- 1Department of Adult Psychiatry, Jagiellonian University Medical College, Kraków, Poland
- 2Department of Adult, Child and Adolescent Psychiatry, University Hospital in Cracow, Kraków, Poland
- 3J. Dietl Specialist Hospital, Kraków, Poland
- 4Department of Cognitive Neuroscience and Neuroergonomics, Institute of Applied Psychology, Jagiellonian University, Kraków, Poland
- 5Doctoral School in the Social Sciences, Jagiellonian University, Kraków, Poland
- 6Chair of Radiology, Jagiellonian University Medical College, Kraków, Poland
- 7Faculty of Psychology, SWPS University, Katowice, Poland
- 8Department of Affective Disorders, Jagiellonian University Medical College, Kraków, Poland
Introduction: Schizophrenia (SZ) and bipolar disorder (BD) share common clinical features, symptoms, and neurocognitive deficits, which results in common misdiagnosis. Recently, it has been suggested that alterations within brain networks associated with perceptual organization yield potential to distinguish SZ and BD individuals. The aim of our study was to evaluate whether functional connectivity (FC) of the dorsal attention network (DAN) may differentiate both conditions
Methods: The study involved 90 participants: 30 remitted SZ patients, 30 euthymic BD patients, and 30 healthy controls (HC). Resting state functional magnetic resonance imaging was used to compare the groups in terms of the FC within the core nodes of the DAN involving frontal eye fields (FEF) and intraparietal sulcus (IPS)
Results: BD patients presented weaker inter-hemispheric FC between right and left FEF than HC. While SZ did not differ from HC in terms of inter-FEF connectivity, they presented increased inter- and intra-hemispheric FC between FEF and IPS. When compared with BD, SZ patients showed increased FC between right FEF and other nodes of the network (bilateral IPS and left FEF)
Conclusion: We have shown that altered resting state FC within DAN differentiates BD, SZ, and HC groups. Divergent pattern of FC within DAN, consisting of hypoconnectivity in BD and hyperconnectivity in SZ, might yield a candidate biomarker for differential diagnosis between both conditions. More highly powered studies are needed to confirm these possibilities
1 Introduction
Schizophrenia (SZ) and bipolar disorder (BD) are chronic and severe mental disorders that presents significant overlap in their clinical features (1), cognitive dysfunctions (2), neurological deficits (3–5), as well as structural and functional brain abnormalities (6). Due to the similarities, both conditions are often misdiagnosed (1). Chart records show that SZ is the second most common misdiagnosis of BD (7), and up to 31% of those patients may be diagnosed with SZ or other psychotic disorder (8). Thus, there is a vital need to seek for the objective biomarkers that can differentiate between these two conditions.
With that aim, a growing number of studies use functional magnetic resonance imaging (fMRI), a promising and non-invasive method that can capture the intrinsic architecture of brain activity alterations in SZ and BD (9–11). This technique enables evaluation of large-scale functional networks, distributed sets of brain areas synchronically activated at rest or during task performance that corresponds with cognitive and mental processes (12). Those systems include default mode network (DMN), salience network (SAN), limbic network (LIN), frontoparietal network (FPN), somatosensory network (SMN), visual network (VIN), and dorsal attention network (DAN) (12). Recently, the latter one has become an object of interest due to its potential to differentiate SZ and BD patient groups (13). The DAN is recruited when attention is voluntarily shifted to spatial locations or during intentional visual exploration using eye movements (14). This network is composed of bilateral regions in the parietal and frontal cortex involving the intraparietal sulcus (IPS), which plays a key role in the selection among competing stimuli, and the frontal eye fields (FEF), which contain a representation of the priority of items in the visual field, to plan and execute eye movements (15). Recently, Keane et al. (2023) analyzed the activity of this network showing that among 12 large-scale functional networks, only the DAN was capable of differentiating BD and SZ during perceptual organization task (13). Authors suggested that altered activity within this functional network might yield a candidate biomarker for differential diagnosis of those conditions (13).
Neuroimaging and neurophysiological studies report alterations within DAN structures in both conditions. SZ patients have been shown to present oculomotor deficits that are associated with disrupted activity of the FEF (16–18) and parietal cortex (18). Moreover, increased activity within parietal regions of DAN as well as FEF hypoactivation has been linked with working memory impairments in this clinical group (19, 20). rs-fMRI and recent EEG studies demonstrated that SZ patients present DAN dysconnectivity that may be related to disruptions in attentional processes and integration of information from a different brain area (21–23). Only a few studies evaluated dysfunctions of this network in BD. Two rs-fMRI analyses demonstrated that euthymic BD patients present altered FEF activity and a decreased functional connectivity (FC) with thalamus and cerebellar structures related to oculomotor control (24, 25). A single fMRI study has shown that hypoactivation of this region is associated with working memory deficits in this clinical group (20). Studies comparing both clinical groups demonstrated that contrary to BD, SZ individuals present altered brain responses during attentional processes (26, 27). These observations encourage further studies on DAN activity in those conditions. Despite the popularity of fMRI analyses in psychiatric disorders, there is scarcity of studies directly comparing the activity of the large-scale functional networks between SZ and BD patients. Interestingly, most of the single scanner studies did not include DAN in their analyses (28–33). Majority of research on functional connectivity (FC) in SZ and BD perform whole-brain analyses rather than hypothesis-driven evaluation of specific networks resulting in substantial variety of findings and leading to contradictory conclusions (34). To the best of our knowledge, no study has performed hypothesis-driven comparisons of DAN FC in these disorders.
Resting state fMRI (rs-fMRI) evaluates baseline neuronal brain activity during the absence of goal-directed external stimuli. An important advantage of this method is the lack of behavioral task performed by the participants, which significantly simplifies the procedure, shortens scanning time, and minimizes its burden on patients. While Kaene et al. (2023) showed that DAN activity during perceptual organization task may differentiate the BD and SZ groups (13), in our study, we aimed to test whether FC of this network measured during resting state may also reveal such potential. If alterations in resting state FC (rs-FC) within DAN are sufficient to differentiate the abovementioned groups, the use of the rs-fMRI would significantly facilitate application of this biomarker in clinical practice. We hypothesize that altered FC of this large-scale network may differentiate BD, SZ, and healthy individuals.
2 Methods
2.1 Participants
The study was conducted on a group of participants described in our previous research (3). They were 30 SZ and 30 BD patients (12 BD I and 18 BD II patients), and 30 healthy controls (HCs). The diagnosis and clinical evaluation were performed by an experienced psychiatrist according to DSM-5 and ICD-10. The inclusion criteria for the BD group were the state of euthymia defined as <11 points in the Montgomery–Åsberg Depression Rating Scale (35) and <5 points in the Young Mania Rating Scale (36). SZ patients were recruited in the state of symptomatic remission, defined as three or fewer points on all Positive and Negative Syndrome Scale items. In our study, we focused on patients during a state of symptomatic remission as we aimed to assess FC alterations related to the diagnosis (trait marker) rather than those associated with the presence of affective or psychotic episode (state marker). Both clinical groups were treated with neuroleptics from the group of dibenzoxazepines (quetiapine, olanzapine, or clozapine). The selection of the abovementioned drugs provided a relative pharmacological homogeneity across patient groups. Patients undertaking lithium were excluded as the treatment may have had an impact on patients’ motor performance and cerebellar functions, which were evaluated in our project (3). Valproic acid and lamotrigine treatment was allowed. Exclusion criteria involved the following: history of drug or alcohol abuse according to the substance use disorder of DSM-5; chronic, severe, or acute somatic diseases; severe personality disorders; and treatment other than those mentioned in the inclusion criteria. HCs were recruited from the group of all of the authors’ social network (family members, friends, friends’ family members, as well as their acquaintances), and they were evaluated by an experienced psychiatrist. Healthy volunteers represented a wide range of communities and were not restricted to a single institution (e.g., University or Hospital). All of the individuals in this group reported a negative history of neurological and mental disorders and did not meet any exclusion criteria for patients. The SZ, BD, and HC groups were matched in terms of age and gender. Patient groups were matched for duration of their treatment. All of the participants were right-handed as measured by the Neurological Evaluation Scale (37). Table 1 presents the demographic characteristics of the studied groups. The study was approved by the Jagiellonian University Bioethic Committee. All participants signed a written consent prior to the assessment.
