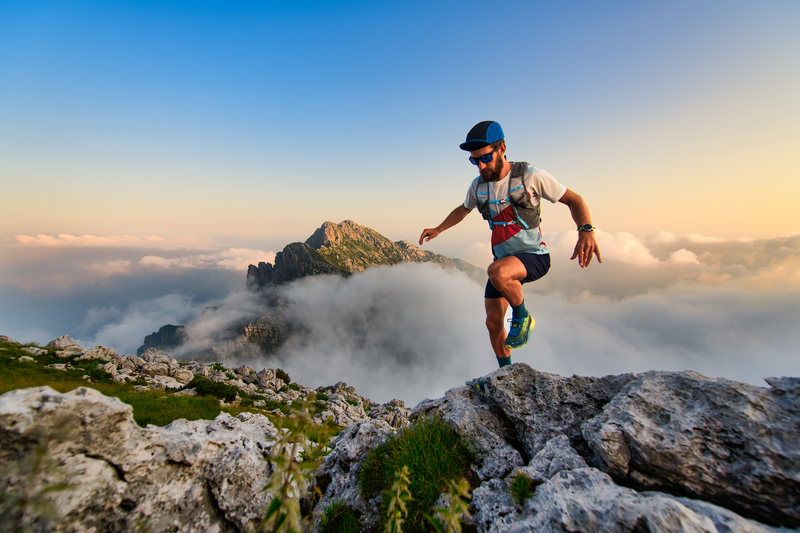
94% of researchers rate our articles as excellent or good
Learn more about the work of our research integrity team to safeguard the quality of each article we publish.
Find out more
ORIGINAL RESEARCH article
Front. Psychiatry
Sec. Schizophrenia
Volume 16 - 2025 | doi: 10.3389/fpsyt.2025.1557659
The final, formatted version of the article will be published soon.
You have multiple emails registered with Frontiers:
Please enter your email address:
If you already have an account, please login
You don't have a Frontiers account ? You can register here
Objective:Catatonia was often complicated by pneumonia, and the development of severe pneumonia after admission posed significant challenges to its treatment. This study aimed to develop a Nomogram Model based on pre-admission characteristics of patients with catatonia to predict the risk of pneumonia after admission. Methods:This retrospective observational study reviewed catatonia patients hospitalized at Hangzhou Seventh People's Hospital from September 2019 to November 2024. Data included demographic characteristics, medical history, maintenance medications, and pre-admission clinical presentations. Patients were divided into catatonia with and without pneumonia groups. The LASSO Algorithm was used for feature selection, and seven machine learning models: Decision Tree(DT), Logistic Regression(LR), Naive Bayes(NB), Random Forest(RF), K Nearest Neighbors(KNN), Gradient Boosting Machine(GBM), Support Vector Machine(SVM) were trained. Model performance was evaluated using AUC, Accuracy, Sensitivity, Specificity, Positive Predictive Value, Negative Predictive Value, F1 Score, Cohen's Kappa, and Brier Score, and Brier score. The best-performing model was selected for multivariable analysis to determine the variables included in the final Nomogram Model. The Nomogram Model was further validated through ROC Curves, Calibration Curves, Decision Curve Analysis (DCA), and Bootstrapping to ensure discrimination, calibration, and clinical applicability. Results:Among 156 patients, 79 had no pneumonia, and 77 had pneumonia. LASSO Algorithm identified 15 non-zero coefficient variables (LASSO 1-SEλ=0.076). The GBM showed the best performance (AUC = 0.954, 95% CI: 0.924-0.983, vs other models by DeLong's test: P < 0.05). Five key variables: Age, Clozapine, Diaphoresis, Intake Refusal, and Waxy Flexibility were used to construct the Nomogram Model. Validation showed good discrimination (AUC = 0.803, 95% CI: 0.735-0.870), calibration, and clinical applicability. Internal validation (Bootstrapping, n=500) confirmed model stability (AUC = 0.814, 95% CI: 0.743-0.878; Hosmer-Lemeshow P = 0.525).Conclusion:This study developed a Nomogram Model based on five key factors, demonstrating significant clinical value in predicting the risk of pneumonia in hospitalized patients with catatonia.
Keywords: :Catatonia, Pneumonia, Clinical prediction model, machine learning, nomogram
Received: 08 Jan 2025; Accepted: 20 Feb 2025.
Copyright: © 2025 Wang, He, Wu, Zhang, Wu, Fang, Jia and Luo. This is an open-access article distributed under the terms of the Creative Commons Attribution License (CC BY). The use, distribution or reproduction in other forums is permitted, provided the original author(s) or licensor are credited and that the original publication in this journal is cited, in accordance with accepted academic practice. No use, distribution or reproduction is permitted which does not comply with these terms.
* Correspondence:
Fu-gang Luo, Hangzhou Seventh Peoples Hospital, Hangzhou, China
Disclaimer: All claims expressed in this article are solely those of the authors and do not necessarily represent those of their affiliated organizations, or those of the publisher, the editors and the reviewers. Any product that may be evaluated in this article or claim that may be made by its manufacturer is not guaranteed or endorsed by the publisher.
Research integrity at Frontiers
Learn more about the work of our research integrity team to safeguard the quality of each article we publish.