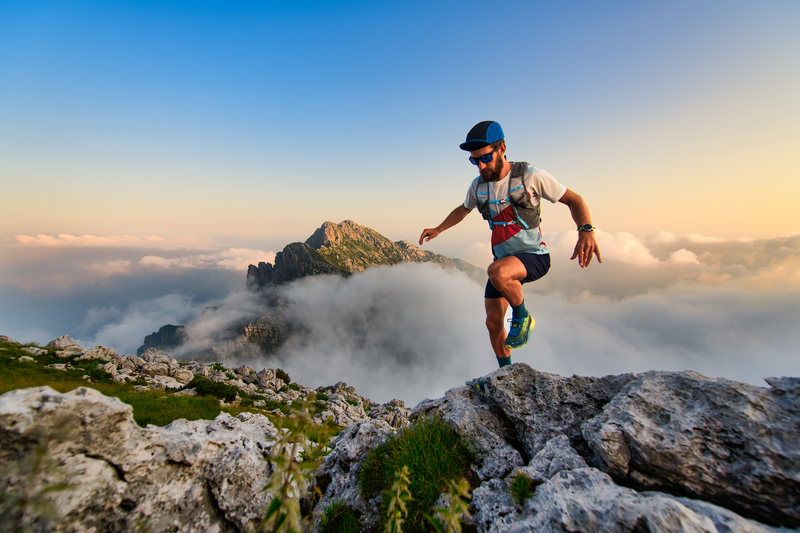
94% of researchers rate our articles as excellent or good
Learn more about the work of our research integrity team to safeguard the quality of each article we publish.
Find out more
ORIGINAL RESEARCH article
Front. Psychiatry
Sec. Mood Disorders
Volume 16 - 2025 | doi: 10.3389/fpsyt.2025.1524046
The final, formatted version of the article will be published soon.
You have multiple emails registered with Frontiers:
Please enter your email address:
If you already have an account, please login
You don't have a Frontiers account ? You can register here
We aimed to investigate the metacognition of patients with major depressive disorder (MDD) and its correlation with their condition, as well as explore its diagnostic significance in the early stages of the disease, thereby providing a reference for clinical treatment. METHODS: Using a cross-sectional research design, we selected 66 patients diagnosed with MDD and 99 healthy controls for a mental rotation task; we examined their metacognitive performance using a post-decisional confidence assessment paradigm. We evaluated various aspects, including their performance on first-order tasks (d'), metacognitive bias (average confidence), metacognitive sensitivity (meta-d'), metacognitive efficiency (the M Ratio). RESULTS: In terms of the first-order task performance (d'), the group with MDD scored significantly lower than the healthy controls (t = -4.274, p < 0.001, respectively). Regarding metacognitive bias(average confidence), metacognitive sensitivity (meta-d'), and metacognitive efficiency (the M ratio), the group with MDD performed significantly worse than the healthy controls (t = -4.280, p < 0.001; t = -3.540, p < 0.001; t = -2.104, p = 0.039, respectively). In addition, the Hamilton Rating Scale for Depression (HAMD-17) scores of the patients with MDD were significantly negatively correlated with their d' , average confidence levels , meta-d' , and M ratio(r = -0.468, p < 0.001; r = -0.601, p < 0.001;r = -0.457, p < 0.001; r = -0.362, p = 0.003),The average confidence levels of MDD patients are significantly positively correlated with d', meta-d', and M ratio. (r = -0.552, p < 0.001; r = 0.738, p < 0.001;r =0.273, p =0.02) .CONCLUSION: The metacognitive abilities of patients with MDD were significantly impaired, and the degree of metacognitive impairment was related to the severity of clinical depressive symptoms. Moreover, the impairment of their metacognitive abilities was correlated with negative metacognitive bias.
Keywords: metacognition, Metacognitive ability, Major Depressive Disorder, Neuropsychology, mental rotation
Received: 07 Nov 2024; Accepted: 17 Feb 2025.
Copyright: © 2025 Hong, Chu, Chen, Chi, Zheng and Tao. This is an open-access article distributed under the terms of the Creative Commons Attribution License (CC BY). The use, distribution or reproduction in other forums is permitted, provided the original author(s) or licensor are credited and that the original publication in this journal is cited, in accordance with accepted academic practice. No use, distribution or reproduction is permitted which does not comply with these terms.
* Correspondence:
Fengfeng Chu, Zhejiang Chinese Medical University, Hangzhou, China
Yuping Zheng, The Fifth People's Hospital of Lin'an District,, Hangzhou, China
Ming Tao, Zhejiang Chinese Medical University, Hangzhou, China
Disclaimer: All claims expressed in this article are solely those of the authors and do not necessarily represent those of their affiliated organizations, or those of the publisher, the editors and the reviewers. Any product that may be evaluated in this article or claim that may be made by its manufacturer is not guaranteed or endorsed by the publisher.
Research integrity at Frontiers
Learn more about the work of our research integrity team to safeguard the quality of each article we publish.