- 1Laboratory of Integrative Neuroscience (LiNC), Universidade Federal de São Paulo (UNIFESP), São Paulo, Brazil
- 2Post-Graduation Program in Psychiatry and Medical Psychology, UNIFESP, São Paulo, Brazil
- 3National Institute of Developmental Psychiatry & National Center for Innovation and Research in Mental Health (CISM), Sao Paulo, Brazil
- 4Genetics Division, Department of Morphology and Genetics, UNIFESP, São Paulo, Brazil
- 5Centro de Oncologia Molecular, Hospital Sírio-Libanês, São Paulo, Brazil
- 6National Institute of Developmental Psychiatry & National Center for Innovation and Research in Mental Health (CISM), Porto Alegre, Brazil
- 7Department of Psychiatry, Hospital de Clínicas de Porto Alegre, Universidade Federal do Rio Grande do Sul (UFRGS), Porto Alegre, Brazil
- 8Department of Neuropsychiatry, Universidade Federal de Santa Maria (UFSM), Santa Maria, Brazil
- 9Mental Health Epidemiology Group (MHEG), Universidade Federal de Santa Maria (UFSM), Santa Maria, Brazil
- 10Graduate Program in Psychiatry and Behavioral Sciences, UFRGS, Porto Alegre, Brazil
- 11Care Policy and Evaluation Centre, London School of Economics and Political Science, London, United Kingdom
- 12Department of Psychiatry, Queen’s University School of Medicine, Kingston, ON, Canada
- 13Translational Neuropsychiatry Unit, Aarhus University, Aarhus, Denmark
- 14ADHD Outpatient Program & Developmental Psychiatry Program, Hospital de Clinicas de Porto Alegre, Federal University of Rio Grande do Sul, Porto Alegre, Brazil
- 15Medical Council, Centro Universitário de Jaguariúna (UNIFAJ), Jaguariúna, Brazil
- 16Medical Council, Centro Universitário Max Planck (UNIMAX), Indaiatuba, Brazil
- 17Departamento de Psiquiatria do Hospital das Clínicas da Faculdade de Medicina da Universidade de São Paulo, São Paulo, Brazil
- 18Disciplina de Biologia Molecular, Departamento de Bioquímica, UNIFESP, São Paulo, Brazil
- 19Department of Global Initiatives, Child Mind Institute, New York, NY, United States
Introduction: While the influence of both genetic and environmental factors on the development of psychiatric symptoms is well-recognized, the precise nature of their interaction throughout development remains a subject of ongoing debate. This study investigated the association between the expression of 78 candidate genes, previously associated with psychiatric phenotypes, in peripheral blood and both adversity and psychopathology in a sample of 298 young individuals assessed at two time points from the Brazilian High Risk Cohort Study for Mental Conditions (BHRCS).
Methods: Psychopathology was assessed using the Child Behavior Checklist (CBCL), considering the total CBCL, p-factor (i.e. general factor of psychopathology), and internalizing and externalizing symptoms as clinical variables. The life adversities considered in this study includes four composite variables: child maltreatment, stressful life events, threat and deprivation. Gene expression was measured using next-generation sequencing for target genes and differential gene expression was analyzed with the DESeq2 package.
Results: Mixed models revealed six genes associated with internalizing symptoms: NR3C1, HSPBP1, SIN3A, SMAD4, and CRLF3 genes exhibited a negative correlation with these symptoms, while FAR1 gene showed a positive correlation. Additionally, we also found a negative association between USP38 gene expression and externalizing symptoms. Finally, DENND11 and PRRC1 genes were negatively associated with deprivation, a latent factor characterized by neglect, parental absence, and measures of material forms of deprivation. No mediation or moderation effect was observed of gene expression on the association between life adversities and psychiatric symptoms, meaning that they might influence distinct pathways.
Discussion: Among these nine genes, NR3C1, which encodes a glucocorticoid receptor, is by far the most investigated, being associated with depressive symptoms, early life adversity, and stress. While further research is needed to fully understand the complex relationship between gene expression, life adversities, and psychopathology, our findings provide valuable insights into the molecular mechanisms underlying mental disorders.
1 Introduction
Psychiatric symptoms frequently co-occur, meaning that having one condition increases the risk of developing another (1). Moreover, psychiatry has struggled to define clear boundaries between disorders, and between normal and pathological variation, suggesting that the diagnostic categories may be inadequate (2).
This has led researchers to increasingly focus on transdiagnostic approaches, such as dimensional models, to better understand the complex presentations of mental health. These models often combine into higher-order factors, like internalizing and externalizing symptoms For instance, the internalizing dimension reflects the overlap between depression and anxiety symptoms, while the externalizing dimension captures disruptive disorders and substance abuse (1). Additionally, a general factor of psychopathology, the “p factor,” has been suggested, which captures the shared variance between these dimensions (1, 3).
Previous research found evidence for both genetic and environmental influences on psychiatric symptoms. Epidemiological studies have shown that almost all forms of psychopathology are partly heritable and polygenic, transcending diagnostic boundaries (2, 4), and supporting a transdiagnostic structure of psychopathology. Also, genome-wide association studies (GWAS) have demonstrated that genetic influences are often pleiotropic, affecting multiple psychiatric outcomes (5, 6). However, recent GWASs on internalizing (7), externalizing symptoms (8), and general psychopathology (9), have shown a low single nucleotide polymorphism (SNP) heritability (1.7% - 23.5%), indicating the influence of other genetic and environmental factors on these dimensions. For instance, data obtained from the Brazilian High-Risk Cohort Study for Mental conditions (BHRCS) demonstrated that an increase in stressful life events (SLE) (10), child maltreatment (CM) (11), or threat and deprivation (12) was associated with elevated psychopathology.
However, the molecular mechanisms underlying the complex interplay of genetic and environmental factors in the development of psychopathology remain a subject of ongoing debate. Alterations in gene expression, as well as epigenetic modifications that regulate it, have emerged as promising candidates to elucidate this intricate relationship, given that: i) genetic variants associated with psychopathology are enriched for regulatory elements, suggesting a role in gene expression modulation (13, 14); and ii) gene expression and epigenetic changes are related to both environmental factors and psychopathology (15–18). Of particular interest, a growing body of research has associated exposure to early-life adversities (ELA) with significant changes in gene expression, particularly in genes involved in inflammation and immune system function (19–21). This compelling evidence points towards altered gene expression in leukocytes as a potential mechanism linking immune dysregulation to the increased risk of poor health outcomes commonly observed in individuals who have experienced ELA (20, 22). Supporting this notion, the BHRCS identified a significant indirect effect of the aggregate expression of four genes related to the hypothalamic-pituitary-adrenal (HPA) axis and inflammation, mediating the association between CM and major depressive disorder (MDD) (16). Beyond the impact of ELA, other environmental stressors, such as food restriction and low socioeconomic status, have also been demonstrated to influence gene expression patterns (23, 24). Additionally, a transcriptome-wide association study revealed significant genotype-by-environment interactions, wherein the effects of psychosocial stressors (i.e., deprivation and cumulative lifetime stress) on the risk of MDD were modulated by imputed gene expression levels in both whole blood and brain tissues (25). However, no study to date has comprehensively investigated the longitudinal dynamics of blood gene expression and its interaction with psychopathology and life adversities, nor the interplay between these factors in a cohort of children and adolescents.
Thus, this study aims to investigate within the BHRCS the longitudinal association between blood candidate gene expression and adversity (i.e. SLE, CM, threat and deprivation) or psychopathology (total Child Behavior Checklist (CBCL) score, p factor, internalizing and externalizing problems). Additionally, we aim to investigate the potential mediation or moderation role of gene expression in the relationship between life adversities and psychiatric symptoms (Supplementary Figure S8).
2 Methods
2.1 Study procedures
In this study, we investigated a sample of 320 individuals (Figure 1) from the Brazilian High Risk Cohort Study (BHRCS), a large, prospective, community school-based study enriched for high familial risk of psychopathology. Details of the cohort characteristics and study design are described elsewhere (26). Briefly, this cohort is initially comprised 2,511 children and adolescents, with two-thirds presenting a high risk for developing mental conditions based on the presence of early psychiatric symptoms and high family loading of psychopathology. We assessed subjects from two Brazilian cities (Sao Paulo and Porto Alegre) at two different time points: time point 1 (baseline, N=2,511) and time point 2, after 3-year follow-up (N=2,010), with a retention rate of 80%. The total sample comprises children with an average age of 9.7 years, of which 53.1% are male.
For both time points, evaluations were conducted over multiple visits, including a household parent interview and a blood sample collection. The ethics committees of the Institutions involved in the cohort approved the project (the Faculdade de Medicina da Universidade de Saão Paulo (IORG0004884), the Universidade Federal de Saão Paulo (CAAE: 74563817.7.2002.5505), and the Universidade Federal do Rio Grande do Sul (CAAE: 74563817.7.1001.5327). The study was conducted in accordance with approved guidelines and, written informed consent or assent was obtained from all parents and children/youths before their inclusion in the cohort.
