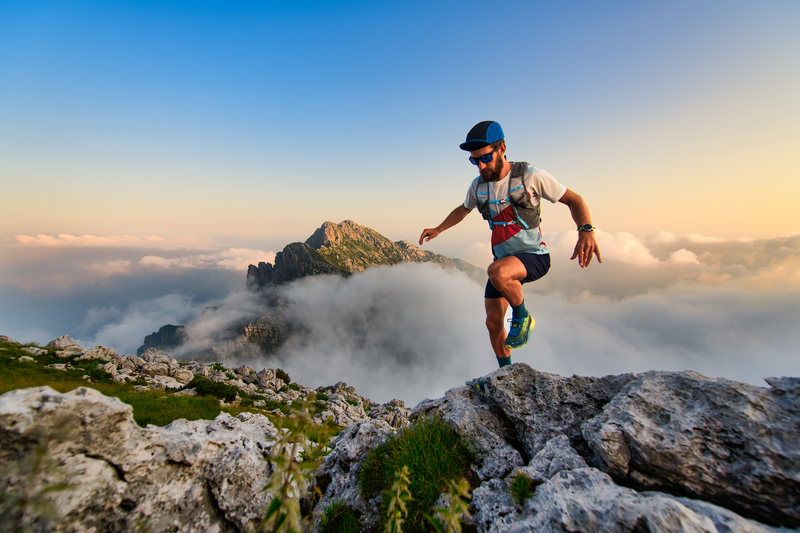
95% of researchers rate our articles as excellent or good
Learn more about the work of our research integrity team to safeguard the quality of each article we publish.
Find out more
MINI REVIEW article
Front. Public Health , 22 May 2017
Sec. Digital Public Health
Volume 5 - 2017 | https://doi.org/10.3389/fpubh.2017.00110
This article is part of the Research Topic Real-time Point-of-Care Quantitative Biomarkers: Trekkie Hype or the Future of Personalized Medicine? View all 6 articles
The lack of standard tools and methodologies and the absence of a streamlined multimarker approval process have hindered the translation rate of new biomarkers into clinical practice for a variety of diseases afflicting humankind. Advanced novel technologies with superior analytical performance and reduced reagent costs, like the programmable bio-nano-chip system featured in this article, have potential to change the delivery of healthcare. This universal platform system has the capacity to digitize biology, resulting in a sensor modality with a capacity to learn. With well-planned device design, development, and distribution plans, there is an opportunity to translate benchtop discoveries in the genomics, proteomics, metabolomics, and glycomics fields by transforming the information content of key biomarkers into actionable signatures that can empower physicians and patients for a better management of healthcare. While the process is complicated and will take some time, showcased here are three application areas for this flexible platform that combines biomarker content with minimally invasive or non-invasive sampling, such as brush biopsy for oral cancer risk assessment; serum, plasma, and small volumes of blood for the assessment of cardiac risk and wellness; and oral fluid sampling for drugs of abuse testing at the point of need.
Despite the abundance of biomarker discovery data, presently absent are the tools to retrieve and the processes to translate these initial findings into clinically relevant settings.
It has been estimated that the regulatory approval rate of new protein analytes in the United States over the past 15 years has remained steady, averaging a low rate of 1.5 new proteins per year despite tens of thousands of scientific articles describing advances in cancer and cardiac biomarkers (1, 2). Unless Food and Drug Administration (FDA) approval is secured, these biomarker discoveries remain in research settings and never enter widespread US clinical practice. Part of the problem here is the fact that distinct phases of the process, such as biomarker discovery, validation, and clinical implementation, remain disjointed. In fact, in the majority of the cases, each phase is completed by different groups utilizing different instruments and, even, different “standards.”
Likewise, up to this juncture, there has been no effective vehicle or technology platform to validate biomarkers in large studies and to ultimately drive these biomarkers to FDA approval and clinical practice. Thus, most modern bioscience research efforts remain largely decoupled from real-world clinical practice, for both the biomarkers and the devices that measure them, despite huge investments in translational research programs.