2.2 MRI acquisition
The procedure of MRI acquisition was adopted from our previous study (38). Siemens Skyra MR System (Siemens Medical Solutions, Erlangen, Germany) was used to acquire MRI data. Anatomical images were obtained using sagittal 3D, T1-weighted MPRAGE sequence with TR = 1,800 ms, TE = 2.26 ms, TI = 900 ms, voxel size of 1 mm3, and with the use of 64 channels coil. The total number of slices in the sagittal plane equaled 208, FOV = 256 × 256 mm2. Whole-brain images were covered with 20% gap axial slices and a distant factor of 0.5 mm. Generalized Autocalibrating Partial Parallel Acquisition (GRAPPA) with a factor of 3 was used to reduce the imaging time. A total of 13 min and 20 s of rs-fMRI EPI images were acquired using gradient-echo single-shot echo planar imaging sequence with the following parameters: TR = 800 ms; TE = 27 ms; slice thickness = 0.8 mm, voxel size = 3 mm3, and with no gap using 64-channel coil. A total of 52 inter leaved transverse slices and 1,000 volumes were obtained. Participants were instructed to keep their eyes open and not to think about anything during the scanning procedure. Simultaneous-multi-slice acquisition was acquired to enhance the sensitivity of hemodynamic response resulting in reduction of TR to 0.8 s.
2.3 Imaging data preprocessing
The rs-fMRI data processing was performed with the use of Data Processing & Analysis for Brain Imaging (DPABI) V6.0 (39) as well as SPM 12 (Wellcome Trust Centre for Neuroimaging, UCL, London, United Kingdom) in MATLAB version R2018a (The MathWorks, Inc., Natick, MA, United States). Preprocessing consisted of the following steps: 1) conversion of data from DICOM to Nifti format; 2) removing the first 10 time points to eliminate the effect of magnetic field instability; 3) slice timing correction, which is responsible for synchronizing the image that was originally collected at different time points. Image timing correction has been shown to reliably increase the sensitivity and effect power; 4) realignment—data on head movements were extracted and processed for each subject. The maximum deviation criterion was <3 mm or <3o; 5) voxel specific head motion—analysis and detection of head movements with an accuracy of 1–2 voxels (the smallest brain measurement units, 2 mm × 2 mm × 2 mm); 6) coregistration Fun-T1—the data of each subject were checked for matching functional (EPI) to structural data (T1) based on anatomical landmarks (brain ventricles and border points); 7) cropping and reorienting T1 images—cropping and transforming the image structural; 8) T1 Segmentation + DARTEL brain segmentation and normalization; 9) T1 coregistration to Fun—unification of all time points (1,000 points in time) so that each patient has one map of brain activity; 10) normalization using EPI template to the MNI space; 11) covariate regression: removing signal from white matter and cerebrospinal fluid so that only signal from gray matter remains; 12) CompCor—principal component analysis (PCA) of the first five components. PCA is a technique for reducing the dimensionality of such data sets, which minimizes information loss and increasing interpretability of the results.
2.4 Functional connectivity analysis
Region of interest (ROI)-to-ROI FC analysis was performed to evaluate the resting state activity with the DAN. ROIs were chosen based on the Harvard–Oxford Atlas and localized regarding their coordinates on the x-, y-, and z-axes. Raw time courses were extracted from each subject using “ROI Signal Extractor” module in Data Processing & Analysis for Brain Imaging (DPABI) V4.3 (39) in MATLAB version R2018a and SPM 12. The DAN was defined with the following ROIs: right FEF [FEF(R), 30, −6, 64], left FEF [FEF(L), −27, −9, 64], right IPS [IPS(R), 39, −42, 54], and left IPS [IPS(L), −39, −43, 52].
2.5 Statistical analysis
Demographic characteristics were compared with the use of one-way ANOVA, t-tests, and χ2 tests as appropriate. The Levene test was used to evaluate the homogeneity of variance. These data were reported by Chrobak et al. (2023) (3). One-way ANOVA corrected with the use of Benjamini and Hochberg (1995) false discovery rate (FDR, p < 0.05) was applied to compare FC among ROIs within the DAN between the SZ, BD, and HC groups (40). Bonferroni post-hoc tests were applied to perform pairwise comparisons. Antipsychotics’ daily dosage was converted to the equivalent of olanzapine according to Leucht et al. (2015) (41). Associations between demographic and clinical variables (age, duration of the treatment, years of education, and the equivalent of the daily dose of olanzapine) and FC measures were evaluated using Pearson correlations. Statistical analyses were made in R software (42).
3 Results
There were no statistically significant differences between the studied groups in terms of age, sex, years of education, duration of treatment, and equivalent of olanzapine daily dosage. Detailed results of those comparisons are presented in Table 1. There were significant differences between the SZ, BD, and HC groups in FC measures between the following ROI–ROI pairs: FEF(R)–FEF(L), FEF(R)–IPS(R), FEF(R)–IPS(L), and FEF(L)–IPS(L). In comparison to HC, BD patients presented decreased FC between FEF(R) and FEF(L). The SZ group showed stronger FC between FEF(R)–IPS(R), FEF(R)–IPS(L), FEF(L)–IPS(L) than HC. Finally, in comparison to BD, SZ patients revealed increased FC between FEF(R)–FEF(L), FEF(R)–IPS(R), and FEF(R)–IPS(L). Detailed results of those comparisons are presented in Table 2. Figures 1–3 depict networks revealing significant differences between the studied groups. Figure 4 presents the effect sizes, which reflect the strength of FC between the ROIs compared between the groups. There were no statistically significant correlations between FC measures and participant age (p = 0.08), years of education (p = 0.12), duration of treatment (p = 0.07), and the daily dose of olanzapine (p = 0.5).