2.2 Measures of psychopathology
Psychopathology was assessed using the Child Behavior Checklist (CBCL) at all timepoints, including total CBCL score, p-factor, internalizing, and externalizing domains. While the total score and p-factor are highly correlated (the former being the sum of all items, while the latter reflects a non-specific liability to psychopathology), both were examined to determine whether bifactor models better captured biological variation compared to the total score alone. Moreover, the p-factor refers to a shared factor of psychopathology, while the internalizing problems score aggregates anxious-depressed, withdrawn-depressed, and somatic complaints symptoms. The externalizing problems score refers to rule-breaking behavior and aggressive behavior. Confirmatory factor analysis (CFA) was conducted using a bifactor model in which all items are loaded onto a general factor (the p-factor) and residual variance is captured by internalizing and externalizing domains as outlined by the CBCL scoring system (12).
2.3 Measures of life adversities
Three environmental variables — stressful life events (SLE), child maltreatment (CM), and threat/deprivation — were constructed based on multiple questionnaires. Detailed information on the generation of these variables is provided in the Supplementary Information.
2.3.1 Stressful life events
It was calculated using a Life History Schedule answered by parents at time point 2 (N=2010). This reported exposure to 13 different stressors over the three previous years (between time point 1 and time point 2). Briefly, after exploration of the factor structure using Exploratory Factor Analysis and Confirmatory Factor Analysis (Supplementary Information), we used a 1-factor solution comprising nine indicators composed of experiences of family distress (parents’ unemployment, divorce, or death, and family fights, or financial problems), friend or family member’s illnesses or death, loss of a pet and household loss due to a natural disaster (flood or fire).
2.3.2 Child maltreatment
Child maltreatment (CM) was assessed using questionnaires specifically designed for the BHRCS and answered by children and parents covering physical/emotional abuse and neglect, and sexual abuse, as we described in detail previously (11). For the analysis, we used the categorical classification of the children’s level of exposure described in (11).This variable was calculated for all time points (time point 1: N=2511; time point 2: N=2,010).
2.3.3 Threat and deprivation
Threat, defined as the presence of unexpected events endangering the physical integrity or well-being of the child (e.g., abuse, violence), contrasts with deprivation, characterized by neglect, parental absence, and measures of material forms of deprivation (e.g., neglect, poverty). Factor analysis was conducted to assess the latent structure of these experiences at time point 1. Threat was measured using four indicators from The Posttraumatic Stress Disorder (PTSD) assessment of the DAWBA (physical abuse, attack or threat, domestic violence witnessing, attack witnessing); and seven additional questions: Bullying exposure (parent report), Bullying exposure (child report), Physical abuse (parent report), Physical abuse (child report), Emotional abuse (parent report), Emotional abuse (child report), Sexual abuse (total). For deprivation, six indicators were considered to measure deprivation according questionnaires applied in our cohort: Mother’s educational level, socioeconomic classification according to Brazilian Economic Classification Criterion (A/B — the wealthiest, C, or D/E — the poorest), father presence (in contact, noncontact, deceased, or unknown), neglect (parent report), Neglect (child report), Family income. These variables were described in detail previously (12).
2.4 Gene expression
We selected only participants with blood samples collected at both time points (N=320). The workflow of the study sample is depicted in Figure 1. Blood samples were collected in PAXgene RNA tubes (PreAnalytix, Switzerland), and leukocyte RNA was extracted from whole blood using the PAXgene Blood RNA Kit (Qiagen, Germany). RNA quality and quantity were assessed with the NanoDrop™ 1000 Spectrophotometer (Thermo Fisher Scientific) and the Qubit 2.0 fluorometer with the Qubit RNA BR Assay Kit (Thermo Fisher Scientific), respectively. DNA contamination was verified on a 1.5% agarose gel, and RNA integrity was evaluated using the Agilent 2100 Bioanalyzer and RNA 6000 Nano Kit (Agilent). Gene expression was measured using next-generation sequencing with the TruSeq® Targeted RNA Expression protocol (Illumina). Genes were selected based on four previous studies, two of them in the BHRCS. Briefly, 55 genes exhibited differential expression in the blood of BHRCS individuals who transitioned from low to high psychiatric symptoms over a 3-year follow-up period, indicating the onset of symptoms (15). Conversely, four genes were differentially expressed in individuals who showed a reduction in psychiatric symptoms, suggesting remission (15). Additionally, three genes displayed differential expression in the blood of BHRCS participants with major depressive disorder (16). However, it is important to note that these two previous studies involved a smaller sample size from the BHRCS. Furthermore, we included 20 genes known to be differentially expressed in the brains of individuals with autism spectrum disorders, schizophrenia, bipolar disorder, depression, or general mental health conditions (27), as well as 6 endogenous control genes for blood samples for normalization procedures (28). The probes sequences and references are provided in Supplementary Table S4. A total of 100 ng of RNA was reverse transcribed into cDNA using ProtoScript II Reverse Transcriptase (New England Biolabs). Libraries were prepared using the TruSeq Targeted RNA Expression Kit and the TruSeq® Targeted RNA Custom Oligonucleotide Pool (Illumina). Libraries were pooled and sequenced on the NextSeq 500 instrument (Illumina) using the MidOutput v2 kit (150 cycles) and 100 single end reads.
To quantify gene expression, we downloaded the transcript list (manifest file) provided by Illumina (Supplementary Table S4). We then converted the target coordinates from the hg19 reference genome to the hg38 reference genome using the liftOver tool. Each target was assigned a unique GENCODE ID by comparing its coordinates with genes annotated by GENCODE [version 38 (29)] using the bedtools intersect tool [default parameters (30)]. To guarantee precise GENCODE ID assignment, a manual verification was performed. Other coding genes that overlapped with the targets in the GENCODE annotated transcripts were removed. Kallisto (31) was used to initiate the quantification of target expression. We generated a transcript index based on this new transcript list (excluding overlapping genes) using the command “kallisto index -i”. Kallisto was then executed for each sample individually (single end mode). The analysis considered the average read length calculated for each sample and a standard deviation of 0.0000001. To quantify gene-level expression, the tximport tool (32) was employed. A pre-filtering step was applied to remove genes with low read counts (less than 10 reads in 10 samples). Read counts were then normalized using the DESeq2 package and the variance stabilizing transformation (VST) function. Finally, outlier values were removed, and the data was standardized.
2.5 Statistical analysis
For descriptive analyses comparing time point 1 and time point 2 assessments, we employed paired t-tests for continuous variables and McNemar’s chi-squared tests for categorical variables. Pearson’s correlation tests were conducted to examine the relationships between continuous psychopathological variables and life adversities.
Principal Component Analysis (PCA) was performed to assess potential batch effects in the sequencing data. To investigate the association between gene expression and various factors, we utilized mixed-effect models implemented with the lme() function from the nlme R package. Gene expression, measured using normalized read counts, served as the dependent variable in all analyses. To assess the relationship between gene expression and psychopathology (or CM), we performed separate mixed models for each time point (1 and 2). These models included psychopathology (or CM) as fixed effect, along with sequencing run, age and sex. Data collection site was included as a random effect. To identify longitudinal effects and assess how the relationship between gene expression and psychopathology (or CM) changes over time, we fit mixed-effects models that included data collection site and each subject as random variable. In these models, we included psychopathology (or CM), its interaction with time point, time point, sequencing run, age and sex as fixed effects. This analysis followed the model: gene expression ~ psychopathology (or CM) * time point + sequencing run + age + sex + (1|data collection site/individual ID). For the association between gene expression and SLE, threat, or deprivation, separate analyses were performed for each time point due to differences in data availability: time point 1 for threat and deprivation, and time point 2 for SLE. In these mixed models, the respective stressor was included as the fixed effect, with sequencing run, age, and sex as additional fixed effects, and data collection site as a random effect. To identify potential bias due to changes in gene expression over time, we examined the association between gene expression and time point by including time point, sequencing run, and age as fixed effects, with each subject as a random effect.
Pearson correlation was used to assess correlations between the differentially expressed genes. Network analysis was then performed using GeneMania, considering only co-expression networks, max resultant genes = 5 and max resultant attributes =5, and the automatically selected weighting method (33). To understand the role of the differentially expressed genes, we utilized the gene2func function from the FUMA GWAS software (Functional Mapping and Annotation of Genome-Wide Association Studies) (34) to identify enriched gene sets, such as those in Gene Ontology (GO), Kyoto Encyclopedia of Genes and Genomes (KEGG), and Reactome, as well as gene expression patterns in the Genotype-Tissue Expression (GTEx) version 8 and BrainSpan databases.
We also evaluated the interaction and mediation between gene expression and life adversities on psychopathology using the lavaan package (Supplementary Figure S8). The statistical analyses were carried out in R v4.3.3 using RStudio interface. To adjust for multiple comparisons, the Benjamini-Hochberg false discovery rate (FDR) method was applied, using a significance threshold of FDR < 0.05.
