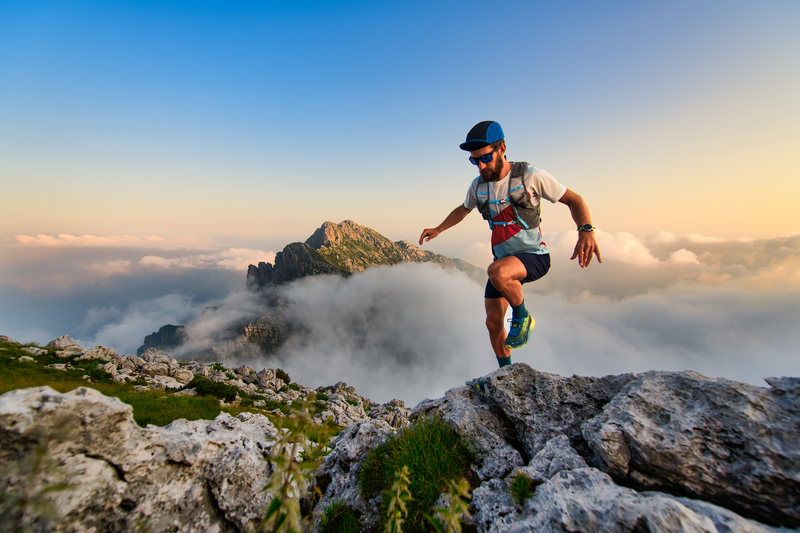
95% of researchers rate our articles as excellent or good
Learn more about the work of our research integrity team to safeguard the quality of each article we publish.
Find out more
ORIGINAL RESEARCH article
Front. Psychiatry
Sec. Mood Disorders
Volume 16 - 2025 | doi: 10.3389/fpsyt.2025.1584673
The final, formatted version of the article will be published soon.
You have multiple emails registered with Frontiers:
Please enter your email address:
If you already have an account, please login
You don't have a Frontiers account ? You can register here
Treatment-resistant depression (TRD) affects approximately 30% of patients with major depressive disorder (MDD), for whom effective treatment options are limited. Repetitive transcranial magnetic stimulation (rTMS) has shown efficacy in alleviating depressive symptoms in TRD. However, it remains unclear if these improvements are driven or mediated by changes in cognitive function or biological markers, such as brain-derived neurotrophic factor (BDNF). This study examines the effects of rTMS on depressive symptoms, cognition, and BDNF levels, as well as the potential moderating role of lifetime suicidal attempts (LSA) on cognition and the predictive value of baseline BDNF for clinical outcomes. Twenty-five TRD patients were included, with 13 in the rTMS treatment group (receiving 20 sessions of rTMS over four weeks) and 12 as control group. Depression severity, cognitive function (Mini-Mental State Examination, Verbal Fluency, Digit Span), and serum BDNF levels were measured pre-and post-treatment. Mixed-effects linear regression models assessed clinical and biological associations. rTMS significantly reduced HAM-D (p < 0.001) and CGI (p < 0.001) scores compared to controls. Cognitive performance improved significantly in MMSE (p = 0.049) and Digit Span (p = 0.04), with no significant changes in BDNF levels (p = 0.39). LSA did not moderate cognitive outcomes, and baseline BDNF did not predict clinical improvement (p = 0.68). rTMS reduced depressive symptoms in TRD patients, with modest cognitive benefits. Baseline BDNF did not predict outcomes, though the lack of significant change suggests complex neuroplastic responses. Future studies should include larger samples and refined biomarker assessments.
Keywords: rTMS, Depression, treatment-resistance, BDNF, Suicidality, neuroplasticity
Received: 27 Feb 2025; Accepted: 20 Mar 2025.
Copyright: © 2025 Ginelli, Sanna, Paribello, Isayeva, Corona, Zai, Manca, Iaselli, COLLU, Pinna, Scherma, Manchia, Fadda and Carpiniello. This is an open-access article distributed under the terms of the Creative Commons Attribution License (CC BY). The use, distribution or reproduction in other forums is permitted, provided the original author(s) or licensor are credited and that the original publication in this journal is cited, in accordance with accepted academic practice. No use, distribution or reproduction is permitted which does not comply with these terms.
* Correspondence:
Mirko Manchia, Department of Medical Sciences and Public Health, University of Cagliari, Cagliari, B3H 2E2, Nova Scotia, Italy
Disclaimer: All claims expressed in this article are solely those of the authors and do not necessarily represent those of their affiliated organizations, or those of the publisher, the editors and the reviewers. Any product that may be evaluated in this article or claim that may be made by its manufacturer is not guaranteed or endorsed by the publisher.
Research integrity at Frontiers
Learn more about the work of our research integrity team to safeguard the quality of each article we publish.