- 1Department of Psychiatry, The First Affiliated Hospital of Chongqing Medical University, Chongqing, China
- 2Mental Health Center, University-Town Hospital of Chongqing Medical University, Chongqing, China
Objective: To explore the risk factors that affect adolescents’ suicidal and self-injurious behaviors and to construct a prediction model for adolescents’ suicidal and self-injurious behaviors based on machine learning algorithms.
Methods: Stratified cluster sampling was used to select high school students in Chongqing, yielding 3,000 valid questionnaires. Based on whether students had engaged in suicide or self-injury, they were categorized into a suicide/self-injury group (n=78) and a non-suicide/self-injury group (n=2,922). Gender, age, insomnia, and mental illness data were compared between the two groups, and a logistic regression model was used to analyze independent risk factors for adolescent suicidal and self-injurious behavior. Six methods—multi-level perceptron, random forest, K-nearest neighbor, support vector machine, logistic regression, and extreme gradient boosting—were used to build predictive models. Various model indicators for suicidal and self-injurious behavior were compared across the six algorithms using a confusion matrix to identify the optimal model.
Result: In the self-injury and suicide groups, the proportions of male adolescents, late adolescence, insomnia, and mental illness were significantly higher than in the non-suicide and self-injury groups (p <0.05). Compared with the non-suicidal self-injury group, this group also showed significantly increased scores in cognitive subscales, impulsivity, psychoticism, introversion–extroversion, neuroticism, interpersonal sensitivity, depression, anxiety, hostility, terror, and paranoia (p <0.05). These statistically significant variables were analyzed in a logistic regression model, revealing that gender, impulsivity, psychoticism, neuroticism, interpersonal sensitivity, depression, and paranoia are independent risk factors for adolescent suicide and self-injury. The logistic regression model achieved the highest sensitivity and specificity in predicting adolescent suicide and self-injury behavior (0.9948 and 0.9981, respectively). Performance of the random forest, multi-level perceptron, and extreme gradient models was acceptable, while the K-nearest neighbor algorithm and support vector machine performed poorly.
Conclusion: The detection rate of suicidal and self-injurious behaviors is higher in women than in men. Adolescents displaying impulsiveness, psychoticism, neuroticism, interpersonal sensitivity, depression, and paranoia have a greater likelihood of engaging in such behaviors. The machine learning model for classifying and predicting adolescent suicide and self-injury risk effectively identifies these behaviors, enabling targeted interventions.
1 Introduction
Suicide and self-injury are one of the risk behaviors of adolescent health. That is, suicidal behavior disorder and non-suicidal self-injurious behavior (NSSI) can cause direct or indirect damage. They are relatively complex behavioral problems and seriously affect the mental health and safety of adolescents. At the same time, they can also lead to the loss of social resources and human costs (1, 2). Suicidal and self-injurious behaviors usually co-exist. In theory, it is easier to distinguish whether an individual who self-injures has suicidal intentions. However, in practice, it is not easy to clinically determine whether an individual has a suicidal intention when performing an injurious behavior (3, 4). Some NSSI people have suicidal intentions, especially those with poor subjective pain perception, who are more likely to have suicidal thoughts when they engage in self-injurious behavior. In addition, the data and information obtained through retrospective methods are not completely accurate, and some individuals many times deny the suicidal intention they once admitted, which may be related to shame (5–8). In recent years, the incidence of suicide and self-injury has been significantly increasing, which has sounded the alarm for us (9). Therefore, it is particularly important to explore effective methods for early identification of suicidal and self-injurious behaviors among adolescents and to provide timely intervention. Machine learning can assist in diagnosis, disease classification prediction, medical image recognition, etc. It is an important tool that can be used to assess and predict specific content in the real world (10–13). However, there are currently few studies on the application of machine learning algorithms in predicting suicidal and self-injurious behavior among adolescents. Based on the above background, this study analyzes the risk factors of adolescent suicidal and self-injurious behavior by constructing a machine learning model of suicidal and self-injurious behavior, with a view of providing prediction of suicidal and self-injurious behaviors, which offers additional possibilities.
2 Objects and methods
2.1 Research object
Stratified cluster sampling was used to select high school students in Chongqing as the research subjects. A total of 3,094 students were surveyed. After excluding questionnaires with obvious logical errors, a total of 3,000 questionnaires were recovered, with an effective response rate of 96.96%. This study was approved by the Ethics Committee of Chongqing Medical University. All research subjects were aware of the purpose and content of this study, and parents of minors were informed and signed informed consent forms.
2.2 Method
2.2.1 Grouping
With the “Suicide Attitude and Mental Health Status Questionnaire (University Edition IV)” survey questionnaire, “Have you ever had self-injury or suicidal behavior such as drinking medication or cutting your wrist?,” the teenagers who answered “Yes” will be regarded as the self-injury suicide group, while those who answered “No” will be regarded as the non-suicide self-injury group.
2.2.2 Data collection
The collection and comparative analysis of the information on gender, age, presence or absence of insomnia, and presence or absence of mental illness among adolescents were made in the suicide and self-injury groups and the non-suicide and self-injury groups.