3 Results
This study included 298 participants from the BHRCS, selected based on high-quality RNA data at both time point 1 and time point 2. The sample comprised more male participants (n=181, 60.7%) and participants from São Paulo state (n=176, 59.1%).
Comparisons between time point 1 and time point 2 are shown in Table 1. The prevalence of individuals with high CM significantly decreased from time point 1 (66/22.1%) to time point 2 (43/14.4%; p=0.014). Participant age ranged from 5 to 14 years at time point 1 and 9 to 17 years at time point 2. Internalizing domain scores increased significantly from time point 1 (mean=-0.03, SD=0.30) to time point 2 (mean=0.03, SD=0.32; p<0.001).
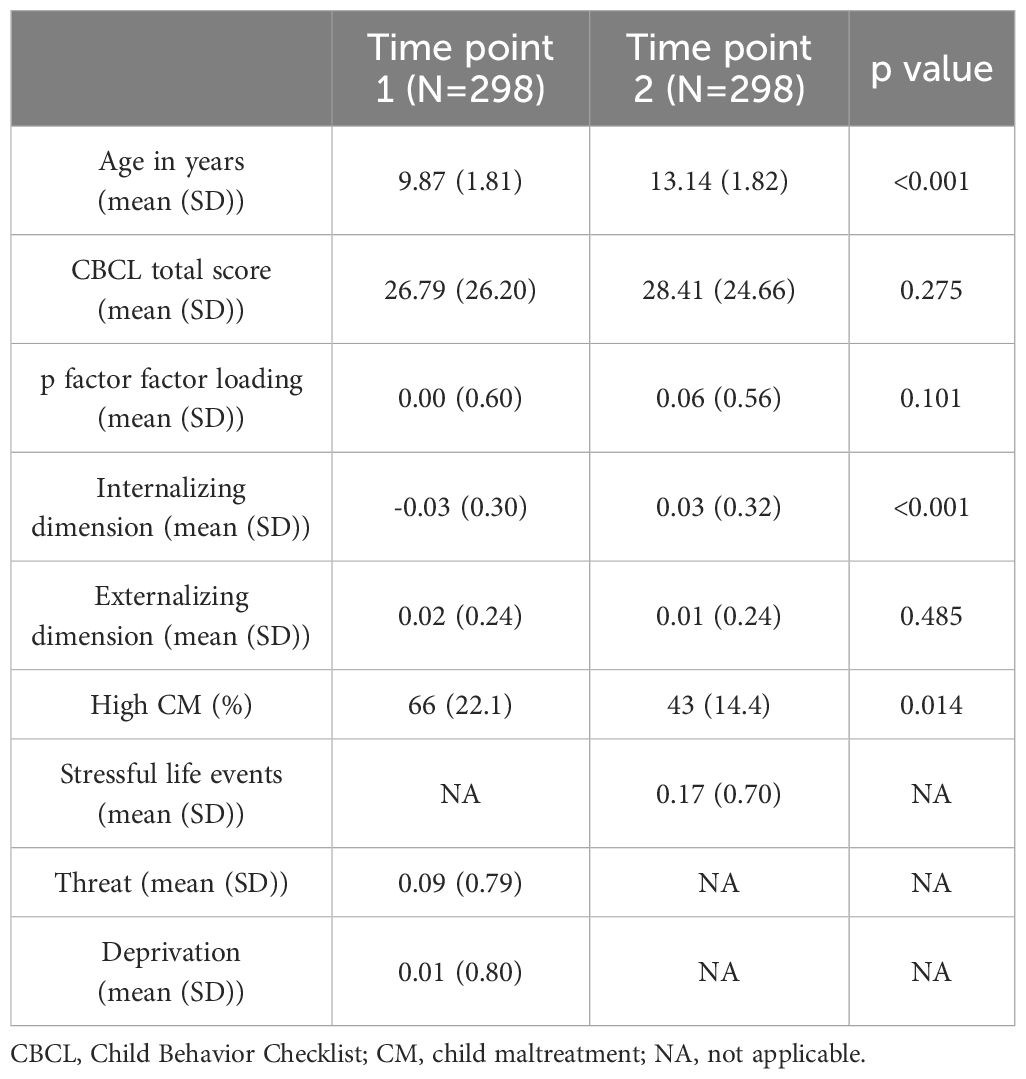
Table 1. Study population characteristics comparing baseline (time point 1) and follow-up (time point 2).
Additionally, this subsample of 298 participants demonstrated a significant association between life events and psychopathology measures (Supplementary Figure S2), consistent with findings from the original study population (12). Also, the threat and deprivation variables calculated for time point 1 are positively correlated with SLE calculated at time point 2 (Supplementary Figure S3). Finally, individuals in the high CM group exhibit more SLE, threat and deprivation than those in the low CM group (Supplementary Figure S4).
Of the 88 target genes, six endogenous genes and four with low expression (fewer than 10 reads in at least 10 samples) were excluded. This resulted in a final set of 78 genes for differential expression analysis. Principal component analysis revealed an effect of the sequencing run, leading us to include this variable as a covariate in further analysis (Supplementary Figure S5A). While no clustering by time point was identified (Supplementary Figure S5B), a total of 22 genes exhibited significant associations with time point (Supplementary Table S5), suggesting that the expression of these genes might change over time.
We investigated the association between expression levels of the 78 target genes and psychopathology, as measured by the total CBCL score, the p factor, internalizing and externalizing specific factors. Analyzing each time point separately, we observed a significant negative association between USP38 gene expression and externalizing symptoms at time point 1 (Table 2; Supplementary Figure S6). At the time point 2, we found significant positive associations between internalizing symptoms and FAR1 expression; and negative associations between internalizing symptoms and the expression of NR3C1, HSPBP1, SMAD4, CRLF3 and SIN3A genes (Table 2; Supplementary Figure S6). However, mixed models revealed no longitudinal changes in the relationship between gene expression and psychopathology.
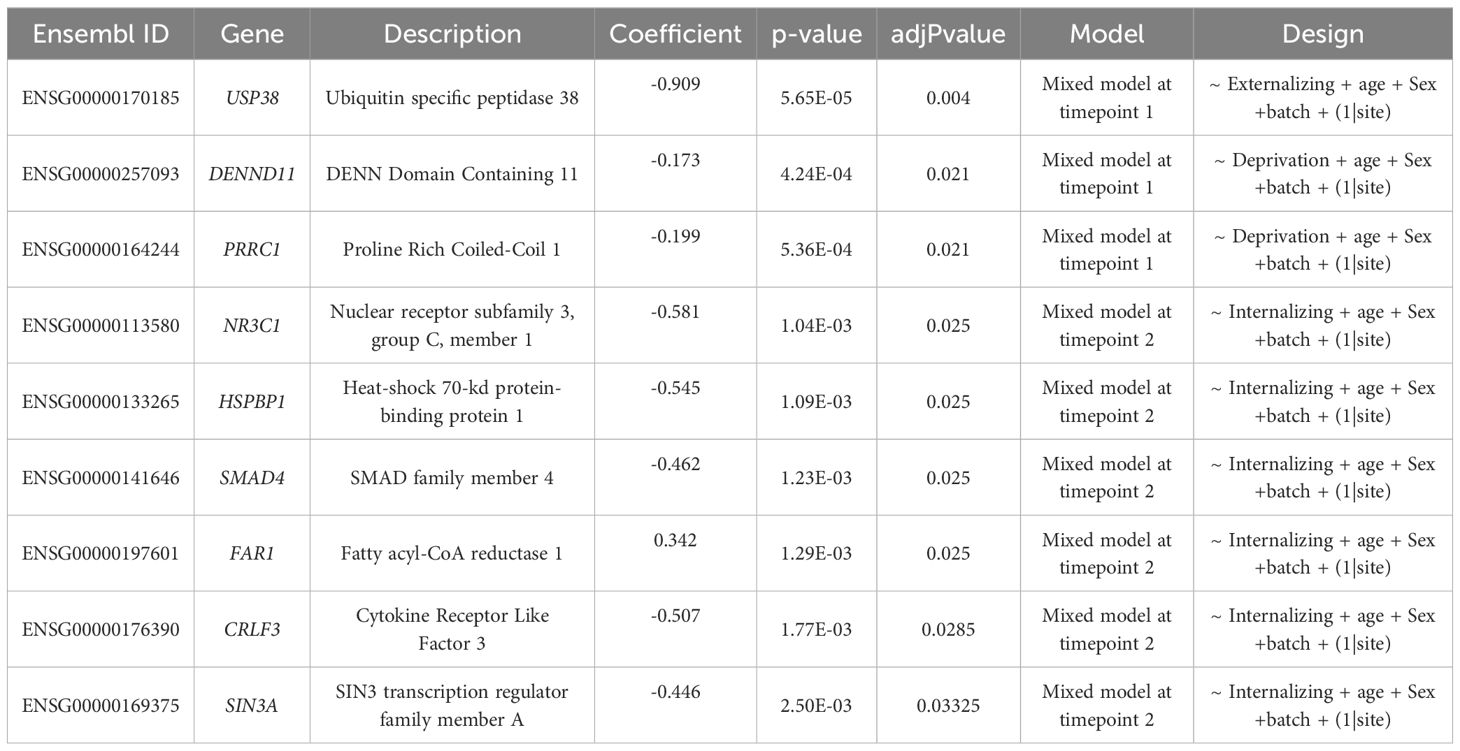
Table 2. Significant results from mixed models comparing gene expression and psychopathology or life adversities.
