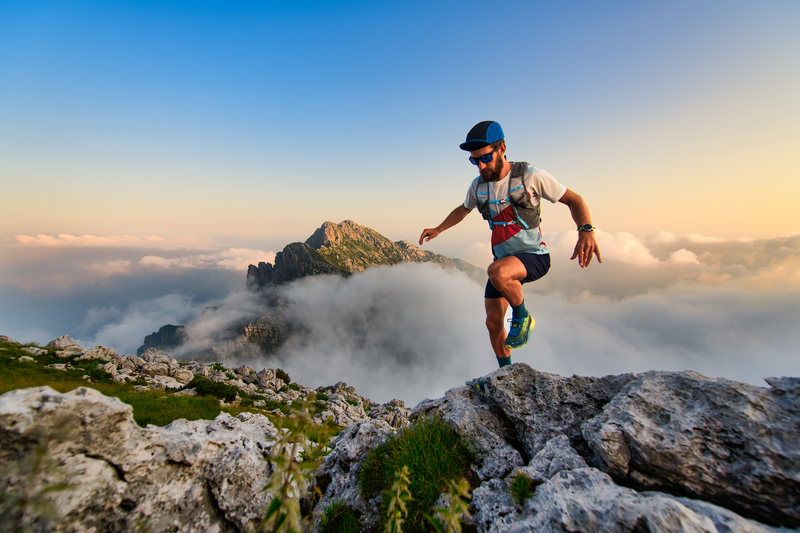
95% of researchers rate our articles as excellent or good
Learn more about the work of our research integrity team to safeguard the quality of each article we publish.
Find out more
BRIEF RESEARCH REPORT article
Front. Psychiatry , 17 March 2025
Sec. Schizophrenia
Volume 16 - 2025 | https://doi.org/10.3389/fpsyt.2025.1550571
This article is part of the Research Topic Machine Learning Algorithms and Software Tools for Early Detection and Prognosis of Schizophrenia View all 4 articles
Introduction: Formal Thought Disorder (FTD) is a significant clinical feature of early-onset psychosis, often associated with poorer outcomes. Current diagnostic methods rely on clinical assessment, which can be subjective and time-consuming. This study aimed to investigate the potential of neuropsychological tests and machine learning to differentiate individuals with and without FTD.
Methods: A cohort of 27 young people with early-onset psychosis was included. Participants underwent neuropsychological assessment using the Iowa Gambling Task (IGT) and Simple Reaction Time (SRT) tasks. A range of machine learning models (Logistic Regression (LR), Support Vector Machines (SVM), Random Forest (RF) and eXtreme Gradient Boosting (XGBoost)) were employed to classify participants into FTD-positive and FTD-negative groups based on these neuropsychological measures and their antipsychotic regimen (medication load in chlorpromazine equivalents).
Results: The best performing machine learning model was LR with mean +/- standard deviation of cross validation Receiver Operating Characteristic Area Under Curve (ROC AUC) score of 0.850 (+/- 0.133), indicating moderate-to-good discriminatory performance. Key features contributing to the model’s accuracy included IGT card selections, SRT reaction time (most notably standard deviation) and chlorpromazine equivalent milligrams. The model correctly classified 24 out of 27 participants.
Discussion: This study demonstrates the feasibility of using neuropsychological tests and machine learning to identify FTD in early-onset psychosis. Early identification of FTD may facilitate targeted interventions and improve clinical outcomes. Further research is needed to validate these findings in larger, more diverse populations and to explore the underlying neurocognitive mechanisms.
