential to improve current wait times in services for all young people. In addition to improving care pathways, the allocation of care plans according to clinical stage has the potential to guide the type, intensity and duration of treatments according to a young person's illness trajectory.
While, clinical staging has been validated (24–26, 36), the automated clinical staging protocol has yet to be validated within a clinical setting (i.e., does the application of these concepts result in an improved delivery of mental health care and improved mental health outcomes for young people). Hence, an important next step will be to conduct this clinical validation to determine the actual predictive power in the sample of young people attending the mental health services that use the HIT (28). Further work will need to also evaluate the applicability of this automated protocol across different culturally and linguistically diverse populations to determine its generalizability.
As health systems embrace digital health, the medicolegal, and ethical guidelines for the legal and ethical use of health data, algorithms, and clinical decision support tools more generally is critical. It is important that all young people, clinicians, and service managers have a clear understanding of what personal and health information is being collected and how this data will be shared and used. This ensures transparency about how the decision support tool works and generates an output, which will be critical to managing any biases in any algorithm or tool using data to make recommendations about treatment. For this, engaging young people and clinicians in the further design, implementation and roll out of this tool is important for its real-world applicability.
Finally, one of the challenges in the broad implementation of this work (and the wider clinical staging framework to deliver technology-enabled mental health care) is the sector's relative lack of integration with technology, such as HITs. While a consequence of COVID-19 has been the rapid digitization of mental health service provision (12), this needs to continue in order to provide a base from which to execute the described service delivery model in a sustainable and scalable manner. It is important that this continual integration [for example implementing the capability for long term monitoring; (37)] always keeps mental health consumers at the center of their care and promotes their best interests.
Here, we have presented the translation of the clinical staging model into a decision support tool using HITs and its potential use in youth mental health services. The automated clinical staging protocol leverages the clinical validation and evidence base of the staging model to improve its standardization, scalability, and utility by deploying it using HITs. Its use has the potential to enhance clinical decision-making and transform existing care pathways, but further validation and evaluation of the tool in real-world settings is needed.
The original contributions presented in the study are included in the article/supplementary material, further inquiries can be directed to the corresponding author/s.
FI and VC wrote the manuscript. FI, SC, VC, and HY developed the automated staging protocol. TD, ES, and IH supervised the work and provided scientific leadership. All authors assisted with manuscript drafting and approved the final manuscript.
This research was funded by the Australian Government Department of Health.
IH was an inaugural Commissioner on Australia's National Mental Health Commission (2012–2018). He is the Co-Director, Health and Policy at the Brain and Mind Centre (BMC) University of Sydney. The BMC operates an early-intervention youth services at Camperdown under contract to headspace. He is the Chief Scientific Advisor to, and a 5% equity shareholder in, InnoWell Pty Ltd. InnoWell was formed by the University of Sydney (45% equity) and PwC (Australia; 45% equity) to deliver the $30 M Australian Government-funded Project Synergy (2017–2020; a 3-year program for the transformation of mental health services) and to lead transformation of mental health services internationally through the use of innovative technologies. ES is the Medical Director, Young Adult Mental Health Unit, St Vincent's Hospital Darlinghurst, Discipline Leader of Adult Mental Health, School of Medicine, University of Notre Dame, Principal Research Fellow, Brain and Mind Centre, The University of Sydney and Consultant Psychiatrist. She has received honoraria for educational seminars related to the clinical management of depressive disorders supported by Servier and Eli-Lilly pharmaceuticals. She has participated in a national advisory board for the antidepressant compound Pristiq, manufactured by Pfizer. She was the National Coordinator of an antidepressant trial sponsored by Servier.
The remaining authors declare that the research was conducted in the absence of any commercial or financial relationships that could be construed as a potential conflict of interest.
All claims expressed in this article are solely those of the authors and do not necessarily represent those of their affiliated organizations, or those of the publisher, the editors and the reviewers. Any product that may be evaluated in this article, or claim that may be made by its manufacturer, is not guaranteed or endorsed by the publisher.
This research was conducted through Project Synergy (2017–2020) which is an Australian Government Department of Health-funded initiative ($30 million) that is being delivered by InnoWell Pty Ltd—a joint venture between the University of Sydney and PwC (Australia).