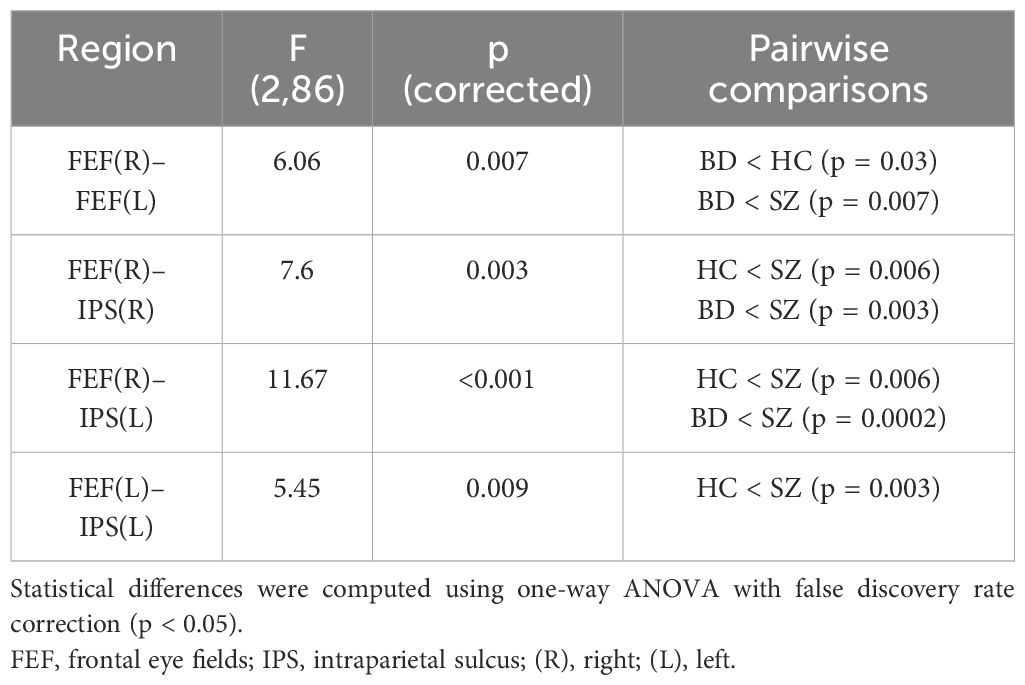
Table 2. Functional connectivity differences among schizophrenia (SZ), bipolar disorder (BD), and healthy controls (HC) groups.
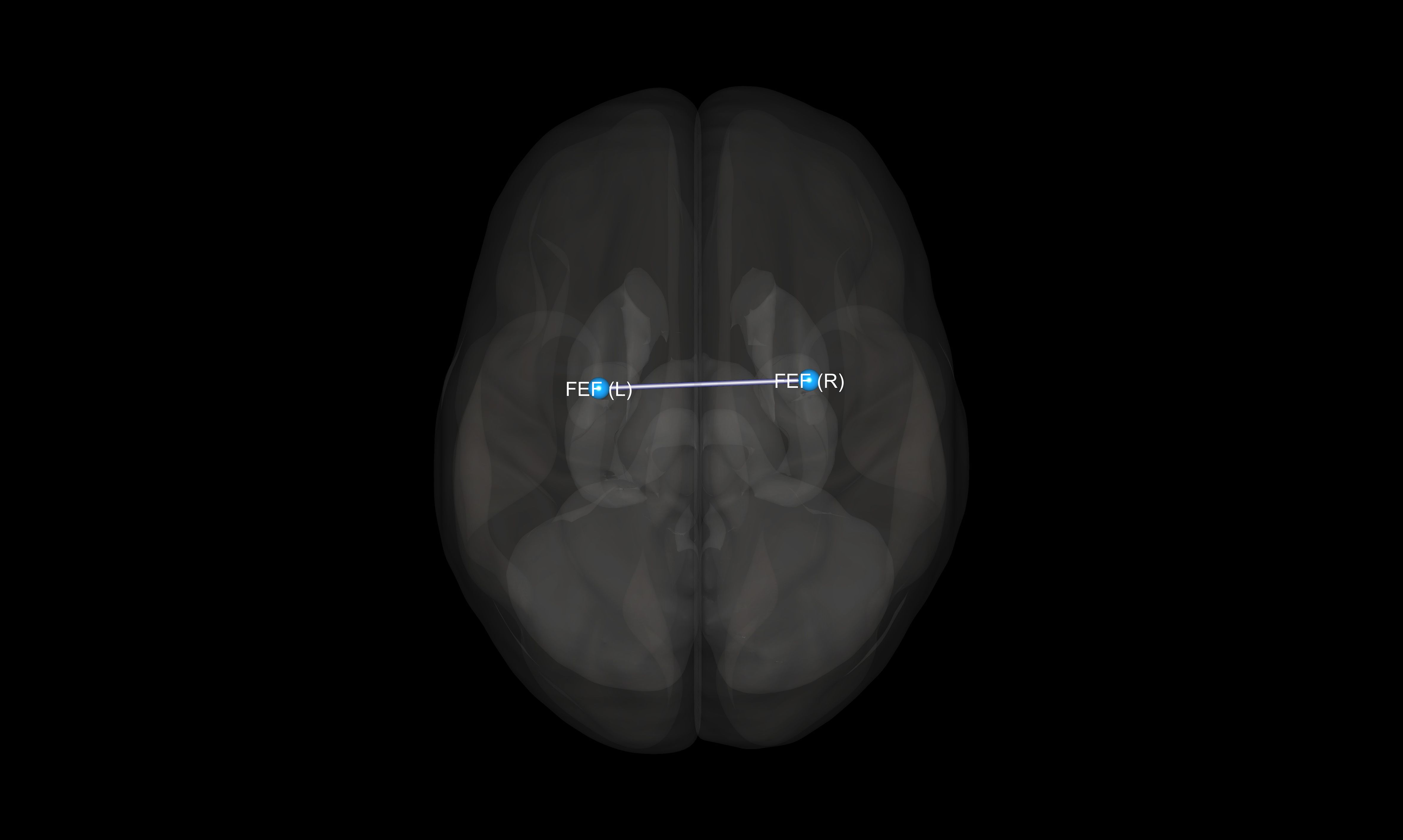
Figure 1. Visualization of significant differences in FC between bipolar disorder and healthy controls groups. FEF, frontal eye fields; (R), right; (L), left.
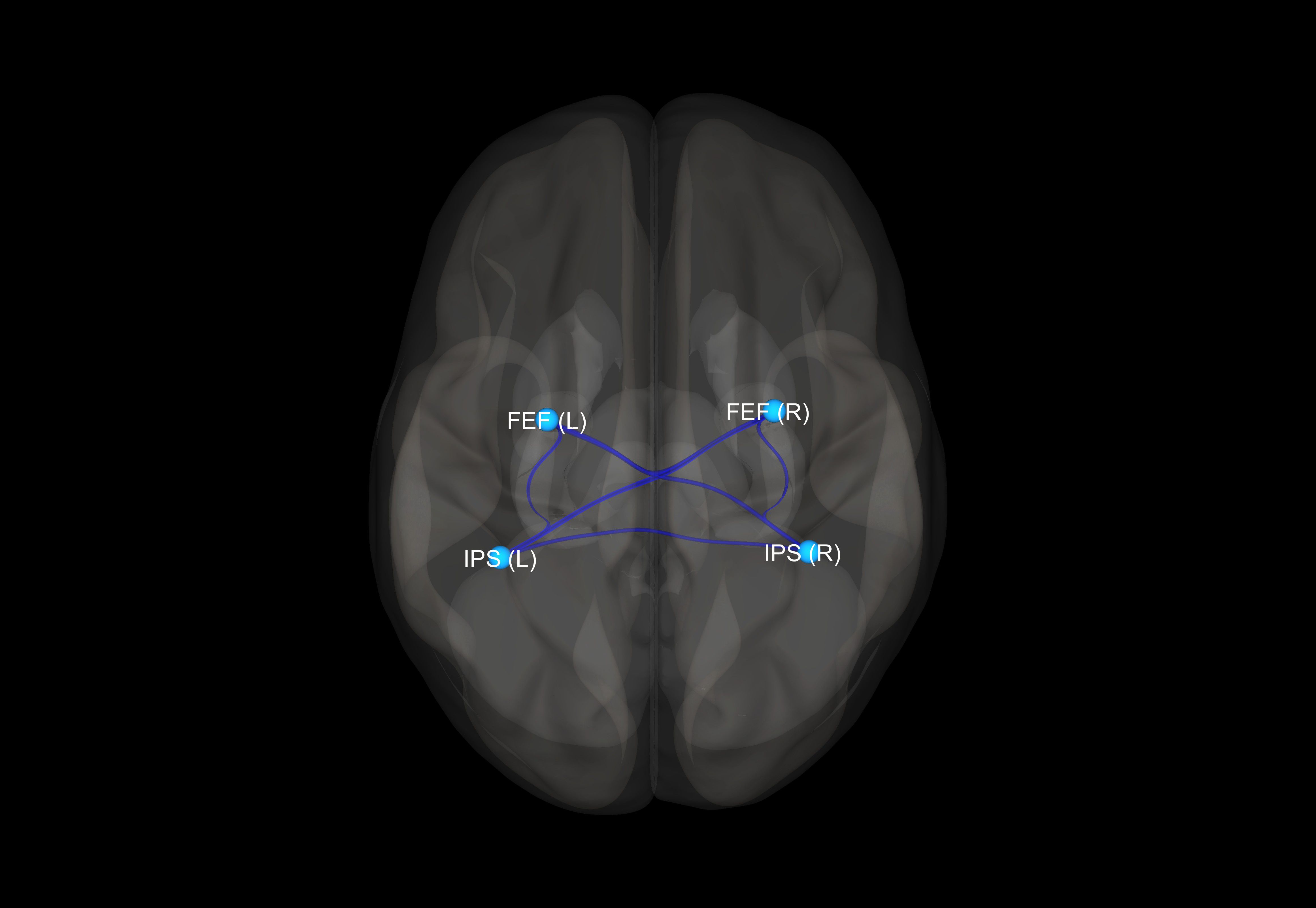
Figure 2. Visualization of significant differences in FC between schizophrenia and healthy control groups. FEF, frontal eye fields; IPS, intraparietal sulcus; (R), right; (L), left.