2.2.3 Model construction
Construct a classification prediction model for suicidal and self-injurious behavior based on the general information of high school students in Chongqing. The first step is to determine whether the target behavior is suicidal and self-injurious behavior, and set a two-category label, where 0=none and 1=yes. Integrate all adolescent information and construct a classification prediction model for suicidal and self-injurious behavior. Model construction is carried out with six methods, namely, multi-level perceptron, random forest, K-neighbor algorithm, support vector machine, logistic regression, and extreme gradient boosting method; comparison was made of the multi-level perceptron, random forest, K-neighbor algorithm, support vector machine, and logistic regression and various indicators of the suicide and self-injury behavior model under the six extreme gradient boosting algorithms, and the optimal model was further selected.
2.2.4 The evaluation index
The confusion matrix was used to evaluate the model construction results of six methods: multi-level perceptron, random forest, K-neighbor algorithm, support vector machine, logistic regression, and extreme gradient boosting method.
2.3 Statistical method
The research data were analyzed by SPSS 21.0, the counting data were expressed by [n(%)], and the x2 test was used for pairwise comparison. The measurement data that conform to the normal distribution and homogeneity of variance are expressed by (`x ± s), and the pairwise comparison passes the independent sample t-test; logistic regression model was used to analyze the independent risk factors affecting adolescents’ suicide and self-injury. The results of model construction are evaluated by the confusion matrix. p < 0.05 was considered as a significant difference.
3 Results
3.1 Single factor analysis of the relationship between adolescents and suicidal and self-injurious behaviors
The proportion of male adolescent, late adolescence, insomnia, and mental illness in the self-injury and suicide groups was significantly higher than that in the non-suicide and self-injury group (p <0.05); the cognitive subscales, impulsivity scale scores, psychoticism of adolescents in the self-injury and suicide group, introversion–extroversion, neuroticism, interpersonal sensitivity, depression, anxiety, hostility, terror, and paranoia scores were significantly increased compared with the non-suicidal self-injury group (p <0.05). See Table 1.
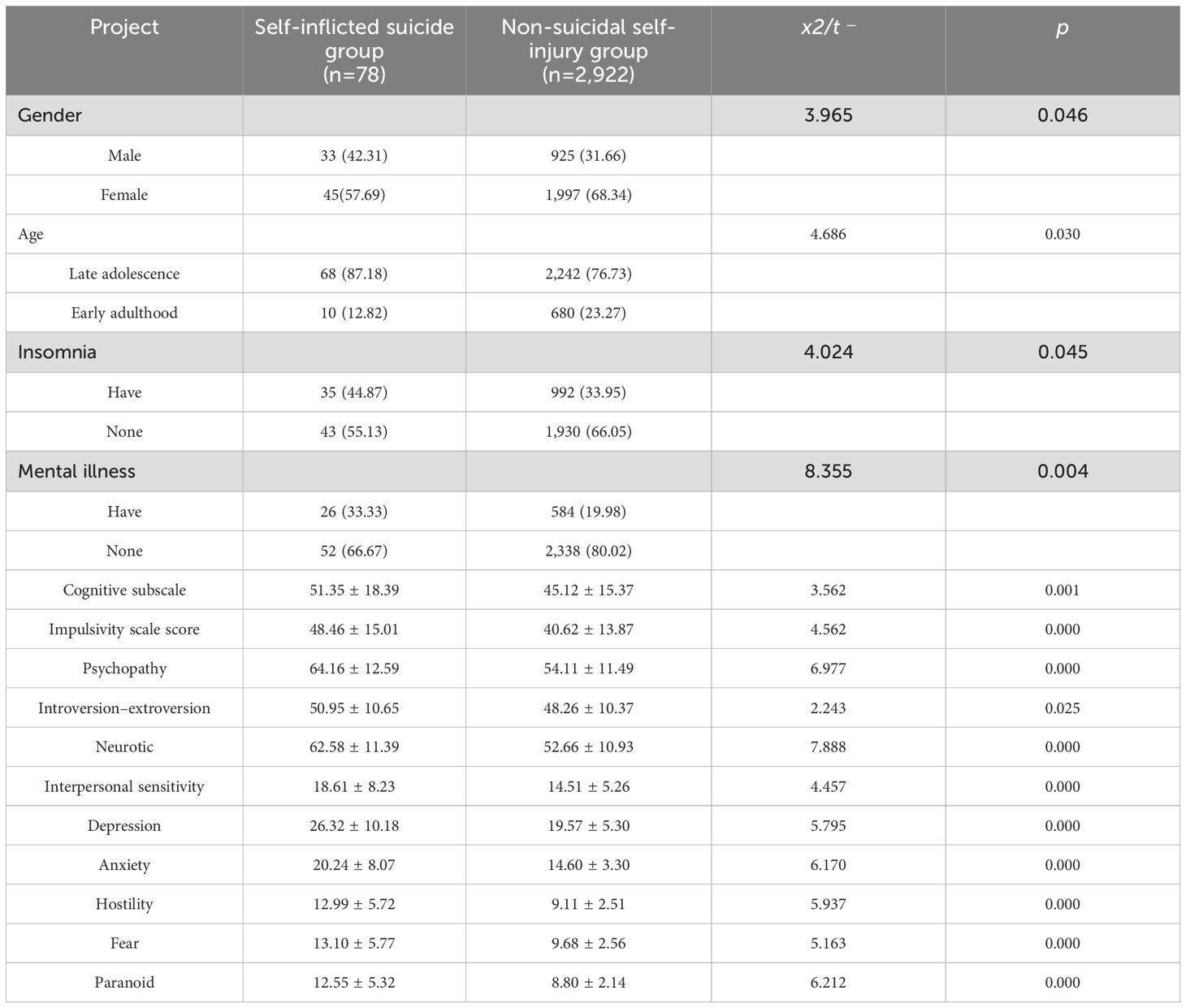
Table 1. Univariate analysis of adolescents’ relationship with suicidal and self-injurious behaviors [n (%), (`x ± s)].
