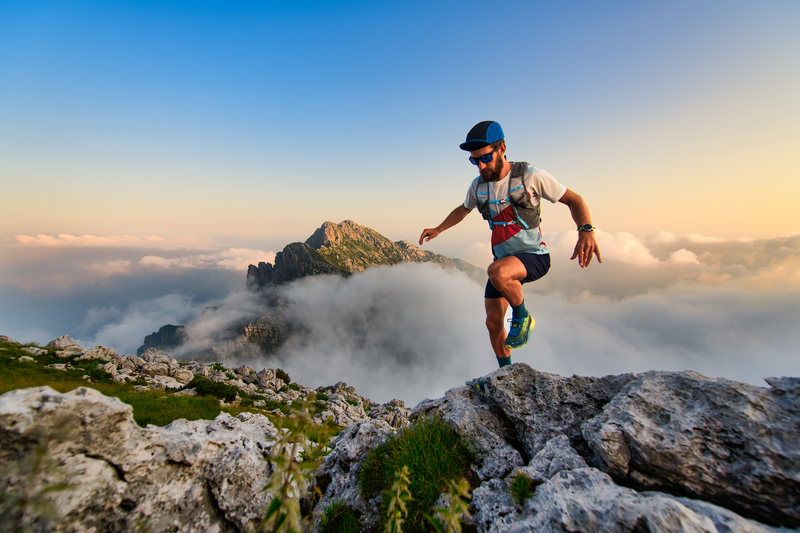
95% of researchers rate our articles as excellent or good
Learn more about the work of our research integrity team to safeguard the quality of each article we publish.
Find out more
ORIGINAL RESEARCH article
Front. Psychiatry , 25 October 2024
Sec. Anxiety and Stress Disorders
Volume 15 - 2024 | https://doi.org/10.3389/fpsyt.2024.1469372
Background: The mental health of patients with chronic liver disease (CLD) warrants greater attention and understanding, especially concerning its risk factors.
Method: Patients from our hospital’s hepatology clinic were consecutively enrolled and completed a questionnaire assessing anxiety, depression, and sleep quality using the GAD-7, PHQ-9, and PSQI scales, respectively. Reliability and validity were evaluated with Cronbach’s α and Kaiser-Meyer-Olkin (KMO). Continuous and categorical variables were analyzed using the Mann-Whitney U and Chi-square tests. Univariate and multivariate logistic regression were used to identify risk factors, while restricted cubic spline (RCS) were used to explored non-linear associations. Subgroup analyses were stratified by sex, age, and education.
Result: A total of 1030 questionnaires were collected, and after quality control, 1003 were included. 56.2% (564/1003), 53.2% (534/1003), and 67.4% (676/1003) individuals had anxiety, depression, and sleep disorders. Differences in age, gender, and education level were observed (P<0.05). Subgroup analysis showed similar demographic trends. Univariate and multivariate regression analysis found age negatively correlated with anxiety (OR=0.98, 95%CI=0.97-0.99, P=0.02) and depression (OR=0.98, 95%CI=0.96-0.99, P<0.001), but positively correlated with sleep disorders (OR=1.03, 95%CI= 1.01-1.05, P< 0.001); males are less prone to anxiety (OR=0.68, 95%CI=0.52-0.88, P=0.004) and sleep disorders (OR=0.72, 95%CI: 0.55-0.94, P=0.02); university degree is more susceptible to depression (OR=1.36, 95%CI=1.04-1.77, P=0.02) and anxiety (OR=1.45, 95%CI=1.11-1.89, P=0.007). RCS analysis suggested a linear relationship between the age and affective disorders among different population.
Conclusion: Young individuals, female, and those with higher education are more vulnerable to mental health, warranting increased attention.
