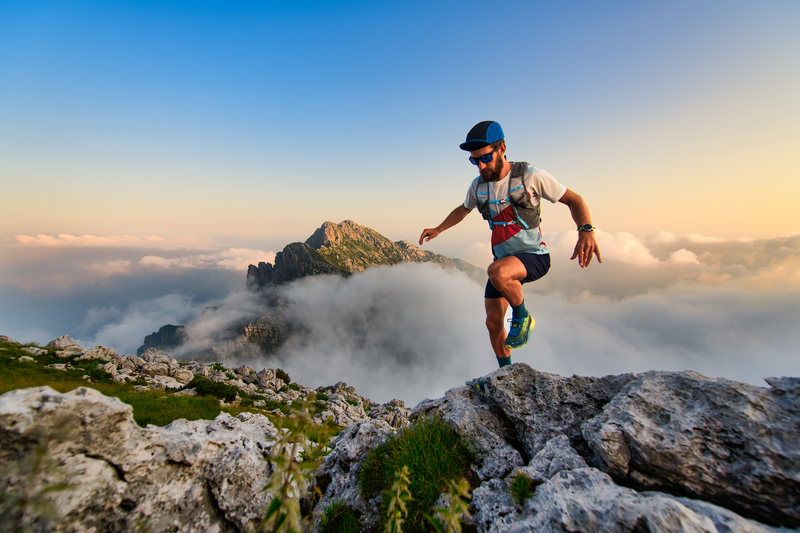
94% of researchers rate our articles as excellent or good
Learn more about the work of our research integrity team to safeguard the quality of each article we publish.
Find out more
ORIGINAL RESEARCH article
Front. Psychiatry
Sec. Adolescent and Young Adult Psychiatry
Volume 16 - 2025 | doi: 10.3389/fpsyt.2025.1559068
This article is part of the Research Topic Adolescent Emotional Disorders and Suicide Self-Harm Crisis Intervention View all 4 articles
The final, formatted version of the article will be published soon.
You have multiple emails registered with Frontiers:
Please enter your email address:
If you already have an account, please login
You don't have a Frontiers account ? You can register here
In this study, we compared the behavioral performance, ERP time-domain and time-frequency characteristics among depressed adolescents with NSSI, depressed adolescents, and healthy controls when exposed to negative emotional stimuli. The aim was to investigate the impact of negative emotional stimuli on the response inhibition in depressed adolescents with NSSI, clarify the role of response inhibition in NSSI behaviors, and provide neurophysiological evidence for its underlying mechanisms.Methods: Seventy-one depressed adolescents with NSSI (MDD+NSSI group: 12 males, 59 females; mean age: 14.37 years), 55 depressed adolescents (MDD group: 24 males, 31 females; mean age: 15.29 years) and 25 healthy subjects (HC group, 13 males, 12 females, mean age: 15.72 years) were recruited to perform a two-choice oddball task related to negative emotional cues. All participants completed a self-administered questionnaire to gather demographic information. A trained psychiatrist administered the Hamilton Depression Scale (HAMD-17) to assess depression severity, and used the Ottawa Self-Injury Inventory (OSI) to assess self-injury. Multichannel EEG was recorded continuously from 64 scalp electrodes using the Curry 8 system. EEG signal preprocessing and analysis was performed offline using the EEGLAB toolbox in MATLAB. The ERP time-domain features related to response inhibition were extracted from the difference waves, converted to the time-frequency features using the shorttime Fourier transform (STFT), and the time-frequency values of the region of interest (ROI) were extracted and statistically analyzed.Results: Under exposure to negative emotional stimuli, depressed adolescents with NSSI exhibited significantly larger P300 amplitudes compared to both depressed adolescents and healthy controls. Moreover, depressed adolescents with NSSI showed significant event-related synchronization (ERS) in the Delta and Theta bands of FCz electrode from 0 to 0.6 seconds, and event-related desynchronization (ERD) in the Theta and Alpha bands of Pz electrode from 0.2 to 1.2 seconds, collectively reflecting functional processes associated with response inhibition.Conclusions: Depressed adolescents with NSSI showed increased P3d amplitudes, enhanced Delta/Theta ERS, and heightened Theta/Alpha ERD when receiving negative emotional stimuli, suggesting that depressed adolescents with NSSI have impaired response inhibition, which may contribute to the development of NSSI.
Keywords: nonsuicidal self-injury, adolescents, response inhibition, negative emotional stimulation, time-frequency analysis
Received: 11 Jan 2025; Accepted: 10 Mar 2025.
Copyright: © 2025 Zhao, Hong, Peng, He, Hu, Ma, Liu, Tao, Chen, Jiang, Zhang, Liao, Xiang, Zeng, Dai, Zhang, Wang and Kuang. This is an open-access article distributed under the terms of the Creative Commons Attribution License (CC BY). The use, distribution or reproduction in other forums is permitted, provided the original author(s) or licensor are credited and that the original publication in this journal is cited, in accordance with accepted academic practice. No use, distribution or reproduction is permitted which does not comply with these terms.
* Correspondence:
Li Kuang, First Affiliated Hospital of Chongqing Medical University, Chongqing, China
Disclaimer: All claims expressed in this article are solely those of the authors and do not necessarily represent those of their affiliated organizations, or those of the publisher, the editors and the reviewers. Any product that may be evaluated in this article or claim that may be made by its manufacturer is not guaranteed or endorsed by the publisher.
Research integrity at Frontiers
Learn more about the work of our research integrity team to safeguard the quality of each article we publish.