- 1AI for Health, Institute Patient-centered Digital Health, Bern University of Applied Sciences, Biel, Switzerland
- 2Department of Education, ICT and Learning, Østfold University College, Halden, Norway
- 3Norwegian Centre for E-health Research, University Hospital of North Norway, Tromsø, Norway
Introduction: Digital health interventions specifically those realized as chatbots are increasingly available for mental health. They include technologies based on artificial intelligence that assess user’s sentiment and emotions for the purpose of responding in an empathetic way, or for treatment purposes, e.g. for analyzing the expressed emotions and suggesting interventions.
Methods: In this paper, we study the ethical dimensions of integrating these technologies in chatbots for depression intervention using the digital ethics canvas and the DTx Risk Assessment Canvas.
Results: As result, we identified some specific risks associated with the integration of sentiment and emotion analysis methods into these systems related to the difficulty to recognize correctly the expressed sentiment or emotion from statements of individuals with depressive symptoms and the appropriate system reaction including risk detection. Depending on the realization of the sentiment or emotion analysis, which might be dictionary-based or machine-learning based, additional risks occur from biased training data or misinterpretations.
Discussion: While technology decisions during system development can be made carefully depending on the use case, other ethical risks cannot be prevented on a technical level, but by carefully integrating such chatbots into the care process allowing for supervision by health professionals. We conclude that a careful reflection is needed when integrating sentiment and emotion analysis into chatbots for depression intervention. Balancing risk factors is key to leveraging technology in mental health in a way that enhances, rather than diminishes, user autonomy and agency.
1 Introduction
The integration of artificial intelligence (AI) into mental health interventions, particularly in the treatment of depression, represents a significant advance in therapeutic practice in recent years (1). AI-powered chatbots are increasingly being used to provide cost-effective, scalable and accessible mental health services (2). These systems interact with users via text or voice, simulating human-human interaction paradigms. In terms of efficacy, first results reveal that “chatbot-delivered psychotherapy can be adopted in health care institutions as an alternative treatment for depression and anxiety” (3).
In the current landscape, chatbots for depression intervention are primarily used to provide immediate support, psychoeducation and cognitive behavioral therapy (CBT) (4, 5). Some chatbots have been proven to reduce depression and anxiety short-course interventions (6). Further, there is a trend to assess the effectiveness of non-specialized intelligent agents such as ChatGPT (7), Siri, or Cortana in answering questions related to mental health or even support in mental health problems (8). Recently, researchers started to compare ChatGPT with competencies of health professionals for diagnosing and treating patients with depressions (9).
A recent trend is the application of large language models (LLM) to realize natural language processing tasks. An LLM is a machine learning model that encodes complex patterns of language use derived from vast quantities of input texts (10). While some studies indicate that LLMs show promise in providing clinically accurate or good-quality responses (9, 11), other studies point to significant inconsistencies in models’ ability to provide mental health support or advice (12, 13) and their insufficient capacity to manage mental health risk scenarios safely (14). In this paper, the focus is on chatbots designed for depression intervention. However, assessing the ethical risks associated with using non-specialized systems for depression intervention is also highly relevant, especially in the current hype surrounding ChatGPT. Specifically, we are focusing on the ethical dimensions of integrating sentiment and emotion analysis into chatbots for depression intervention.
1.1 Approaches to sentiment and emotion analysis
Sentiment analysis is a method that assesses whether an author’s or user’s viewpoint toward a topic, product, or service is positive, negative, or neutral (15, 16). It can be considered at different levels: sentence level, document level and aspect level. The latter is most challenging since various aspects in a sentence may have different polarities and the aspects have to be identified together with related information on them.
Emotion detection goes beyond sentiment analysis by identifying specific human emotions (e.g., anger, joy, sadness). It helps determining an individual’s emotional/mental state precisely (17). Depending on the underlying emotion model, the emotions to be distinguished differ. Broadly, there are two groups of emotion models: Within the dimensional emotion model, emotions are represented based on three parameters: valence, arousal and power. In the categorical model, emotions are defined discretely (e.g. anger, sadness, fear) (15).
The analysis of sentiments or emotions can be realized using a lexicon-based approach, a machine learning-based approach, a deep learning-based approach, a transfer learning or hybrid approach (15). The different types of methods require different resources for their realization, e.g. word dictionaries or training data. An overview on these types of methods is provided as Supplementary Material.
There are different lexicons available for sentiment and emotion analysis that have been not specifically developed for the mental health context. Datasets for training originate often from social media. A comprehensive overview of resources for sentiment and emotion analysis in the healthcare domain is provided by Denecke (16). It can be recognized, that most resources are neither specifically from the mental health domain nor from the field of depression. Standard sentiment analysis models are typically trained on datasets that do not adequately represent individuals with mental health conditions. This can lead to biases and inaccuracies when these models are applied to this demographic. Only in 2023, some large language models were published specifically fine-tuned for the mental health domain (18, 19).