Regarding the association between gene expression and measures of life adversities, we identified a negative correlation at time point 1 between deprivation and the expression of both DENND11 (or KIAA1147) and PRRC1 (Table 2; Supplementary Figure S6). No associations with other measures of life adversities were identified.
Notably, all nine genes were correlated with each other (Supplementary Figure S7A), suggesting potential co-expression or involvement in shared pathways. GeneMania analysis confirmed this, showing that all genes except HSPBP1 are related by co-expression networks (Supplementary Figure S7B). In particular, NR3C1, SIN3A, and SMAD4, all associated with internalizing symptoms, are correlated with each other (NR3C1-SIN3A: r=0.594, p=2.2E-16; NR3C1-SMAD4: r=0.673, p=2.2E-16; SIN3A-SMAD4: r=0.797, p=2.2E-16; Supplementary Figure S7A). Furthermore, these three genes are enriched in two Reactome pathways: FOXO-mediated transcription of oxidative stress, metabolic, and neuronal genes (R-HSA-9615017; 3/29 genes, p=1.277E-07, FDR=3.946E-04) and FOXO-mediated transcription (R-HSA-9614085; 3/64 genes; p=1.447E-06, FDR=0.001).
Analysis of gene expression databases (Supplementary Figures S7C, D) revealed ubiquitous expression of HSPBP1 (average log2TPM from 3.527 in blood to 5.628 in the uterus; average log2TPM of 5.056 across multiple tissues), with high levels also observed in brain tissues (average log2TPM=5.345). Moreover, DENND11 (average log2TPM = 4.058), PRRC1 (average log2TPM = 3.740), SIN3A (average log2TPM = 3.870), NR3C1 (average log2TPM = 4.002), SMAD4 (average log2TPM = 4.332), and FAR1 (average log2TPM = 4.536) were also highly expressed in multiple tissues. Of note, the average expression across multiple tissues was particularly similar for NR3C1, SMAD4, and SIN3A genes (Supplementary Figure S7C). Interestingly, USP38 expression appears to be more tissue-specific, being highly expressed in muscle (average log2TPM=4.297), whereas CRLF3 is lowly expressed in this tissue (average log2TPM=1.398) and highly expressed in the spleen (average log2TPM=4.406).
Analysis of gene expression in the BrainSpan database (Supplementary Figure S7D) showed that HSPBP1 is also highly expressed in the brain across all developmental stages (average log2RPKM =5.146 in multiple developmental stages), similar to DENND11, although the latter showed more moderate expression (average log2RPKM =3.331 in multiple developmental stages). Notably, while the expression of SIN3A, SMAD4, and PRRC1 appeared higher in prenatal stages (peaking in early prenatal stages: SIN3A log2RPKM=3.878, SMAD4 log2RPKM=4.232, PRRC1 log2RPKM=3.336), NR3C1 expression increased in postnatal stages (peaking in young adulthood: log2RPKM=3.222), similar to FAR1 (peaking in young adulthood, log2RPKM=3.191).
We investigated whether the nine genes were mediators or moderators between the association of life adversities and psychiatric symptoms (Supplementary Figure S8). We tested four mediation or moderation models (considering CM, SLE, threat, and deprivation) for each of the NR3C1, SIN3A, SMAD4, FAR1, CRLF3, HSPBP1 and USP38 genes, which were associated with psychiatric symptoms. We also tested four mediation or moderation models (considering total CBCL score, p-factor, internalizing, and externalizing symptoms) for each of the DENND11 and PRRC1 genes, which were associated with deprivation. This resulted in a total of 36 mediation and 36 moderation models tested. We identified HSPBP1 as a mediator of the association between CM and internalizing symptoms at time point 2 (estimate =0.013; p=0.048). Moreover, we found that DENND11 expression moderated the relationship between deprivation and the p factor (estimate = -0.080, p = 0.0407), CRLF3 expression moderated the relationship between CM and internalizing symptoms (estimate = -0.044, p = 0.043), and NR3C1 expression moderated the relationship between deprivation and internalizing symptoms (estimate = -0.049, p = 0.029) at time point 2. However, these associations were not significant after adjusting for multiple comparisons (adjusted p = 1.000).
4 Discussion
We conducted targeted RNA sequencing to identify genes associated with changes in psychopathology and life adversities. Of the 78 genes examined, six genes (NR3C1, SMAD4, SIN3A, FAR1, CRLF3 and HSPBP1) were associated with internalizing symptoms and one (USP38) with externalizing symptoms. Additionally, we identified two genes (DENND11 and PRRC1) associated with deprivation, a latent factor constructed from variables such as socioeconomic status, family income, mother’s educational level, neglect, and father presence. Seven genes (SMAD4, SIN3A, CRLF3, USP38, FAR1, DENND11 and PRRC1) were selected based on our previous microarray analysis in the Sao Paulo subsample of the BHRCS (N=103 at baseline and N=103 at follow-up). The results from the current study align with our previous findings, with SMAD4, SIN3A, CRLF3, USP38, DENND11 (previously known as KIAA1147) and PRRC1 expression decreasing in individuals who transitioned from low to high psychopathology (as measured by the CBCL) (Supplementary Table S2 from (15)). Consistent with this, the first three were negatively associated with internalizing symptoms, USP38 with externalizing symptoms and DENND11 and PRRC1 were negatively correlated with deprivation in the present study (Table 2). FAR1, on the other hand, showed increased expression in individuals who transitioned from high to low psychopathology (Supplementary Table S3 from (15)), contrasting with the positive correlation between FAR1 expression and internalizing symptoms observed at time point 2 in the current study. Finally, both NR3C1 and HSPBP1 genes were selected because they were differentially expressed in the brains of individuals with mental disorders (27), with NR3C1 also being differentially expressed in the blood of BHRCS individuals with major depressive disorder (16). After adjusting for multiple comparisons, no significant mediation or moderation effects of gene expression on the association between life adversities and psychiatric symptoms were found for these genes.
Adolescence is a period that is particularly vulnerable to environmental influences, and traumatic experiences that occur before or during this stage can significantly impact the likelihood of developing externalizing or internalizing behaviors. Such experiences leave a lasting impact on various biological systems, affecting neuronal, endocrine, immune, transcriptomic, and epigenetic processes (35). Emerging research in genomics, epigenomics, and transcriptomics aims to understand why some individuals are more susceptible to developing certain mental health issues after experiencing trauma (36, 37). For instance, a transcriptome-wide study found that the expression of genes related to the immune system may mediate the link between emotional abuse and MDD (38). Additionally, studies in animal models have shown that patterns of gene expression in cortical and amygdala pyramidal neurons can predict whether fear extinction will be adaptive or maladaptive. which can help to understand trauma-related disorders (39). These findings highlight the complex interactions between biological and environmental factors in shaping mental health outcomes during adolescence.
Notably, traumatic events can disrupt the activity of the HPA axis, which is reflected in cortisol levels. This disruption can lead to changes in functional connectivity in the brain and is associated with internalizing problems later in life (40). Cortisol activates glucocorticoid receptors, which are expressed throughout the brain and can function as transcription factors, regulating genes related to metabolism and immune function (41). In our study, we found the NR3C1 gene (nuclear receptor subfamily 3, group C, member 1), which encodes the glucocorticoid receptor, to be associated with internalizing. Many studies have associated it with CM and depressive symptoms, particularly those investigating NR3C1 methylation (42, 43). One of the first studies to investigate this in humans observed decreased expression of NR3C1, along with increased methylation of its promoter, in the hippocampus of suicide victims with a history of childhood abuse (44). Changes in NR3C1 gene expression in blood have also been detected, with some studies identifying increased expression in patients with depression (45) and others finding a negative correlation with depressive scores (46). Our previous study in the BHRCS at time point 1 evaluated gene expression in 20 children and adolescents with major depressive disorder (MDD), 49 with no MDD but with high levels of depressive symptoms, and 61 healthy controls. This study identified decreased expression of NR3C1 in those with MDD compared to both the depressive symptom group and healthy controls (16), corroborating our current findings at time point 2.
The SIN3A gene (SIN3 transcription regulator family member A) encodes a transcriptional regulatory protein. It appears to play an important role in cell cycle events and the proliferation of embryonic stem cells, and it is highly expressed throughout brain development (47), consistent with our analysis in BrainSpan. Indeed, functional studies have revealed that SIN3A is a key regulator of cortical expansion and maturation, and its haploinsufficiency has been associated with intellectual disability and autism spectrum disorder (47). Apart from increased SIN3A protein levels in the hippocampus of suicide victims (48), no other association of this gene with mental disorders has been reported, particularly in blood.