Mental health, including anxiety, depression, and sleep disorders, encompass a wide range of conditions that affect mood, thinking, and behavior (1, 2). These conditions can occur as separate issues or may overlap, significantly impacting daily lives and well-being (3, 4). Recently, epidemiological studies showed that mental health disorders are on the rise globally. A study by Liu, Sitong et al. revealed that the anxiety and depression in Chinese residents was 19.7% and 45.02% respectively (5). During the COVID-19 pandemic, the rates of mental health symptoms among Chinese were 27.9% (95% CI, 27.5%-28.2%) for depression, 31.6% (95% CI, 31.2%-32.0%) for anxiety, and 29.2% (95% CI, 28.8%-29.6%) for insomnia (6). The World Health Organization (WHO) has highlighted mental health as a critical global issue, with millions of people affected each year, and the numbers are expected to increase (7, 8). The harm of mental health disorders extends beyond individual suffering but also impose economic burdens on healthcare systems and societies.
Chronic liver disease (CLD) is a major global health threat and has become a leading cause of human mortality. Despite advances in treatment and prevention, the incidence and prevalence of CLD continue to grow, posing challenges for healthcare systems globally (9, 10). The relationship between CLD and mental health disorders is complex and bidirectional.
Patients with CLD often experience psychological distress, which can significantly affect their quality of life and overall health outcomes (11). The stress of living with a chronic condition, alongside the social and economic impacts, contribute to the higher prevalence of mental health issues in CLD patients. Moreover, mental health disorders can negatively impact the management and progression of liver disease, creating a vicious circle. Qin et al. reported that 75% CLD patients had mental health problems, and education levels, course of disease, annual hospitalizations, complications, and nursing satisfaction levels were all independent risk factors for the mental health of patients with CLD (12). Duan et al. revealed that patients with hepatitis B virus (HBV)-related acute-on-chronic liver failure and cirrhosis are at a higher risk of depression, and concluded that lower education level, anxiety, poor sleep quality, and greater severity of disease were associated with elevated depression (13). Therefore, mental problems in CLD patients need urgent attention. Most previous studies were conducted on hospitalized patients. Outpatients, navigating their daily lives in a prolonged and chronic disease state, necessitate further exploration into the effects of long-term illness on mental health. Therefore, this study aimed to investigate the mental health status and explore risk factors of patients with CLD in outpatients.
The questionnaire was developed by psychologists, hepatologists and caregivers. All outpatients completed the anonymous questionnaire under the guidance of trained nursing staff. The diagnosis of CLD followed the clinical practice guidelines. 7-tiem generalized anxiety disorder scale (GAD-7), patient health questionnaire-9 (PHQ-9), and Pittsburgh sleep quality index (PSQI) were used to examine anxiety, depression, and sleep quality of patients, respectively.
Demographic data included sex, age, height, weight, education level, place of residence, smoking, drinking, diabetes, hypertension, chronic kidney disease, malignancy, disease duration, drug therapy or not, and drug use duration. BMI was calculated with formula BMI = weight (kg) divided by height (m2). GAD-7 scale is a simple self-rating scale commonly used in primary care settings (14). The scale consists of 7 items. Each entry has a score ranging from 0 to 4 points, depending on the severity of the disease. A total score greater than 4 is considered anxiety. The scale has also been verified in different populations and was proved to have a good efficacy (15, 16). PHQ-9 is a simple and effective self-rating scale for depressive disorder (17). It has good reliability and validity in assisting the diagnosis of depression and evaluating the severity of symptoms (18, 19). The scale consists of 9 items, each of which is scored from 0 to 3, with a score of more than 4 being considered depressed. PSQI contains a total of 15 entries (20). The scale is used to assess the sleep quality of the subjects for nearly 1 month and is based on 19 self-rating questions. The 19 self-rating questions are scored on a scale of 0 to 3, with a score of more than 5 indicating a sleep disorder. PSQI scale was proved to have a good diagnostic accuracy (21, 22).
The questionnaire was designed by psychologists, hepatologists and nursing staff collectively to ensure the rationality of the questionnaire design. The questionnaire was completed under the guidance of the trained nurses. During data processing stage, the outliers were identified, and internal logic judgment was made. For missing values, if a variable was more than 30% missing, multiple imputation was performed. Cronbach’s α was used to test reliability. Kaiser-Meyer-Olkin (KMO) and Bartlett test were used to perform validity analysis.