1.2 Use of sentiment and emotion analysis in mental health chatbots
The application of sentiment and emotion analysis within chatbots allows these systems to detect subtle cues in users’ text or speech that indicate emotional states (20). By analyzing these signals, chatbots can adapt their interactions in real time, providing responses that are empathetically aligned with the user’s emotional needs (21). Devaram’s paper emphasizes the crucial role of empathetic chatbots in mental health care, highlighting their capacity to analyze and respond to users’ emotional states (22). Sentiment or emotion analysis integrated into chatbots for depression could help in providing tailor-made treatments to patients. Extracted opinions or emotions can be used by therapeutic chatbots to suggest an appropriate treatment or to react accordingly (22).
Experiments studying the difference in perceived empathy with ChatGPT compared to neurologists have been conducted; their results indicated that ChatGPT may be superior to neurologists in providing empathy (23). Furthermore, text written by clinicians in collaboration with a chatbot are being perceived as more empathetic than humans (24). These and similar research raises concerns on ethical aspects and safety related to the use of sentiment and emotion analysis in chatbots for mental health in general and for depression in particular (16). Key issues include the accuracy of emotion recognition algorithms, the privacy and confidentiality of sensitive emotional data, and the potential consequences of misinterpreting users’ emotions. Detected emotions can be misused for non-medical purposes including profiling or to manipulate individuals (16). When applying machine learning algorithms to patient data for realizing medical sentiment analysis, multiple instances of biases can be induced which might impact on the clinical decision making.
There is some research available on ethical risks of emotion analysis technology without specific focus on the mental health domain (25). Wang et al. aggregated ethical considerations of using ChatGPT in healthcare (26). Coghlan et al. studied ethical issues with using chatbots in mental health (27). They based their research on the five key ethics principles non-maleficience, beneficience, respect for autonomy, justice, and explicability.
Mohammad (28) discussed several ethical concerns regarding the use of word–emotion association lexicons. One key point is that sentiment lexicons primarily capture implicit emotions—words are linked to sentiments or emotions, but this does not necessarily reflect their true meaning. Different socio-cultural groups interpret words in varied ways; when a lexicon consolidates a specific cultural perspective, it reinforces that interpretation, potentially leading to misleading impressions.
Straw (29) explored the ethical implications of emotion mining in medicine, particularly in the context of the ethical principles of beneficence (promoting the welfare of individuals) and non-maleficence (avoiding harm to individuals). These principles bring into focus critical questions of responsibility and accountability. For instance, the question if a sentiment analysis algorithm fails to detect a suicide risk, who bears the responsibility for the algorithm’s mistake. She also raises concerns related to the impact on equity and fairness. Emotion mining systems may perpetuate biases present in their training data, potentially leading to unfair or discriminatory outcomes for certain demographic groups. Emotion mining in medicine involves analyzing sensitive personal data, raising significant privacy concerns. There are risks of data breaches or misuse of intimate mental health information collected through these systems (29).
Skorburg and Friesen (30) found that using emotions recorded in clinical notes and their use for prediction without patient consent could be interpreted as privacy violation as this might reveal information, patients were not willing to disclose. Although these aspects might also be relevant for sentiment and emotion analysis in chatbots for depression intervention, none of the existing work specifically studied the ethical aspects for this application. Our work differs from the existing research by specifically considering the integration of sentiment and emotion analysis within chatbots for depression intervention. Expressing emotions and reacting empathetically is key of human interaction. Simulating empathy by chatbots or analyzing sentiments and emotions by technology comes along with ethical questions in particular in a mental health settings where systems interact with vulnerable users.
1.3 Contributions of this work
A key to reducing concerns regarding specific technology such as sentiment or emotion analysis in chatbots for depression intervention is to systematically assess possible risks. In light of these considerations, the aim of this study is to systematically capture and analyze the risks including ethical risks associated with the integration of sentiment and emotion analysis into chatbots for depression intervention. Given the vulnerability of individuals suffering from depressions and the critical nature of therapeutic interventions, this research aims to assess the potential harms these technologies may pose to users. By identifying and addressing these ethical concerns, the research aims to contribute to the responsible development and use of artificial intelligence in mental health care, ensuring that these innovations truly benefit those they aim to serve.
This paper presents an overview of the ethical aspects and risks associated with integrating sentiment and emotion analysis into a chatbot for depression. The main contributions are:
● a comprehensive assessment of potential risks associated with sentiment and emotion analysis within chatbots for depression by applying the DTx Risk Assessment Canvas (31),
● a thorough evaluation of the ethical risks by applying the Digital Ethics Canvas (32),
● demonstration of the feasibility of using these methods for assessing the risk of technologies to develop mitigation strategies, and
● mitigation strategies for the identified risks.
2 Methods
In this study, we focused on chatbots aimed at improving depression specifically. General mental health chatbots were not included unless they directly targeted depressive symptoms. Additionally, we are focusing on purely text-based chatbots for depression intervention, i.e. those that analyze emotions and sentiments expressed in written natural language and thereby exclude systems analyzing face and voice of users.