The typical device development process, whether from academia, national labs, or the industrial sector, is a succession of lengthy steps that often happen in a linear, suboptimal, and disjointed manner. The process is lengthy and inefficient, draining precious resources and momentum out of venture capital and federal funding, alike.
A case in point for the dismal translation rate of new medical tests into real-world practice is extracted from an analysis by Schully and coworkers (3) of the fiscal year 2007 extramural grant portfolio of the National Cancer Institute as well as cancer genetic research articles published in 2007. The group classified both funded grants and publications as follows: T0 as discovery research; T1 as research to develop a candidate health application (e.g., device or therapy); T2 as research that evaluates a candidate application and develops evidence-based recommendations; T3 as research that assesses how to integrate an evidence-based recommendation into cancer care and prevention; and T4 as research that assesses health outcomes and population impact. They found that only 1.8% of the grant portfolio and 0.6% of the published literature were T2 research or beyond.
Similar problems exist for other major disease areas such as cardiovascular disease (CVD), autoimmune disease, and many more. Importantly, the translation of new medical tests is also burdened by the plethora of disparate disciplines in the lab-on-a-chip/point-of-care (POC)/medical microdevice communities. Here, critical to the ultimate goal is the bridge between bioscience and microfabrication, two areas that up to this juncture have remained largely separate.
There is now a tremendous opportunity for new technologies to greatly enhance bench-to-bedside translation. Multiclass, multiplexed medical microdevices with enhanced throughput capabilities may help facilitate the translation of biomarkers into real-world practice (4). Integrating these new platforms with mobile health (mHealth) applications, an area that remained strikingly silent until today and lacked attention from the biomarker research community, may capitalize on the fact that an estimated 70% of all medical decisions regarding a patient’s diagnosis, treatment, hospital admission, and discharge are based on laboratory test results (Figure 1A). As health-care reform focuses on diagnosis and early intervention, timely and accurate lab quality mHealth results at the POC promise a higher quality of patient care and better management of downstream costs (5–7).
Figure 1. (A) Finding a way to provide patients and physicians the ability to cull biomarker information via diagnostic tests and then using the processing power and rich interface of smart phones opens the door to a more empowered patient and a more data savvy clinician. (B) The point-of-care (POC) programmable bio-nano-chip includes a universal analyzer with functional integrated mechanical/optical interfaces and flexible microchip architecture. An injection-molded, “credit-card”-sized cartridge encloses either a bead array or a membrane, where complex immunoassays or cellular analyses are performed, respectively. (C) Nanomaterials and microelectronics have been combined and adapted for the practical implementation of two classes of mini-sensors (membrane for cellular analyses and bead array for soluble target analyses) that read out with high-performance, yet affordable, imaging systems. These systems promise POC capabilities of tests traditionally completed by larger and more expensive systems confined in the lab, without sacrificing performance.
The use of new medical POC microdevices with minimally invasive or non-invasive sampling methods has the potential to expand in vitro diagnostics in traditional and non-traditional settings.
Pioneer work by Whitesides (8) in the basic sciences defined the ideal coatings, materials, and designs for the development of microfluidic channels and manipulations of biological fluids, while Quake’s group (9) advanced the “large-scale integration” of microfluidics, analogous to the electronics field. Other groups, such as those of Mirkin (10), Wang (11), and Heath (12), measured diverse sample types and created a variety of assembly types by using precious metal nanoparticles, nanowires, and magnetic techniques, respectively. Advancements by Sia (13) via microelectromechanical systems and by Singh (14) using chip-based separation and quantitation benefited integration of such systems. Others have extended their integrated approaches into the rapid, multiplexed detection of analytes, such as toxins and other biothreats (15), while Walt’s work (16) with electronic noses used arrays of optical fibers as the underlying infrastructure for biological sensing systems. Finally, researchers in the Toner group have explored a number of novel methods for the isolation and enumeration of lymphocytes, erythrocytes, and circulating tumor cells (17, 18).
These medical microdevices have recently reached the tipping point where they are starting to exceed the analytical performance of remote laboratory systems and do so with reduced cost. This exciting new trend overcomes previous limitations for POC technologies with respect to their higher cost and inferior performance, compared to macroscopic, laboratory-confined instrumentation. Likewise, these innovative POC technologies have the potential to serve as tools for more efficient translation of discovered biomarkers.
