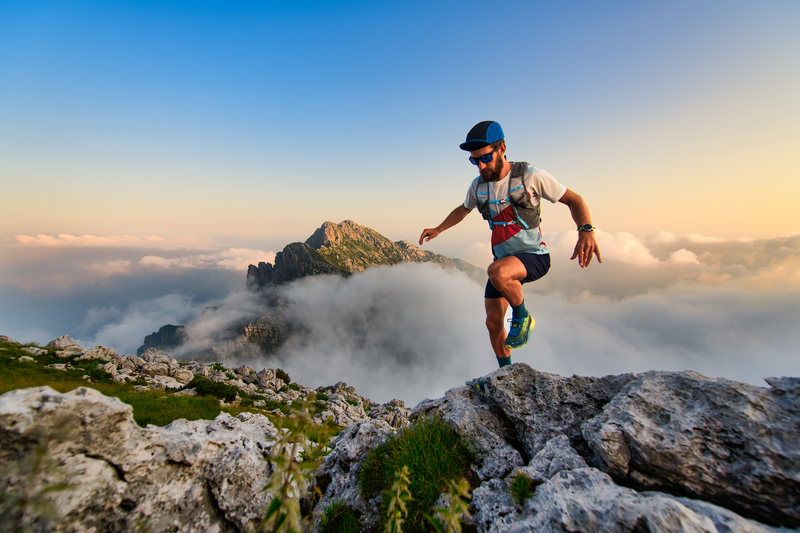
94% of researchers rate our articles as excellent or good
Learn more about the work of our research integrity team to safeguard the quality of each article we publish.
Find out more
ORIGINAL RESEARCH article
Front. Psychiatry
Sec. Molecular Psychiatry
Volume 16 - 2025 | doi: 10.3389/fpsyt.2025.1566155
This article is part of the Research Topic Non-Neuronal Cells in Neurodegenerative Diseases and Psychiatric Disorders View all 6 articles
The final, formatted version of the article will be published soon.
You have multiple emails registered with Frontiers:
Please enter your email address:
If you already have an account, please login
You don't have a Frontiers account ? You can register here
Background: Although neuronal dysfunction has been the focus of many studies on psychiatric disorders, accumulating evidence suggests that white matter abnormalities and oligodendrocyte lineage cells, including oligodendrocyte precursor cells (OPCs), play an important role. Beyond their established contribution to myelination, synaptic genes in OPCs form connections to neurons and influence neuronal circuits and plasticity, thereby potentially contributing to psychiatric pathology.Methods: We analyzed publicly available single–nucleus RNA sequencing (snRNA–seq) data from white matter cells of healthy donors with SCZ genome–wide association study (GWAS) summary statistics. We assessed cell–type–specific enrichment of SCZ–associated genetic variants and performed weighted gene co–expression network analysis (WGCNA) to identify disease–related gene modules in implicated cell types. Results: OPCs exhibited significant enrichment of SCZ–associated genetic risk variants and showed pronounced specificity in gene expression patterns. Through WGCNA, we identified a distinct co–expression module in OPCs that was enriched for synaptic genes associated with SCZ.Conclusion: The present results highlight the previously underappreciated role of OPCs in psychiatric disorders, suggesting that OPC–involved synaptic interactions may contribute to the pathophysiology of SCZ. This work underscores the importance of considering OPCs as active players in neural network dysfunction, with potential implications for future therapeutic strategies.
Keywords: schizophrenia1, Autism spectrum disorder2, oligodendrocyte precursor cells3, single-cell analysis4, Weighted gene co-expression network analysis5
Received: 24 Jan 2025; Accepted: 19 Mar 2025.
Copyright: © 2025 Kondo, Kimura and Ikeda. This is an open-access article distributed under the terms of the Creative Commons Attribution License (CC BY). The use, distribution or reproduction in other forums is permitted, provided the original author(s) or licensor are credited and that the original publication in this journal is cited, in accordance with accepted academic practice. No use, distribution or reproduction is permitted which does not comply with these terms.
* Correspondence:
Hiroki Kimura, Nagoya University, Nagoya, Japan
Disclaimer: All claims expressed in this article are solely those of the authors and do not necessarily represent those of their affiliated organizations, or those of the publisher, the editors and the reviewers. Any product that may be evaluated in this article or claim that may be made by its manufacturer is not guaranteed or endorsed by the publisher.
Research integrity at Frontiers
Learn more about the work of our research integrity team to safeguard the quality of each article we publish.