In this paper, depression interventions refer to chatbots specifically designed to target the symptoms of clinical depression, as reported in the supporting literature. These chatbots are typically grounded in evidence-based therapeutic approaches, such as Cognitive Behavioral Therapy (CBT), and aim to reduce the core symptoms of depression (i.e., persistent sadness, loss of interest, fatigue, and cognitive difficulties among others). Regarding the evaluation of these chatbots, researchers have typically employed a range of outcome measures to track users’ progress, including self-reported symptoms and engagement metrics.
We focused specifically on text-based chatbots and depression interventions because text-based chatbots are a new digital form of intervention that allow users to engage at their own pace, asynchronously and non-intrusively. This type of “interaction” can reduce the pressure some users face with real-time interactions, such as voice or video chats. Text-based chatbot interventions are easily accessible across devices, and require fewer technological resources. Text-based chatbots, as other text-based platforms can also provide a sense of anonymity, which has been shown to increase openness and self-disclosure, particularly in sensitive contexts like mental health. We recognize the potential value in expanding chatbot interventions to other modalities (e.g. voice user interface, avatars) and conditions, and this is something we plan to explore in future work. However, for the scope of this study, we focused on text-based interventions for depression due to their current practicality, and accessibility.
We focused on depression as it is one of the most prevalent mental health conditions globally (33) and often co-occurs with other mental health disorders, such as anxiety, Attention deficit hyperactivity disorder or trauma-related conditions. Effective depression interventions can have a significant impact on overall mental health, as alleviating depressive symptoms can also reduce symptoms of co-morbid conditions. While we acknowledge the importance of addressing a range of mental health issues, we chose to focus on depression due to its widespread impact and the substantial evidence supporting the effectiveness of text-based chatbot interventions for depressive symptoms.
We applied the Digital Ethics Canvas (32), and the DTx Risk Assessment Canvas (31) for collecting ethical and other risks aspects to be considered when integrating sentiment and emotion analysis into chatbots for depression intervention. The tools are described in the following.
2.1 Digital Ethics Canvas
The Digital Ethics Canvas (32) is a visual tool for assessing ethical risks from six ethical perspectives specific to the digital domain: beneficence, non-maleficence, privacy, fairness, sustainability and empowerment. By means of guiding questions, researchers and developers are supported in conducting a benefit-risk analysis of the technical solution. Depending on whether the canvas is used in the design or development phase or at use time, mitigation strategies can either be artifact-oriented or context-oriented. That is, the strategies could include changing the technological artifact (e.g., avoid collecting personal data that is not needed to reduce a privacy risk) or changing the usage context (e.g., ask users to provide a nickname rather than their actual name).
In our assessment, we decided to drop the perspective “Sustainability” that is foreseen in the Digital Ethics Canvas since this depends from the approach chosen to realize sentiment or emotion analysis within the chatbot. In the context of chatbots, sustainability refers to the long-term viability and impact of their use over time, including their impact on the environment, as well as on promoting healthy social and economic growth Kotlarsky et al. (34). For example, a chatbot might involve an assessment of the energy efficiency of the algorithm used for emotion analysis, or an ethical handling of the data. In this work, we are not considering a specific approach to sentiment or emotion analysis, but the technology for analyzing emotions and sentiments as such. Therefore, judging the sustainability is not possible.
2.2 DTx Risk Assessment Canvas
The potential unintended consequences or risks of using sentiment and emotion analysis in chatbots for depression intervention were assessed using the DTx (Digital Therapeutics) Risk Assessment Canvas (31). Designed for researchers, developers and practitioners, the DTx Risk Assessment Canvas allows to reflect on the potential negative consequences of a digital health solution. It is organized into 15 thematic blocks divided into three groups:
● DTx: Problem, purpose, technology aspects impacting on outcome, clinical evidence, privacy, underlying clinical model;
● Users of the DTx: Individuals using the DTx, behavior of the user, relations of the user;
● Impact of the DTx: Problematic use, relations to other interventions, contraindications, undesired impact, expected outcome, risks and limitations.
For each thematic block, a set of guiding questions is provided, which can form the basis for collecting information on the block (31).
2.3 Assessment procedure
The two authors considered sentiment and emotion analysis integrated into chatbots for depression intervention and reflected on the guiding questions for each thematic block provided by the Digital Ethics Canvas and the DTx Risk Assessment Canvas. Thoughts were collected individually in a Miro board. In an online meeting, the different aspects were discussed, descriptions were concretized and redundant aspects were removed. Reflexive thematic analysis was used to group the thematic blocks from both canvases under similar concepts, as shown in Figure 1. It can be seen that the information was grouped into four groups. Then, we searched for concrete examples in literature to support our reflections. For this, we used the literature included into a previously conducted review on chatbots for depression intervention, which was carried out following the PRISMA guidelines (Denecke et al., 2024)1. This literature review was conducted on January 8th 2024 and considered the libraries PubMed, ACM Digital Library, IEEExplore, PsychInfo, and CINAHL. We used keywords related to chatbots (chatbot, conversational agent, intelligence agent, virtual assistant) and keywords related to depression (depression, Depressive disorder, dysthymia, affective disorder, dysthymic disorder) for the search. 215 references were found; after duplicate removal and screening of title and abstract, 41 articles remained for full-text review. Additional 18 papers were rejected in that step, resulting in 23 articles included in the review. They refer to 15 chatbots for depression intervention.