3.2 Logistic multi-factor analysis on the relationship between adolescents and suicidal and self-injurious behaviors
Gender, age, insomnia, mental illness, cognitive subscale, impulsivity scale score, psychoticism, introversion–extroversion, and neuroticism scores were included in the logistic regression model for analysis. The results showed that gender, impulsivity scale score, psychoticism, neuroticism, sensitivity to interpersonal relationships, depression, and paranoia are all independent risk factors affecting adolescent suicide and self-injury behavior. See Table 2.
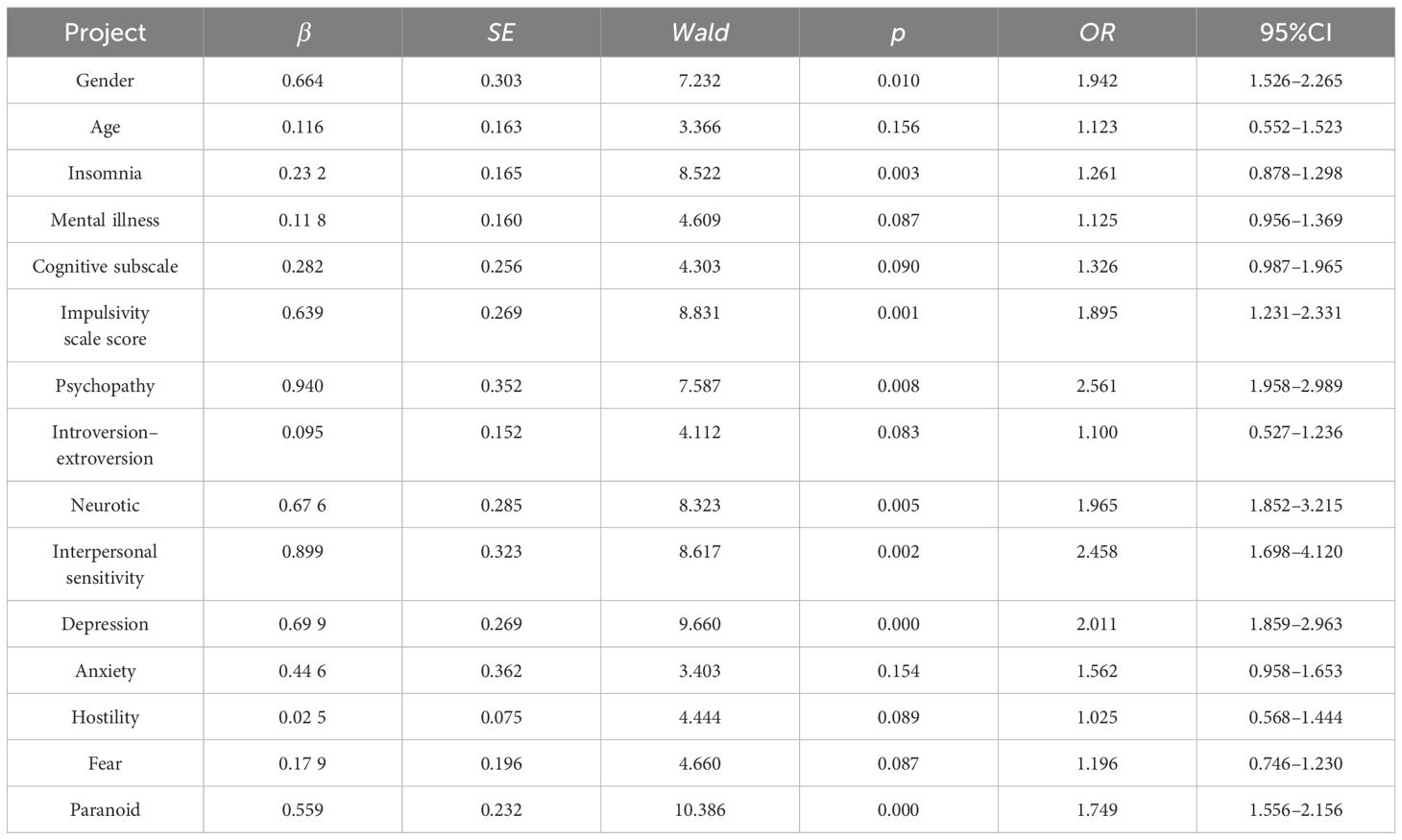
Table 2. Logistic multi-factor analysis of adolescents’ relationship with suicidal and self-injurious behaviors.
3.3 Analysis of different models of early warning models for adolescent suicide and self-injury behavior
The sensitivity and specificity of logistic regression in the early warning model of adolescent suicide and self-injury behavior are 0.9948 and 0.9981, respectively, which is the best performance; the performance of random forest, multi-level perceptron, and extreme gradient in the early warning model of adolescent suicide and self-injury behavior is acceptable. The K-neighbor algorithm and support vector machine performed poorly. See Figure 1 and Table 3.
