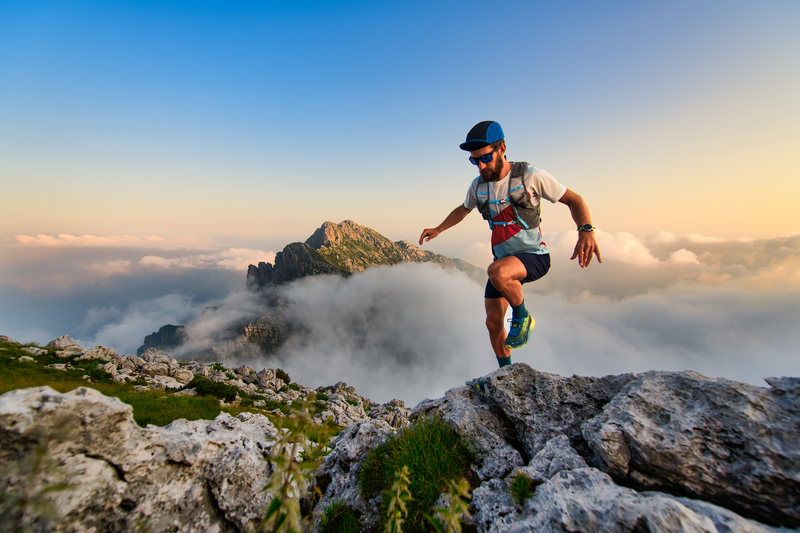
94% of researchers rate our articles as excellent or good
Learn more about the work of our research integrity team to safeguard the quality of each article we publish.
Find out more
ORIGINAL RESEARCH article
Front. Psychiatry
Sec. Public Mental Health
Volume 16 - 2025 | doi: 10.3389/fpsyt.2025.1555513
This article is part of the Research Topic The Intersection of Psychology, Healthy Behaviors, and its Outcomes View all 69 articles
The final, formatted version of the article will be published soon.
You have multiple emails registered with Frontiers:
Please enter your email address:
If you already have an account, please login
You don't have a Frontiers account ? You can register here
Background: Patients with somatization symptoms are at elevated risk of depression, yet underdiagnosis persists due to cultural tendencies (e.g., in China) to express psychological distress via physical complaints. Existing predictive models lack integration of sociocultural and physiological factors, particularly in non-Western populations.Objective: To develop a culturally tailored risk-prediction model for depression in patients with somatization symptoms, emphasizing early identification and personalized intervention.Methods: A prospective cohort study included 200 somatization patients (SSS≥38, PHQ-2<3) from a Chinese hospital (May 2020–August 2022). LASSO regression identified predictors from 18 variables, followed by multivariate logistic regression to construct a nomogram. Model performance was assessed via ROC-AUC, calibration curves, Hosmer-Lemeshow test, and decision curve analysis (DCA). Internal validation used 200 bootstrap resamples.Results:Five independent predictors were identified: advanced age (OR=1.11, 95% CI: 1.02–1.20), poor self-rated health (OR=2.07, 95% CI: 1.04–4.30), lack of co-residence with children (OR=1.63, 95% CI: 1.10–2.42), low income (OR=1.45, 95% CI: 1.05–2.01), and self-medication (OR=1.32, 95% CI: 1.01–1.73). The nomogram demonstrated strong discrimination (AUC=0.810, 95% CI: 0.728–0.893) and calibration (Hosmer-Lemeshow p=0.32). DCA confirmed clinical utility: at threshold probabilities >5%, the model provided higher net benefit than "treat-all" or "treat-none" strategies.Conclusion: This model integrates sociocultural (e.g., family structure) and behavioral factors to predict depression risk in somatizing patients, particularly in East Asian contexts. It offers a practical tool for clinicians to prioritize high-risk individuals, reducing diagnostic delays and healthcare burdens. Future multicenter studies should validate its generalizability and incorporate biomarkers (e.g., inflammatory markers) to enhance mechanistic insights.
Keywords: predictive model, Depression risk, Somatization symptoms, Clinical 49 Validation, Risk factors identification
Received: 04 Jan 2025; Accepted: 05 Mar 2025.
Copyright: © 2025 Tang, Zhong, Zeng, Deng, Huang, Ye, Li, Lai, Huang, Chen, Deng, Lai, Wu, Zou, Qiu and Liao. This is an open-access article distributed under the terms of the Creative Commons Attribution License (CC BY). The use, distribution or reproduction in other forums is permitted, provided the original author(s) or licensor are credited and that the original publication in this journal is cited, in accordance with accepted academic practice. No use, distribution or reproduction is permitted which does not comply with these terms.
* Correspondence:
Liming Tang, Longyan First Hospital Affiliated to Fujian Medical University, Longyan, China
Hanzhong Qiu, Longyan First Hospital Affiliated to Fujian Medical University, Longyan, China
Ying Liao, Longyan First Hospital Affiliated to Fujian Medical University, Longyan, China
Disclaimer: All claims expressed in this article are solely those of the authors and do not necessarily represent those of their affiliated organizations, or those of the publisher, the editors and the reviewers. Any product that may be evaluated in this article or claim that may be made by its manufacturer is not guaranteed or endorsed by the publisher.
Research integrity at Frontiers
Learn more about the work of our research integrity team to safeguard the quality of each article we publish.