Continuous variables were presented as the mean ± standard deviation or median with the 25th and 75th percentiles. Categorical variables were presented as frequencies and percentages and. Shapiro-Wilk test was used to verify the normality of variable distribution. Mann-Whitney U tests was used for continuous variables, while the Chi square test was used for comparing categorical variables between different groups. Univariate and multivariate logistic regression analyses were used to evaluate the risk factors affecting mental health. Restricted cubic spline (RCS) was used to examine the potential non-linear associations. Analyses were completed in R (4.2.1), SPSS 27, and Sangerbox (23). P<0.05 was considered statistically significant.
In total, 1030 questionnaires were collected. Following quality control measures, 1003 questionnaires were suitable for inclusion in analysis. The study encompassed 888 individuals with hepatitis, 124 with advanced liver disease (cirrhosis, liver failure, and liver cancer), 54 with metabolically related liver diseases (such as alcoholic liver disease and non-alcoholic liver disease), 14 with autoimmune liver diseases, and 60 with other types of liver diseases. The reliability and validity of the questionnaire were robust, as demonstrated by Cronbach’s α scores of 0.895, 0.887, and 0.768 and the KMO values of 0.906, 0.916, and 0.852 for GAD-7, PHQ-9, and PSQI respectively. 564 out of 1003 individuals had anxiety, 534 out of 1003 had depression, and 676 out of 1003 had sleep disorders (Figure 1).
Figure 1. The disease distribution of patients in our study. MRLD, metabolic related liver disease; AIL, autoimmune liver disease.
We found that compared to the anxiety-free group, the anxious population differed in gender, age, educational level, hypertension, disease duration, depression, sleep disorders, GAD-7, PHQ-9, and PSQI score (Table 1). Subsequently, we conducted subgroup analyses for gender, age, and educational level separately, and we found that the demographic differences were essentially still present (Supplementary Tables 1, 4, 7). After univariate logistic regression, gender, age, educational level, and hypertension were found to be related to anxiety (Supplementary Table 10). Further multivariate logistic regression revealed that age (OR=0.98, 95% CI=0.97-0.99, P=0.02) and male (OR=0.68, 95%CI=0.52-0.88, P=0.004) were protective factors against anxiety. University education levels (OR=1.45, 95%CI=1.11-1.89, P=0.007) were associated with a higher risk of anxiety (Figure 2). RCS curve did not observe a nonlinear association between age and anxiety across different sex and education populations (Figures 3A, B).
Figure 2. Forest plot of different risk factors of mental disorder after multivariate logistic regression.
Figure 3. RCS analysis on the association between the age and mental disorder. (A, B) RCS curve of the association between the age and anxiety among sex and education. (C, D) RCS curve of the association between the age and Depression among sex and education. (E, F) RCS curve of the association between the age and sleep disorder among sex and education.
We found that compared to the depress-free group, the depressed population differed in gender, educational level, disease duration, drug therapy, anxiety, sleep disorder, GAD-7, PHQ-9, and PSQI score (Table 1). Subgroup analysis indicated that demographic disparities remained unchanged (Supplementary Tables 2, 5, 8). After univariate logistic regression, age and educational level were identified as factors associated with depression. Further multivariate logistic regression revealed that age (OR=0.98, 95%CI=0.96-0.99, P<0.001) was a protective factor against depression (Supplementary Table 10). University education levels (OR=1.36, 95%CI=1.04-1.77, P=0.02) were associated with a higher risk of depression (Figure 2). RCS curve did not observe nonlinear association between age and depression across different sex and education populations (Figures 3C, D).