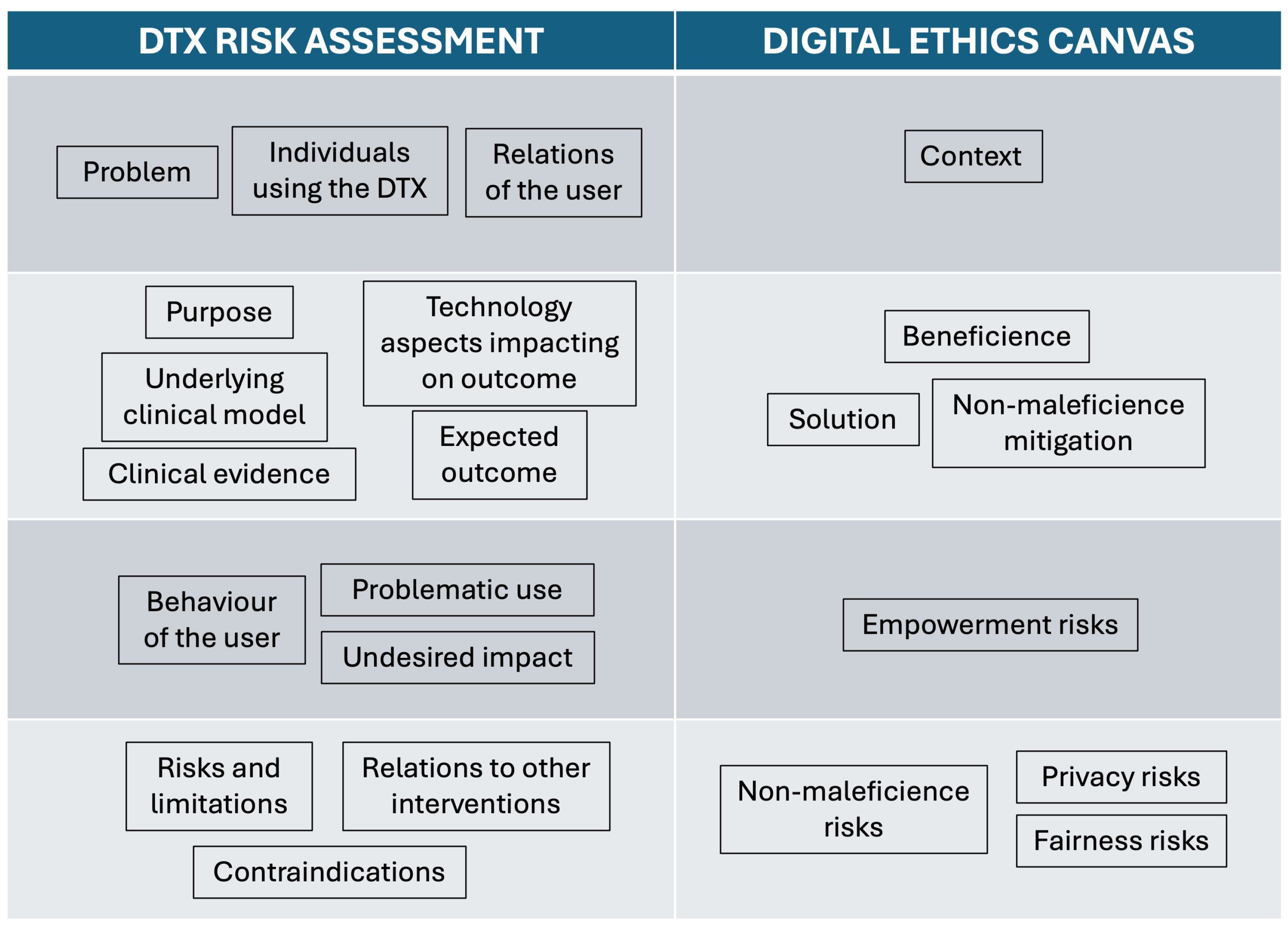
Figure 1. Grouping of thematic blocks from the DTx risk assessment canvas and the digital ethics canvas.
The reflections were based on the authors’ experiences in chatbots in mental health (35) and on their experiences in the development (36–39) and analysis (40–42) of chatbots in healthcare. One author has a background in psychology while the other author has a background in computer science and health informatics.
3 Results
In this section, we summarize the results grouped in the way that resulted from the reflexive thematic analysis. In section 4, we discuss possible mitigation strategies for the identified ethical and other risks. We first describe the information on the context, user and problem associated with chatbots for depression intervention in general with specific focus on sentiment and emotion analysis. Second, we summarize the purpose of these chatbots, technology aspects related to the outcome, and expected outcome of chatbots for depression intervention and specifically sentiment and emotion analysis. Third, we consider the user behavior and undesired impact followed by the risks and limitations of the technology.