Formal Thought Disorders (FTDs) comprise a range of complex psychiatric phenomena characterized by a disruption in the form (as opposed to the content) of thought processes (1). This distinguishes them from delusions, which are predominantly content based (2). Clinical examination of FTDs focus on the analysis of time-related parameters as well as qualitative (logical) structure of thoughts’ stream (3). Due to a broad spectrum of neuropathological changes affecting cognitive, linguistic, and emotional domains, FTDs can significantly impair a patient’s ability to communicate. This, in turn, compromises their quality of life and restricts social and occupational participation. The presence of FTDs is also a poor prognostic factor, heralding a limited response to therapeutics and a decreased rate of remission. FTDs are more prevalent in early-onset schizophrenia (54.5%) as compared to a late-onset schizophrenia (5.6%) and can even precede overt psychotic symptoms (4). Early-onset schizophrenia (EOS) spectrum is defined as the onset of psychotic symptoms below the 18 years of life (early-onset), as well as below the 13 life year (very-early onset, VEOS). EOS populations links with different clinical picture, including: autism-spectrum features, psychoactive substances misuse, longer duration of untreated psychosis (DUP) and poorer prognosis. Thus, a timely and accurate identification of FTDs holds a significant value in child and adolescent psychiatric practice.
Quantifying formal thought disorders usually involves clinical assessment using standardized psychometric scales. Many such scales have been proposed. The most commonly used are the Thought Disorder Index (TDI) (5) and the Bizarre – Idiosyncratic Thinking scale (6). These are adequately reliable and feature a valid scoring system but tend to be time consuming. The well-established Thought, Language and Communication scale (TLC) (7) concisely encapsulates many aspects of thought disorders but does not include all of the FTD symptoms. This means that it may miss more subtle and early manifestations of FTD in children. The Thought and Language index (TLI) (8), while capable of detecting subtle disorders, fails to capture the more nuanced subjective elements, and its coding of verbal transcripts tends to be time-consuming as well. The Clinical language (CLANG) (9) scale investigates thought, as well as the speech quality (e.g. enunciation, impairments), but operates on a strict linguistic hypothesis, which means it may miss other domains of FTD presentation. Aiming towards a more holistic understanding of FTDs, the Formal Thought Disorder (FTD) scales (10) were introduced. These scales provide an explanation of thought disorders from the viewpoint of both patient and caregiver. However, they tend to lack an objective assessment of symptoms and may lead to misinterpretations due to the absence of clinical interviews complementing the assessment. The relatively newer Thought and Language Disorder (TALD) (11) scale offers a thorough and sensitive evaluation and encompasses the multidimensional range of FTD, but is less scientifically validated, and has a limited subjective evaluation (24 hrs prior to interview).
There is a clear need for a more objective and less interviewer-dependent way of assessing FTDs that could be more easily deployed in clinical practice. To that end, one interesting avenue of exploration presented itself in the cognitive domain of FTD (12). Positive FTD features such as circumstantiality, tangentiality, derailment or logorrhea have been linked to impairments of executive function (13). Similar executive deficits have been observed in negative FTD symptoms such as dysfunction of thought production (14). FTDs have also been associated with impairments in attention and working memory, as well as verbal and semantic fluency deficits (15). Despite a relative abundance of research in this area, results of some cognitive studies have been inconclusive (12, 16–18). The vast majority of the studied population were psychotic or manic adult patients (18), who may present with a different neurobiology of symptoms as compared with the child and adolescent population (19, 20). Furthermore, there is currently no research investigating whether executive dysfunction can be used to screen for the presence of FTDs in early psychosis patients. Since FTDs can be associated with poorer treatment responses, an early signal of the disease can be of clinical importance.
Machine learning approaches have emerged as powerful tools in psychiatric research, offering the potential to identify complex patterns in clinical, neurobiological, and behavioral data that may not be apparent through traditional statistical methods. The success of these applications, combined with the need for more objective and efficient diagnostic tools in early-onset psychosis, provides a strong rationale for investigating machine learning approaches in FTD detection.
The aim of the present study is to determine whether commonly used, rapid, and inexpensive executive function tests can be used to differentiate individuals with early-onset psychosis with an FTD element from those with similarly presenting early-onset psychosis, but without a discernible functional thought disorder. Our hypothesis is that individuals with aberrant neural networks will underperform in neuropsychometric testing due to the increased cognitive load of the underlying psychotic symptoms.