1. Jones PB. Adult mental health disorders and their age at onset. Br J Psychiatry. (2013) 202:s5–10. doi: 10.1192/bjp.bp.112.119164
2. Patel V, Flisher AJ, Hetrick S, McGorry P. Mental health of young people: a global public-health challenge. Lancet. (2007) 369:1302–13. doi: 10.1016/S0140-6736(07)60368-7
3. Gibb SJ, Fergusson DM, Horwood LJ. Burden of psychiatric disorder in young adulthood and life outcomes at age 30. Br J Psychiatry. (2010) 197:122–7. doi: 10.1192/bjp.bp.109.076570
4. Goodman A, Joyce R, Smith JP. The long shadow cast by childhood physical and mental problems on adult life. Proc Natl Acad Sci USA. (2011) 108:6032–7. doi: 10.1073/pnas.1016970108
5. Hamilton BA, Naismith SL, Scott EM, Purcell S, Hickie IB. Disability is already pronounced in young people with early stages of affective disorders: data from an early intervention service. J Affect Disord. (2011) 131:84–91. doi: 10.1016/j.jad.2010.10.052
6. O'Dea B, Glozier N, Purcell R, McGorry PD, Scott J, Fields KL, et al. A cross-sectional exploration of the clinical characteristics of disengaged (NEET) young people in primary mental healthcare. BMJ Open. (2014) 4:e006378. doi: 10.1136/bmjopen-2014-006378
7. Rickwood D, Telford NR, Parker AG, Tanti CJ, McGorry PD. Headspace—Australia's innovation in youth mental health: who are the clients and why are they presenting? Med J Aust. (2014) 200:108–11. doi: 10.5694/mja13.11235
8. Scott J, Scott EM, Hermens DF, Naismith SL, Guastella AJ, White D, et al. Functional impairment in adolescents and young adults with emerging mood disorders. Br J Psychiatry. (2014) 205:362–8. doi: 10.1192/bjp.bp.113.134262
9. Commission NMH. Contributing Lives, Thriving Communities: Report of the National Review of Mental Health Programmes and Services. Sydney, NSW. (2014).
10. Kilbourne AM, Fullerton C, Dausey D, Pincus HA, Hermann RC. A framework for measuring quality and promoting accountability across silos: the case of mental disorders and co-occurring conditions. Qual Saf Health Care. (2010) 19:113–6. doi: 10.1136/qshc.2008.027706
11. Vigo D, Thornicroft G, Atun R. Estimating the true global burden of mental illness. Lancet Psychiatry. (2016) 3:171–8. doi: 10.1016/S2215-0366(15)00505-2
12. Wind TR, Rijkeboer M, Andersson G, Riper H. The COVID-19 pandemic: the 'black swan' for mental health care and a turning point for e-health. Inter Interv. (2020) 20:100317. doi: 10.1016/j.invent.2020.100317
13. Fleming TM, de Beurs D, Khazaal Y, Gaggioli A, Riva G, Botella C, et al. Maximizing the impact of e-therapy and serious gaming: time for a paradigm shift. Front Psychiatry. (2016) 7:65. doi: 10.3389/fpsyt.2016.00065
14. Hickie IB, Davenport TA, Burns JM. Project synergy: co-designing technology-enabled solutions for Australian mental health services reform. Med J Aust. (2019) 211(Suppl. 7):S3–39. doi: 10.5694/mja2.50349
15. Price M, Yuen EK, Goetter EM, Herbert JD, Forman EM, Acierno R, et al. mHealth: a mechanism to deliver more accessible, more effective mental health care. Clin Psychol Psychotherapy. (2014) 21:427–36. doi: 10.1002/cpp.1855
16. Hardy JA, Weatherford RD, Locke BD, DePalma NH, D'Iuso NT. Meeting the demand for college student concerns in college counseling centers: evaluating a clinical triage system. J College Stud Psychother. (2011) 25:220–40. doi: 10.1080/87568225.2011.581929
17. Cross SPM, Hermens DF, Hickie IB. Treatment patterns and short-term outcomes in an early intervention youth mental health service. Early Interv Psychiatry. (2016) 10:88–97. doi: 10.1111/eip.12191
18. Hickie IB, Scott EM, Hermens DF, Naismith SL, Guastella AJ, Kaur M, et al. Applying clinical staging to young people who present for mental health care. Early Interv Psychiatry. (2013) 7:31–43. doi: 10.1111/j.1751-7893.2012.00366.x
19. Hickie IB, Scott EM, Cross SP, Iorfino F, Davenport TA, Guastella AJ, et al. Right care, first time: a highly personalised and measurement-based care model to manage youth mental health. Med J Austr. (2019) 211:S42–6. doi: 10.5694/mja2.50383
21. Patel V, Saxena S, Lund C, Thornicroft G, Baingana F, Bolton P, et al. The lancet commission on global mental health and sustainable development. Lancet. (2018) 392:1553–98. doi: 10.1016/S0140-6736(18)31612-X
22. Shah JL, Scott J, McGorry PD, Cross SP, Keshavan MS, Nelson B, et al. Transdiagnostic clinical staging in youth mental health: a first international consensus statement. World Psychiatry. (2020) 19:233–42. doi: 10.1002/wps.20745
23. Iorfino F, Scott EM, Carpenter JS, Cross SP, Hermens DF, Killedar M, et al. Clinical stage transitions in persons aged 12 to 25 years presenting to early intervention mental health services with anxiety, mood, and psychotic disorders. JAMA psychiatry. (2019) 76:1167–75. doi: 10.1001/jamapsychiatry.2019.2360