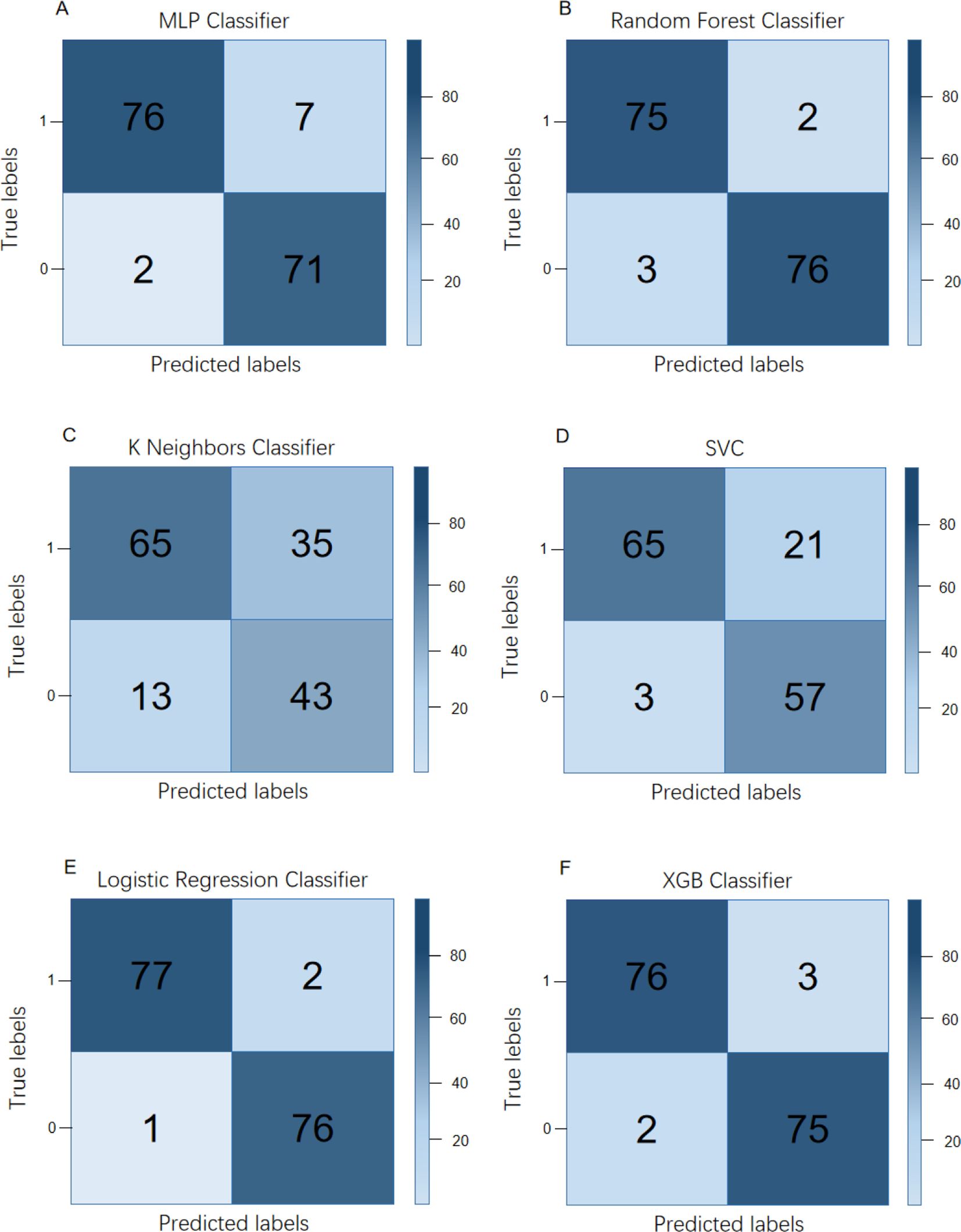
Figure 1. Confusion matrix of different models of adolescent suicide and self-injury behavior early warning models. (A) Multi-level perceptron; (B) random forest; (C) K-neighbor algorithm; (D) support vector machine; (E) logistic regression; and (F) extreme gradient boosting method.
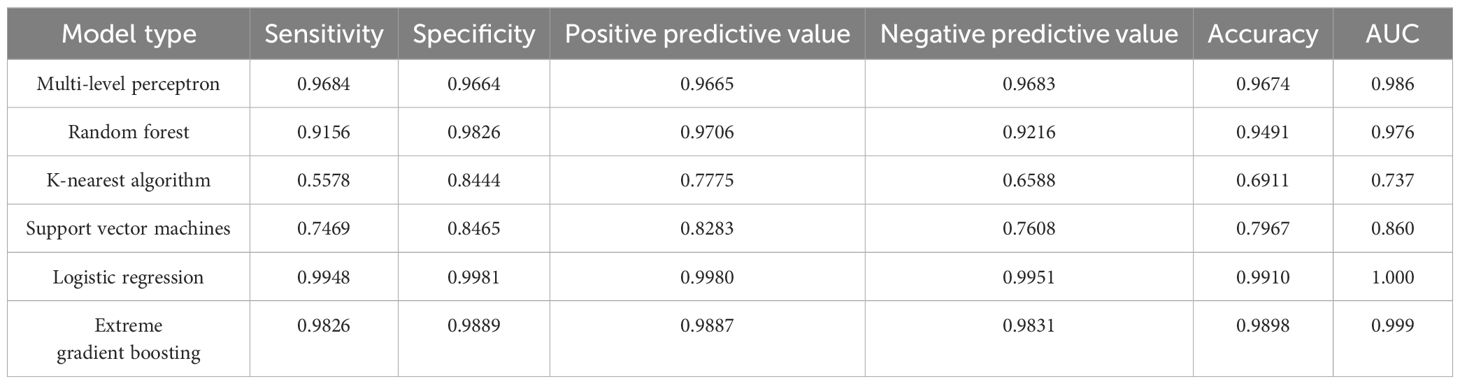
Table 3. Performance analysis of different models of early warning models for adolescent suicide and self-injury behavior.