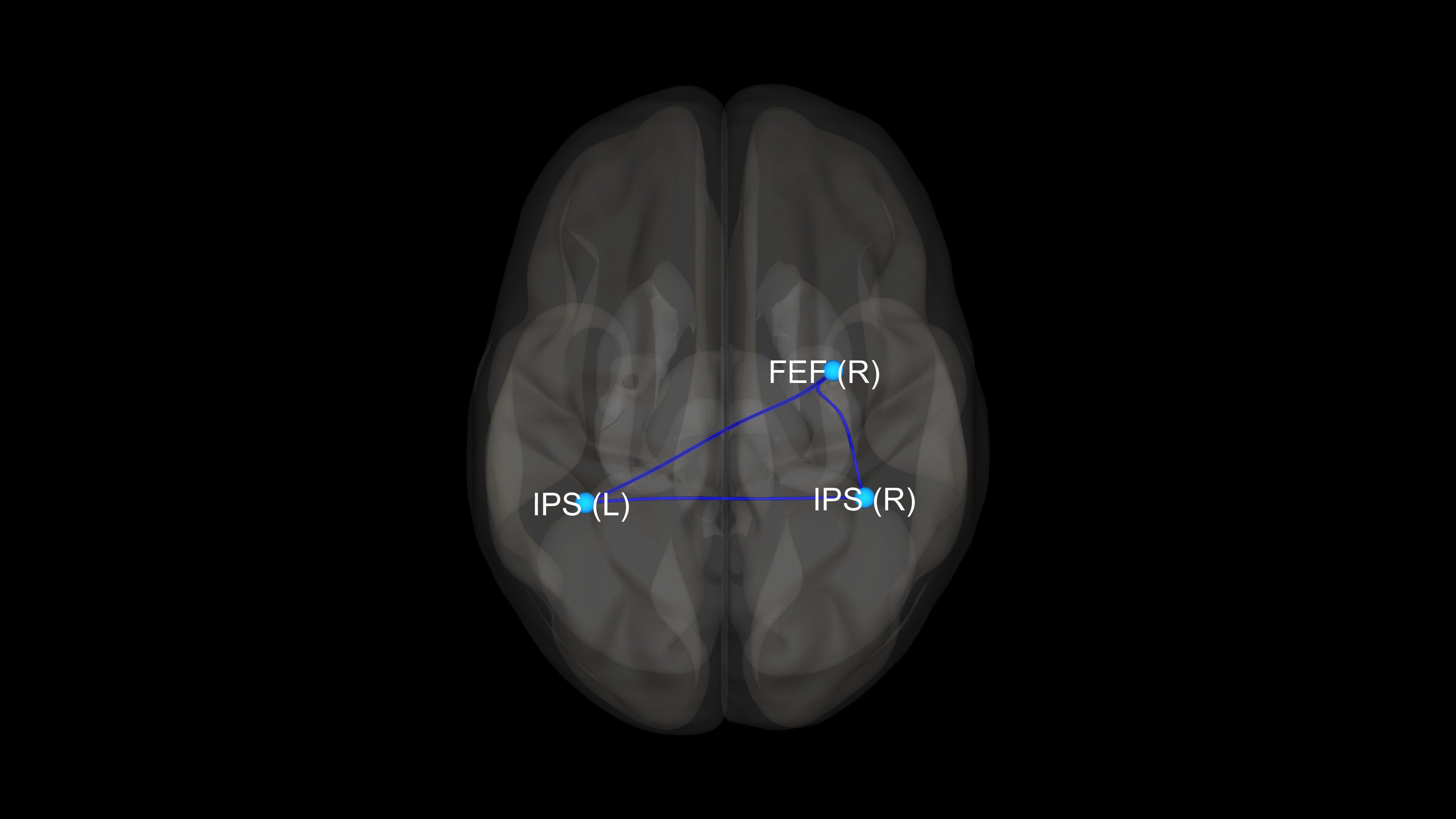
Figure 3. Visualization of significant differences in FC between schizophrenia and bipolar disorder patient groups. FEF, frontal eye fields; IPS, intraparietal sulcus; (R), right; (L), left.
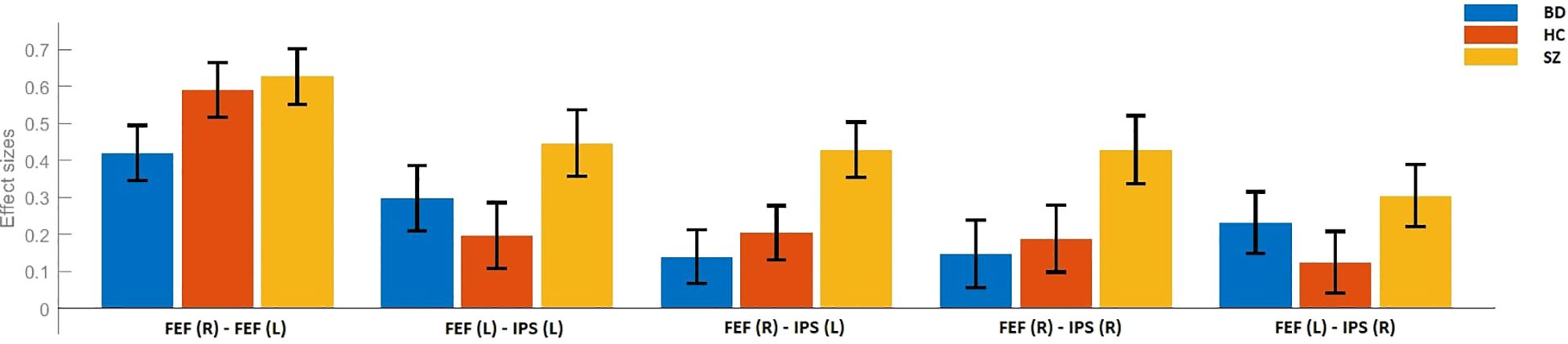
Figure 4. Effect sizes for the differences in FC between schizophrenia (SZ), bipolar disorder (BD), and healthy control (HC) groups. FEF, frontal eye fields; IPS, intraparietal sulcus; (R), right; (L), left.