We discovered that compared to the control group, the population with sleep disorders differed in gender, age, duration of illness, anxiety, depression, GAD-7, PHQ-9, and PSQI score (Table 1). Subgroup analyses demonstrated that the demographic characteristics remained unchanged (Supplementary Tables 3, 6, 9). After univariate logistic regression, gender and age were determined to be factors linked to sleep disorders (Supplementary Table 10). Further multivariate logistic regression revealed that age was associated with a higher risk of sleep disorders (OR=1.03, 95%CI: 1.01-1.05, P < 0.001), and male was less likely to have sleep disorders (OR=0.72, 95%CI: 0.55-0.94, P = 0.02) (Figure 2). RCS curve did not observe a nonlinear association between age and sleep disorder among sex and education populations (Figures 3E, F).
CLD is a major health issue, the long-term course of the disease can damage patients’ mental health. In CLD population, mental health issues are often overlooked, affecting the effectiveness of disease treatment, and prolonging the duration of the illness. In this study, we investigated the psychological condition of the CLD population through a questionnaire survey. We found that nearly half of the CLD patients suffer from anxiety, depression, and sleep disorders. After multivariate logistic regression, we found that age, gender, and educational level are independent risk factors for mental disorders. RCS analysis revealed that the relationship between age and mental disorders is not nonlinear in different populations.
The relationship between age and anxiety is complex. Some studies suggested that older people suffer from at least one chronic disease and face greater health issues than younger individuals (24). However, younger people also encountered more social pressures, including socializing, academics, work, and balancing family life (25, 26). Anxiety sensitivity and an intolerance of uncertainty may make both older and younger individuals more prone to anxiety (24). As for the relationship between age and depression, some research indicated that the incidence of depression decreases with age (27), while other reports have shown a U-shaped relationship between age and depression (28, 29). In our study, we found a negative correlation between age and both depression and anxiety, with younger individuals with CLD being more susceptible to anxiety and depression. Young people’s extensive internet access leads to more online health information seeking, potentially causing unnecessary anxiety. Older adults might have identified their health issues earlier but faced restricted treatment options and access, leading to extended periods without medication or regular check-ups. This reduced focus on disease progression could lower mental health concerns.
Studies reveal that the female population was more susceptible to depression, with a male to female ratio of 2:1 (30), but other studies suggested that there are no gender differences in the incidence of depression in children. A recent meta-analysis showed that gender differences in the incidence of depression peak during adolescence, but decline and stabilize after adulthood (31). Repeated reductions in estrogen can interfere with the ability of estrogen to neutralize the release of glucocorticoids when feeling stressed, making women more susceptible to stress and thus at risk of anxiety and depression (32, 33). Many traits of women, including changes occurring in the biological lifecycle such as menstruation, pregnancy, and menopause, increase the risk of sleep disorders (34). Sex hormones may play a role in sleep regulation and arousal (35, 36). Studies suggested that due to differences in brain chemicals and the stress response system, women may be physiologically more prone to anxiety disorders (37). For example, differences in the neurotransmitter serotonin are considered a factor in the higher incidence of anxiety disorders in women (38, 39).
Research showed that the education level was negatively correlated with mental health problems in CLD patients (40), and protective effects were higher among women (41). Some research found there was not protective role of education in mental health (42). In our study, we found that having a university education or higher increase the risk of anxiety and depression in patients with CLD. Their propensity for analytical thinking, perfectionism, and extensive mental activity of university education increased sleep disorder risk (43).
There are several limitations in our article. Firstly, our samples came from a single center, and multi-center data is needed to assess the relationship between CLD and mental disorders. Secondly, selection bias cannot be excluded Third, more socioeconomic and family support needs to be included in the questionnaire analysis. Fourth, the population included in our study was mostly viral hepatitis patients, which may misevaluate mental health in other liver diseases.
In summary, we revealed that at least half of the population with CLD suffer from mental disorders. Age, gender, and education level may be independent risk factors for mental illness. Given the high prevalence of mental illness among patients with CLD, the use of screening tools in clinic may identify those at greatest need of attention from a psychologist.
The raw data supporting the conclusions of this article will be made available by the authors, without undue reservation.
Ethical approval was not required for the study involving humans in accordance with the local legislation and institutional requirements.