Measurements obtained for this paper originate from Comparison of Biomarkers in Schizophrenia and Bipolar Affective Disorder study, which was a case control study approved by the Regional Ethics Committee at Poznan University of Medical Sciences (reference number 1066/19), in line with European regulations. All participants and/or legal guardians received written information about the study prior to recruitment. Informed consent was taken by a Good Clinical Practice (GCP)-trained clinician member of the research team. The recruitment process was as follows. All patients admitted with psychotic symptoms to the Zabor Centre for Child and Adolescent Psychiatry (a tertiary referral hospital for western Poland) were offered participation in the study [for full protocol see (21)], and if eligible, were included in the present analysis. Diagnosis of FTD was made using Structured Clinical Interview for DSM Disorders (SCID-I) by a licensed child and adolescent psychiatrist and later confirmed by an independent board-certified specialist and principal provincial consultant for child and adolescent psychiatry. The FTD criteria used for the diagnosis were as per DSM-IV. The inclusion criteria were primary psychotic disorder and ability to perform cognitive task, as well as stabilization of medication. Exclusion criteria were presence of any acute medical or metabolic disorder, detection of alcohol or psychoactive substances during admission procedure or moderate-severe learning disability. Finally, in order to be eligible for inclusion, patients had to be clear of secondary psychosis, or other internal medicine diagnoses presenting with psychotic symptoms. All participants were tested in their on-treatment state, whereby stabilization and effective dosage of antipsychotic medication was achieved for at least 6 weeks. Pharmacological treatment is listed in Table 1.
Table 1. List of all antipsychotic medication used in treatment of participants included in the study, at the time of neuropsychometric evaluation.
Two tests were used in this study: the Iowa Gambling Task (IGT) and the Simple Response Time (SRT), which were chosen for their ubiquitous use in assessment of executive function. IGT was administered by Psychological Experiment Building Language (PEBL) software using standard protocol (22). SRT was delivered by the same software, with the task featuring an instruction to press the left mouse button upon presentation of a visual stimulus on the screen (a letter X). The features for these tasks were extracted as follows. For IGT, the number of advantageous cards (sum of, out of 100) and disadvantageous cards (sum of, out of 100) were used. For SRT, the mean, standard deviation was used for the 4 x 50 trials, with breaks in between. Minimum and maximum reaction times were also used.
A range of machine learning models were used for classification, namely LR, SVM, RF, and XGBoost. The choice of models was based on their versatility in handling small size samples. Feature scaling was performed using standardization to ensure comparable feature ranges. To optimize model performance and prevent overfitting, a standardized sklearn library pipeline was used, incorporating standardization (Standard Scaler) and model fitting. Hyperparameter tuning was conducted using a grid search with the parameters shown in Table 2.
Nested cross-validation was implemented to provide a robust estimate of model performance. The outer loop employed a stratified five-fold cross-validation to partition the data into training and testing sets. For each training set, an inner five-fold cross-validation was performed to select the optimal hyperparameters based on the area under the receiver operating characteristic curve (AUC). Model performance was evaluated using a range of metrics, including accuracy, precision, recall, F1-score, and AUC. Additionally, a confusion matrix was generated to evaluate the model’s classification accuracy. Our analysis uses the default 0.5 probability threshold for class separation when calculating accuracy, precision, recall and F1-score; this threshold was not explicitly changed or optimized within training process.
Out of N = 31 patients experiencing psychotic episode, N = 27 (N = 11 FTD negative; N = 16 FTD positive, as diagnosed by SCID-I) were well enough to complete neuropsychometric tests. Demographic and clinical information about this sample is presented in Table 3.
Nested cross-validation results for all models are presented in Table 4. The winning model was Logistic Regression (mean +/- standard deviation): Accuracy: 0.673 (+/- 0.213), Precision: 0.750 (+/- 0.224), Recall: 0.750 (+/- 0.247), ROC AUC: 0.850 (+/- 0.133), F1: 0.723 (+/- 0.195). Validation curve for C can be found in Figure 1B. The final feature coefficients (relative contributions) to the winning model were advantageous cards: -0.299, disadvantages cards: 1.645, mean SRT: 2.592, minimum SRT: 2.428, maximum SRT: 3.617, standard deviation of SRT: -6.360, and chlorpromazine equivalent in milligrams: 2.187. The correlation matrix revealed an expected perfect (1) correlation of IGT advantageous and disadvantageous card selections and an equally anticipated high (0.86) correlation between maximum SRT reaction time and standard deviation of the SRT reaction time. The remainder of features were not significantly correlated (Figure 1D).