The p-BNC (Figure 1B) is one such platform technology that combines powerful machine-learning algorithms with unique chemsensing and biosensing capabilities (19–36).
The p-BNC includes a universal analyzer with functional integrated mechanical/optical interfaces and flexible microchip architecture. An injection-molded, “credit card”-sized cartridge encloses either a bead array or a membrane, where complex immunoassays or cellular analyses are performed, respectively. The cartridge is composed of a network of microfluidic components that ensure the complete transfer and processing of biological samples to provide quantitative information of the targeted biomarkers. The sample introduction requirements are consistent with the use of saliva, serum, plasma, and small volumes of blood for the assessment of cardiac risk and wellness or tissue brush samples that can be directly administered into the sample introduction port. Reagents are stored dry on a conjugate pad embedded within the biochip and are dissolved as needed through the release of prepacked buffer contained in biochip-integrated pouches. Here, all processing steps are conducted within the microfluidic network of the p-BNC via actuation inside the analyzer without human intervention. These features eliminate the need for external fluidics such as pumps, tubing, and connectors.
The p-BNC system combines and adapts nanomaterials and microelectronics to create two distinct classes of minisensors that read out with high-performance, yet affordable, imaging systems. Combined, the two p-BNC sensor ensembles form a modular platform compatible with possibly the largest and most diverse analyte portfolios available to date (Figure 1C). Importantly, the performance metrics of these miniaturized sensor systems have been shown to correlate closely with established macroscopic gold standard methods, making them suitable for use as subcomponents of highly functional detection systems for the analysis of complex fluid samples. These efforts remain unique in terms of functional p-BNC methods having a demonstrated capacity to meet or exceed the analytical characteristics (sensitivity, selectivity, assay variance, and limit of detection) of mature macroscopic instrumentation (19–36).
This platform is intended to be used outside of the traditional laboratory environments, such as in the ambulance, the emergency room, the intensive care unit, or cancer screening facilities at reduced cost with enhanced analytical and clinical performance. The rich data streams derived from this “Internet of Things”-enabled biosensing platform can be managed with a novel database, and mHealth tools can be integrated to enroll patients, manage and collect their data, and provide precision feedback to health-care providers and patients, alike.
The p-BNC technology and methods were developed and validated in six major clinical studies funded by the National Institute of Dental and Craniofacial Research division of the National Institutes of Health, the Cancer Prevention Research Institute of Texas, and the United Kingdom’s Home Office Centre of Applied Science and Technology. These studies yielded innovations in application areas, including cardiac wellness, oral cancer screening, and drugs of abuse testing. These programs are described below.
Cardiovascular disease, including coronary artery disease, remains the leading cause of death and a major contributor to health-care costs worldwide. While it is highly desirable to have tools suitable for early detection for effective management of CVD, the modern cardiac diagnostics area tends to focus on late-stage disease syndromes like acute myocardial infarction (AMI) and heart failure (HF) (37, 38). Lacking today are tools to manage patients from holistic cardio perspective. The authors have developed recently the Cardiac ScoreCard test, a series of models based on a multivariate index assay with the potential to assist in the diagnosis and prognosis of multiple CVD progression levels (39, 40). The approach implements three lasso penalized logistic regression models in the areas of cardiac wellness, AMI, and HF. Validated through a major clinical study involving 1,050 subjects, the Cardiac ScoreCard test uses a panel of up to 16 biomarkers to yield a number between 0 and 100 that is indicative of overall cardiac wellness (Figure 2A). The score is literally interpreted as “the probability of being healthy,” as derived from a population of recruited healthy individuals and non-case chest pain patients. The Cardiac ScoreCard applies a time- and cost-effective approach in an attempt to develop predictive models for diagnosis and assessment of cardiac wellness, AMI, and HF. This expanded panel has redundancy that may eliminate blind spots in current diagnosis and prognosis and help establish useful baseline measurements for future reference that could assist in the development of more powerful learning algorithms.
