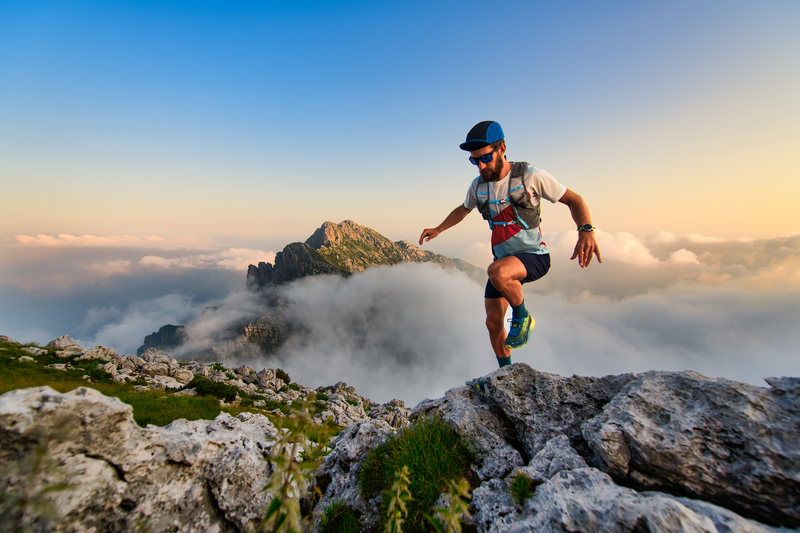
95% of researchers rate our articles as excellent or good
Learn more about the work of our research integrity team to safeguard the quality of each article we publish.
Find out more
OPINION article
Front. Psychiatry
Sec. Digital Mental Health
Volume 16 - 2025 | doi: 10.3389/fpsyt.2025.1535309
This article is part of the Research Topic Technological Advances in Psychiatric Treatments: A Focused Exploration of Human-Computer Interaction (HCI) and Human Factors in Digital Therapeutics View all 4 articles
The final, formatted version of the article will be published soon.
You have multiple emails registered with Frontiers:
Please enter your email address:
If you already have an account, please login
You don't have a Frontiers account ? You can register here
Schizophrenia is a psychotic disorder characterized by hallucinations, delusions, disorganized thought and behavior, and psychomotor abnormalities. Cognitive deficits are core features, contributing to long-term morbidity and poor functional outcomes (McCutcheon, 2023). Thus, addressing cognitive deficits is a top research priority. However, the effectiveness of treatments for cognitive impairment associated with schizophrenia (CIAS) remains uncertain. Research suggests that atypical antipsychotics generally have a beneficial effect on neurocognitive deficits (Hou et al., 2020;Baldez et al., 2021). However, many atypical antipsychotics are associated with metabolic side effects, such as weight gain, diabetes, and elevated cholesterol or triglyceride levels, which can potentially exacerbate cognitive impairments (MacKenzie et al., 2018). Cariprazine offers better cognitive improvement in schizophrenia compared to atypical antipsychotics, but it also has some side effects such as akathisia, extrapyramidal symptoms, weight gain, etc (Laszlovszky et al., 2021). (Lahera et al., 2021), and Computerized cognitive remediation therapy (Yamanushi et al., 2024) effectively enhance cognitive function, social interaction, or working memory in patients with schizophrenia. While these approaches offer benefits, they are also limited by factors such as the requirements for professionals in cognitive training and physical training environments. Recent research has explored using digital technology combined with traditional cognitive training to address CIAS. This approach replaces traditional training with digital systems, including PC applications, mobile apps, and immersive virtual reality (VR) environments, which have been shown to improve cognitive abilities. However, more quantitative methods are needed to evaluate the effectiveness of these interventions and adjust training programs. Given the non-invasive, convenient, and high-temporal-resolution nature of electroencephalogram (EEG) signals, researchers often use them to assess cognitive functions in various brain-computer interface (BCI) tasks (Wen et al., 2020).EEG signal analysis has been employed to evaluate the effectiveness of spatial memory training using VR software (Wen et al., 2023). However, significant variability in EEG signals across individuals and tasks diminishes the generalizability of EEG analysis algorithms (Wu et al., 2022).To address these challenges, researchers have developed cross-subject EEG signal analysis methods to enhance model generalization across different individuals. For instance, the FMLAN framework (Yu et al., 2025) has been applied to cross-subject emotion recognition, while the DDA model (Zhou et al., 2023) has been utilized for cross-subject cognitive workload recognition. And others have focused on cross-task EEG signal analysis to enhance model generalization across different tasks (Duan et al., 2023;Wang et al., 2024). Nonetheless, there is a lack of research that simultaneously addresses both cross-subject and cross-task EEG signal analysis in cognitive assessment.Current digital therapies for treating CIAS have several limitations, such as monotonous scenarios and low immersion, which lead to decreased patient adherence and retention rates. This study reviews the literature on digital therapeutics for CIAS, examines the challenges, and offers recommendations for advancement from the perspective of computer science and technology.Stakeholders benefiting from these advancements include clinicians (improved diagnostic tools), patients (better engagement and outcomes), and policymakers (cost-effectiveness and scalability).Research shows that digital therapeutics can address the unique needs of schizophrenia patients, such as enhancing social skills (Campellone et al., 2023), and have significant potential to improve outcomes in these patients (Fulford et al., 2024). Additionally, digital therapeutics may improve visual attention and logical memory in individuals with schizophrenia during the early stages of the disorder (Fernandez-Gonzalo et al., 2015). Active video games can enhance prefrontal activity in schizophrenia patients, facilitating their cognitive rehabilitation (Shimizu et al., 2017) (Wang et al., 2022). Moreover, VR-SCIT