JL: Writing – original draft. XP: Writing – original draft. WJ: Data curation, Formal analysis, Writing – original draft, Visualization, Methodology. XW: Data curation, Formal analysis, Methodology, Visualization, Writing – original draft. DW: Writing – review & editing. XT: Writing – review & editing. TZ: Writing – review & editing.
The author(s) declare financial support was received for the research, authorship, and/or publication of this article. This research was supported by key development projects of the Sichuan Provincial Science and Technology Plan (2024YFHZ0055).
The authors declare that the research was conducted in the absence of any commercial or financial relationships that could be construed as a potential conflict of interest.
All claims expressed in this article are solely those of the authors and do not necessarily represent those of their affiliated organizations, or those of the publisher, the editors and the reviewers. Any product that may be evaluated in this article, or claim that may be made by its manufacturer, is not guaranteed or endorsed by the publisher.
The Supplementary Material for this article can be found online at: https://www.frontiersin.org/articles/10.3389/fpsyt.2024.1469372/full#supplementary-material
2. Broome MR, Saunders KE, Harrison PJ, Marwaha S. Mood instability: significance, definition and measurement. Br J Psychiatry. (2015) 207:283–5.
3. Liang W, Chikritzhs T, Lenton S. Affective disorders and anxiety disorders predict the risk of drug harmful use and dependence. Addiction. (2011) 106:1126–34.
4. Uher R, Payne JL, Pavlova B, Perlis RH. Major depressive disorder in DSM-5: implications for clinical practice and research of changes from DSM-IV. Depress Anxiety. (2014) 31:459–71.
5. Liu S, Mei Y, Huang L, Liu X, Xi Y. Association of habitual physical activity with depression and anxiety: a multicentre cross-sectional study. BMJ Open. (2024) 14:e076095. doi: 10.1136/bmjopen-2023-076095
6. Shi L, Lu ZA, Que JY, Huang XL, Liu L, Ran MS, et al. Prevalence of and risk factors associated with mental health symptoms among the general population in China during the coronavirus disease 2019 pandemic. JAMA Netw Open. (2020) 3:e2014053. doi: 10.1001/jamanetworkopen.2020.14053
7. Liu Q, He H, Yang J, Feng X, Zhao F, Lyu J. Changes in the global burden of depression from 1990 to 2017: Findings from the Global Burden of Disease study. J Psychiatr Res. (2020) 126:134–40. doi: 10.1016/j.jpsychires.2019.08.002
8. Pitsillou E, Bresnehan SM, Kagarakis EA, Wijoyo SJ, Liang J, Hung A, et al. The cellular and molecular basis of major depressive disorder: towards a unified model for understanding clinical depression. Mol Biol Rep. (2020) 47:753–70. doi: 10.1007/s11033-019-05129-3
9. Younossi ZM. Non-alcoholic fatty liver disease - A global public health perspective. J Hepatol. (2019) 70:531–44.
10. Huang DQ, Terrault NA, Tacke F, Gluud LL, Arrese M, Bugianesi E, et al. Global epidemiology of cirrhosis - aetiology, trends and predictions. Nat Rev Gastroenterol Hepatol. (2023) 20:388–98.
11. Spiegel BM, Bolus R, Han S, Tong M, Esrailian E, Talley J, et al. Development and validation of a disease-targeted quality of life instrument in chronic hepatitis B: the hepatitis B quality of life instrument, version 1.0. Hepatology. (2007) 46:113–21. doi: 10.1002/hep.21692
12. Qin Z, Shen Y, Wu Y, Tang H, Zhang L. Analysis of risk factors for mental health problems of inpatients with chronic liver disease and nursing strategies: A single center descriptive study. Brain Behav. (2021) 11:e2406. doi: 10.1002/brb3.2406
13. Duan Z, Kong Y, Zhang J, Guo H. Psychological comorbidities in Chinese patients with acute-on-chronic liver failure. Gen Hosp Psychiatry. (2012) 34:276–81.