The SMAD4 (SMAD family member 4) gene encodes a member of the SMAD family of signal transduction proteins. It is involved in signal transduction of the transforming growth factor beta (TGFβ) superfamily and pathogenic variants in this gene cause Myhre syndrome, which can include neurobehavioral phenotypes such as developmental delay, autism spectrum disorder, attention-deficit/hyperactivity disorder, and anxiety (49). Moreover, these mutations also disrupt neuronal morphogenesis in both mouse and human neurons (50). The TGFβ pathway has been associated with depression and stress, as it is an anti-inflammatory signal that exerts neuroprotective effects and influences memory formation and synaptic plasticity (51). Preliminary analysis showed that children with lower SMAD4 expression (predicted by genetic variants) exhibited a positive correlation between prenatal maternal depressive symptoms and amygdala volumes, while those with higher SMAD4 expression presented a negative correlation between prenatal maternal depression and amygdala volumes (52).
The FAR1 gene (fatty acyl-CoA reductase 1) encodes a rate-limiting enzyme in plasmalogen biosynthesis (53). Mutations in this gene have been identified in individuals affected by severe intellectual disability, early-onset epilepsy, microcephaly, congenital cataracts, growth retardation, and spasticity (54, 55). Although little is known about this gene, plasmalogens are abundant in the myelin sheath, and thus, plasmalogen homeostasis is likely important for myelination in different regions of the central nervous system (53). Indeed, impaired plasmalogen synthesis has already been implicated in schizophrenia (56–58). Interestingly, the role of FAR1 in psychopathology appears complex. In a previous study, we found that FAR1 expression was increased in individuals who transitioned from high to low psychopathology, as measured by the total CBCL score (15). However, in the current study, FAR1 expression was positively correlated with internalizing symptoms, suggesting a potential association with increased internalizing problems. This apparent discrepancy may be due to several factors, including differences in sample size and the distinct biological underpinnings of internalizing symptoms compared to the broader measure of psychopathology used previously. It highlights the need for further research to fully elucidate the role of FAR1 in different facets of psychopathology and across different developmental stages.
The CRLF3 gene (cytokine receptor-like factor 3) encodes a cytokine receptor-like factor. Studies in human and mouse cells have revealed that it is a critical regulator of neurogenesis, neuron survival, and dendritic development, and its deletion appears to cause developmental delay, intellectual disability, and autistic traits (59, 60). Although few studies have investigated this gene, its expression appears to be associated with Alzheimer’s disease (61) and epigenetic age acceleration (62).
The HSPBP1 gene (heat-shock 70-kd protein-binding protein 1) encodes a cochaperone of heat shock protein 70 (Hsp70) that inhibits the activity of Hsp70 ATPase. It is highly expressed in neurons but lowly expressed in astrocytes. Knocking down HspBP1 in neurons rescued neuropathology in a Huntington’s disease mouse model (63). Although no study investigated this particular gene in relation to mental disorders, alterations in Hsp70 have been reported in bipolar disorder (64) and MDD (65), and medications such as lithium and clozapine appear to alter Hsp70 levels (65).
The USP38 (Ubiquitin Specific Peptidase 38) gene encodes a deubiquitinating enzyme that acts on different cellular processes, such as the DNA repair, regulation of the cell cycle, and the immune response (66, 67). Recently, the variant rs7681616-C present in the USP38 gene was associated with an increase in the risk of developing schizophrenia (68), suggesting the importance of this region in psychopathology. Another study identified that USP38 blood expression was significantly downregulated in schizophrenia patients compared to biological siblings and unaffected controls (69), aligning with our findings of decreased USP38 expression in individuals with increased externalizing symptoms. Furthermore, a CpG site (cg01769344) located in a CpG island near the USP38 gene was identified within a co-methylated module associated with general psychopathology in a sample of 440 children from the Generation R cohort (18). Based on this evidence, we suggest that the USP38 gene may play a significant role in psychopathology.
While our knowledge of the DENND11 gene (formerly KIAA1147) remains limited, its protein product is predicted to participate in regulation of catalytic activity. By similarity, DENND11 is hypothesized to play a role in neuritogenesis (70), aligning with its elevated expression in multiple brain developmental stages and tissues (Supplementary Figures S7 C, D). Furthermore, abnormal DNA methylation levels and the presence of risk-associated single nucleotide polymorphisms (SNPs) have led to its consideration as a candidate gene for frontotemporal dementia with amyotrophic lateral sclerosis (71). Even less is known about the PRRC1 gene. It is predicted to be involved in the activation of protein kinase A activity and was previously associated with fluid intelligence in a genome-wide association study (GWAS) (72). Interestingly, the same study found that methylation of this gene in the temporal cortex was also associated with fluid intelligence.
We observed correlations among these nine genes (Supplementary Figure S7A); however, HSPBP1 did not appear to be correlated within the co-expression networks (Supplementary Figure S7B), which may be explained by its lower expression in blood (Supplementary Figure S6B, S7C). Of note, SIN3A, SMAD4, and NR3C1, despite being highly correlated (Supplementary Figure S7A), seem to exhibit similar expression patterns in multiple tissues (Supplementary Figure S7C) and are involved in two Reactome pathways: FOXO-mediated transcription of oxidative stress, metabolic, and neuronal genes, and FOXO-mediated transcription. None of the genes were associated with time point (Supplementary Table S5) or with psychopathology in longitudinal models. This suggests that these results might be time-specific, particularly for internalizing symptoms, considering their increase at time point 2, when the mean age was 13 years (Table 1).
The results of this study should be interpreted in light of certain limitations. First, the relatively small sample size may be an issue, warranting further replication studies. While few studies have investigated gene expression in longitudinal children and adolescent cohorts (73), most existing studies in this type of cohort (such as Generation R and Avon Longitudinal Study of Parents and Children) have focused on DNA variations, such as in GWAS (7–9), or DNA methylation (18). Second, this targeted RNA sequencing approach may have missed other genes relevant to psychopathology or be biased by cell type composition. Comprehensive transcriptome analysis could provide a more complete picture in future studies. Third, gene expression is tissue-specific, and our findings do not accurately reflect gene expression changes in the brain. Therefore, these results are more likely to be potential biomarkers of trait or state, and may not be clues about the pathophysiology. Furthermore, longitudinal gene expression analysis is not feasible in brain tissues. Finally, it is uncertain whether other factors that may influence gene expression, such as puberty or infection may have, affected our results, despite adjusting our analyses for sex, age, and time point.
In conclusion, our study has shed light on the complex interplay between genes, life adversities, and psychopathology in young individuals. We identified six genes associated with internalizing symptoms: NR3C1, HSPBP1, SIN3A, SMAD4, and CRLF3 exhibited a negative correlation with these symptoms, while FAR1 showed a positive correlation. Notably, NR3C1 has been consistently associated with internalizing symptoms and traumatic events, corroborating our findings. Additionally, decreased USP38 expression was associated with more externalizing problems, suggesting its possible role in regulating behavioral control or emotional reactivity. Furthermore, we observed lower expression of DENND11 and PRRC1 correlated with higher levels of deprivation, indicating a potential role in the biological response to environmental adversity. While the exact mechanisms remain unclear, these findings underscore the complex interplay between genetic predisposition and environmental stressors in shaping mental health outcomes. Although life adversities undeniably contribute to the development of psychiatric symptoms, our study did not find evidence for a direct mediating or moderating effect of gene expression on this relationship. This suggests that the influence of these genes on psychopathology may operate through independent pathways or interact with environmental factors in more nuanced ways. Importantly, our findings highlight the potential of specific gene expression patterns as early indicators of psychiatric vulnerability. The associations observed for NR3C1, HSPBP1, SIN3A, SMAD4, CRLF3, FAR1 and USP38 with internalizing and externalizing symptoms across multiple time points suggest their potential utility in predicting future mental health trajectories. Similarly, the association between DENND11 and PRRC1 expression and deprivation underscores the importance of considering both genetic and environmental factors in understanding and addressing mental health challenges.
Data availability statement
RNA sequencing data were deposited into the Gene Expression Omnibus database under accession number GSE288870.
Ethics statement
The studies involving humans were approved by Faculdade de Medicina da Universidade de São Paulo (IORG0004884), the Universidade Federal de São Paulo (CAAE: 74563817.7.2002.5505), and the Universidade Federal do Rio Grande do Sul (CAAE: 74563817.7.1001.5327). The studies were conducted in accordance with the local legislation and institutional requirements. Written informed consent for participation in this study was provided by the participants’ legal guardians/next of kin.