4 Discussion
Our results have shown that altered resting state FC within DAN differentiates the BD, SZ, and HC groups. In comparison with HC, BD patients revealed inter-hemispheric hypoconnectivity between FEF regions. While the SZ group did not differ from HC in terms of these connections, they presented significant inter- and intra-hemispheric hyperconnectivity between FEF and IPS [FEF(R)–IPS(R), FEF(L)–IPS(L), and FEF(R)–IPS(L)]. Finally, according to our hypothesis, FC within DAN differentiated both clinical groups. In comparison with BD, SZ patients presented increased FC between FEF(R) and other nodes of this network [IPS(L), IPS(R), and FEF(L)].
It is hypothesized that interhemispheric functional connections between FEF regions are responsible for achieving consensus on the destination of the next saccade. In such system, cells that command similar movements mutually excite each other while inhibiting those that would produce conflicting movements, thus resolving the conflict through winner-takes-it-all mechanism (43). Machner et al. (2022) have shown that in the neurotypical brain, increased FC between FEFs is associated with improved learning (shorter reaction times) during orienting/reorienting task (14). Our results indicating lower FC among FEF in BD correspond with the observations of oculomotor deficiencies in BD including decreased ratio of eye velocity to target velocity, higher number of saccades, and vergence eye movement impairments (44, 45). Decreased FC in BD within DAN has been also linked with the presence of euthymia (46). Brady et al. (2017) have evaluated the group of 23 manic and 24 euthymic BD I patients, and 23 HC, indicating that FC between frontal, parietal, and occipital nodes within the DAN are significantly greater in mania than euthymia or HC subjects (46). However, BD patients in euthymia presented lower FC within DAN than HC, which is congruent with our study, strengthening the results. Further neuroimaging studies are necessary to identify the impact of the abovementioned factors on FC within DAN in BD patients across different affective episodes. Notably, BD patients do not differ from HC in FC associated with bilateral IPS indicating that BD patients do not exhibit significant impairments in visuospatial working memory, which involves recalling and manipulating images while remaining oriented in space (47). Surprisingly, the intrinsic architecture of brain activity in BD patients resembles HC participants more than SZ, while the FC between BD and HC remains significantly different only in FEF.
Connectivity patterns can be interpreted using the Gaussian curve and the Yerkes–Dodson law. Up to a certain point, higher functional connectivity signifies a healthy brain with substantial cognitive potential. This is also observed when concentrating on a difficult task or compensating for temporary or chronic deficits in functioning. Deficits and disconnections in one network have been shown to cause hyperconnectivity in another compensating for losses in the disrupted network. Presumably, hyperconnectivity constitutes an observable brain response to neuronal network disturbances (48, 49). However, full compensation is characteristic only of relatively healthy brains or as an early sign of disease (50–53). Various studies suggest that the neuronal networks of individuals with disturbed functioning are both ineffective as well as inadequate due to an inability to sufficiently compensate for their deficits (53–56). Notably, only transient hyperconnectivity is considered advantageous, while prolonged hyperconnectivity can lead to detrimental effects on the human brain. Hillary and Grafman (2017) present similar observations highlighting that while hyperconnectivity is adaptive in the short term, chronic hyperconnectivity makes network hubs vulnerable to secondary pathological processes due to chronically elevated metabolic stress (48). Moreover, chronic hyperconnectivity is associated with serious progressive neuronal impairments observed in schizophrenia (57, 58). Excessive connectivity increases brain metabolism leading to higher amyloid beta (Aβ) deposition, which accelerates brain aging and destabilizes its functioning. In summary, chronic hyperconnectivity, associated with compensatory mechanisms, can be seen as a biomarker of progressive neuronal impairments (48, 59–61).
Increased FC between bilateral FEF and IPS in SZ is consistent with previous studies indicating dysconnectivity within DAN in this clinical group (22). Disrupted activity of this system corroborates with the studies linking dorsal stream abnormalities with deficits of stereopsis (62), fragmented object recognition (63), motion perception (64), and cognitive disorganization (13). Our observations of IPSs dysconnectivity corresponds with the findings that SZ patients present altered activation of posterior parietal cortex and IPS that is linked with impairments of visual working memory and perceptual organization deficits, respectively (27, 65). Our results provide further evidence of DAN dysconnectivity in SZ. The observation that SZ patients present excessive FC within DAN compared to BD patients correspond with the results of the studies evaluating attention deficits and their neuronal underpinnings in those clinical groups. Several studies have shown that SZ patients present worse performance than HC during tasks known to activate DAN (visual search and Posner cueing tasks) (26, 66), while individuals with BD obtain results comparable to those of HC (67, 68). VanMeerten et al. (2016) have shown that contrary to BD, SZ patients present abnormal early brain responses during visual search task suggesting that diminished early posterior brain responses are specifically associated with SZ neuropathology (26). Pokorny et al. (2021) have demonstrated that SZ patients present aberrant cortical connectivity of IPS during object recognition task, which is not observed in BD (27). This observation corresponds with our results indicating that excessive between IPS and FEF are observed only in SZ, while there are no significant differences in terms of those connections between the BD and HC groups. Keane et al. (2023) suggested that differences in DAN task activity between BD, SZ, and HC are so large that they might yield a candidate biomarker for differential diagnosis. Results of our study points out that resting-state DAN-FC may have similar potential of discriminating the abovementioned groups as it reveals an opposite profile of activity between BD and SZ individuals (hypo- vs. hyperconnectivity) (13). The possibility of using rs-fMRI for this purpose is promising as this technique may be used without requiring any specific task or stimulus. To the best of our knowledge, this is the first study identifying differences within DAN FC between the abovementioned clinical groups. Most of the single-scanner studies evaluating large-scale functional networks did not include DAN in their analyses (28–33). A recent study of Huang et al. (2020) evaluating transdiagnostic and illness-specific functional dysconnectivity across SZ, BD, and major depressive disorder patients did not find differences in FC within DAN between the studied group; however, an abnormal connectivity pattern between DAN and the left hippocampus has been recognized as one of the SZ-specific deficits (20). Further studies are required to establish the potential of DAN–FC to differentiate the abovementioned groups.
We are aware of several limitations of our study as follows: (a) a relatively small number of participants; (b) heterogeneity of BD patient group in terms of the disorders type (12 BD I and 18 BD II individuals)—small number of subjects in those groups did not allow us to analyze the effect of this variable on DAN–FC; (c) patients were not drug naïve, which could affect the results; however, we have shown that equivalent of the daily dose of olanzapine is not associated with FC measures, which corresponds with the results of a recent study indicating no impact of antipsychotics on connectivity strength in BD and SZ (69).
Our study shows that resting state FC within DAN may differentiate euthymic BD, remitted SZ, and healthy individuals. Divergent patterns of FC within DAN, reflected in hyperconnectivity in BD and hypoconnectivity in SZ, might yield a candidate biomarker for differential diagnosis between both conditions. More highly powered analyses are needed to confirm these possibilities. Future studies should evaluate whether resting state FC within DAN is associated with attention deficits in SZ and BD.
Data availability statement
The raw data supporting the conclusions of this article will be made available by the authors, without undue reservation.
Ethics statement
The studies involving humans were approved by Jagiellonian University Bioethic Committee, Kraków, Poland. The studies were conducted in accordance with the local legislation and institutional requirements. The participants provided their written informed consent to participate in this study.
