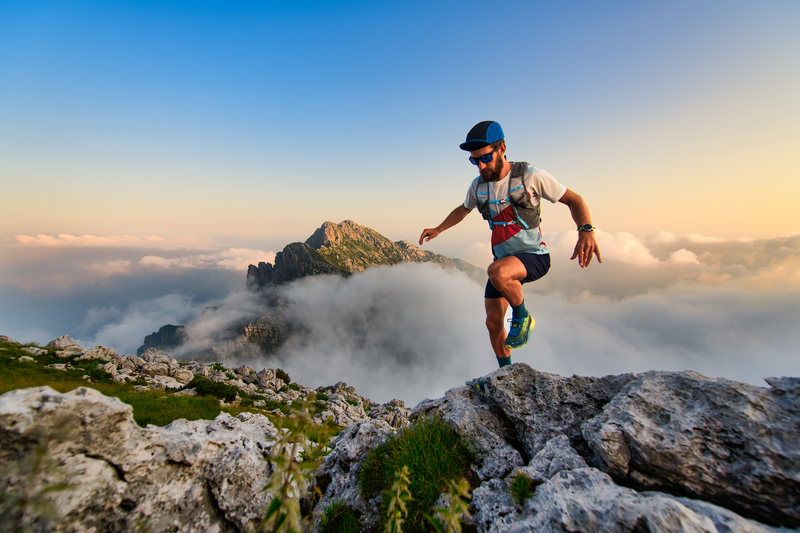
95% of researchers rate our articles as excellent or good
Learn more about the work of our research integrity team to safeguard the quality of each article we publish.
Find out more
ORIGINAL RESEARCH article
Front. Psychiatry
Sec. Sleep Disorders
Volume 16 - 2025 | doi: 10.3389/fpsyt.2025.1585732
The final, formatted version of the article will be published soon.
You have multiple emails registered with Frontiers:
Please enter your email address:
If you already have an account, please login
You don't have a Frontiers account ? You can register here
Background: Sleep disturbance has become a significant concern among college students, as it can lead to various mental and physical disorders. This study aims to provide a fresh perspective by developing and validating a predictive model for sleep quality among college students.Methods: Data from 20,645 college students in Fujian Province, China, collected between 5th April and 16th April 2022, were analyzed. Participants completed the Pittsburgh Sleep Quality Index (PSQI) scale, a self-designed general data questionnaire, and a sleep quality influencing factor questionnaire. Multinomial logistic regression, LASSO regression, and Boruta feature selection methods were utilized to select relevant variables. The data were then divided into a training–testing set (70%) and an independent validation set (30%) using stratified sampling. Six machine learning techniques, including artificial neural network (ANN), decision tree, gradient-boosting tree, k-nearest neighbor, naïve Bayes, and random forest, were developed and validated. Finally, an online sleep evaluation website was established based on the best-fitting prediction model.Results: The mean global PSQI score was 6.02 ± 3.112, with a sleep disturbance prevalence of 28.9% (defined as a global PSQI score > 7). The LASSO regression model identified eight predictors: age, specialty, respiratory history, coffee consumption, staying up late, prolonged online activity, sudden changes, and impatient closed-loop management. Among the evaluated models, the ANN demonstrated superior performance with an area under the receiver operating characteristic curve (AUC) of 0.713 (95% CI: 0.696–0.730), accuracy of 0.669 (95% CI: 0.669–0.669), sensitivity of 0.682 (95% CI: 0.699–0.665), specificity of 0.637 (95% CI: 0.665–0.610). Decision curve analysis and clinical impact analysis further confirmed the model’s clinical utility.Conclusions: This study developed a prediction model for sleep disturbance among college students using a LASSO regression and ANN, incorporating eight predictors. The model can serve as an intuitive and practical tool for predicting sleep quality and supporting effective management and healthcare on college campuses.
Keywords: sleep quality, college students, machine learning, LASSO regression, PSQI, ANN, Prediction model
Received: 01 Mar 2025; Accepted: 02 Apr 2025.
Copyright: © 2025 Yao, Chen, Kang, Zheng, Chen, Wang, Xia, Chen and Chen. This is an open-access article distributed under the terms of the Creative Commons Attribution License (CC BY). The use, distribution or reproduction in other forums is permitted, provided the original author(s) or licensor are credited and that the original publication in this journal is cited, in accordance with accepted academic practice. No use, distribution or reproduction is permitted which does not comply with these terms.
* Correspondence:
Lufeng Chen, The Second Affiliated Hospital of Fujian Medical University, Quanzhou, China
Disclaimer: All claims expressed in this article are solely those of the authors and do not necessarily represent those of their affiliated organizations, or those of the publisher, the editors and the reviewers. Any product that may be evaluated in this article or claim that may be made by its manufacturer is not guaranteed or endorsed by the publisher.
Research integrity at Frontiers
Learn more about the work of our research integrity team to safeguard the quality of each article we publish.