Figure 1. (A) Receiver operating characteristic curve of the winning model for detecting formal thought disorder in child and adolescent patients experiencing psychosis (B) Validation curve for the model (C) confusion matrix showing overall classification performance of the model (D) Correlation matrix of the features included in the model.
The overall ROC AUC for the best model was 0.972 (Figure 1A). The confusion matrix provides a detailed overview of the winning model’s classification performance. As shown in Figure 1C, based on neuropsychometry data alone, the model was able to correctly classify 10 participants without FTD and 14 with FTD. One participant was incorrectly classified as having FTD, whilst two were predicted as not having FTD diagnosis despite being confirmed by ground truth psychiatric assessment. Overall, the model demonstrated moderate-to-good performance in assigning participants to both classes.
This study demonstrated the potential of neuropsychological testing, specifically the IGT and SRT, combined with machine learning, to differentiate individuals with and without formal thought disorders within a cohort of children and adolescent experiencing psychotic episodes. A logistic regression model, optimized through nested cross-validation, achieved moderate-to-good discriminatory performance. The model’s classification accuracy revealed correct identification of all but three participants. Feature analysis highlighted the significant contributions of the minimum and standard deviation SRT reaction time, and IGT advantageous and disadvantageous card selections to the model’s predictive capacity. No differences were observed in overall disease severity as assessed by PANSS, at baseline and after treatment, which may support the added clinical value of neuropsychometric testing in this population. These findings suggest that distinct patterns of decision-making and reaction time variability, as measured by the IGT and SRT, respectively, may serve as valuable indicators in the diagnostic process of FTD in individuals presenting with psychosis.
Feature importance analysis yield further insights into the utility of neuropsychometric testing, as response time measurement enables real-time and independent clinical insight into executive processing. SRT reflects the quality of processing speed in cortico-subcortical loops, crucial for higher cognitive functions like thinking operations. According to dysexecutive theory of FTDs, thinking disorders may be the effect of general processing speed disorder. Among general population the software functions like thinking and language networks operate on healthy background (hardware) of brain cybernetics. Regarding psychotic patients, impaired linguistic networks operations and decision making may be the effect of brain disconnection and cognitive load due to concurring processes, like aberrant salience phenomena. Hence the minimal and standard deviation of SRT may be an important proxy feature for the background functioning of the brain hardware.
To our knowledge this is the first study specifically investigating the use of SRT and IGT cognitive modalities in child and adolescent psychotic population. The executive function (EF) impairments demonstrated through these tests add to the body of evidence suggesting that goal-directed behavior deficits are prevalent in children and adolescents at risk for psychosis, as well as those diagnosed with schizophrenia spectrum disorders. A study involving 100 genetically high-risk children and adolescents identified four neurocognitive clusters based on EF performance alone, highlighting significant impairments self-control and decision-making capabilities (23). Another study found that poor “hot” executive functions, such as decision-making under uncertainty, were significantly associated with psychotic symptoms in a cohort of 156 young patients, suggesting a specific vulnerability in spatial working memory and Cambridge Gambling Task (24). Research on adolescents with antipsychotic-treated schizophrenia showed that these individuals had higher scores on the Behavior Rating Inventory of Executive Function, indicating notable EF impairments compared to healthy controls (25). Additionally, a study of adolescents with schizophrenia spectrum disorders revealed that executive function was among the most impaired cognitive domains, paralleling findings in adult populations (26). EF impairments were also predictive of persistent semantic and language production impairments in first episode psychosis population.
The results of our experiment can be corroborated by measurements obtained in adult psychotic population. One study revealed a moderate (R2 = -0.48) correlation of Wechsler Adult Intelligence Scale processing speed and FTD symptoms in adults fulfilling the DSM IV-TR criteria for schizophrenia disorder (27). Similar observations (R2 = -0.54) were made for association of communication disturbance index (a measure of FTD) and processing speed in an arrow task, whereby patients with primary psychotic disorder needed to react to the arrow pointing right or left and choose the correct or opposite direction on the basis of the color of the cue (28). FTD symptoms also seem to intensify when participants are asked to perform cognitive tasks, which may explain why their real-life functioning is often impaired (29).