3.1 Context, problem, individuals using DTx and relations of the user
Chatbots designed for depression interventions are typically used in two main contexts: either as tools for self-management or self-therapy, or as part of a therapeutic setting. In these scenarios, users may interact with the chatbot as a complement to working with a therapist or as an alternative to traditional therapy methods (43). Chatbots for depression intervention are designed to deliver self-help opportunities and in this way, they aim to mitigate real world problems such as the shortage of mental health staff, the time gap between therapy sessions, and fears of stigmatization. The target users of this technology include individuals diagnosed with clinical depression, but also those exhibiting depressive symptoms without a formal diagnosis, or individuals with anxiety or other disorders with depressive symptoms. Chatbots for depression interventions are designed to serve a diverse range of users across all genders and age groups, from children to younger and older adults - ideally tailored to one specific target group. Additionally, relatives and friends can also utilize the chatbot to support their loved ones.
3.2 Purpose, underlying clinical model, clinical evidence, technology aspects impacting on outcome, expected outcome, beneficience, solution
The purpose of chatbots for intervention in depression is to deliver therapy aimed at reducing depressive symptoms. They may also deliver only psychoeducational content or administer some form of co-regulation which may improve depression symptoms but are not necessarily directed therapy. To achieve this, the chatbots may include various therapeutic techniques (44–47), such as training, enablement, goal setting, self-assessment, monitoring symptoms, analyzing responses to treatment or intervention, cognitive restructuring, coping strategies, emotion regulation, environmental restructuring suggestions, psychoeducation, encouraging activities, or incentivization, among others. The chatbot should obtain a clear overview of the user’s emotional state and be capable of identifying emergencies, such as suicide risk or other situations of emotional risks. The underlying clinical model of these chatbots commonly reported in the literature is Cognitive Behavioral Therapy (CBT) as therapeutic model (37, 45, 48–52).
For realizing sentiment and emotion analysis, sentiment models (three classes, 5-item scale), and emotion models (45) are considered when designing the algorithms to be integrated in the chatbot. On the clinical evidence, while CBT efficacy has been extensively studied in therapeutic settings, there is very limited evidence of CBT delivered through chatbots (53). Nevertheless some research has shown that the use of chatbots - including those incorporating sentiment or emotion analysis - lead to a significant improvement of depression symptoms (43, 45, 52, 54, 55). Furthermore, our assessment highlights that there is no evidence on the impact of emotion analysis and empathy in chatbots on users. The efficacy of sentiment or emotion analysis integrated into these systems seems to have not yet been studied independently from other involved therapeutic techniques, making it difficult to judge the specific contribution of sentiment and emotion analysis alone.
The expected outcome of interacting with the chatbot is a reduction or elimination of depressive symptoms and related symptoms, prevention of relapse, and improved user knowledge about depression and coping strategies. In terms of technological aspects impacting outcomes, it is important to consider that emotions can be indirectly expressed by users through descriptions of situations and that a single statement can contain multiple sentiments and emotions, making an automatic analysis difficult. Chatbots for depression are mostly text-based and use various methods for emotion analysis, such as rule-based (43, 56), machine learning (37, 44, 45, 57, 58), deep learning (51), and transfer learning.
On the benefits of using chatbots for depression, our assessment indicates that it is important to highlight their anonymity, accessibility (easy access, ease of use, and availability 24/7) (44), their potential to benefit vulnerable residential populations (59) and the emotional support they can provide. Regarding the solution, the canvas evaluation suggests that chatbots can offer guidance and information, help users feel respected and less judged, allow for the monitoring of sentiments and emotions to recognize possible patterns, reduce feelings of isolation, and encourage openness about emotions without fear of criticism or misunderstandings. Additionally, the sentiment and emotion analysis methods integrated can help in tailoring content according to the user’s emotional state and identify crises by analyzing expressed emotions. This together with empathy shown towards the user can lead to a closer bond of trust between the user and the technology (47), increasing its acceptance.
3.3 Behavior of the user, problematic use, undesired impact, and empowerment risks
Regarding user behavior, we have identified that the user can ask the chatbot for tips to improve depressive symptoms, but also for other issues unrelated to depression (e.g., groceries), or even for disclosing crimes (27). User interactions can follow heterogeneous usage patterns (60), and high dropout rates have been reported (49, 54). On the problematic use, it is anticipated that the user may display self-harm behaviors that would require appropriate reaction. They may also provide only short statements open to interpretation leading to failure in the sentiment and emotion analysis. Users might not disclose their true emotions and sentiments to the chatbot, hiding or masking them. The adherence to the chatbot intervention can be low which is specifically problematic when this intervention is aimed at replacing face-to-face interaction with a therapist. Problematic use could also include the user preferring interaction with the bot due to more predictable emotional reactions, developing an addiction to the chatbot, or not interacting with it at all. On the undesired impact, we presume that there can be an over-reliance on the tool’s capacity and the risk of developing a false sense of security and trust in human-like chatbots because of their built-in empathy and emotional analysis, perceiving them as capable of providing the same level of care as human therapists (61). This may lead to users disclosing sensitive information and relying on the chatbot for depression intervention, which the chatbot may not be equipped to deal with effectively. On the other hand, increased dependency on the chatbot caused by the adaptation of the system to user preferences could increase isolation. As exemplified, a chatbot would most probably be always polite and will not be designed to show negative emotions towards its user. This could be perceived in a positive way by the user, becoming unwilling (or unable) to interact with humans and deal with the different behaviors of humans.