Author contributions
VO: Conceptualization, Data curation, Formal analysis, Funding acquisition, Investigation, Methodology, Resources, Writing – original draft, Writing – review & editing. AO: Formal analysis, Methodology, Writing – review & editing. AB: Methodology, Validation, Writing – review & editing. HC: Formal analysis, Investigation, Methodology, Writing – review & editing. PG: Methodology, Supervision, Writing – review & editing. PA: Investigation, Methodology, Writing – review & editing. JS: Investigation, Methodology, Writing – review & editing. MH: Conceptualization, Formal analysis, Investigation, Methodology, Supervision, Writing – review & editing. RB: Conceptualization, Funding acquisition, Supervision, Writing – review & editing. EB: Conceptualization, Investigation, Supervision, Writing – review & editing. GM: Conceptualization, Methodology, Writing – review & editing. RG-O: Conceptualization, Investigation, Methodology, Writing – review & editing. AG: Conceptualization, Investigation, Methodology, Writing – review & editing. LR: Conceptualization, Methodology, Supervision, Writing – review & editing. EM: Conceptualization, Funding acquisition, Project administration, Writing – review & editing. PP: Conceptualization, Investigation, Methodology, Project administration, Supervision, Writing – review & editing. MS: Conceptualization, Investigation, Methodology, Project administration, Writing – review & editing. GS: Conceptualization, Investigation, Methodology, Project administration, Supervision, Writing – review & editing. CC: Data curation, Methodology, Project administration, Supervision, Writing – original draft, Writing – review & editing. SB: Conceptualization, Funding acquisition, Investigation, Methodology, Supervision, Writing – original draft, Writing – review & editing.
Funding
The author(s) declare financial support was received for the research, authorship, and/or publication of this article. This study was supported by Fundação de Amparo à Pesquisa do Estado de São Paulo (FAPESP; grant numbers: 2017/05339-7 and 2025/00302-4), CAPES (Finance Code 001). The Brazilian High Risk Cohort for Mental Conditions (BHRCS) was funded by the National Institute of Development Psychiatric for Children and Adolescent (INPD), supported by CNPq-FAPESP (573974/2008-0; 465550/2014-2), INCT (FAPESP/CNPQ 2014/50917-0) and FAPESP (2008/57896-8, 2021/05332-8 and 2021/12901-9). PAFG was supported by Fundação de Amparo à Pesquisa do Estado de São Paulo (FAPESP 2018/15579–8; and partially supported by funds from CNPq and Hospital Sı́rio-Libanês. HBC was supported by Fundação de Amparo à Pesquisa do Estado de São Paulo (FAPESP 2018/13613–4 and 2024/00078-4) . CMC was supported by Fundação de Amparo à Pesquisa do Estado de São Paulo (FAPESP 2020/10599-0). MSH is supported by the United States National Institutes of Health grant R01MH120482 under his post-doctoral fellowship at UFRGS.
Acknowledgments
We would like to thank the participants and their families.
Conflict of interest
LR has received grant or research support from, served as a consultant to, and served on the speakers’ bureau of Abdi Ibrahim, Abbott, Aché, Adium, Apsen, Bial, Cellera, EMS, Hypera Pharma, Knight Therapeutics, Libbs, Medice, Novartis/Sandoz, Pfizer/Upjohn/Viatris, Shire/Takeda, and Torrent in the last three years. The ADHD and Juvenile Bipolar Disorder Outpatient Programs chaired by LR have received unrestricted educational and research support from the following pharmaceutical companies in the last three years: Novartis/Sandoz and Shire/Takeda. LR has received authorship royalties from Oxford Press and ArtMed. EB got research grants from Queen’s University and PSI Foundation unrelated to this manuscript. PP received payment or honoraria for lectures and presentations in educational events for Abbot, Libbs, Instituto Israelita de Pesquisa e Ensino Albert Einstein.
The remaining authors declare that the research was conducted in the absence of any commercial or financial relationships that could be construed as a potential conflict of interest.
Generative AI statement
The author(s) declare that Generative AI was used in the creation of this manuscript. Generative AI was used only to correct the English.
Publisher’s note
All claims expressed in this article are solely those of the authors and do not necessarily represent those of their affiliated organizations, or those of the publisher, the editors and the reviewers. Any product that may be evaluated in this article, or claim that may be made by its manufacturer, is not guaranteed or endorsed by the publisher.
Supplementary material
The Supplementary Material for this article can be found online at: https://www.frontiersin.org/articles/10.3389/fpsyt.2025.1505421/full#supplementary-material
References
1. Caspi A, Houts RM, Ambler A, Danese A, Elliott ML, Hariri A, et al. Longitudinal assessment of mental health disorders and comorbidities across 4 decades among participants in the dunedin birth cohort study. JAMA Netw Open. (2020) 3:e203221. doi: 10.1001/jamanetworkopen.2020.3221
2. Smoller JW, Andreassen OA, Edenberg HJ, Faraone SV, Glatt SJ, Kendler KS. Psychiatric genetics and the structure of psychopathology. Mol Psychiatry. (2019) 24:409–20. doi: 10.1038/s41380-017-0010-4
3. Martel MM, Pan PM, Hoffmann MS, Gadelha A, do Rosário MC, Mari JJ, et al. A general psychopathology factor (P factor) in children: Structural model analysis and external validation through familial risk and child global executive function. J Abnorm Psychol. (2017) 126:137–48. doi: 10.1037/abn0000205
4. Polderman TJC, Benyamin B, de Leeuw CA, Sullivan PF, van Bochoven A, Visscher PM, et al. Meta-analysis of the heritability of human traits based on fifty years of twin studies. Nat Genet. (2015) 47:702–9. doi: 10.1038/ng.3285
5. Cross-Disorder Group of the Psychiatric Genomics Consortium. Identification of risk loci with shared effects on five major psychiatric disorders: a genome-wide analysis. Lancet. (2013) 381:1371–9. doi: 10.1016/S0140-6736(12)62129-1
6. Cross-Disorder Group of the Psychiatric Genomics Consortium. Electronic address:cGxlZTBAbWdoLmhhcnZhcmQuZWR1LA== Cross-Disorder Group of the Psychiatric Genomics Consortium. Genomic Relationships, Novel Loci, and Pleiotropic Mechanisms across Eight Psychiatric Disorders. Cell. (2019) 179:1469–1482.e11. doi: 10.1016/j.cell.2019.11.020
7. Jami ES, Hammerschlag AR, Ip HF, Allegrini AG, Benyamin B, Border R, et al. Genome-wide association meta-analysis of childhood and adolescent internalizing symptoms. J Am Acad Child Adolesc Psychiatry. (2022) 61:934–45. doi: 10.1016/j.jaac.2021.11.035
8. Karlsson Linnér R, Mallard TT, Barr PB, Sanchez-Roige S, Madole JW, Driver MN, et al. Multivariate analysis of 1.5 million people identifies genetic associations with traits related to self-regulation and addiction. Nat Neurosci. (2021) 24:1367–76. doi: 10.1038/s41593-021-00908-3
9. Neumann A, Nolte IM, Pappa I, Ahluwalia TS, Pettersson E, Rodriguez A, et al. A genome-wide association study of total child psychiatric problems scores. PloS One. (2022) 17:e0273116. doi: 10.1371/journal.pone.0273116
10. Ziebold C, Evans-Lacko S, Andrade MCR, Hoffmann M, Fonseca L, Barbosa M, et al. Childhood poverty and mental health disorders in early adulthood: evidence from a Brazilian cohort study. Eur Child Adolesc Psychiatry. (2023) 32:903–14. doi: 10.1007/s00787-021-01923-2
11. Salum GA, DeSousa DA, Manfro GG, Pan PM, Gadelha A, Brietzke E, et al. Measuring child maltreatment using multi-informant survey data: a higher-order confirmatory factor analysis. Trends Psychiatry Psychother. (2016) 38:23–32. doi: 10.1590/2237-6089-2015-0036
12. Schäfer JL, McLaughlin KA, Manfro GG, Pan P, Rohde LA, Miguel EC, et al. Threat and deprivation are associated with distinct aspects of cognition, emotional processing, and psychopathology in children and adolescents. Dev Sci. (2023) 26:e13267. doi: 10.1111/desc.13267