The connection between FTD and EF deficits observed in our population have a strong neurobiological basis, which may be understood in three dimensions: the anatomical impact, the neurotransmitter system dysregulation, and the genetic background (2). One of the major findings in structural MRI in schizophrenic patients is the reduction of cortical volumes in the left superior temporal gyrus, middle temporal gyrus, and the frontal operculum (30). The affected areas are within the Wernicke’s and Broca’s areas, therefore, possibly affecting the neurodevelopment of language and speech (31). Findings from Kircher et al., also suggest that dysfunctional glutamatergic receptors caused by reduced synapses in the superior temporal gyrus are the main drive for developing positive FTD. Moreover, dopaminergic hyperactivity and hyper-priming has shown to affect both glutamatergic excitatory and GABAergic inhibitory networks, causing positive FTD in schizophrenia (2). The pathophysiology of psychotic state in schizophrenia involves both dopaminergic dysregulations in aberrant salience and neural network synaptic plasticity disruption linking with loosening of associations. Neurobiological processes, underlying the psychotic pathology of the brain, show impaired positive reinforcement cybernetic loop between dopaminergic outbursts and synaptic disorganization, enhancing each other. Lastly, twin studies and adoption studies have shown a positive correlation between FTD and heritability, suggesting genetic risk of developing FTD (32, 33). It may be hypothesized that the poor treatment outcomes for FTD population stem from a lack of adequate focus on these neurobiological pathways in conventional therapeutic options for child and adolescent psychotic population. The pharmacological options deployed in FTD cases often target acute positive FTD, utilizing neuroleptic action and providing the stabilized dopaminergic pathway without any effects on cortical plasticity mechanisms. There are a few studies supporting the effects of cognitive-behavioral therapy (CBT) on FTD (34). However, no specific psychotherapy has so far been established.
The implementation of machine learning-based FTD detection in clinical settings appears feasible, given the relatively straightforward administration of IGT and SRT tasks requiring only standard computing equipment and a few minutes of testing time. The model’s moderate-to-good discriminatory performance suggests utility as a screening tool to complement traditional clinical assessment, potentially improving early detection of FTD in resource-limited settings. Caution must be applied, however, as all small number studies have inherent limitations regarding their external validity in real-life settings. Integration into existing workflows could thus follow a two-stage approach, where positive machine learning-based screening results trigger comprehensive clinical evaluation, while regular administration could facilitate longitudinal monitoring of cognitive function and treatment response.
The study is not without limitations. First, we only used two relatively simple tasks, producing features with very limited granularity and cognitive domain precision. This was done to maximize participation and enhance clinical adaptation, which is easier with simpler and less time-consuming tasks. Not all participants were correctly classified by the model. Therefore, post-hoc analysis of medical notes was conducted to look for any clinicodemographic differences that may explain these discrepancies. In one false-negative case, the treating clinicians recorded a rapid onset of symptoms, with a short duration of untreated psychosis. It may be hypothesized that an early stabilization of the disease may have spared cognitive function in this case. Conversely, in two false-positive cases, the treatment duration was relatively long, with repeated extensive stay hospitalizations and exacerbations of the disease. This may have caused greater cognitive decline despite lack of the FTD element. Further research on a larger population is clearly warranted to establish whether there is any significant link between FTD and clinicodemographic features such as disease duration, number of hospitalizations, specific drug agents, or treatment intensity.
The original contributions presented in the study are included in the article/supplementary material. Further inquiries can be directed to the corresponding author.
The studies involving humans were approved by Regional Ethics Committee at Poznan University of Medical Sciences (reference number 1066/19). The studies were conducted in accordance with the local legislation and institutional requirements. Written informed consent for participation in this study was provided by the participants’ legal guardians/next of kin.