Figure 2. Recent application areas of the programmable bio-nano-chip (p-BNC) include the Cardiac ScoreCard (A), the oral cancer test (B), and tests for drugs of abuse (C), all of which are conducted with minimally invasive or non-invasive sampling. (A) Results of the cardiac wellness model showing receiver operating characteristic curves for the Framingham 10-year cardiovascular disease risk score (gold standard), biomarker model, and the ScoreCard. The Cardiac ScoreCard model shows superior performance for discriminating high- and low-risk subjects, demonstrating the importance of using both traditional risk factors and biomarker measurements in predicting cardiac wellness. (B) In the oral cancer area, the p-BNC platform was adapted to service a “cytology on a chip” application whereby cell and nuclear morphology along with cancer-associated biomarkers are measured using non-invasive brush biopsy sampling. (C) For drug testing, the bead-based p-BNC platform was programmed to host a fully quantitative, competitive type of immunoassay, whereby fluorescent signal on the bead was inversely proportional to the drug levels in the oral fluid sample processed.
Oral squamous cell carcinoma (OSCC) is a significant health problem afflicting approximately 400,000 people globally each and every year. In the United States alone, greater than 45,000 new cases and nearly 8,650 deaths are estimated in 2015, representing approximately 4% of all cancers in men and 3% in women (41). The significant morbidity and mortality associated with OSCC is often attributed to the advanced disease stage of many OSCCs when the initial identification and biopsy are done. Despite advances in surgical procedures and treatment, the long-term prognosis for patients with OSCC remains poor with a 5-year survival rate at approximately 64%. This prognosis is among the lowest for all cancers. However, the survival rate increases dramatically to 83% when this abnormality is detected in its early stage. Unfortunately, only 32–47% of oral cancer cases are detected in the early stages. In addition to improving survival, early detection of oral cancers can identify the disease when less aggressive, non-disfiguring, less toxic, and less costly therapies are required, underscoring the need for new diagnostic methods that are able to identify early-stage disease. In the essential oral cancer area, the p-BNC platform was adapted to service a “cytology on a chip” application whereby cell and nuclear morphology along with expression of cancer-associated biomarkers are measured using non-invasive brush biopsy sampling (42–45). The 999-patient, 4-site international clinical trial, sponsored by the National Institutes of Health Grand Opportunity program, has led to the establishment of one of the largest oral cytology databases ever for potentially malignant oral lesions (PMOLs). Unlike existing adjunctive diagnostic aids, the new p-BNC data have fostered a robust capacity to assess in a quantitative manner the level of disease progression for PMOLs using a non-invasive sampling method combined with the automated chip-based sampling modality. This new approach exceeds in a major way the level of quantitation possible with existing adjunctive oral cancer diagnostic aids (45). From these careful studies of model performance, it is clear that the chip-based image approaches are effective for classifying patients in six diagnostic categories (Figure 2B). Sensitivity values in excess of 90% with specificity values around 75% have been achieved for this prospective trial exceeding capabilities of existing commercial approaches. Over 200 cellular features related to biomarker expression, nuclear parameters and cellular morphology were recorded per cell. By cataloging an average of 2,000 cells per patient, these efforts resulted in nearly 13 million indexed objects. Binary “low-risk”/“high-risk” models yielded AUC values of 0.88 and 0.84 for training and validation models, respectively, with an accompanying difference in sensitivity + specificity of 6.2%. In terms of accuracy, this model accurately predicted the correct diagnosis approximately 70% of the time, compared to the 69% initial agreement rate of the pool of expert pathologists. Key parameters identified in these models included cell circularity, Ki67 and EGFR expression, nuclear–cytoplasmic ratio, nuclear area, and cell area. This chip-based approach yields objective data that can be leveraged for diagnosis and management of patients with PMOL as well as uncovers new molecular-level insights behind cytological differences across the OED spectrum.