Author contributions
AC: Conceptualization, Data curation, Funding acquisition, Investigation, Project administration, Resources, Writing – original draft, Writing – review & editing. SB: Investigation, Writing – review & editing. DN: Investigation, Writing – review & editing. AŻ: Investigation, Writing – review & editing. AS: Formal analysis, Methodology, Visualization, Writing – review & editing. MF: Methodology, Supervision, Writing – review & editing. AB: Investigation, Writing – review & editing. TM: Methodology, Resources, Supervision, Writing – review & editing. DD: Methodology, Supervision, Writing – review & editing. MS: Methodology, Project administration, Supervision, Writing – review & editing.
Funding
The author(s) declare financial support was received for the research, authorship, and/or publication of this article. This research was funded as a research project being a part of the Preludium grant (2017/27/N/NZ4/00771) sponsored by the National Science Centre, Poland.
Conflict of interest
The authors declare that the research was conducted in the absence of any commercial or financial relationships that could be construed as a potential conflict of interest.
The author(s) declared that they were an editorial board member of Frontiers, at the time of submission. This had no impact on the peer review process and the final decision.
Publisher’s note
All claims expressed in this article are solely those of the authors and do not necessarily represent those of their affiliated organizations, or those of the publisher, the editors and the reviewers. Any product that may be evaluated in this article, or claim that may be made by its manufacturer, is not guaranteed or endorsed by the publisher.
References
1. Meyer F, Meyer TD. The misdiagnosis of bipolar disorder as a psychotic disorder: Some of its causes and their influence on therapy. J Affect Disord. (2009) 112:174–83. doi: 10.1016/J.JAD.2008.04.022
2. Bortolato B, Miskowiak KW, Köhler CA, Vieta E, Carvalho AF. Cognitive dysfunction in bipolar disorder and schizophrenia: A systematic review of meta-analyses. Neuropsychiatr Dis Treat. (2015) 11:3111–25. doi: 10.2147/NDT.S76700
3. Chrobak AA, Siuda-Krzywicka K, Soltys Z, Bielak S, Nowaczek D, Żyrkowska A, et al. When practice does not make a perfect - paradoxical learning curve in schizophrenia and bipolar disorder revealed by different serial reaction time task variants. Front Psychiatry. (2023) 14:1238473/BIBTEX. doi: 10.3389/FPSYT.2023.1238473/BIBTEX
4. Chrobak AA, Siwek GP, Siuda-Krzywicka K, Arciszewska A, Starowicz-Filip A, Siwek M, et al. Neurological and cerebellar soft signs do not discriminate schizophrenia from bipolar disorder patients. Prog Neuropsychopharmacol Biol Psychiatry. (2016) 64:96–101. doi: 10.1016/j.pnpbp.2015.07.009
5. Chrobak AA, Siuda-Krzywicka K, Sołtys Z, Siwek GP, Bohaterewicz B, Sobczak AM, et al. Relationship between neurological and cerebellar soft signs, and implicit motor learning in schizophrenia and bipolar disorder. Prog Neuropsychopharmacol Biol Psychiatry. (2021) 111:110137. doi: 10.1016/j.pnpbp.2020.110137
6. Dobri ML, Diaz AP, Selvaraj S, Quevedo J, Walss-Bass C, Soares JC, et al. The limits between schizophrenia and bipolar disorder: what do magnetic resonance findings tell us? Behav Sci. (2022) 12:78. doi: 10.3390/bs12030078
7. Hirschfeld RMA, Lewis L, Vornik LA. Perceptions and impact of bipolar disorder: How far have we really come? Results of the National Depressive and Manic-Depressive Association 2000 Survey of individuals with bipolar disorder. J Clin Psychiatry. (2003) 64:161–74. doi: 10.4088/JCP.v64n0209
8. Gonzalez-Pinto A, Gutierrez M, Mosquera F, Ballesteros J, Lopez P, Ezcurra J, et al. First episode in bipolar disorder: Misdiagnosis and psychotic symptoms. J Affect Disord. (1998) 50:41–4. doi: 10.1016/S0165-0327(98)00032-9
9. Bohaterewicz B, Sobczak AM, Podolak I, Wójcik B, Metel D, Chrobak AA, et al. Machine learning-based identification of suicidal risk in patients with schizophrenia using multi-level resting-state fMRI features. Front Neurosci. (2021) 14:605697. doi: 10.3389/fnins.2020.605697
10. Chrobak AA, Bohaterewicz B, Sobczak AM, Marszał-Wiśniewska M, Tereszko A, Krupa A, et al. Time-frequency characterization of resting brain in bipolar disorder during euthymia—a preliminary study. Brain Sci. (2021) 11:599. doi: 10.3390/brainsci11050599
11. Sobczak AM, Bohaterewicz B, Marek T, Fafrowicz M, Dudek D, Siwek M, et al. Altered functional connectivity differences in salience network as a neuromarker of suicide risk in euthymic bipolar disorder patients. Front Hum Neurosci. (2020) 14:585766. doi: 10.3389/fnhum.2020.585766
12. Ishida T, Nakamura Y, Tanaka SC, Mitsuyama Y, Yokoyama S, Shinzato H, et al. Aberrant large-scale network interactions across psychiatric disorders revealed by large-sample multi-site resting-state functional magnetic resonance imaging datasets. Schizophr Bull. (2023) 49:933–43. doi: 10.1093/schbul/sbad022
13. Keane BP, Krekelberg B, Mill RD, Silverstein SM, Thompson JL, Serody MR, et al. Dorsal attention network activity during perceptual organization is distinct in schizophrenia and predictive of cognitive disorganization. Eur J Neurosci. (2023) 57:458–78. doi: 10.1111/ejn.15889
14. Machner B, Braun L, Imholz J, Koch PJ, Münte TF, Helmchen C, et al. Resting-state functional connectivity in the dorsal attention network relates to behavioral performance in spatial attention tasks and may show task-related adaptation. Front Hum Neurosci. (2022) 15:757128. doi: 10.3389/fnhum.2021.757128
15. Lanssens A, Pizzamiglio G, Mantini D, Gillebert CR. Role of the dorsal attention network in distracter suppression based on features. Cognit Neurosci. (2020) 11:37-46. doi: 10.1080/17588928.2019.1683525
16. Hong LE, Tagamets M, Avila M, Wonodi I, Holcomb H, Thaker GK. Specific motion processing pathway deficit during eye tracking in schizophrenia: a performance-matched functional magnetic resonance imaging study. Biol Psychiatry. (2005) 57:726–32. doi: 10.1016/J.BIOPSYCH.2004.12.015