14. Kroenke K, Spitzer RL, Williams JB, Monahan PO, Löwe B. Anxiety disorders in primary care: prevalence, impairment, comorbidity, and detection. Ann Intern Med. (2007) 146:317–25.
15. Löwe B, Decker O, Müller S, Brähler E, Schellberg D, Herzog W, et al. Validation and standardization of the Generalized Anxiety Disorder Screener (GAD-7) in the general population. Med Care. (2008) 46:266–74.
16. Zhong QY, Gelaye B, Zaslavsky AM, Fann JR, Rondon MB, Sánchez SE, et al. Diagnostic validity of the generalized anxiety disorder - 7 (GAD-7) among pregnant women. PloS One. (2015) 10:e0125096. doi: 10.1371/journal.pone.0125096
17. Maurer DM, Raymond TJ, Davis BN. Depression: screening and diagnosis. Am Fam Physician. (2018) 98:508–15.
18. Lakkis NA, Mahmassani DM. Screening instruments for depression in primary care: a concise review for clinicians. Postgrad Med. (2015) 127:99–106. doi: 10.1080/00325481.2015.992721
19. Levis B, Benedetti A, Thombs BD. Accuracy of Patient Health Questionnaire-9 (PHQ-9) for screening to detect major depression: individual participant data meta-analysis. Bmj. (2019) 365:l1476. doi: 10.1136/bmj.l1476
20. Buysse DJ, Reynolds CF 3rd, Monk TH, Berman SR, Kupfer DJ. The Pittsburgh Sleep Quality Index: a new instrument for psychiatric practice and research. Psychiatry Res. (1989) 28:193–213. doi: 10.1016/0165-1781(89)90047-4
21. Omachi TA. Measures of sleep in rheumatologic diseases: Epworth Sleepiness Scale (ESS), Functional Outcome of Sleep Questionnaire (FOSQ), Insomnia Severity Index (ISI), and Pittsburgh Sleep Quality Index (PSQI). Arthritis Care Res (Hoboken). (2011) 63 Suppl 11:S287–296. doi: 10.1002/acr.20544
22. Chiu HY, Chang LY, Hsieh YJ, Tsai PS. A meta-analysis of diagnostic accuracy of three screening tools for insomnia. J Psychosom Res. (2016) 87:85–92.
23. Shen W, Song Z, Zhong X, Huang M, Shen D, Gao P, et al. Sangerbox: A comprehensive, interaction-friendly clinical bioinformatics analysis platform. Imeta. (2022) 1:e36. doi: 10.1002/imt2.36
24. Gerolimatos LA, Edelstein BA. Predictors of health anxiety among older and young adults. Int Psychogeriatr. (2012) 24:1998–2008.
25. Chronis-Tuscano A, Danko CM, Rubin KH, Coplan RJ, Novick DR. Future directions for research on early intervention for young children at risk for social anxiety. J Clin Child Adolesc Psychol. (2018) 47:655–67.
26. Seemi T, Sharif H, Sharif S, Naeem H, Naeem FUA, Fatima Z. Anxiety levels among school-going adolescents in peri-urban areas of Karachi, Pakistan. PloS One. (2023) 18:e0289967. doi: 10.1371/journal.pone.0289967
27. Wade TJ, Cairney J. Age and depression in a nationally representative sample of Canadians: a preliminary look at the National Population Health Survey. Can J Public Health. (1997) 88:297–302.
28. Wu Z, Schimmele CM, Chappell NL. Aging and late-life depression. J Aging Health. (2012) 24:3–28.
29. García-Blanco A, Monferrer A, Grimaldos J, Hervás D, Balanzá-Martínez V, Diago V, et al. A preliminary study to assess the impact of maternal age on stress-related variables in healthy nulliparous women. Psychoneuroendocrinology. (2017) 78:97–104.
30. Bromet E, Andrade LH, Hwang I, Sampson NA, Alonso J, De Girolamo G, et al. Cross-national epidemiology of DSM-IV major depressive episode. BMC Med. (2011) 9:90.