Current onsite detection methods for drugs of abuse involve invasive sampling of blood and urine specimens, or collection of oral fluid, followed by qualitative screening tests using immunochromatographic cartridges. The instrumentation used by remote laboratories to provide subsequent confirmation and quantitative assessment of a presumptive positive is expensive and decoupled from the initial sampling, making the current drug-screening program inefficient and costly. In contrast, the p-BNC assay methodology (applied recently for the detection of 12 drugs of abuse initially in spiked buffered samples and, ultimately, in non-invasive oral fluid specimen collected from consented volunteers) is easy to use, rapid, low cost, multiplexed, and fully quantitative (Figure 2C) (46, 47).
Customized macros developed and optimized for the automated analysis of drug-specific bead-based assays serve to determine the exact bead location on the chip, followed by their respective bead-specific assignments and to extract bead data using “regional pixel extraction analysis” strategies that are automatically applied for the generation of dose response curves and used for the measurement of the various drug concentrations in unknown samples. Analysis of each bead within the group, for each of the drug tests reported here, at each exposure time was initiated within the macro through line profile and circular area of interest routines for data extraction (46, 47).
Furthermore, the system’s superior analytical performance allows visibility to time course of select drug and metabolite profiles in oral fluids that, when combined with concentration measurements from this and prior impairment studies, reveals additional information about drug-induced impairment. This chip-based p-BNC detection modality promises to serve as a valuable tool in law enforcement roadside drug testing and for a variety of other settings, including outpatient and inpatient drug rehabilitation centers, emergency rooms, prisons, schools, and the workplace.
Simple to use, rapid, minimally invasive or non-invasive, accurate, and sensitive technologies, such as the p-BNC, that offer advanced screening, diagnosis, and monitoring of diseases in a variety of settings (e.g., physicians’ and dentists’ offices, pharmacies, and at home) promise to overcome major past limitations for POC technologies, such as cost and lack of sensitivity.
By integrating experts in clinical unmet needs and market assessment, clinical research, device development and testing, regulatory process and commercialization, and outreach for education and training, there is promise to improve success rates for the translation of medical devices and of the biomarker tests for which these technologies host.
JM: principal investigator, inventor of programmable bio-nano-chip technology. NC: senior scientist in McDevitt lab, director of assay development, and drug study project manager. MM: developed CardiacScoreCard and latest iteration of portable analyzer and cardiac study project manager. TA: scientist at SensoDx and oral cancer study manager. GS: p-BNC cartridge development engineering lead.
JM is one of the founders of SensoDx and serves as the chief scientific officer for the same. The other authors declare no conflict of interest.
This work was supported in part by the National Institutes of Health (NIH) through the National Institute of Dental and Craniofacial Research (award numbers 5U01 DE017793 and 5RC2DE020785), the Cancer Prevention Research Institute of Texas (award number RP101382), and the United Kingdom’s Home Office Centre for Applied Science and Technology (CAST) (Grant Number 7165721). The content of this article is solely the responsibility of the authors and does not necessarily represent or reflect the official views of the NIH or the government and other sponsors.
