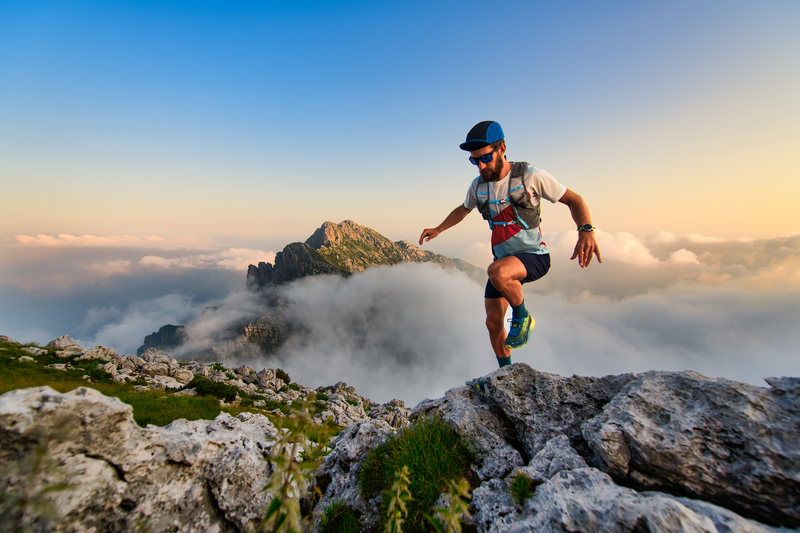
94% of researchers rate our articles as excellent or good
Learn more about the work of our research integrity team to safeguard the quality of each article we publish.
Find out more
ORIGINAL RESEARCH article
Front. Psychiatry
Sec. Social Psychiatry and Psychiatric Rehabilitation
Volume 16 - 2025 | doi: 10.3389/fpsyt.2025.1511841
The final, formatted version of the article will be published soon.
You have multiple emails registered with Frontiers:
Please enter your email address:
If you already have an account, please login
You don't have a Frontiers account ? You can register here
ObjectiveAim -We aimed Tto examine determinants of the prevalence and frequency of criminal victimization (i.e. both personal and property crime victimization) in outpatients with severe mental illness.-Data was collected using a multisite epidemiological survey including a random sample of 956 adult outpatients with SMI. Data were collected between 2010 and 2012. Data on 12-month victimization prevalence and frequency were obtained using the victimization scale of the Dutch Crime and Victimization Survey. Demographic characteristics, clinical determinants e.g Demographic characteristics, clinical diagnosis, psychosocial functioning, drug use and alcohol abuse over the past 12 months, co-morbid PTSD diagnosis, and victimological determinants e.g. physical abuse, physical neglect and sexual abuse in childhood, perpetration of violence over the past 12-months, and anger disposition were assessed as determinants. Univariable and multivariable hurdle regression analyses were conducted to test associations of the potential determinants with victimization prevalence and frequency.Results --Twelve-month prevalence rates of personal and property crime were 19% and 28%, respectively. In total 612, incidents of personal and 447 incidents of property crime were reported. We found that different sets of demographic and clinical characteristics were associated with personal and property crime victimization . Clinical characteristics were more pronounced regarding personal crime victimization. In the multivariable model, presence of psychotic disorder, drug use, childhood physical and sexual abuse, and recent violent perpetration were associated with the 12-month prevalence or frequency rate of personal crime victimization. Native Dutch and divorced patients were more at risk as well. Next to this being employed, poor social functioning, having perpetrated a violent crime, as well as alcohol abuse and recent drug use were all significantly related to property crime prevalence or frequency rate
Keywords: Victimization, Violence, Crime, determinants, Psychiatric patients, Epidemiology
Received: 15 Oct 2024; Accepted: 11 Feb 2025.
Copyright: © 2025 Kamperman, Zarchev, Henrichs, Willemsen, Lesaffre, Swildens, Nijssen, Kroon, Van Schaik, Van Der Gaag, Delespaul, Van Weeghel, Van De Mheen, Bogaerts and Mulder. This is an open-access article distributed under the terms of the Creative Commons Attribution License (CC BY). The use, distribution or reproduction in other forums is permitted, provided the original author(s) or licensor are credited and that the original publication in this journal is cited, in accordance with accepted academic practice. No use, distribution or reproduction is permitted which does not comply with these terms.
* Correspondence:
Astrid M. Kamperman, Erasmus Medical Center, Rotterdam, Netherlands
Disclaimer: All claims expressed in this article are solely those of the authors and do not necessarily represent those of their affiliated organizations, or those of the publisher, the editors and the reviewers. Any product that may be evaluated in this article or claim that may be made by its manufacturer is not guaranteed or endorsed by the publisher.
Research integrity at Frontiers
Learn more about the work of our research integrity team to safeguard the quality of each article we publish.