4 Discussion
Suicide and self-injury among teenagers are a relatively complex behavioral problem that is not conducive to healthy physical and mental development. It also brings huge losses to social resources and human costs (14). The current domestic research reports on adolescent suicidal and self-injurious behaviors have a small sample size and insufficient attention, which may lead to repeated screening and missed selections, resulting in the inability to early identify adolescent suicidal and self-injurious behaviors and implement the timely and effective intervention.
The results of this study showed that 78 teenagers had suicidal and self-injurious behaviors, with a detection rate of 2.60% (78/3,000). According to the presence of suicidal and self-injury behaviors, they were divided into suicide and self-injury groups and non-suicide and self-injury groups. The outcomes showed an obvious increase in the proportion of male adolescents, late adolescence, and insomnia in the self-injury and suicide ones compared to that in the non-suicide and self-injury ones (p < 0.05). It had an obvious increase in the points of cognitive subscales, impulsivity scales, various dimensions of psychoticism, introversion extroversion, neuroticism, and interpersonal sensitivity, depression, anxiety, hostility, terror, and paranoia among adolescents in the self-injury and suicide ones in comparison to those in the non-suicide and self-injury ones (p <0.05). However, in the suicide and self-injury behavior group, the detection rate of women is higher than that of men. This may be because female adolescents are emotionally fragile and highly dependent, which leads to a higher detection rate of suicide and self-injury; in addition, compared with men, women mature psychologically earlier and are more emotionally sensitive, so they should be a key focus for preventing suicide and self-harm behavior (15, 16). Before the age of 18, adolescents are at greater risk of committing suicide and self-harm, but after the age of 18 and beyond, adolescents are more likely to engage in behaviors such as smoking and drinking (17). At the age of 18 and early adulthood, if adolescents fail to form a better identity or encounter new challenges and pressures from family and society after adulthood, they may suffer from temporary or long-term identity confusion, leading to excessive and risky behavior during the process of trial and error and, in severe cases, even deviating from social requirements (18). Research shows that family conflicts, psychological problems, interpersonal distress, and emotional problems are all important factors leading to suicide and self-harm among teenagers (19, 20). The above statistically significant variables were included in the logistic risk regression model for analysis. The results showed that gender, impulsivity scale score, psychoticism, neuroticism, interpersonal sensitivity, depression, and paranoia are independent risks that affect adolescent suicide and self-injury behavior factor. Studies such as Korczak (21) and O’Beaglaoich (22) have shown that suicidal ideation can be one of the most important proximal risk factors for suicide and can also be an independent risk factor. However, various factors for example self-esteem and social interaction can have a certain effect on suicidal ideation, which leads to a lack of specificity. The final suicide mortality rate of people with a history of suicide attempts is significantly higher than that of the general population, approximately 20–30 times; more than 50% of adolescents with a history of suicide attempts will commit suicide again in the future, and approximately one-half of those with suicidal ideation will attempt suicide within 1 year. Factors such as personality impulsivity and aggression, psychoticism, and neuroticism are inseparable from the occurrence and development of adolescent suicide and self-injury behaviors. Among them, impulsive and aggressive personalities can play a role in promoting suicide and self-injury behaviors in adolescents, especially the latter are more likely to commit suicide and self-injury. Neurotic personalities lack goals and plans, behave impulsively and blindly, and will show strong emotional reactions to the slightest stimulation, which may also be accompanied by suicide. Self-injurious behavior, in states of anxiety and depression, recent physical and mental health conditions, and the quality of intimate relationships can have an impact on adolescents, prompting them to make life and death decisions and further take action (23, 24).
Machine learning can assist in diagnosis, disease classification prediction, medical image recognition, etc. It is an important tool that can be used to judge and predict specific content in the real world. It has been widely used in the field of science and engineering and has gradually penetrated people’s daily life (25, 26). When adolescents engage in suicidal and self-injurious behaviors, large-sample studies can be used to study and further understand the social psychology related to the behavior, especially the personality and mental health of adolescents, and even key biological characteristics and risk factors, which have a crucial function in the early prediction of adolescent suicidal self-injury behavior and timely and effective intervention (27–30). In recent years, domestic and foreign scholars have used machine learning methods to construct classification and prediction models for suicide and self-injury behaviors, which have significantly improved the accuracy of predicting suicide and self-injury behaviors. For example, Wang (31) and Di (32) et al. discovered the representation of individuals with suicidal tendencies in a functional magnetic resonance imaging (fMRI) study and proposed to try to find patients with a high risk of suicidal behavior. Based on fMRI, the support vector machine recursive feature elimination method (SVM-RFE) was used to effectively identify patients with only suicidal ideation and a history of suicide attempts. The results showed that the cross-validation sensitivity was 73.10%, the specificity was 84.00%, and the accuracy was 88.20%.