31. Salk RH, Hyde JS, Abramson LY. Gender differences in depression in representative national samples: Meta-analyses of diagnoses and symptoms. Psychol Bull. (2017) 143:783–822. doi: 10.1037/bul0000102
32. Solomon MB, Herman JP. Sex differences in psychopathology: of gonads, adrenals and mental illness. Physiol Behav. (2009) 97:250–8.
33. Oldehinkel AJ, Bouma EM. Sensitivity to the depressogenic effect of stress and HPA-axis reactivity in adolescence: a review of gender differences. Neurosci Biobehav Rev. (2011) 35:1757–70.
35. Stevenson JC, Chines A, Pan K, Ryan KA, Mirkin S. A pooled analysis of the effects of conjugated estrogens/bazedoxifene on lipid parameters in postmenopausal women from the selective estrogens, menopause, and response to therapy (SMART) trials. J Clin Endocrinol Metab. (2015) 100:2329–38. doi: 10.1210/jc.2014-2649
36. Gómez-Santos C, Saura CB, Lucas JA, Castell P, Madrid JA, Garaulet M. Menopause status is associated with circadian- and sleep-related alterations. Menopause. (2016) 23:682–90.
37. Rubinow DR, Schmidt PJ. Sex differences and the neurobiology of affective disorders. Neuropsychopharmacology. (2019) 44:111–28.
38. Dazzi F, Scicchitano C. Neurotransmitters: gender’s differences. Riv Psichiatr. (2014) 49:237–4.
39. Marcinkiewcz CA, Mazzone CM, D’agostino G, Halladay LR, Hardaway JA, Diberto JF, et al. Serotonin engages an anxiety and fear-promoting circuit in the extended amygdala. Nature. (2016) 537:97–101.
40. Sperandei S, Page A, Spittal MJ, Pirkis J. Low education and mental health among older adults: the mediating role of employment and income. Soc Psychiatry Psychiatr Epidemiol. (2023) 58:823–31.
41. Kondirolli F, Sunder N. Mental health effects of education. Health Econ. (2022) 31 Suppl 2:22–39.
42. Dahmann SC, Schnitzlein DD. No evidence for a protective effect of education on mental health. Soc Sci Med. (2019) 241:112584.
Keywords: chronic liver disease, mental health, epidemiology, risk factor, affective disorder
Citation: Long J, Pei X, Jiang W, Wang X, Wu D, Tang X and Zhou T (2024) Mental health disorder in chronic liver disease: a questionnaire survey. Front. Psychiatry 15:1469372. doi: 10.3389/fpsyt.2024.1469372
Received: 23 July 2024; Accepted: 02 October 2024;
Published: 25 October 2024.
Edited by:
Shinsuke Hidese, Teikyo University, JapanReviewed by:
Luca Steardo, University Magna Graecia of Catanzaro, ItalyCopyright © 2024 Long, Pei, Jiang, Wang, Wu, Tang and Zhou. This is an open-access article distributed under the terms of the Creative Commons Attribution License (CC BY). The use, distribution or reproduction in other forums is permitted, provided the original author(s) and the copyright owner(s) are credited and that the original publication in this journal is cited, in accordance with accepted academic practice. No use, distribution or reproduction is permitted which does not comply with these terms.
*Correspondence: Dongbo Wu, ZG9uZ2JvaHVheGlAc2N1LmVkdS5jbg==; Xiangdong Tang, MjM3MjU2NDYxM0BxcS5jb20=; Taoyou Zhou, bWFyc2hlbHp0eWh4QDE2My5jb20=
†These authors have contributed equally to this work
Disclaimer: All claims expressed in this article are solely those of the authors and do not necessarily represent those of their affiliated organizations, or those of the publisher, the editors and the reviewers. Any product that may be evaluated in this article or claim that may be made by its manufacturer is not guaranteed or endorsed by the publisher.
Research integrity at Frontiers
Learn more about the work of our research integrity team to safeguard the quality of each article we publish.