13. Gusev A, Lee SH, Trynka G, Finucane H, Vilhjálmsson BJ, Xu H, et al. Partitioning heritability of regulatory and cell-type-specific variants across 11 common diseases. Am J Hum Genet. (2014) 95:535–52. doi: 10.1016/j.ajhg.2014.10.004
14. Lynall ME, Soskic B, Hayhurst J, Schwartzentruber J, Levey DF, Pathak GA, et al. Genetic variants associated with psychiatric disorders are enriched at epigenetically active sites in lymphoid cells. Nat Commun. (2022) 13:6102. doi: 10.1038/s41467-022-33885-7
15. Ota VK, Santoro ML, Spindola LM, Pan PM, Simabucuro A, Xavier G, et al. Gene expression changes associated with trajectories of psychopathology in a longitudinal cohort of children and adolescents. Transl Psychiatry. (2020) 10:99. doi: 10.1038/s41398-020-0772-3
16. Spindola LM, Pan PM, Moretti PN, Ota VK, Santoro ML, Cogo-Moreira H, et al. Gene expression in blood of children and adolescents: Mediation between childhood maltreatment and major depressive disorder. J Psychiatr Res. (2017) 92:24–30. doi: 10.1016/j.jpsychires.2017.03.015
17. Spindola LM, Santoro ML, Pan PM, Ota VK, Xavier G, Carvalho CM, et al. Detecting multiple differentially methylated CpG sites and regions related to dimensional psychopathology in youths. Clin Epigenet. (2019) 11:146. doi: 10.1186/s13148-019-0740-z
18. Rijlaarsdam J, Barker ED, Caserini C, Koopman-Verhoeff ME, Mulder RH, Felix JF, et al. Genome-wide DNA methylation patterns associated with general psychopathology in children. J Psychiatr Res. (2021) 140:214–20. doi: 10.1016/j.jpsychires.2021.05.029
19. Etzel L, Apsley AT, Hastings WJ, Ye Q, Shalev I. Early life adversity is associated with differential gene expression in immune cells: A cluster-based analysis across an acute psychosocial stressor. Brain Behav Immun. (2024) 119:724–33. doi: 10.1016/j.bbi.2024.04.035
20. Kuhlman KR, Cole SW, Craske MG, Fuligni AJ, Irwin MR, Bower JE. Enhanced immune activation following acute social stress among adolescents with early-life adversity. Biol Psychiatry Glob Open Sci. (2023) 3:213–21. doi: 10.1016/j.bpsgos.2022.03.001
21. Kumsta R. The role of stress in the biological embedding of experience. Psychoneuroendocrinology. (2023) 156:106364. doi: 10.1016/j.psyneuen.2023.106364
22. Maes M, Rachayon M, Jirakran K, Sodsai P, Klinchanhom S, Debnath M, et al. Adverse childhood experiences predict the phenome of affective disorders and these effects are mediated by staging, neuroimmunotoxic and growth factor profiles. Cells. (2022) 11:1564. doi: 10.3390/cells11091564
23. Oh EY, Park BS, Yang HR, Lee HG, Tu TH, Yang S, et al. Whole transcriptome analysis of hypothalamus in mice during short-term starvation. Int J Mol Sci. (2023) 24:3204. doi: 10.3390/ijms24043204
24. Bertrand A, Sugrue J, Lou T, Bourke NM, Quintana-Murci L, Saint-André V, et al. Impact of socioeconomic status on healthy immune responses in humans. Immunol Cell Biol. (2024) 102:618–29. doi: 10.1111/imcb.v102.7
25. Wang R, Su Y, O’Donnell K, Caron J, Meaney M, Meng X, et al. Differential interactions between gene expressions and stressors across the lifespan in major depressive disorder. J Affect Disord. (2024) 362:688–97. doi: 10.1016/j.jad.2024.07.069
26. Salum GA, Gadelha A, Pan PM, Moriyama TS, Graeff-Martins AS, Tamanaha AC, et al. High risk cohort study for psychiatric disorders in childhood: rationale, design, methods and preliminary results: High Risk Cohort Study. Int J Methods Psychiatr Res. (2015) 24:58–73. doi: 10.1002/mpr.v24.1
27. Gandal MJ, Haney JR, Parikshak NN, Leppa V, Ramaswami G, Hartl C, et al. Shared molecular neuropathology across major psychiatric disorders parallels polygenic overlap. Science. (2018) 359:693–7. doi: 10.1126/science.aad6469
28. Stamova BS, Apperson M, Walker WL, Tian Y, Xu H, Adamczy P, et al. Identification and validation of suitable endogenous reference genes for gene expression studies in human peripheral blood. BMC Med Genomics. (2009) 2:49. doi: 10.1186/1755-8794-2-49
29. Frankish A, Carbonell-Sala S, Diekhans M, Jungreis I, Loveland JE, Mudge JM, et al. GENCODE: reference annotation for the human and mouse genomes in 2023. Nucleic Acids Res. (2023) 51:D942–9. doi: 10.1093/nar/gkac1071
30. Quinlan AR, Hall IM. BEDTools: a flexible suite of utilities for comparing genomic features. Bioinformatics. (2010) 26:841–2. doi: 10.1093/bioinformatics/btq033
31. Bray NL, Pimentel H, Melsted P, Pachter L. Near-optimal probabilistic RNA-seq quantification. Nat Biotechnol. (2016) 34:525–7. doi: 10.1038/nbt.3519
32. Soneson C, Love MI, Robinson MD. Differential analyses for RNA-seq: transcript-level estimates improve gene-level inferences. F1000Res. (2015) 4:1521. doi: 10.12688/f1000research
33. Warde-Farley D, Donaldson SL, Comes O, Zuberi K, Badrawi R, Chao P, et al. The GeneMANIA prediction server: biological network integration for gene prioritization and predicting gene function. Nucleic Acids Res. (2010) 38:W214–20. doi: 10.1093/nar/gkq537
34. Watanabe K, Taskesen E, van Bochoven A, Posthuma D. Functional mapping and annotation of genetic associations with FUMA. Nat Commun. (2017) 8:1826. doi: 10.1038/s41467-017-01261-5
35. Laricchiuta D, Panuccio A, Picerni E, Biondo D, Genovesi B, Petrosini L. The body keeps the score: The neurobiological profile of traumatized adolescents. Neurosci Biobehav Rev. (2023) 145:105033. doi: 10.1016/j.neubiorev.2023.105033
36. Layfield SD, Duffy LA, Phillips KA, Lardenoije R, Klengel T, Ressler KJ. Multiomic biological approaches to the study of child abuse and neglect. Pharmacol Biochem Behav. (2021) 210:173271. doi: 10.1016/j.pbb.2021.173271
37. Gladish N, Merrill SM, Kobor MS. Childhood trauma and epigenetics: state of the science and future. Curr Environ Health Rep. (2022) 9:661–72. doi: 10.1007/s40572-022-00381-5
38. Minelli A, Magri C, Giacopuzzi E, Gennarelli M. The effect of childhood trauma on blood transcriptome expression in major depressive disorder. J Psychiatr Res. (2018) 104:50–4. doi: 10.1016/j.jpsychires.2018.06.014
39. Laricchiuta D, Gimenez J, Sciamanna G, Termine A, Fabrizio C, Della Valle F, et al. Synaptic and transcriptomic features of cortical and amygdala pyramidal neurons predict inefficient fear extinction. Cell Rep. (2023) 42:113066. doi: 10.1016/j.celrep.2023.113066
40. Burghy CA, Stodola DE, Ruttle PL, Molloy EK, Armstrong JM, Oler JA, et al. Developmental pathways to amygdala-prefrontal function and internalizing symptoms in adolescence. Nat Neurosci. (2012) 15:1736–41. doi: 10.1038/nn.3257
41. Lupien SJ, McEwen BS, Gunnar MR, Heim C. Effects of stress throughout the lifespan on the brain, behaviour and cognition. Nat Rev Neurosci. (2009) 10:434–45. doi: 10.1038/nrn2639
42. Nie Y, Wen L, Song J, Wang N, Huang L, Gao L, et al. Emerging trends in epigenetic and childhood trauma: Bibliometrics and visual analysis. Front Psychiatry. (2022) 13:925273. doi: 10.3389/fpsyt.2022.925273
43. Watkeys OJ, Kremerskothen K, Quidé Y, Fullerton JM, Green MJ. Glucocorticoid receptor gene (NR3C1) DNA methylation in association with trauma, psychopathology, transcript expression, or genotypic variation: A systematic review. Neurosci Biobehav Rev. (2018) 95:85–122. doi: 10.1016/j.neubiorev.2018.08.017
44. McGowan PO, Sasaki A, D’Alessio AC, Dymov S, Labonté B, Szyf M, et al. Epigenetic regulation of the glucocorticoid receptor in human brain associates with childhood abuse. Nat Neurosci. (2009) 12:342–8. doi: 10.1038/nn.2270
45. Hori H, Yoshida F, Ishida I, Matsuo J, Ogawa S, Hattori K, et al. Blood mRNA expression levels of glucocorticoid receptors and FKBP5 are associated with depressive disorder and altered HPA axis. J Affect Disord. (2024) 349:244–53. doi: 10.1016/j.jad.2024.01.080
46. Schurgers G, Walter S, Pishva E, Guloksuz S, Peerbooms O, Incio LR, et al. Longitudinal alterations in mRNA expression of the BDNF neurotrophin signaling cascade in blood correlate with changes in depression scores in patients undergoing electroconvulsive therapy. Eur Neuropsychopharmacol. (2022) 63:60–70. doi: 10.1016/j.euroneuro.2022.07.183