1. Anderson L. Candidate-based proteomics in the search for biomarkers of cardiovascular disease. J Physiol (2005) 563:23–60. doi:10.1113/jphysiol.2004.080473
2. Boja ES, Jortani SA, Ritchie J, Hoofnagle AN, Tezak Z, Mansfield E, et al. The journey to regulation of protein-based multiplex quantitative assays. Clin Chem (2011) 57:560–7. doi:10.1373/clinchem.2010.156034
3. Schully SD, Benedicto CB, Gillanders EM, Wang SS, Khoury MJ. Translational research in cancer genetics: the road less traveled. Public Health Genomics (2011) 14:1–8. doi:10.1159/000272897
4. Hoofnagle AN, Aebersold R, Anderson NL, Felsenfeld A, Liebler DC. Painting a moving picture: large-scale proteomics efforts and their potential for changing patient care. Clin Chem (2011) 57:1357–60. doi:10.1373/clinchem.2010.158311
5. World Development Indicators. (2014). Available from: www.data.worldbank.org
6. Health Care Employment Projections: An Analysis of Bureau of Labor Statistics Occupational Projections 2010-2020. (2012). Available from: https://www.healthit.gov/sites/default/files/chws_bls_report_2012.pdf
7. Hospitals & Health Networks. Clinical Management: Hospital Labs Go Under the Microscope. (2014). Available from: www.hhnmag.com
8. Whitesides GM. The origins and the future of microfluidics. Nature (2006) 442:368–73. doi:10.1038/nature05058
9. Thorsen T, Maerkl SJ, Quake SR. Microfluidic large-scale integration. Science (2002) 298:580–4. doi:10.1126/science.1076996
10. Goluch ED, Nam J-M, Georganopolou DG, Chiesl TN, Shaikh KA, Ryu KS, et al. A bio-barcode assay for on-chip attomolar-sensitivity protein detection. Lab Chip (2006) 6:1293–9. doi:10.1039/b606294f
11. Osterfield SJ, Yu H, Gaster RS, Caramuta S, Xu L, Han S-J, et al. Multiplex protein assays based on real-time magnetic nanotag sensing. Proc Natl Acad Sci U S A (2008) 105:20637–40. doi:10.1073/pnas.0810822105
12. Qin L, Vermesh O, Shi Q, Heath JR. Self-powered microfluidic chips for multiplexed protein assays from whole blood. Lab Chip (2009) 9:2016–20. doi:10.1039/b821247c
13. Chin CD, Linder V, Sia SK. Lab-on-a-chip devices for global health: past studies and future opportunities. Lab Chip (2007) 7:41–57. doi:10.1039/B611455E
14. Srivastava N, Brennan JS, Renzi RF, Wu M, Branda SS, Singh AK, et al. Fully integrated microfluidic platform enabling automated phosphoprofiling of macrophage response. Anal Chem (2009) 81:3261–9. doi:10.1021/ac8024224
15. Kim JS, Anderson GP, Erickson JS, Golden JP, Nasir M, Ligler FS. Multiplexed detection of bacteria and toxins using a microflow cytometer. Anal Chem (2009) 81:5426–32. doi:10.1021/ac9005827
16. Walt DR. Chemistry: miniature analytical methods for medical diagnostics. Science (2005) 308:217–9. doi:10.1126/science.1108161
17. Cheng X, Gupta A, Chen C, Tompkins RG, Rodriguez W, Toner M. Enhancing the performance of a point-of-care CD4+ T-cell counting microchip through monocyte depletion for HIV/AIDS diagnostics. Lab Chip (2009) 9:1357–84. doi:10.1039/b818813k
18. Maheswaran S, Sequist LV, Nagrath S, Ulkus L, Brannigan B, Collura CV, et al. Detection of mutations in EGFR in circulating lung-cancer cells. N Engl J Med (2008) 359:366–77. doi:10.1056/NEJMoa0800668
19. Jokerst JV, Chou J, Camp JP, Wong J, Lennart A, Pollard AA, et al. Location of biomarkers and reagents within agarose beads of a programmable bio-nano-chip. Small (2011) 7:613–24. doi:10.1002/smll.201002089
20. Weigum SE, Floriano PN, Redding SW, Yeh CK, Westbrook SD, McGuff HS, et al. Nano-bio-chip sensor platform for examination of oral exfoliative cytology. Cancer Prev Res (2010) 3:518–28. doi:10.1158/1940-6207.CAPR-09-0139
21. Jokerst JV, Jacobson JW, Bhagwandin BD, Floriano PN, Christodoulides N, McDevitt JT. Programmable nano-bio-chip sensors: analytical meets clinical. Anal Chem (2010) 82:1571–9. doi:10.1021/ac901743u
