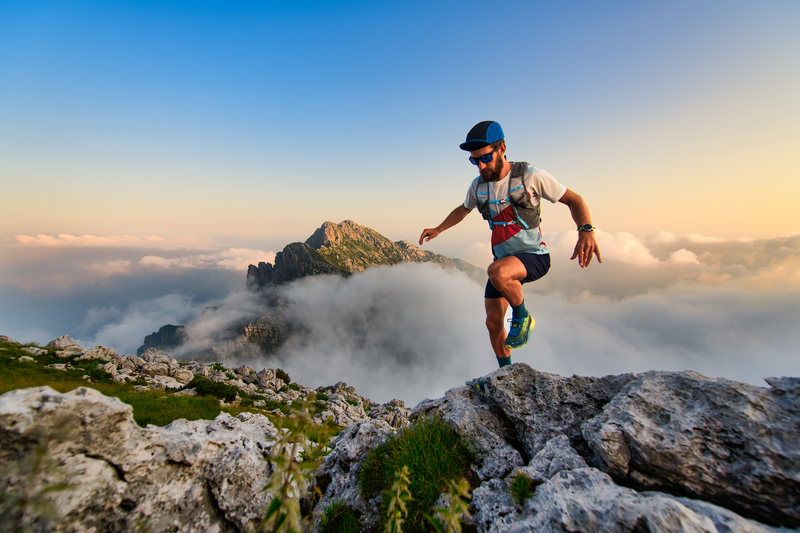
94% of researchers rate our articles as excellent or good
Learn more about the work of our research integrity team to safeguard the quality of each article we publish.
Find out more
ORIGINAL RESEARCH article
Front. Psychiatry
Sec. Public Mental Health
Volume 15 - 2024 | doi: 10.3389/fpsyt.2024.1521051
This article is part of the Research Topic The Intersection of Psychology, Healthy Behaviors, and its Outcomes View all 67 articles
The final, formatted version of the article will be published soon.
You have multiple emails registered with Frontiers:
Please enter your email address:
If you already have an account, please login
You don't have a Frontiers account ? You can register here
Objective To explore the risk factors affecting adolescents’ Internet addiction behavior and build a prediction model for adolescents’ Internet addiction behavior based on machine learning algorithms. Methods A total of 4461 high school students in Chongqing were selected using stratified cluster sampling, and questionnaires were administered. Based on the presence of Internet addiction behavior, students were categorized into an Internet addiction group (n=1210) and a non-Internet addiction group (n=3115). Gender, age, residence type, and other data were compared between the groups, and independent risk factors for adolescent Internet addiction were analyzed using a logistic regression model. Six methods—multi-level perceptron, random forest, K-nearest neighbor, support vector machine, logistic regression, and extreme gradient boosting—were used to construct the model. The model’s indicators under each algorithm were compared, evaluated with a confusion matrix, and the optimal model was selected. Result: The proportion of male adolescents, urban household registration, and scores on the family function, planning, action, and cognitive subscales, along with psychoticism, introversion-extroversion, neuroticism, somatization, obsessive-compulsiveness, interpersonal sensitivity, depression, anxiety, hostility, paranoia, and psychosis, were significantly higher in the Internet addiction group than in the non-Internet addiction group (P < 0.05). No significant differences were found in age or only-child status (P > 0.05). Statistically significant variables were analyzed using a logistic regression model, revealing that gender, household registration type, and scores on planning, action, introversion-extroversion, psychoticism, neuroticism, cognitive, obsessive-compulsive, depression, and hostility scales are independent risk factors for adolescent Internet addiction. The area under the curve (AUC) for multi-level perceptron, random forest, K-nearest neighbor, support vector machine, logistic regression, and extreme gradient boosting models were 0.843, 0.817, 0.778, 0.846, 0.847, and 0.836, respectively, with extreme gradient boosting showing the best predictive performance among these models. Conclusion The detection rate of Internet addiction is higher in males than in females, and adolescents with impulsive, extroverted, psychotic, neurotic, obsessive, depressive, and hostile traits are more prone to developing Internet addiction. While the overall performance of the machine learning models for predicting adolescent Internet addiction is moderate, the extreme gradient boosting method outperforms others, effectively identifying risk factors and enabling targeted interventions.
Keywords: machine learning, Adolescent, Internet behavior addiction, Risk factors, Prediction model
Received: 01 Nov 2024; Accepted: 30 Dec 2024.
Copyright: © 2024 Gan, Kuang, Xu, Ai, He, Wang, Hong, Chen, Cao and Zhang. This is an open-access article distributed under the terms of the Creative Commons Attribution License (CC BY). The use, distribution or reproduction in other forums is permitted, provided the original author(s) or licensor are credited and that the original publication in this journal is cited, in accordance with accepted academic practice. No use, distribution or reproduction is permitted which does not comply with these terms.
* Correspondence:
Li Kuang, First Affiliated Hospital of Chongqing Medical University, Chongqing, China
Disclaimer: All claims expressed in this article are solely those of the authors and do not necessarily represent those of their affiliated organizations, or those of the publisher, the editors and the reviewers. Any product that may be evaluated in this article or claim that may be made by its manufacturer is not guaranteed or endorsed by the publisher.
Research integrity at Frontiers
Learn more about the work of our research integrity team to safeguard the quality of each article we publish.