PZ: Conceptualization, Data curation, Formal analysis, Funding acquisition, Investigation, Methodology, Project administration, Resources, Software, Supervision, Validation, Visualization, Writing – original draft, Writing – review & editing. MB: Conceptualization, Data curation, Formal analysis, Funding acquisition, Investigation, Methodology, Project administration, Resources, Software, Supervision, Validation, Visualization, Writing – original draft, Writing – review & editing. CL: Formal analysis, Writing – original draft, Writing – review & editing. SK: Writing – original draft, Writing – review & editing. OW: Writing – original draft, Writing – review & editing. ZW: Writing – original draft, Writing – review & editing. DB: Writing – original draft, Writing – review & editing. BR: Formal analysis, Supervision, Writing – original draft, Writing – review & editing.
The author(s) declare that financial support was received for the research and/or publication of this article. PZ was supported by a grant from the Provincial Government of the Lubuskie Province, Poland. This grant was used to support the development of child and adolescent psychiatry in the west of Poland.
The authors declare that the research was conducted in the absence of any commercial or financial relationships that could be construed as a potential conflict of interest.
The author(s) declare that no Generative AI was used in the creation of this manuscript.
All claims expressed in this article are solely those of the authors and do not necessarily represent those of their affiliated organizations, or those of the publisher, the editors and the reviewers. Any product that may be evaluated in this article, or claim that may be made by its manufacturer, is not guaranteed or endorsed by the publisher.
1. Zamperoni G, Tan EJ, Rossell SL, Meyer D, Sumner PJ. Evidence for the factor structure of formal thought disorder: A systematic review. Schizophr Res. (2024) 264:424–34. doi: 10.1016/j.schres.2024.01.006
2. Kircher T, Bröhl H, Meier F, Engelen J. Formal thought disorders: From phenomenology to neurobiology. Lancet Psychiatry. (2018) 5:515–26. doi: 10.1016/S2215-0366(18)30059-2
3. Dornelles E, Correia DT. The neurobiology of formal thought disorder. Curr Topics Medicinal Chem. (2024) 24:1773–83. doi: 10.2174/0115680266272521240108102354
4. Roche E, Creed L, MacMahon D, Brennan D, Clarke M. The epidemiology and associated phenomenology of formal thought disorder: A systematic review. Schizophr Bull. (2015) 41:951–62. doi: 10.1093/schbul/sbu129
5. Solovay MR, Shenton ME, Gasperetti C, Coleman M, Kestnbaum E, Carpenter JT, et al. Scoring manual for the thought disorder index. Schizophr Bull. (1986) 12:483–96. doi: 10.1093/schbul/12.3.483
6. Marengo JT, Harrow M, Lanin-Kettering I, Wilson A. Evaluating bizarre-idiosyncratic thinking: A comprehensive index of positive thought disorder. Schizophr Bull. (1986) 12:497–511. doi: 10.1093/schbul/12.3.497
7. Andreasen NC. Thought, language, and communication disorders. II. Diagnostic significance. Arch Gen Psychiatry. (1979) 36:1325–30. doi: 10.1001/archpsyc.1979.01780120055007
8. Liddle PF, Ngan ETC, Caissie SL, Anderson CM, Bates AT, Quested DJ, et al. Thought and Language Index: An instrument for assessing thought and language in schizophrenia. Br J Psychiatry: J Ment Sci. (2002) 181:326–30. doi: 10.1192/bjp.181.4.326
9. Chen E, Lam L, Kan CS, Chen R. Language disorganisation in schizophrenia: validation and assessment with A new clinical rating instrument. Hong Kong J Psychiatry. (1996) 6:4–13.
