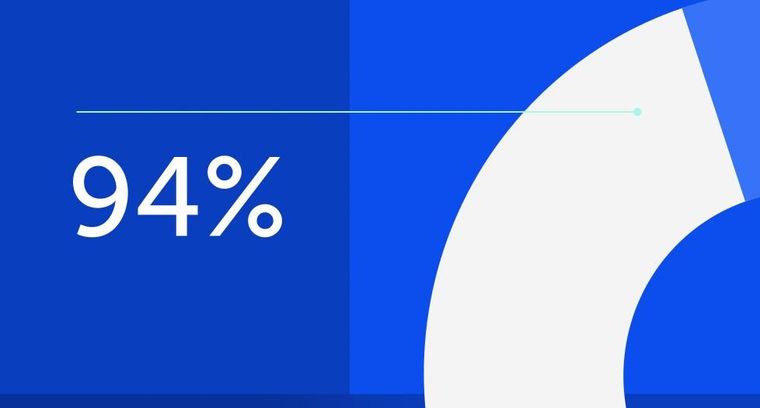
94% of researchers rate our articles as excellent or good
Learn more about the work of our research integrity team to safeguard the quality of each article we publish.
Find out more
ORIGINAL RESEARCH article
Front. Psychiatry
Sec. Digital Mental Health
Volume 16 - 2025 | doi: 10.3389/fpsyt.2025.1504306
This article is part of the Research TopicApplication of chatbot Natural Language Processing models to psychotherapy and behavioral mood healthView all 6 articles
The final, formatted version of the article will be published soon.
Select one of your emails
You have multiple emails registered with Frontiers:
Notify me on publication
Please enter your email address:
If you already have an account, please login
You don't have a Frontiers account ? You can register here
Purpose: In the context of psychotherapy, emotions play an important role both through their association with symptom severity, as well as their effects on the therapeutic relationship. In this analysis, we aim to train a large language model (LLM) for the detection of emotions in German speech. We want to apply this model on a corpus of psychotherapy transcripts to predict symptom severity and alliance aiming to identify the most important emotions for the prediction of symptom severity and therapeutic alliance.We employed a public labeled dataset of 28 emotions (Demszky et al., 2020) and translated the dataset into German. A pre-trained LLM was then fine-tuned on this dataset for emotion classification. We applied the fine-tuned model to a dataset containing 553 psychotherapy sessions of 124 patients. Using machine learning (ML) and explainable artificial intelligence (AI), we predicted symptom severity and alliance by the detected emotions.Our fine-tuned model achieved modest classification performance (F1macro =0.45, Accuracy=0.41, Kappa=0.42) across the 28 emotions. Incorporating all emotions, our ML model showed satisfying performance for the prediction of symptom severity (𝑟 = .50; 95%-CI:.42, .57) and moderate performance for the prediction of alliance scores (𝑟 = .20; 95%-CI:.06, .32). The most important emotions for the prediction of symptom severity were approval, anger, and fear. The most important emotions for the prediction of alliance were curiosity, confusion, and surprise.Conclusions: Even though the classification results were only moderate, our model achieved a good performance especially for prediction of symptom severity. The results confirm the role of negative emotions in the prediction of symptom severity, while they also highlight the role of positive emotions in fostering a good alliance. Future directions entail the improvement of the labeled dataset, especially with regards to domain-specificity and incorporating context information. Additionally, other modalities and Natural Language Processsing (NLP)-based alliance assessment could be integrated.
Keywords: Natural Language Processing, Computational Psychotherapy Research, machine learning, Explainable artificial intelligence, symptom severity, Alliance, Process-Outcome-Research
Received: 30 Sep 2024; Accepted: 14 Apr 2025.
Copyright: © 2025 Lalk, Targan, Steinbrenner, Schaffrath, Eberhardt, Schwartz, Vehlen, Lutz and Rubel. This is an open-access article distributed under the terms of the Creative Commons Attribution License (CC BY). The use, distribution or reproduction in other forums is permitted, provided the original author(s) or licensor are credited and that the original publication in this journal is cited, in accordance with accepted academic practice. No use, distribution or reproduction is permitted which does not comply with these terms.
* Correspondence: Christopher Lalk, Osnabrück University, Osnabrück, Germany
Disclaimer: All claims expressed in this article are solely those of the authors and do not necessarily represent those of their affiliated organizations, or those of the publisher, the editors and the reviewers. Any product that may be evaluated in this article or claim that may be made by its manufacturer is not guaranteed or endorsed by the publisher.
Supplementary Material
Research integrity at Frontiers
Learn more about the work of our research integrity team to safeguard the quality of each article we publish.