Empowerment risks may involve the user’s lack of interest in the chatbot due to depressive symptoms or non-interaction. Additional risks of empowerment could be associated with the lack of transparency in the communication of privacy policies (62), difficulties in understanding sentiment and emotion analysis technologies, the lack of transparency about the capabilities and limitations of emotion analysis, the lack of explicit consent for emotion analysis, and the user potentially developing a dependency on the empathy or emotional support provided by the chatbot.
3.4 Risks and limitations, relations to other interventions, contraindications, non-maleficience risks, privacy risks, and fairness risks
Risks and limitations of using chatbots for depression intervention and specifically integrating sentiment and emotion analysis identified by the canvas assessment include the potential for providing conflicting, incomplete, inaccurate, or incorrect information, lacking empathy, and missing context, especially when only analyzing text without sources of information such as voice patterns or facial expressions. Users could misinterpret the chatbot’s formulations, feel uncomfortable interacting with a machine, or prefer human interaction. Regarding contraindications and relation to other interventions, we have inferred that chatbots might be contraindicated for individuals at high risk of suicide and those in therapy settings that conflict with chatbot recommendations. Non-maleficence risks may include the chatbot misunderstanding the user, especially with irony, which can negatively affect the user’s mood. Chatbots may lack empathy, provide misinformation, or even give information on self-harm. Emotion analysis could be biased due to training data or dictionaries, and misunderstandings can arise from users’ impaired capacity to express emotions. Chatbots might overlook suicide and self-harm behavior, and the quality of emotion analysis might be low.
For many people with depression who feel isolated, interacting with chatbots might increase feelings of isolation, especially if the chatbot pretends to be a good friend who never expresses negative emotions. This can result in a loss of skills in handling criticism or misunderstandings related to emotions and sentiments. Privacy risks noted in the canvas assessment include the collection of personal and sensitive data, enabling emotional profiling, lack of encryption for conversations, and vulnerability to hacking, which could allow third-party access to data. Fairness risks could include ignoring cultural or educational differences in expressing emotions (63), missing context leading to unfair suggestions, and models trained on specific datasets not generalizing well to diverse populations with depression among others, resulting in inaccurate analysis. People who cannot express their emotions well might not achieve good results with the chatbot (64).
4 Discussion
4.1 Main findings
Despite their potential benefits regarding anonymity and accessibility (27), chatbots for depression intervention must navigate significant risks. The main findings from the canvas assessments highlight several significant general risks associated with the use of chatbots for depression intervention (e.g. user discomfort with machine interaction, missed detection of critical situations), but also risks that are specifically associated with the integration of sentiment and emotion analysis into these systems (e.g. misunderstandings of irony, bias in sentiment analysis). Primarily, these risks include the potential for chatbots to provide conflicting or inaccurate information due to low-quality emotion analysis due to technical limitations or limitations in contextual understanding which would be necessary for an appropriate interpretation of the expressed emotion. User misinterpretation of chatbot responses and discomfort with machine interaction were identified as risks, with particular concerns for those at high risk of suicide, for whom chatbots may not provide adequate support. The analysis also identified issues with bias in emotion recognition models due to unrepresentative training data, leading to inaccurate emotional assessments, particularly for users from different cultural or educational backgrounds.
In addition, the findings point to the risk of increased isolation due to over-reliance on chatbots. The risk of over-reliance could be considered for any chatbot, but it may increase when the chatbot becomes more human-like due to the integration of emotion and sentiment analysis. Research showed users who perceive chatbots as more human-like and conscious also report higher perceived social health benefits from interacting with them, suggesting a relationship between human-likeness and user engagement, where increased anthropomorphism may lead to stronger emotional attachments (65). There is a risk of user dependency on chatbots, highlighting the need for balance and integration with human support systems to ensure comprehensive mental health care. Further, privacy concerns around data collection are rather general risks of chatbots. However, the emotional profiling is specifically associated with the integration of sentiment and emotion analysis, increasing the risk of misusing this information. Table 1 summarizes the main risks and possible mitigation strategies that will be described in more detail in the following.