47. Witteveen JS, Willemsen MH, Dombroski TCD, van Bakel NHM, Nillesen WM, van Hulten JA, et al. Haploinsufficiency of MeCP2-interacting transcriptional co-repressor SIN3A causes mild intellectual disability by affecting the development of cortical integrity. Nat Genet. (2016) 48:877–87. doi: 10.1038/ng.3619
48. Misztak P, Pańczyszyn-Trzewik P, Nowak G, Sowa-Kućma M. Epigenetic marks and their relationship with BDNF in the brain of suicide victims. PloS One. (2020) 15:e0239335. doi: 10.1371/journal.pone.0239335
49. Lin AE, Scimone ER, Thom RP, Balaguru D, Kinane TB, Moschovis PP, et al. Emergence of the natural history of Myhre syndrome: 47 patients evaluated in the Massachusetts General Hospital Myhre Syndrome Clinic (2016-2023). Am J Med Genet A. (2024) 194:e63638. doi: 10.1002/ajmg.a.v194.10
50. Nakashima H, Tsujimura K, Irie K, Ishizu M, Pan M, Kameda T, et al. Canonical TGF-β Signaling negatively regulates neuronal morphogenesis through TGIF/smad complex-mediated CRMP2 suppression. J Neurosci. (2018) 38:4791–810. doi: 10.1523/JNEUROSCI.2423-17.2018
51. Hiew LF, Poon CH, You HZ, Lim LW. TGF-β/smad signalling in neurogenesis: implications for neuropsychiatric diseases. Cells. (2021) 10:1382. doi: 10.3390/cells10061382
52. Qiu A, Zhang H, Wang C, Chong YS, Shek LP, Gluckman PD, et al. Canonical TGF-β signaling regulates the relationship between prenatal maternal depression and amygdala development in early life. Transl Psychiatry. (2021) 11:170. doi: 10.1038/s41398-021-01292-z
53. Honsho M, Fujiki Y. Regulation of plasmalogen biosynthesis in mammalian cells and tissues. Brain Res Bull. (2023) 194:118–23. doi: 10.1016/j.brainresbull.2023.01.011
54. Buchert R, Tawamie H, Smith C, Uebe S, Innes AM, Al Hallak B, et al. A peroxisomal disorder of severe intellectual disability, epilepsy, and cataracts due to fatty acyl-CoA reductase 1 deficiency. Am J Hum Genet. (2014) 95:602–10. doi: 10.1016/j.ajhg.2014.10.003
55. Ferdinandusse S, McWalter K, Te Brinke H, IJlst L, Mooijer PM, Ruiter JPN, et al. An autosomal dominant neurological disorder caused by de novo variants in FAR1 resulting in uncontrolled synthesis of ether lipids. Genet Med. (2021) 23:740–50. doi: 10.1038/s41436-020-01027-3
56. Wood PL, Unfried G, Whitehead W, Phillipps A, Wood JA. Dysfunctional plasmalogen dynamics in the plasma and platelets of patients with schizophrenia. Schizophr Res. (2015) 161:506–10. doi: 10.1016/j.schres.2014.11.032
57. Kaddurah-Daouk R, McEvoy J, Baillie R, Zhu H, K Yao J, Nimgaonkar VL, et al. Impaired plasmalogens in patients with schizophrenia. Psychiatry Res. (2012) 198:347–52. doi: 10.1016/j.psychres.2012.02.019
58. Tessier C, Sweers K, Frajerman A, Bergaoui H, Ferreri F, Delva C, et al. Membrane lipidomics in schizophrenia patients: a correlational study with clinical and cognitive manifestations. Transl Psychiatry. (2016) 6:e906. doi: 10.1038/tp.2016.142
59. Wegscheid ML, Anastasaki C, Hartigan KA, Cobb OM, Papke JB, Traber JN, et al. Patient-derived iPSC-cerebral organoid modeling of the 17q11.2 microdeletion syndrome establishes CRLF3 as a critical regulator of neurogenesis. Cell Rep. (2021) 36:109315. doi: 10.1016/j.celrep.2021.109315
60. Wilson AF, Barakat R, Mu R, Karush LL, Gao Y, Hartigan KA, et al. A common single nucleotide variant in the cytokine receptor-like factor-3 (CRLF3) gene causes neuronal deficits in human and mouse cells. Hum Mol Genet. (2023) 32:3342–52. doi: 10.1093/hmg/ddad155
61. Sharma A, Dey P. A machine learning approach to unmask novel gene signatures and prediction of Alzheimer’s disease within different brain regions. Genomics. (2021) 113:1778–89. doi: 10.1016/j.ygeno.2021.04.028
62. Lu AT, Hannon E, Levine ME, Crimmins EM, Lunnon K, Mill J, et al. Genetic architecture of epigenetic and neuronal ageing rates in human brain regions. Nat Commun. (2017) 8:15353. doi: 10.1038/ncomms15353
63. Zhao T, Hong Y, Yin P, Li S, Li XJ. Differential HspBP1 expression accounts for the greater vulnerability of neurons than astrocytes to misfolded proteins. Proc Natl Acad Sci U S A. (2017) 114:E7803–11. doi: 10.1073/pnas.1710549114
64. Bei ES, Salpeas V, Alevizos B, Anagnostara C, Pappa D, Moutsatsou P. Pattern of heat shock factor and heat shock protein expression in lymphocytes of bipolar patients: increased HSP70-glucocorticoid receptor heterocomplex. J Psychiatr Res. (2013) 47:1725–36. doi: 10.1016/j.jpsychires.2013.07.018
65. Valiati FE, Feiten JG, Géa LP, Silveira Júnior É de M, Scotton E, Caldieraro MA, et al. Inflammation and damage-associated molecular patterns in major psychiatric disorders. Trends Psychiatry Psychother. (2023) 45:e20220576. doi: 10.47626/2237-6089-2022-0576
66. Xu Z, Hu H, Fang D, Wang J, Zhao K. The deubiquitinase USP38 promotes cell proliferation through stabilizing c-Myc. Int J Biochem Cell Biol. (2021) 137:106023. doi: 10.1016/j.biocel.2021.106023
67. Yang Y, Yang C, Li T, Yu S, Gan T, Hu J, et al. The deubiquitinase USP38 promotes NHEJ repair through regulation of HDAC1 activity and regulates cancer cell response to genotoxic insults. Cancer Res. (2020) 80:719–31. doi: 10.1158/0008-5472.CAN-19-2149
68. Trubetskoy V, Pardiñas AF, Qi T, Panagiotaropoulou G, Awasthi S, Bigdeli TB, et al. Mapping genomic loci implicates genes and synaptic biology in schizophrenia. Nature. (2022) 604:502–8. doi: 10.1038/s41586-022-04434-5
69. Glatt SJ, Stone WS, Nossova N, Liew CC, Seidman LJ, Tsuang MT. Similarities and differences in peripheral blood gene-expression signatures of individuals with schizophrenia and their first-degree biological relatives. Am J Med Genet B Neuropsychiatr Genet. (2011) 156B:869–87. doi: 10.1002/ajmg.b.v156.8
70. Szklarczyk D, Franceschini A, Wyder S, Forslund K, Heller D, Huerta-Cepas J, et al. STRING v10: protein-protein interaction networks, integrated over the tree of life. Nucleic Acids Res. (2015) 43:D447–452. doi: 10.1093/nar/gku1003
71. Taskesen E, Mishra A, van der Sluis S, Ferrari R, International FTD-Genomics Consortium, Veldink JH, et al. Susceptible genes and disease mechanisms identified in frontotemporal dementia and frontotemporal dementia with Amyotrophic Lateral Sclerosis by DNA-methylation and GWAS. Sci Rep. (2017) 7:8899. doi: 10.1038/s41598-017-09320-z
Keywords: psychopathology, transcriptome, internalizing symptoms, externalizing symptoms, genetics, trauma
Citation: Ota VK, Oliveira AM, Bugiga AVG, Conceição HB, Galante PAF, Asprino PF, Schäfer JL, Hoffmann MS, Bressan R, Brietzke E, Manfro GG, Grassi-Oliveira R, Gadelha A, Rohde LA, Miguel EC, Pan PM, Santoro ML, Salum GA, Carvalho CM and Belangero SI (2025) Impact of life adversity and gene expression on psychiatric symptoms in children and adolescents: findings from the Brazilian high risk cohort study. Front. Psychiatry 16:1505421. doi: 10.3389/fpsyt.2025.1505421
Received: 02 October 2024; Accepted: 13 January 2025;
Published: 13 February 2025.
Edited by:
Rodrigo G. Arzate-Mejía, Medical Faculty of the University of Zurich, SwitzerlandReviewed by:
Daniela Laricchiuta, University of Perugia, ItalyGabriela Xavier, Massachusetts General Hospital, and Harvard Medical School, United States
Copyright © 2025 Ota, Oliveira, Bugiga, Conceição, Galante, Asprino, Schäfer, Hoffmann, Bressan, Brietzke, Manfro, Grassi-Oliveira, Gadelha, Rohde, Miguel, Pan, Santoro, Salum, Carvalho and Belangero. This is an open-access article distributed under the terms of the Creative Commons Attribution License (CC BY). The use, distribution or reproduction in other forums is permitted, provided the original author(s) and the copyright owner(s) are credited and that the original publication in this journal is cited, in accordance with accepted academic practice. No use, distribution or reproduction is permitted which does not comply with these terms.
*Correspondence: Sintia Iole Belangero, c2luYmVsYW5nZXJvQGdtYWlsLmNvbQ==