24. Lagopoulos J, Hermens DF, Hatton SN, Battisti RA, Tobias-Webb J, White D, et al. Microstructural white matter changes are correlated with the stage of psychiatric illness. Transl Psychiatry. (2013) 3:e248. doi: 10.1038/tp.2013.25
25. Tickell AM, Lee RSC, Hickie IB, Hermens DF. The course of neuropsychological functioning in young people with attenuated vs discrete mental disorders. Early Interv Psychiatry. (2019) 13:425–33. doi: 10.1111/eip.12499
26. Scott EM, Robillard R, Hermens DF, Naismith SL, Rogers NL, Ip TKC, et al. Dysregulated sleep–wake cycles in young people are associated with emerging stages of major mental disorders. Early Interv Psychiatry. (2016) 10:63–70. doi: 10.1111/eip.12143
27. Sawrikar V, Stewart E, LaMonica HM, Iorfino F, Davenport TA, Cross S, et al. Using staged care to provide “right care first time” to people with common affective disorders. Psychiatr Serv. (2021) 72:202000145. doi: 10.1176/appi.ps.202000145
28. Davenport TA, LaMonica HM, Whittle L, English A, Iorfino F, Cross S, et al. Validation of the InnoWell platform: protocol for a clinical trial. JMIR Res Protoc. (2019) 8:e13955. doi: 10.2196/13955
29. Cross SPM, Hermens DF, Scott EM, Ottavio A, McGorry PD, Hickie IB. A clinical staging model for early intervention youth mental health services. Psychiatr Serv. (2014) 65:939–43. doi: 10.1176/appi.ps.201300221
30. Rush AJ, Trivedi MH, Ibrahim HM, Carmody TJ, Arnow B, Klein DN, et al. The 16-Item quick inventory of depressive symptomatology (QIDS), clinician rating (QIDS-C), and self-report (QIDS-SR): a psychometric evaluation in patients with chronic major depression. Biol Psychiatry. (2003) 54:573–83. doi: 10.1016/S0006-3223(02)01866-8
31. Kroenke K, Spitzer RL, Williams JBW. The PHQ-9: validity of a brief depression severity measure. J Gen Intern Med. (2001) 16:606–13. doi: 10.1046/j.1525-1497.2001.016009606.x
32. Batterham PJ, Sunderland M, Slade T, Calear AL, Carragher N. Assessing distress in the community: psychometric properties and crosswalk comparison of eight measures of psychological distress. Psychol Med. (2018) 48:1316–24. doi: 10.1017/S0033291717002835
33. Carlo AD, Barnett BS, Unützer J. Harnessing collaborative care to meet mental health demands in the era of COVID-19. JAMA Psychiatry. (2021) 78:355–6. doi: 10.1001/jamapsychiatry.2020.3216
34. Sands N, Elsom S, Marangu E, Keppich-Arnold S, Henderson K. Mental health telephone triage: managing psychiatric crisis and emergency. Perspect Psychiatr Care. (2013) 49:65–72. doi: 10.1111/j.1744-6163.2012.00346.x
35. Thomas KA, Schroder AM, Rickwood DJ. A systematic review of current approaches to managing demand and waitlists for mental health services. Mental Health Rev J. (2020) 26:1–17. doi: 10.1108/mhrj-05-2020-0025
36. Hickie IB, Carpenter JS, Iorfino F, Scott E, Cross S, Hermens DF. The utility of clinical staging in youth mental health settings: neurobiological and longitudinal data from sydney-based studies of transdiagnostic cohorts. In: Hickie IB, McGorry PD, editors. Clinical Staging in Psychiatry: Making Diagnosis Work for Research and Treatment. Cambridge: Cambridge University Press (2019). p. 81–102.
Keywords: mental health, eHealth, health informatics, clinical decision support, health information technologies
Citation: Iorfino F, Cheng VWS, Cross SP, Yee HF, Davenport TA, Scott EM and Hickie IB (2021) Right Care, First Time: Developing a Theory-Based Automated Protocol to Help Clinically Stage Young People Based on Severity and Persistence of Mental Illness. Front. Public Health 9:621862. doi: 10.3389/fpubh.2021.621862
Received: 27 October 2020; Accepted: 31 July 2021;
Published: 27 August 2021.
Edited by:
Edwin De Beurs, Leiden University, NetherlandsReviewed by:
June S. L. Brown, King's College London, United KingdomCopyright © 2021 Iorfino, Cheng, Cross, Yee, Davenport, Scott and Hickie. This is an open-access article distributed under the terms of the Creative Commons Attribution License (CC BY). The use, distribution or reproduction in other forums is permitted, provided the original author(s) and the copyright owner(s) are credited and that the original publication in this journal is cited, in accordance with accepted academic practice. No use, distribution or reproduction is permitted which does not comply with these terms.
*Correspondence: Frank Iorfino, ZnJhbmsuaW9yZmlub0BzeWRuZXkuZWR1LmF1
†These authors share first authorship
Disclaimer: All claims expressed in this article are solely those of the authors and do not necessarily represent those of their affiliated organizations, or those of the publisher, the editors and the reviewers. Any product that may be evaluated in this article or claim that may be made by its manufacturer is not guaranteed or endorsed by the publisher.
Research integrity at Frontiers
Learn more about the work of our research integrity team to safeguard the quality of each article we publish.