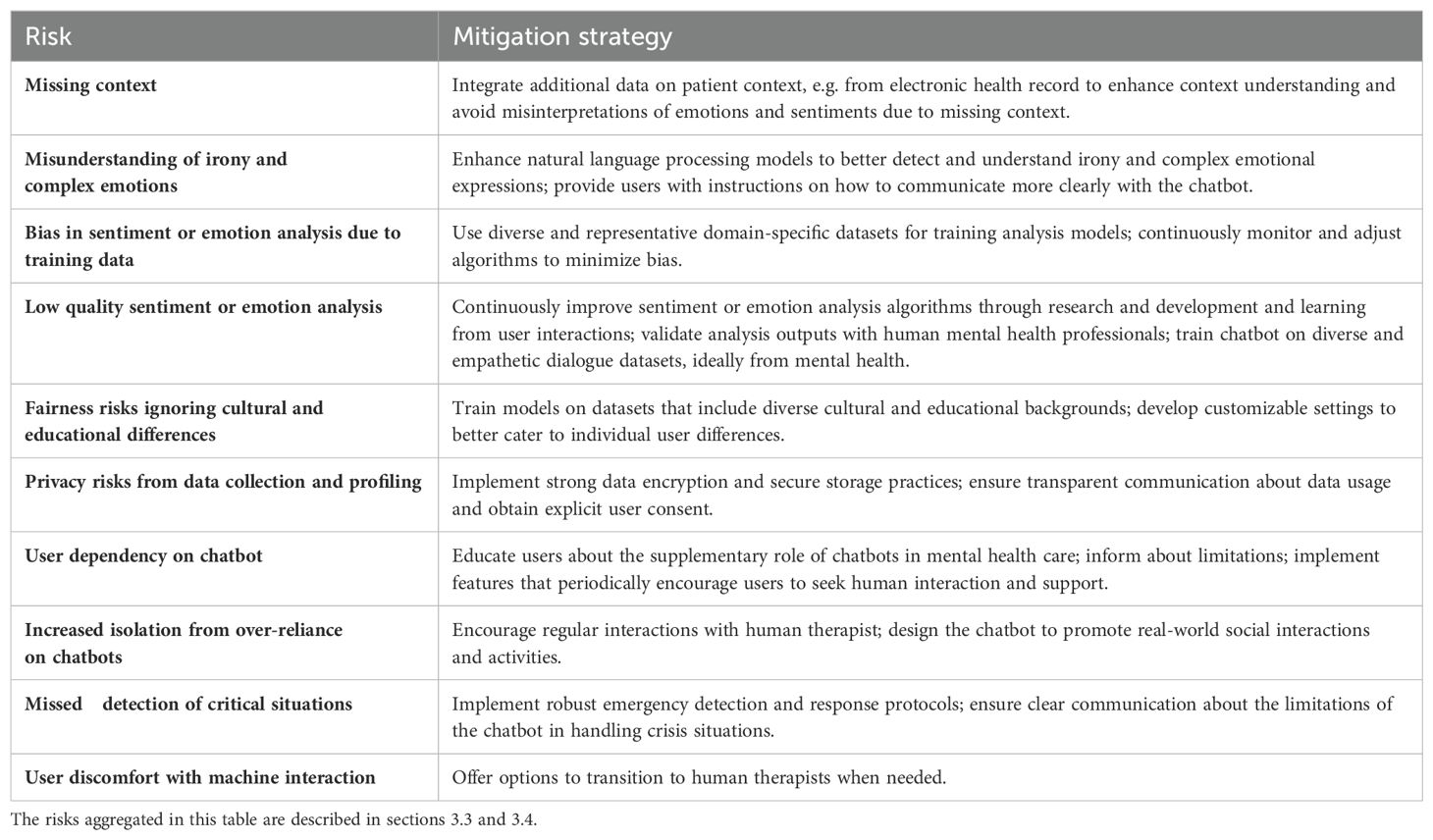
Table 1. Summary of risks associated with sentiment and emotion analysis in chatbots for depression and mitigation strategies.
4.2 Recommendations for mitigation of identified risks
Several risks could be addressed by embedding the chatbot use in a care setting. For example, mitigating user discomfort with interacting with a chatbot - although or because it is human-like - could be realized by offering the option to transit to a human therapist out of the chatbot dialogue. Additionally to mitigate potential harm (non-maleficence), it is suggested to employ human supervision (27). Chatbot developers should ensure that the chatbot provides sufficient benefits to compensate for potential harms (27). Given its criticality, we describe aspects related to risk assessment within chatbots for depression intervention in a separate subsection.
Depending on the implementation of the sentiment and emotion analysis, the associated risks differ. Lexicon-based approaches require dictionaries. Although in these dictionaries meanings of words are fixed allowing for a reproducible analysis, the general purpose sentiment and emotion dictionaries may ignore the expressions used by depressed individuals. Constant updates are needed to incorporate new linguistic patterns. Rule-based systems struggle with context and may misinterpret the sentiment if the user’s input does not match the predefined patterns, leading to inappropriate or harmful advice.
In case the sentiment and emotion analysis relies upon training data, the data might originate from other domains, may include biases and stereotypes (66, 67). Biased or unrepresentative data can lead to inaccurate sentiment analysis and inappropriate responses. Machine learning models are highly dependent on the quality and quantity of training data. It is thus important to use diverse and representative domain-specific datasets for training the sentiment and emotion analysis algorithms. Frequent system updates, learning from user interactions and the use of datasets from the mental health domain are also recommended. Careful assembly of the training data and human assessment of the results are essential.