At this stage, machine learning about suicide is mainly focused on the adult population, and 16–20 years old is the most common stage for mental health problems among adolescents, with approximately 75% of mental health problems occurring at this stage. From a psychological point of view, the “self-awareness” of adolescents at this stage has significantly improved, and they desire to be treated as an adult and have their personality respected. However, their independent requirements are usually ignored. Therefore, this study analyzes the risk factors of suicide and self-injury among adolescents by constructing a machine learning model of suicidal and self-injurious behavior to provide more possibilities for predicting suicidal and self-injurious behavior. The sensitivity and specificity of logistic regression in the early warning model of adolescent suicide and self-injury behavior are 0.9948 and 0.9981, respectively, which is the best performance; the performance of random forest, multi-level perceptron and extreme gradient in the early warning model of adolescent suicide and self-injury behavior is acceptable; and the K-neighbor algorithm and support vector machine performed poorly. Using machine learning methods to construct a classification prediction model for adolescent suicide and self-injury behavior is of great value for the early identification of adolescent risky behaviors. In future research, this method can also be used to analyze and predict the possible risky behaviors of other research subjects.
To sum up, the detection rate of suicidal and self-injurious behaviors in women is higher than that in men, and adolescents with impulsiveness, psychoticism, neuroticism, interpersonal sensitivity, depression, and paranoia are more likely to engage in suicidal and self-injurious behaviors. The classification and prediction model of adolescent suicide and self-injury behavior constructed using machine learning is conducive to identifying adolescent risky behaviors and providing targeted intervention for their risky behaviors. However, this study has obvious limitations. First, the obtained research content is only derived from cross-sectional data and is not analyzed in the direction of forward-looking or cohort studies, which may lead to group effects or neglect of some key points in the development process. The second point is that the time limit for suicidal and self-injurious behavior is relatively long, and this kind of behavior poses a greater threat to life. The practical value of short-term prediction is high and can effectively reduce the incidence of risk events. Third, the survey and analysis are conducted through self-reporting by adolescents. There may be concealment of risky behaviors, and there is also retrospective bias; hence, the accuracy needs to be examined.
Data availability statement
The raw data supporting the conclusions of this article will be made available by the authors, without undue reservation.
Ethics statement
The studies involving humans were approved by First Affiliated Hospital of Chongqing Medical University. The studies were conducted in accordance with the local legislation and institutional requirements. Written informed consent for participation in this study was provided by the participants’ legal guardians/next of kin.
Author contributions
YG: Conceptualization, Data curation, Formal analysis, Funding acquisition, Investigation, Methodology, Project administration, Resources, Supervision, Validation, Writing – original draft, Writing – review & editing. LK: Conceptualization, Data curation, Formal analysis, Funding acquisition, Methodology, Resources, Supervision, Validation, Writing – original draft, Writing – review & editing. X-MX: Conceptualization, Formal analysis, Investigation, Project administration, Software, Visualization, Writing – original draft, Writing – review & editing. MA: Formal analysis, Investigation, Methodology, Project administration, Software, Validation, Visualization, Writing – original draft, Writing – review & editing. J-LH: Data curation, Formal analysis, Investigation, Methodology, Project administration, Resources, Software, Validation, Writing – original draft, Writing – review & editing. WW: Conceptualization, Formal analysis, Investigation, Methodology, Project administration, Resources, Software, Visualization, Writing – original draft, Writing – review & editing. SH: Conceptualization, Formal analysis, Methodology, Project administration, Resources, Software, Validation, Visualization, Writing – original draft, Writing – review & editing. JMC: Data curation, Formal analysis, Methodology, Project administration, Resources, Software, Validation, Visualization, Writing – original draft, Writing – review & editing. JC: Data curation, Formal analysis, Investigation, Methodology, Project administration, Supervision, Validation, Visualization, Writing – original draft, Writing – review & editing. QZ: Formal analysis, Investigation, Methodology, Project administration, Resources, Software, Validation, Visualization, Writing – original draft, Writing – review & editing.
Funding
The author(s) declare financial support was received for the research, authorship, and/or publication of this article. This study was funded by the National Natural Science Foundation of China (Grant 81971286) and the Chongqing Municipal Science and Technology Commission (Grant CSTC2021-jscx-gksb-N0002). The First Affiliated Hospital of Chongqing Medical University “Discipline Peak Plan” scientific and technological achievement transformation project (cyyy-xkdfjh-cgzh-202304).
Acknowledgments
We thank the members of The First Affiliated Hospital of Chongqing Medical University Medical University for helpful discussions. We apologize to the scientists whose work could not be cited due to space limitations.
Conflict of interest
The authors declare that the research was conducted in the absence of any commercial or financial relationships that could be construed as a potential conflict of interest.
Generative AI statement
The author(s) declare that no Generative AI was used in the creation of this manuscript.
Publisher’s note
All claims expressed in this article are solely those of the authors and do not necessarily represent those of their affiliated organizations, or those of the publisher, the editors and the reviewers. Any product that may be evaluated in this article, or claim that may be made by its manufacturer, is not guaranteed or endorsed by the publisher.