22. Floriano PN, Christodoulides N, Miller CS, Ebersole JL, Spertus J, Rose BG, et al. Use of saliva-based nano-biochip tests for acute myocardial infarction at the point of care: a feasibility study. Clin Chem (2009) 55:1530–8. doi:10.1373/clinchem.2008.117713
23. Lavigne JJ, Savoy S, Clevenger MB, Ritchie JE, McDoniel B, Yoo SJ, et al. Solution-based analysis of multiple analytes by a sensor array: toward the development of an “electronic tongue”. J Am Chem Soc (1998) 120:6429–30. doi:10.1021/ja9743405
24. Goodey A, Lavigne JJ, Savoy SM, Rodriguez MD, Curey T, Tsao A, et al. Development of multianalyte sensor arrays composed of chemically derivitized polymeric microspheres localized in micromachined cavities. J Am Chem Soc (2001) 123:2559–70. doi:10.1021/ja003341l
25. Christodoulides N, Tran M, Floriano PN, Rodriguez M, Goodey A, Ali M, et al. A microchip-based multianalyte assay system for the assessment of cardiac risk. Anal Chem (2002) 74:3030–6. doi:10.1021/ac011150a
26. Ali MF, Kirby R, Goodey AP, Rodriguez MD, Ellington AD, Neikirk DP, et al. DNA hybridization and discrimination of single-nucleotide mismatches using chip-based microbead arrays. Anal Chem (2003) 75:4732–9. doi:10.1021/ac034106z
27. Floriano PN, Christodoulides N, Romanovicz DK, Bernard B, Simmons GW, Cavell M, et al. Membrane-based on-line optical analysis system for rapid detection of bacteria and spores. Biosens Bioelectron (2005) 20:2079–88. doi:10.1016/j.bios.2004.08.046
28. Rodriguez WR, Christodoulides N, Floriano PN, Graham S, Mohanty S, Dixon M, et al. A microchip CD4 counting method for HIV monitoring in resource-poor settings. PLoS Med (2005) 2:e182. doi:10.1371/journal.pmed.0020182
29. Weigum SE, Floriano PN, Christodoulides N, McDevitt JT. Cell-based sensor for analysis of EGFR biomarker expression in oral cancer. Lab Chip (2007) 7:995–1003. doi:10.1039/b703918b
30. Jokerst JV, Floriano PN, Christodoulides N, Simmons GW, McDevitt JT. Integration of semiconductor quantum dots into nano-bio-chip systems for enumeration of CD4+ T cell counts at the point-of-need. Lab Chip (2008) 8:2079–90. doi:10.1039/b817116e
31. Christodoulides N, Floriano PN, Acosta SA, Michael-Ballard K, Weigum SE, Dharshan P, et al. Towards the development of a lab-on-a-chip dual function white blood cell and C-reactive protein analysis method for the assessment of inflammation and cardiac risk. Clin Chem (2005) 51:2391–5. doi:10.1373/clinchem.2005.054882
32. Jokerst JV, McDevitt JT. Programmable nano-bio-chips: multifunctional clinical tools for use at the point-of-care. Nanomedicine (2010) 5:143–55. doi:10.2217/nnm.09.94
33. Raamanathan A, Simmons G, Christodoulides N, Floriano PN, Furmaga W, Redding S, et al. Programmable bio-nano-chip systems for serum Ca125 quantification: toward ovarian cancer diagnostics at the point-of-care. Cancer Prev Res (2012) 5:706–16. doi:10.1158/1940-6207.CAPR-11-0508
34. Shadfan B, Simmons A, Simmons G, Ho A, Wong J, Lu K, et al. A multiplexable, microfluidic platform for the rapid quantitation of a biomarker panel for early ovarian cancer detection at the point-of-care. Cancer Prev Res (2015) 8(1):42–53. doi:10.1158/1940-6207.CAPR-14-0248
35. McRae MP, Simmons GW, Wong J, Shadfan B, Gopalkrishnan S, Christodoulides N, et al. Programmable bio-nano-chip system: a flexible point-of-care platform for bioscience and clinical measurements. Lab Chip (2015) 15(20):4020–31. doi:10.1039/c5lc00636h
36. McDevitt JT, McRae MP, Simmons GW, Christodoulides N. Programmable bio-nano-chip system: a flexible diagnostic platform that learns. J Biosens Bioelectron (2015) 06(02):1–2. doi:10.4172/2155-6210.1000e137
37. Cassar A, Holmes DR Jr, Rihal CS, Gersh BJ. Chronic coronary artery disease: diagnosis and management. Mayo Clin Proc (2009) 84(12):1130–46. doi:10.4065/mcp.2009.0391