10. Barrera A, McKenna PJ, Berrios GE. Two new scales of formal thought disorder in schizophrenia. Psychiatry Res. (2008) 157:225–34. doi: 10.1016/j.psychres.2006.09.017
11. Kircher T, Krug A, Stratmann M, Ghazi S, Schales C, Frauenheim M, et al. A rating scale for the assessment of objective and subjective formal Thought and Language Disorder (TALD). Schizophr Res. (2014) 160:216–21. doi: 10.1016/j.schres.2014.10.024
12. Mutlu E, Abaoğlu H, Barışkın E, Gürel Ş.C, Ertuğrul A, Yazıcı MK, et al. The cognitive aspect of formal thought disorder and its relationship with global social functioning and the quality of life in schizophrenia. Soc Psychiatry Psychiatr Epidemiol. (2021) 56:1399–410. doi: 10.1007/s00127-021-02024-w
13. Nagels A, Fährmann P, Stratmann M, Ghazi S, Schales C, Frauenheim M, et al. Distinct neuropsychological correlates in positive and negative formal thought disorder syndromes: the thought and language disorder scale in endogenous psychoses. Neuropsychobiology. (2016) 73:139–47. doi: 10.1159/000441657
14. Bora E, Yalincetin B, Akdede BB, Alptekin K. Neurocognitive and linguistic correlates of positive and negative formal thought disorder: A meta-analysis. Schizophr Res. (2019) 209:2–11. doi: 10.1016/j.schres.2019.05.025
15. Oeztuerk OF, Pigoni A, Wenzel J, Haas SS, Popovic D, Ruef A, et al. The clinical relevance of formal thought disorder in the early stages of psychosis: Results from the PRONIA study. Eur Arch Psychiatry Clin Neurosci. (2022) 272:403–13. doi: 10.1007/s00406-021-01327-y
16. Remberk B, Namysłowska I, Rybakowski F. Clinical and cognitive correlates of formal thought disorder in early onset schizophrenia. Neuro Endocrinol Lett. (2012) 33:347–55. doi: 10.1016/j.pnpbp.2012.07.007
17. Remberk B, Namysłowska I, Rybakowski F. Cognition and communication dysfunctions in early-onset schizophrenia: Effect of risperidone. Prog Neuropsychopharmacol Biol Psychiatry. (2012) 39:348–54. doi: 10.1016/j.pnpbp.2012.07.007
18. Yalincetin B, Bora E, Binbay T, Ulas H, Akdede BB, Alptekin K. Formal thought disorder in schizophrenia and bipolar disorder: A systematic review and meta-analysis. Schizophr Res. (2017) 185:2–8. doi: 10.1016/j.schres.2016.12.015
19. Caplan R, Foy JG, Asarnow RF, Sherman T. Information processing deficits of schizophrenic children with formal thought disorder. Psychiatry Res. (1990) 31:169–77. doi: 10.1016/0165-1781(90)90119-p
20. Pilon F, Boisvert M, Potvin S. Losing the chain of thought: A meta-analysis of functional neuroimaging studies using verbal tasks in schizophrenia. J Psychiatr Res. (2024) 169:238–46. doi: 10.1016/j.jpsychires.2023.11.013
21. Zakowicz P, Skibińska M, Waśniewski F, Skulimowski B, Pawlak J. Plasma biomarkers in adolescents with schizophrenia-spectrum disorder. Early Intervention Psychiatry. (2023) 17:1154–61. doi: 10.1111/eip.13414
22. Mueller ST, Piper BJ. The psychology experiment building language (PEBL) and PEBL test battery. J Neurosci Methods. (2014) 222:250–9. doi: 10.1016/j.jneumeth.2013.10.024
23. Li M, Li Y, Sun J, Shao D, Yang Q, Cao F. Variability of ecological executive function in children and adolescents genetically at high risk for schizophrenia: A latent class analysis. Eur Child Adolesc Psychiatry. (2019) 28:237–45. doi: 10.1007/s00787-018-1168-2
24. MacKenzie LE, Patterson VC, Zwicker A, Drobinin V, Fisher HL, Abidi S, et al. Hot and cold executive functions in youth with psychotic symptoms. psychol Med. (2017) 47:2844–53. doi: 10.1017/S0033291717001374
25. Wiguna T, Guerrero APS, Honjo S, Ismail I, Wr NS, Kaligis F. Executive dysfunction among children with antipsychotic treated schizophrenia. Clinical Psychopharmacology and Neuroscience. (2014) 12:203–8. doi: 10.9758/cpn.2014.12.3.203