17. Tregellas JR, Tanabe JL, Miller DE, Ross RG, Olincy A, Freedman R. Neurobiology of smooth pursuit eye movement deficits in schizophrenia: an fMRI study. Am J Psychiatry. (2004) 161:315–21. doi: 10.1176/APPI.AJP.161.2.315
18. Keedy SK, Ebens CL, Keshavan MS, Sweeney JA. Functional magnetic resonance imaging studies of eye movements in first episode schizophrenia: smooth pursuit, visually guided saccades and the oculomotor delayed response task. Psychiatry Res. (2006) 146:199–211. doi: 10.1016/J.PSCYCHRESNS.2006.01.003
19. Brandt CL, Eichele T, Melle I, Sundet K, Server A, Agartz I, et al. Working memory networks and activation patterns in schizophrenia and bipolar disorder: Comparison with healthy controls. Br J Psychiatry. (2014) 204:290–8. doi: 10.1192/bjp.bp.113.129254
20. Huang AS, Rogers BP, Anticevic A, Blackford JU, Heckers S, Woodward ND. Brain function during stages of working memory in schizophrenia and psychotic bipolar disorder. Neuropsychopharmacology. (2019) 44:2136–42. doi: 10.1038/S41386-019-0434-4
21. Iglesias-Parro S, Soriano MF, Ibáñez-Molina AJ, Pérez-Matres AV, Ruiz de Miras J. Examining neural connectivity in schizophrenia using task-based EEG: A graph theory approach. Sensors (Basel). (2023) 23:8722. doi: 10.3390/S23218722/S1
22. Woodward ND, Rogers B, Heckers S. Functional resting-state networks are differentially affected in schizophrenia. Schizophr Res. (2011) 130:86–93. doi: 10.1016/j.schres.2011.03.010
23. Arkin SC, Ruiz-Betancourt D, Jamerson EC, Smith RT, Strauss NE, Klim CC, et al. Deficits and compensation: Attentional control cortical networks in schizophrenia. NeuroImage Clin. (2020) 27:102348. doi: 10.1016/J.NICL.2020.102348
24. Chrobak AA, Bohaterewicz B, Tereszko A, Krupa A, Sobczak A, Ceglarek A, et al. Altered functional connectivity among frontal eye fields, thalamus and cerebellum in bipolar disorder. Psychiatr Pol. (2019) 133:1–11. doi: 10.12740/pp/onlinefirst/104445
25. Xu K, Liu H, Li H, Tang Y, Womer F, Jiang X, et al. Amplitude of low-frequency fluctuations in bipolar disorder: A resting state fMRI study. J Affect Disord. (2014) 152–154:237–42. doi: 10.1016/J.JAD.2013.09.017
26. VanMeerten NJ, Dubke RE, Stanwyck JJ, Kang SS, Sponheim SR. Abnormal early brain responses during visual search are evident in schizophrenia but not bipolar affective disorder. Schizophr Res. (2016) 170:102–8. doi: 10.1016/j.schres.2015.11.007
27. Pokorny VJ, Espensen-Sturges TD, Burton PC, Sponheim SR, Olman CA. Aberrant cortical connectivity during ambiguous object recognition is associated with schizophrenia. Biol Psychiatry Cognit Neurosci Neuroimaging. (2021) 6:1193–201. doi: 10.1016/j.bpsc.2020.09.018
28. Mamah D, Barch DM, Repovš G. Resting state functional connectivity of five neural networks in bipolar disorder and schizophrenia. J Affect Disord. (2013) 150:601–9. doi: 10.1016/J.JAD.2013.01.051
29. Jimenez AM, Riedel P, Lee J, Reavis EA, Green MF. Linking resting-state networks and social cognition in schizophrenia and bipolar disorder. Hum Brain Mapp. (2019) 40:4703–15. doi: 10.1002/hbm.24731
30. Long Y, Liu Z, Chan CKY, Wu G, Xue Z, Pan Y, et al. Altered temporal variability of local and large-scale resting-state brain functional connectivity patterns in schizophrenia and bipolar disorder. Front Psychiatry. (2020) 11:422/FULL. doi: 10.3389/FPSYT.2020.00422/FULL
31. Öngür D, Lundy M, Greenhouse I, Shinn AK, Menon V, Cohen BM, et al. Default mode network abnormalities in bipolar disorder and schizophrenia. Psychiatry Res. (2010) 183:59–68. doi: 10.1016/J.PSCYCHRESNS.2010.04.008
32. Liu H, Tang Y, Womer F, Fan G, Lu T, Driesen N, et al. Differentiating patterns of amygdala-frontal functional connectivity in schizophrenia and bipolar disorder. Schizophr Bull. (2014) 40:469. doi: 10.1093/SCHBUL/SBT044
33. Khadka S, Meda SA, Stevens MC, Glahn DC, Calhoun VD, Sweeney JA, et al. Is aberrant functional connectivity A psychosis endophenotype? A resting state functional magnetic resonance imaging study. Biol Psychiatry. (2013) 74:458. doi: 10.1016/J.BIOPSYCH.2013.04.024
34. Reavis EA, Lee J, Altshuler LL, Cohen MS, Engel SA, Glahn DC, et al. Structural and functional connectivity of visual cortex in schizophrenia and bipolar disorder: A graph-theoretic analysis. Schizophr Bull Open. (2020) 1:sgaa056. doi: 10.1093/SCHIZBULLOPEN/SGAA056
35. Montgomery SA, Asberg M. A new depression scale designed to be sensitive to change. Br J Psychiatry. (1979) 134:382–9. doi: 10.1192/bjp.134.4.382
36. Young RC, Biggs JT, Ziegler VE, Meyer DA. A rating scale for mania: reliability, validity and sensitivity. Br J Psychiatry. (1979) 133:429–35. doi: 10.1192/bjp.133.5.429
37. Buchanan RW, Heinrichs DW. The Neurological Evaluation Scale (NES): a structured instrument for the assessment of neurological signs in schizophrenia. Psychiatry Res. (1989) 27:335–50. doi: 10.1016/0165-1781(89)90148-0
38. Sobczak AM, Bohaterewicz B, Ceglarek A, Zyrkowska A, Fafrowicz M, Slowik A, et al. Brain under fatigue - can perceived fatigability in multiple sclerosis be seen on the level of functional brain network architecture? Front Hum Neurosci. (2022) 16:852981. doi: 10.3389/FNHUM.2022.852981
39. Yan CG, Wang XD, Zuo XN, Zang YF. DPABI: data processing & Analysis for (Resting-state) brain imaging. Neuroinformatics. (2016) 14:339–51. doi: 10.1007/s12021-016-9299-4
40. Benjamini Y, Hochberg Y. Controlling the false discovery rate: A practical and powerful approach to multiple testing. J R Stat Soc Ser B Stat Methodol. (1995) 57:289–300. doi: 10.1111/j.2517-6161.1995.tb02031.x
41. Leucht S, Samara M, Heres S, Patel MX, Furukawa T, Cipriani A, et al. Dose equivalents for second-generation antipsychotic drugs: the classical mean dose method. Schizophr Bull. (2015) 41:1397–402. doi: 10.1093/schbul/sbv037
42. RCoreTeam. R: A language and environment for statistical computing. In: Vienna, Austria: R Foundation for Statistical Computing (2022).