38. Ramani GV, Uber PA, Mehra MR. Chronic heart failure: contemporary diagnosis and management. Mayo Clin Proc (2010) 85(2):180–95. doi:10.4065/mcp.2009.0494
39. McRae MP, Bozkurt B, Ballantyne C, Sanchez X, Christodoulides N, Simmons GW, et al. Cardiac scorecard: a diagnostic multivariate index assay system for predicting a spectrum of cardiovascular disease. Expert Syst Appl (2016) 54:136–47. doi:10.1016/j.eswa.2016.01.029
40. Christodoulides N, Floriano PN, Sanchez X, Li L, Hocquard K, Patton A, et al. Programmable bio-nanochip technology for the diagnosis of cardiovascular disease at the point-of-care. Methodist Debakey Cardiovasc J (2012) 8(1):6–12. doi:10.14797/mdcj-8-1-6
41. Siegel RL, Miller KD, Jemal A. Cancer statistics. Cancer J Clin (2016) 66:7–30. doi:10.3322/caac.21332
42. Floriano P, Kerr A, Schmidt B, Corby P, Castilla I, Thornhill M, et al. Results of a 950-patient phase 2/3 clinical characterization-association study to classify patients with potentially malignant oral disorders (PMODS) using a non-invasive lab-on-a-chip (LOC) approach. Oral Oncol (2013) 49:S70. doi:10.1016/j.oraloncology.2013.03.183
43. Floriano P, Abram T, Taylor L, Le C, Talavera H, Nguyen M, et al. Programmable bio-nano-chip based cytologic testing of oral potentially malignant disorders in Fanconi anemia. Oral Disord (2015) 21(5):593–601. doi:10.1111/odi.12321
44. Speight P, Abram T, Floriano P, James R, Vick J, Thornhill M, et al. Inter-observer agreement in dysplasia grading: toward an enhanced gold standard for clinical pathology trials. Oral Surg Oral Med Oral Pathol Oral Radiol (2015) 120(4):474–82. doi:10.1016/j.oooo.2015.05.023
45. Abram TJ, Floriano PN, Christodoulides N, James R, Kerr AR, Thornhill MH, et al. “Cytology-on-a-chip” based sensors for monitoring of potentially malignant oral lesions. Oral Oncol (2016) 60:103–11. doi:10.1016/j.oraloncology.2016.07.002
46. Christodoulides N, De La Garza R, Simmons G, McRae M, Wong J, Newton T, et al. Application of programmable bio-nano-chip system for the quantitative detection of drugs of abuse in oral fluids. Drug Alcohol Depend (2015) 153:306–13. doi:10.1016/j.drugalcdep.2015.04.026
Keywords: non-invasive sampling, biomarkers, medical microdevices, in vitro diagnostics, point of care
Citation: Christodoulides NJ, McRae MP, Abram TJ, Simmons GW and McDevitt JT (2017) Innovative Programmable Bio-Nano-Chip Digitizes Biology Using Sensors That Learn Bridging Biomarker Discovery and Clinical Implementation. Front. Public Health 5:110. doi: 10.3389/fpubh.2017.00110
Received: 19 September 2016; Accepted: 02 May 2017;
Published: 22 May 2017
Edited by:
Elizabeth A. Shirtcliff, Iowa State University, USAReviewed by:
Angelo D’Ambrosio, University of Turin, ItalyCopyright: © 2017 Christodoulides, McRae, Abram, Simmons and McDevitt. This is an open-access article distributed under the terms of the Creative Commons Attribution License (CC BY). The use, distribution or reproduction in other forums is permitted, provided the original author(s) or licensor are credited and that the original publication in this journal is cited, in accordance with accepted academic practice. No use, distribution or reproduction is permitted which does not comply with these terms.
*Correspondence: John T. McDevitt, bWNkZXZpdHRAbnl1LmVkdQ==
Disclaimer: All claims expressed in this article are solely those of the authors and do not necessarily represent those of their affiliated organizations, or those of the publisher, the editors and the reviewers. Any product that may be evaluated in this article or claim that may be made by its manufacturer is not guaranteed or endorsed by the publisher.
Research integrity at Frontiers
Learn more about the work of our research integrity team to safeguard the quality of each article we publish.