has proven to be more effective than traditional SCIT, leading to improvements in social functioning and emotion perception in patients with schizophrenia (Shen et al., 2022). Review demonstrated that fully immersive VR can serve as a valuable cognitive rehabilitation intervention for mental illnesses, including schizophrenia (Jahn et al., 2021), with effective clinical feasibility outcomes (Perra et al., 2023).These findings collectively highlight the potential of VR in enhancing cognitive functions, such as working memory, executive function, social functioning, and emotion perception in individuals with schizophrenia. The immersive nature of VR can boost patient engagement and treatment adherence (Shen et al., 2022). It is important to note that VR games may induce cybersickness in patients during gameplay. Therefore, both software and hardware developers should focus on reducing sensory conflicts within the game to mitigate this issue (Shafer et al., 2019). Current VR use for enhancing cognitive function in schizophrenia has several limitations. The VR scenarios often lack diversity, with repetitive tasks like object sorting or navigation exercises, which reduces cognitive stimulation.Moreover, monotonous environments, such as generic virtual rooms or simplistic landscapes, can lead to decreased patient engagement and motivation.Another significant issue is the insufficient personalization of VR scenes. For example, patients may face tasks in environments that do not reflect their daily challenges, such as navigating a virtual supermarket or interacting with avatars in unrealistic settings. These generic setups fail to replicate the complexities and nuances of real-world situations, limiting the relevance of the training. VR scenarios currently used in research often lack diversity and personalized reproduction of real scenes, underscoring the need for more innovative and adaptable VR designs to create meaningful, contextspecific interventions tailored to individual needs.In studies investigating digital therapeutics for CIAS, cognitive level assessment mainly uses traditional cognitive assessment methods, such as rating scale. These conventional approaches often rely on subjective judgment, making participants vulnerable to environmental influences, which can lead to errors and diminish the validity and reliability of the results. In contrast, EEG signals, due to their non-invasive nature and the effectiveness of analysis techniques in filtering out external noise, offer a more objective and precise assessment. This technological advancement is propelling cognitive assessment into a new dimension. Wen et al. explored a feature extraction method for analyzing EEG signals to assess spatial cognitive abilities (Wen et al., 2023), offering an innovative and scientifically robust approach to evaluating patients' cognitive abilities. However, the variability of EEG signals between individuals and tasks can impact the generalizability of EEG analysis algorithms. To address this issue, the EEG signal analysis algorithm is being explored from two perspectives: cross-subject and cross-task.1) Cross-subject EEG signal analysis: This approach aims to address individual differences in EEG data, thereby improving the generalization performance of models. The FMLAN framework, based on multiple sub-networks and a Fine-grained Alignment Module, has significantly advanced crosssubject EEG emotion recognition (Yu et al., 2025). Similarly, the DDA model, incorporating EEG feature extraction, label classification, feature distribution alignment, and domain discrimination, has shown strong performance in cross-subject EEG classification (Zhou et al., 2023). 2) Cross-task EEG signal analysis: This method seeks to manage differences between tasks. CTNAS-EEG framework can automatically design the network structure across tasks and improve the recognition accuracy of EEG signals (Duan et al., 2023). Furthermore, SCDA model achieved the highest average EEG-based mental workload cross-task classification accuracy (75.39% ± 9.56% on experiment data, 90.98% ± 9.36% on the COG-BCI public dataset) (Wang et al., 2024).Research on cross-subject and cross-task EEG signal analysis is enhancing generalization capabilities, offering new insights into cognitive evaluation and optimizing training strategies in CIAS. However, integrating both cross-subject and cross-task analysis in EEG-based cognitive evaluation remains a challenge, especially in developing a generalized model to predict cognitive performance across diverse patients and tasks, requiring further exploration. In the future, cross-subject and cross-task EEG analysis could benefit from integrating EEG with AI models or expanding datasets to include underrepresented populations.The previous section explored digital therapeutics for CIAS, showing promising results but requiring further investigation. This section looks at future pathways and emerging trends in digital therapeutics for schizophrenia, focusing on how innovations like VR, BCI, and AI could offer new solutions