26. Ueland T, Øie M, Inge Landrø N, Rund BR. Cognitive functioning in adolescents with schizophrenia spectrum disorders. Psychiatry Res. (2004) 126:229–39. doi: 10.1016/j.psychres.2004.02.014
27. Galaverna FS, Morra CA, Bueno AM. Severity of negative symptoms significantly affects cognitive functioning in patients with chronic schizophrenia: The slowing in cognitive processing. Eur J Psychiatry. (2014) 28:145–53. doi: 10.4321/S0213-61632014000300002
28. Merrill AM, Karcher NR, Cicero DC, Becker TM, Docherty AR, Kerns JG. Evidence that communication impairment in schizophrenia is associated with generalized poor task performance. Psychiatry Res. (2017) 249:172–9. doi: 10.1016/j.psychres.2016.12.051
29. Minor KS, Marggraf MP, Davis BJ, Mehdiyoun NF, Breier A. Affective systems induce formal thought disorder in early-stage psychosis. J Abnormal Psychol. (2016) 125:537–42. doi: 10.1037/abn0000156
30. Leube D, Whitney C, Kircher T. The neural correlates of ego-disturbances (passivity phenomena) and formal thought disorder in schizophrenia. Eur Arch Psychiatry Clin Neurosci. (2008) 258 Suppl 5:22–7. doi: 10.1007/s00406-008-5017-z
31. Nagels A, Kircher T. Chapter 71—Symptoms and Neurobiological Models of Language in Schizophrenia. In: Hickok G, Small SL, editors. Neurobiology of Language. Irvine, CA, USA: Academic Press (2016). p. 887–97. doi: 10.1016/B978-0-12-407794-2.00071-7
32. Kinney DK, Holzman PS, Jacobsen B, Jansson L, Faber B, Hildebrand W, et al. Thought disorder in schizophrenic and control adoptees and their relatives. Arch Gen Psychiatry. (1997) 54:475–9. doi: 10.1001/archpsyc.1997.01830170101013
33. Lichtenstein P, Yip BH, Björk C, Pawitan Y, Cannon TD, Sullivan PF, et al. Common genetic determinants of schizophrenia and bipolar disorder in Swedish families: A population-based study. Lancet (London England). (2009) 373:234–9. doi: 10.1016/S0140-6736(09)60072-6
Keywords: child and adolescent psychosis, machine learning, cognitive psychiatry, executive deficits, formal thought disorder
Citation: Zakowicz PT, Brzezicki MA, Levidiotis C, Kim S, Wejkuć O, Wisniewska Z, Biernaczyk D and Remberk B (2025) Detection of formal thought disorders in child and adolescent psychosis using machine learning and neuropsychometric data. Front. Psychiatry 16:1550571. doi: 10.3389/fpsyt.2025.1550571
Received: 23 December 2024; Accepted: 03 March 2025;
Published: 17 March 2025.
Edited by:
Amit Singhal, Netaji Subhas University of Technology, IndiaReviewed by:
Animesh Kumar Paul, University of Alberta, CanadaCopyright © 2025 Zakowicz, Brzezicki, Levidiotis, Kim, Wejkuć, Wisniewska, Biernaczyk and Remberk. This is an open-access article distributed under the terms of the Creative Commons Attribution License (CC BY). The use, distribution or reproduction in other forums is permitted, provided the original author(s) and the copyright owner(s) are credited and that the original publication in this journal is cited, in accordance with accepted academic practice. No use, distribution or reproduction is permitted which does not comply with these terms.
*Correspondence: Przemysław T. Zakowicz, cHpha293aWN6QG5ldXJvbG9naWNhbHNvY2lldHkub3Jn
†These authors have contributed equally to this work and share first authorship
Disclaimer: All claims expressed in this article are solely those of the authors and do not necessarily represent those of their affiliated organizations, or those of the publisher, the editors and the reviewers. Any product that may be evaluated in this article or claim that may be made by its manufacturer is not guaranteed or endorsed by the publisher.
Research integrity at Frontiers
Learn more about the work of our research integrity team to safeguard the quality of each article we publish.