References
1. Pfaff N, DaSilva A, Ozer E, Vemula Kaiser S. Adolescent risk behavior screening and interventions in hospital settings: A scoping review. Pediatrics. (2021) 147:e2020020610. doi: 10.1542/peds.2020-020610
2. Vázquez López P, Armero Pedreira P, Martínez-Sánchez L, García Cruz JM, Bonetde Luna C, Notario Herrero F, et al. Self-injury and suicidal behavior in children and youth populations: Learning from the pandemic. Pediatr (Engl Ed). (2023) 98:204–12. doi: 10.1016/j.anpede.2022.11.005
3. Wasserman D, Carli V, Iosue M, Javed A, Herrman H. Suicide prevention in childhood and adolescence: a narrative review of current knowledge on risk and protective factors and effectiveness of interventions. Asia Pac Psychiatry. (2021) 13:e12452. doi: 10.1111/appy.12452
4. Sohn MN, Dimitropoulos G, Ramirez A, McPherson C, Anderson A, Munir A, et al. Non-suicidal self-injury, suicidal thoughts and behaviors in individuals with an eating disorder relative to healthy and psychiatric controls: A systematic review and meta-analysis. Int J Eat Disord. (2023) 56:501–15. doi: 10.1002/eat.23880
5. Ammerman BA, Jacobucci R, Kleiman EM, Uyeji LL, McCloskey MS. The relationship between nonsuicidal self-injury age of onset and severity of self-harm. Suicide Life Threat Behav. (2018) 48:31–7. doi: 10.1111/sltb.2018.48.issue-1
6. Rosario-Williams B, Kaur S, Miranda R. Examining decentering as a moderator in the relation between non-suicidal self-injury and suicide ideation via cognitive-affective factors. Suicide Life Threat Behav. (2021) 51:741–754. doi: 10.1111/sltb.12747
7. Lalouni M, Fust J, Bjureberg J, Kastrati G, Fondberg R, Fransson P, et al. Augmented pain inhibition and higher integration of pain modulatory brain networks in women with self-injury behavior. Mol Psychiatry. (2022) 27:3452–9. doi: 10.1038/s41380-022-01639-y
8. Obegi JH. How common is recent denial of suicidal ideation among ideators, attempters, and suicide decedents? A literature review. Gen Hosp Psychiatry. (2021) 72:92–5. doi: 10.1016/j.genhosppsych.2021.07.009
9. Iskric A, Ceniti AK, Bergmans Y, McInerney S, Rizvi SJ. Alexithymia and self-harm: A review of nonsuicidal self-injury, suicidal ideation, and suicide attempts. Psychiatry Res. (2020) 288:112920. doi: 10.1016/j.psychres.2020.112920
10. Feng G, Zheng KI, Li YY, Rios RS, Zhu PW, Pan XY, et al. Machine learning algorithm outperforms fibrosis markers in predicting significant fibrosis in biopsy-confirmed NAFLD. J Hepatobiliary Pancreat Sci. (2021) 28:593–603. doi: 10.1002/jhbp.v28.7
11. Birchmeier ME, Studer T, Lutterotti A, Penner IK, Bignens S. Digitalisation of the brief visuospatial memory test-revised and evaluation with a machine learning algorithm. Stud Health Technol Inform. (2020) 270:168–72. doi: 10.3233/SHTI200144
12. Chen J, Chokshi S, Hegde R, Gonzalez J, Iturrate E, Aphinyanaphongs Y, et al. Development, implementation, and evaluation of a personalized machine learning algorithm for clinical decision support: case study with shingles vaccination. J Med Internet Res. (2020) 22:e16848. doi: 10.2196/16848
13. Furui K, Morishima I, Morita Y, Kanzaki Y, Takagi K, Yoshida R, et al. Predicting long-term freedom from atrial fibrillation after catheter ablation by a machine learning algorithm: Validation of the CAAP-AF score. J Arrhythm. (2020) 36:297–303. doi: 10.1002/joa3.12303
14. Kothgassner OD, Goreis A, Robinson K, Huscsava MM, Schmahl C, Plener PL. Efficacy of dialectical behavior therapy for adolescent self-harm and suicidal ideation: a systematic review and meta-analysis. Psychol Med. (2021) 51:1057–67. doi: 10.1017/S0033291721001355
15. Jia Z, Wen X, Chen F, Zhu H, Li C, Lin Y, et al. Cumulative exposure to adverse childhood experience: depressive symptoms, suicide intensions and suicide plans among senior high school students in nanchang city of China. Int J Environ Res Public Health. (2020) 17:4718. doi: 10.3390/ijerph17134718
16. Zhang YY, Lei YT, Song Y, Lu RR, Duan JL, Prochaska JJ. Gender differences in suicidal ideation and health-risk behaviors among high school students in Beijing, China. J Glob Health. (2019) 9:010604. doi: 10.7189/jogh.09.010604
17. Asarnow JR, Berk MS, Bedics J, Adrian M, Gallop R, Cohen J, et al. Dialectical behavior therapy for suicidal self-harming youth: emotion regulation, mechanisms, and mediators. J Am Acad Child Adolesc Psychiatry. (2021) 60:1105–15. doi: 10.1016/j.jaac.2021.01.016
18. Lim KS, Wong CH, McIntyre RS, Wang J, Zhang Z, Tran BX, et al. Global lifetime and 12-month prevalence of suicidal behavior, deliberate self-harm and non-suicidal self-injury in children and adolescents between 1989 and 2018: A meta-analysis. Int J Environ Res Public Health. (2019) 16:4581. doi: 10.3390/ijerph16224581