for this complex disorder. Here is the conceptual map for the future.Schizophrenia patients often show low treatment engagement. Personalized VR scenarios can stimulate patients' interest, leading to better treatment outcomes and a more positive recovery outlook.The rapid advancement of AI-generated content (AIGC) has enabled the generation of VR content, including 3D objects and 360-degree images, based on textual prompts, sketches, images, and other inputs (Cai et al., 2024). The 3D Gaussian splatting algorithm allows for fast real-world scene 3D reconstruction from multi-view images, making it possible to rapidly generate interactive and realistic VR environments (Kerbl et al., 2023;Jiang et al., 2024). Using 3D reconstruction and AIGC, along with user language text, images, and videos, to craft personalized and engaging VR scenarios for CIAS training will significantly enhance patient engagement, making the treatment process both efficient and enjoyable. For example, generating diverse VR scenarios, such as space and underwater, or reconstructing real scenes familiar to patients, such as their home, community, and streets, will more effectively support and enhance the training process. 3) Developing AI cognitive rehabilitation specialists to enhance the efficiency and accessibility of rehabilitation servicesAs AI technology evolves, AI conversational systems, such as GPT (Achiam et al., 2023) andLLaMA (Touvron et al., 2023), have become more refined. Integrating these systems with cognitive rehabilitation and developing AI rehabilitation specialists can replace traditional professionals, reduce healthcare costs, and address workforce shortages.This innovative approach will improve the efficiency and reach of rehabilitation services, providing personalized cognitive rehabilitation plans for each patient with CIAS and ensuring precision and customization throughout the rehabilitation process. Imagine that each patient will have their own individual AI cognitive rehabilitation specialist who will remind or cooperate with the patient for cognitive rehabilitation training every day, and automatically develop a more reasonable training plan for the next session based on the patient's training situation, which is very helpful for the patient's cognitive rehabilitation. However, ethical concerns, such as potential bias in AI-driven systems, must be addressed. Regular audits and transparent algorithms can be implemented to ensure reliability and mitigate these risks.This paper explores digital therapeutics for cognitive impairments associated with schizophrenia, highlighting their flexibility and adaptability in cognitive training compared to traditional methods.Besides, the use of VR and EEG analysis can further enhance the effectiveness of cognitive training and assessment. However, current VR scenes for cognitive training always lack diversity, personalization, and realistic scenes. And quantitative EEG-based cognitive assessments remain constrained in their applicability to cross-subject and cross-task scenarios. Therefore, we studied the emerging directions in digital therapeutics for CIAS from the perspective of computer science and technology, focusing on three key areas: 1) enhancing the diversity of VR scenes through real scene reconstruction and 3D AIGC, 2) advancing cross-subject and cross-task cognitive assessment methods using EEG-based BCI models, and 3) developing AI-driven cognitive rehabilitation specialists by integrating conversational AI systems to reduce human labor. We believe that the application of these innovative technologies will not only significantly improve treatment outcomes but also provide patients with a more personalized and precise therapeutic experience for CIAS, while making treatments more accessible and reducing healthcare inequalities.
Keywords: Schizophrenia, digital therapeutics, cognitive impairment, VR, BCI, EEG analysis, cross-subject, cross-task 1
Received: 27 Nov 2024; Accepted: 26 Mar 2025.
Copyright: © 2025 Sun, Li, Xie, Xianglong, Liu, Li, Duan, Yu and Wen. This is an open-access article distributed under the terms of the Creative Commons Attribution License (CC BY). The use, distribution or reproduction in other forums is permitted, provided the original author(s) or licensor are credited and that the original publication in this journal is cited, in accordance with accepted academic practice. No use, distribution or reproduction is permitted which does not comply with these terms.
* Correspondence:
Dong Wen, University of Science and Technology Beijing, Beijing, China
Disclaimer: All claims expressed in this article are solely those of the authors and do not necessarily represent those of their affiliated organizations, or those of the publisher, the editors and the reviewers. Any product that may be evaluated in this article or claim that may be made by its manufacturer is not guaranteed or endorsed by the publisher.
Research integrity at Frontiers
Learn more about the work of our research integrity team to safeguard the quality of each article we publish.