43. Schlag J, Dassonville P, Schlag-Rey M. Interaction of the two frontal eye fields before saccade onset. J Neurophysiol. (1998) 79:64–72. doi: 10.1152/jn.1998.79.1.64
44. Chrobak AA, Rybakowski JK, Abramowicz M, Perdziak M, Gryncewicz W, Tereszko A, et al. Vergence eye movements in bipolar disorder. Psychiatr Pol. (2020) 54:467–85. doi: 10.12740/PP/ONLINEFIRST/105229
45. Carvalho N, Laurent E, Noiret N, Chopard G, Haffen E, Bennabi D, et al. Eye movement in unipolar and bipolar depression: A systematic review of the literature. Front Psychol. (2015) 6:1809. doi: 10.3389/fpsyg.2015.01809
46. Brady RO, Tandon N, Masters GA, Margolis A, Cohen BM, Keshavan M, et al. Differential brain network activity across mood states in bipolar disorder. J Affect Disord. (2017) 207:367–76. doi: 10.1016/j.jad.2016.09.041
47. McAfoose J, Baune BT. Exploring visual-spatial working memory: a critical review of concepts and models. Neuropsychol Rev. (2009) 19:130–42. doi: 10.1007/S11065-008-9063-0
48. Hillary FG, Grafman JH. Injured brains and adaptive networks: the benefits and costs of hyperconnectivity. Trends Cognit Sci. (2017) 21:385–401. doi: 10.1016/J.TICS.2017.03.003
49. Aswendt M, Hoehn M. Functional hyperconnectivity related to brain disease: maladaptive process or element of resilience? Neural Regener Res. (2023) 18:1489. doi: 10.4103/1673-5374.361541
50. Klöppel S, Gregory S, Scheller E, Minkova L, Razi A, Durr A, et al. Compensation in preclinical huntington’s disease: evidence from the track-on HD study. EBioMedicine. (2015) 2:1420–9. doi: 10.1016/J.EBIOM.2015.08.002
51. Colangeli S, Boccia M, Verde P, Guariglia P, Bianchini F, Piccardi L. Cognitive reserve in healthy aging and alzheimer’s disease: A meta-analysis of fMRI studies. Am J Alzheimers Dis Other Demen. (2016) 31:443–9. doi: 10.1177/1533317516653826
52. Moonen AJH, Weiss PH, Wiesing M, Weidner R, Fink GR, Reijnders JSAM, et al. An fMRI study into emotional processing in Parkinson’s disease: Does increased medial prefrontal activation compensate for striatal dysfunction? PloS One. (2017) 12:e0177085. doi: 10.1371/JOURNAL.PONE.0177085
53. Fabbro S, Piccolo D, Vescovi MC, Bagatto D, Tereshko Y, Belgrado E, et al. Resting-state functional-MRI in iNPH: can default mode and motor networks changes improve patient selection and outcome? Preliminary report. Fluids Barriers CNS. (2023) 20:7. doi: 10.1186/S12987-023-00407-6
54. Brier MR, Thomas JB, Snyder AZ, Wang L, Fagan AM, Benzinger T, et al. Unrecognized preclinical Alzheimer disease confounds rs-fcMRI studies of normal aging. Neurology. (2014) 83:1613–9. doi: 10.1212/WNL.0000000000000939/SUPPL_FILE/SUPPLEMENTAL_DATA.DOC
55. Yan H, Sun C, Wang S, Bai L. Stronger activation in widely distributed regions may not compensate for an ineffectively connected neural network when reading a second language. Lecture Notes Comput Sci (including Subseries Lecture Notes Artif Intell Lecture Notes Bioinformatics). (2017) 10654 LNAI:95–106. doi: 10.1007/978-3-319-70772-3_9
56. Filippi M, Basaia S, Canu E, Imperiale F, Meani A, Caso F, et al. Brain network connectivity differs in early-onset neurodegenerative dementia. Neurology. (2017) 89:1764. doi: 10.1212/WNL.0000000000004577
57. Driesen NR, McCarthy G, Bhagwagar Z, Bloch M, Calhoun V, D’Souza DC, et al. Relationship of resting brain hyperconnectivity and schizophrenia-like symptoms produced by the NMDA receptor antagonist ketamine in humans. Mol Psychiatry. (2013) 18:1199–204. doi: 10.1038/MP.2012.194
58. Harikumar A, Solovyeva KP, Misiura M, Iraji A, Plis SM, Pearlson GD, et al. Revisiting functional dysconnectivity: a review of three model frameworks in schizophrenia. Curr Neurol Neurosci Rep. (2023) 23:937–46. doi: 10.1007/S11910-023-01325-8
59. Mueller SG. Persistent Resting-State fMRI Hyperconnectivity as a Risk Factor for Alzheimer’s Disease After TBI (2020) . Available online at: https://apps.dtic.mil/sti/citations/AD1119836 (Accessed August 26, 2024).
60. Vlassenko AG, Vaishnavi SN, Couture L, Sacco D, Shannon BJ, Mach RH, et al. Spatial correlation between brain aerobic glycolysis and amyloid-β (Aβ) deposition. Proc Natl Acad Sci U.S.A. (2010) 107:17763–7. doi: 10.1073/PNAS.1010461107/SUPPL_FILE/PNAS.201010461SI.PDF
61. Yao W, Chen H, Luo C, Sheng X, Zhao H, Xu Y, et al. Hyperconnectivity of self-referential network as a predictive biomarker of the progression of alzheimer’s disease. J Alzheimers Dis. (2021) 80:577–90. doi: 10.3233/JAD-201376
62. Schechter I, Butler PD, Jalbrzikowski M, Pasternak R, Saperstein AM, Javitt DC. A new dimension of sensory dysfunction: stereopsis deficits in schizophrenia. Biol Psychiatry. (2006) 60:1282–4. doi: 10.1016/j.biopsych.2006.03.064
63. Sehatpour P, Dias EC, Butler PD, Revheim N, Guilfoyle DN, Foxe JJ, et al. Impaired visual object processing across an occipital-frontal-hippocampal brain network in schizophrenia: an integrated neuroimaging study. Arch Gen Psychiatry. (2010) 67:772–82. doi: 10.1001/ARCHGENPSYCHIATRY.2010.85
64. O’Donnell BF, Swearer JM, Smith LT, Nestor PG, Shenton ME, McCarley RW. Selective deficits in visual perception and recognition in schizophrenia. Biol Psychiatry. (2006) 153:687–92. doi: 10.1176/AJP.153.5.687
65. Hahn B, Robinson BM, Leonard CJ, Luck SJ, Gold JM. Posterior parietal cortex dysfunction is central to working memory storage and broad cognitive deficits in schizophrenia. J Neurosci. (2018) 38:8378–87. doi: 10.1523/JNEUROSCI.0913-18.2018
66. Wang K, Fan J, Dong Y, Wang CQ, Lee TMC, Posner MI. Selective impairment of attentional networks of orienting and executive control in schizophrenia. Schizophr Res. (2005) 78:235–41. doi: 10.1016/J.SCHRES.2005.01.019
67. Barekatain M, Haghighi M, Jahangard L, Ranjkesh F, Maracy MR. Covert orienting visual attention in full remitted single manic patients. J Res Med Sci. (2008) 13:189–95.
68. Marotta A, Chiaie RD, Spagna A, Bernabei L, Sciarretta M, Roca J, et al. Impaired conflict resolution and vigilance in euthymic bipolar disorder. Psychiatry Res. (2015) 229:490–6. doi: 10.1016/J.PSYCHRES.2015.06.026
Keywords: parietal cortex, frontal eye fields, resting state, functional networks, schizophrenia, bipolar disorder
Citation: Chrobak AA, Bielak S, Nowaczek D, Żyrkowska A, Sobczak AM, Fafrowicz M, Bryll A, Marek T, Dudek D and Siwek M (2024) Divergent pattern of functional connectivity within the dorsal attention network differentiates schizophrenia and bipolar disorder patients. Front. Psychiatry 15:1474313. doi: 10.3389/fpsyt.2024.1474313
Received: 01 August 2024; Accepted: 26 August 2024;
Published: 19 September 2024.
Edited by:
Kiyotaka Nemoto, University of Tsukuba, JapanReviewed by:
Daiki Sasabayashi, University of Toyama, JapanYuko Nakamura, The Center for Evolutionary Cognitive Sciences at the University of Tokyo, Japan
Copyright © 2024 Chrobak, Bielak, Nowaczek, Żyrkowska, Sobczak, Fafrowicz, Bryll, Marek, Dudek and Siwek. This is an open-access article distributed under the terms of the Creative Commons Attribution License (CC BY). The use, distribution or reproduction in other forums is permitted, provided the original author(s) and the copyright owner(s) are credited and that the original publication in this journal is cited, in accordance with accepted academic practice. No use, distribution or reproduction is permitted which does not comply with these terms.
*Correspondence: Marcin Siwek, bWFyY2luLnNpd2VrQHVqLmVkdS5wbA==