19. Devine C, Cohen-Cline H. Social and behavioral pathways between adverse childhood experiences and poor adult physical health: mediation by early adulthood experiences in a low-income population. Int J Environ Res Public Health. (2022) 19:10578. doi: 10.3390/ijerph191710578
20. Nyundo A, Manu A, Regan M, Ismail A, Chukwu A, Dessie Y, et al. Factors associated with depressive symptoms and suicidal ideation and behaviors amongst sub-Saharan African adolescents aged 10-19 years: cross-sectional study. Trop Med Int Health. (2020) 25:54–69. doi: 10.1111/tmi.13336
21. Korczak DJ, Finkelstein Y, Barwick M, Chaim G, Cleverley K, Henderson J, et al. A suicide prevention strategy for youth presenting to the emergency department with suicide related behavior: protocol for a randomized controlled trial. BMC Psychiatry. (2020) 20:20. doi: 10.1186/s12888-019-2422-y
22. O'Beaglaoich C, McCutcheon J, Conway PF, Hanafin J, Morrison TG. Adolescent suicide ideation, depression and self-esteem: relationships to a new measure of gender role conflict. Front Psychol. (2020) 11:111. doi: 10.3389/fpsyg.2020.00111
23. Younes F, Halawi G, Jabbour H, El Osta N, Karam L, Hajj A, et al. Internet addiction and relationships with insomnia, anxiety, depression, stress and self-esteem in university students: A cross-sectional designed study. PloS One. (2016) 11:e0161126. doi: 10.1371/journal.pone.0161126
24. Stanley IH, Boffa JW, Rogers ML, Hom MA, Albanese BJ, Chu C, et al. Anxiety sensitivity and suicidal ideation/suicide risk: A meta-analysis. J Consult Clin Psychol. (2018) 86 :946–60. doi: 10.1037/ccp0000342
25. D'Angelo T, Caudo D, Blandino A, Albrecht MH, Vogl TJ, Gruenewald LD, et al. Artificial intelligence, machine learning and deep learning in musculoskeletal imaging: Current applications. J Clin Ultrasound. (2022) 50:1414–31. doi: 10.1002/jcu.23321
26. Passos IC, Ballester PL, Barros RC, Librenza-Garcia D, Mwangi B, Birmaher B, et al. Machine learning and big data analytics in bipolar disorder: A position paper from the International Society for Bipolar Disorders Big Data Task Force. Bipolar Disord. (2019) 21:582 –594. doi: 10.1111/bdi.12828
27. Maurer JM, Harenski KA, Paul S, Vergara VM, Stephenson DD, Gullapalli AR, et al. Machine learning classification of chronic traumatic brain injury using diffusion tensor imaging and NODDI: A replication and extension study. NeuroImage Rep. (2023) 3:100157. doi: 10.1016/j.ynirp.2023.100157
28. Fisher LB, Curtiss JE, Klyce DW, Perrin PB, Juengst SB, Gary KW, et al. Using machine learning to examine suicidal ideation after traumatic brain injury: A traumatic brain injury model systems national database study. Am J Phys Med Rehabil. (2023) 102:137–43. doi: 10.1097/PHM.0000000000002054
29. Tate AE, McCabe RC, Larsson H, Lundström S, Lichtenstein P, Kuja-Halkola R. Predicting mental health problems in adolescence using machine learning techniques. PloS One. (2020) 15:e0230389. doi: 10.1371/journal.pone.0230389
30. Rothenberg WA, Bizzego A, Esposito G, Lansford JE, Al-Hassan SM, Bacchini D, et al. Predicting adolescent mental health outcomes across cultures: A machine learning approach. J Youth Adolesc. (2023) 52:1595 –1619. doi: 10.1007/s10964-023-01767-w
31. Wang Y, Qin Y, Li H, Yao D, Sun B, Gong J, et al. Identifying internet addiction and evaluating the efficacy of treatment based on functional connectivity density: A machine learning study. Front Neurosci. (2021) 15:665578. doi: 10.3389/fnins.2021.665578
Keywords: machine learning algorithm, suicidal and self-injurious behavior, adolescents, risk factors, prediction model
Citation: Gan Y, Kuang L, Xu X-M, Ai M, He J-L, Wang W, Hong S, Chen Jm, Cao J and Zhang Q (2025) Research on prediction model of adolescent suicide and self-injury behavior based on machine learning algorithm. Front. Psychiatry 15:1521025. doi: 10.3389/fpsyt.2024.1521025
Received: 01 November 2024; Accepted: 30 December 2024;
Published: 06 March 2025.
Edited by:
Yibo Wu, Peking University, ChinaReviewed by:
Brandt Wiskur, University of Oklahoma Health Sciences Center, United StatesYueh-Ming Tai, Tri-Service General Hospital Beitou Branch, Taiwan
Copyright © 2025 Gan, Kuang, Xu, Ai, He, Wang, Hong, Chen, Cao and Zhang. This is an open-access article distributed under the terms of the Creative Commons Attribution License (CC BY). The use, distribution or reproduction in other forums is permitted, provided the original author(s) and the copyright owner(s) are credited and that the original publication in this journal is cited, in accordance with accepted academic practice. No use, distribution or reproduction is permitted which does not comply with these terms.
*Correspondence: Li Kuang, TGlLdWFuZzEwMTZAMTI2LmNvbQ==