The use of pre-trained models raises ethical concerns about the consent and privacy of the original data. Transferring this knowledge to sensitive areas such as mental health requires careful consideration. Further, the effectiveness of transferring general or different domain sentiment or emotion analysis models to the mental health domain learning can be inconsistent, depending on how well the pre-trained model’s knowledge applies to the new context, potentially leading to unpredictable or unreliable chatbot behavior.
There is evidence that empathy is an important element of any therapeutic relationship and that patients have moderately better outcomes in psychotherapy when they perceive therapists as understanding them (68). However, it is still unclear in which situations empathy may be particularly valuable or conversely contraindicated (68). The concrete effects of sentiment and emotion analysis integrated into chatbots for depression intervention still have to be assessed.
Providing users with information on the chatbot’s limitations and any associated risks is necessary (64) to avoid over-reliance and user dependency. Transparency about the technology involved in chatbots for depression intervention, including its limitations, is crucial. Developers should ensure mechanisms to get informed consent of users not only to the general use of the chatbot, but on the analysis of emotions and sentiments and the respective data collection and data use (27). This is because of the sensitive nature of emotion and sentiment information. In principle, users should be provided with detailed information about how the sentiment and emotion algorithms work, what they are used for, which data will be used, and the potential benefits, limitations, and risks associated with their use (69). Only when knowing how a system will process the data, users can decide what they are willing to disclose. Developers must rigorously test and improve the sentiment and emotion analysis methods to minimize errors and prioritize user safety and equity.
4.3 Addressing safety-critical situations
In our risk assessment, we have highlighted that chatbots for depression intervention should obtain a clear overview of the user’s emotional state and be capable of identifying emergencies, such as suicide risk. However, chatbots might overlook signs of suicide and self-harm behavior, making them potentially contraindicated for individuals at high risk of suicide. Chatbots for depression intervention should include features for emergency identification and maintain transparency about limitations to ensure safety and effectiveness. Ethical concerns also include the potential for chatbots to inadvertently encourage self-harm, as highlighted by cases where chatbots suggested suicide, emphasizing the need for rigorous guidelines and continuous monitoring. Specifically, it has been documented an incident where ChatGPT-3, when interacting with a simulated patient, suggested committing suicide (70). More alarmingly, it has also been reported a tragic real-world case where a user followed through with suicide after being encouraged by the chatbot Eliza (71). These cases underscore the urgent need for rigorous ethical guidelines and supervision in the development of chatbots. Ensuring that these digital tools do not exacerbate users’ mental health issues requires continuous monitoring, human oversight, and the implementation of robust safety protocols to prevent harmful interactions.
These examples demonstrate that chatbots such as ChatGPT, which are not specifically designed for mental health purposes, when misused as depression intervention tools, they can provide inconsistent or inappropriate responses that may exacerbate rather than alleviate users’ mental health conditions, and in this way risk patient safety. General-purpose chatbots lack the nuanced understanding and training that mental health professionals have, which could lead to misdiagnoses or harmful advice. Conversely, chatbots designed specifically for depression intervention also carry ethical risks. They may inadvertently reinforce negative thought patterns or fail to recognize severe symptoms that require immediate professional intervention. Relying on these chatbots could discourage individuals from seeking help from human professionals, leading to delayed treatment of serious conditions. In addition, both types of chatbots raise privacy concerns, as sensitive personal data could be mishandled or exploited, further compromising users’ well-being. For chatbots that are not specifically designed for depression intervention, this risk might be even higher. Therefore, while these technologies hold promise, the potential for harm requires strong ethical guidelines and robust oversight to ensure their safe and effective use. Health systems must ensure through regulation that chatbots maintain patient safety and equity in care, requiring robust experimental work for diverse patient populations (69).
4.4 Limitations of this work
This study acknowledges several limitations that must be considered. The digital risks and ethics analyses were conducted by only two individuals. This limited scope may have led to the dismissal of potential risks and the failure to identify new risks that could emerge as technologies evolve. To mitigate this, future assessments should be conducted with a panel of experts and chatbot users to ensure a comprehensive evaluation. We were focusing on purely text-based chatbots. There might be different and additional risks associated with sentiment and emotion analysis when analyzing voice or facial expressions of the user (22). Each chatbot is unique and the individual differences in people with depression present significant variability too. Therefore, each case should undergo specific risks and ethics assessments tailored to the particular chatbot and individual circumstances. We recommend to apply the two tools that were used in this work when developing chatbots for depression intervention. They will help to reflect on the risks and integrate mitigation strategies right from the beginning. Furthermore, although there is a growing body of literature on the development and testing of chatbots for depression interventions, there is a gap in the discussion regarding the risks and ethical implications of such applications. Our study underscores the necessity for more in-depth exploration and dialogue on these critical aspects to ensure the responsible and safe use of chatbots in mental health care.
Therapeutic interventions involve structured, and evidence-based approaches aimed at treating disorders; while interventions offering support or psycho-educational approaches only, although being a type of intervention and providing useful support and encouragement, are typically not considered as therapeutic interventions. In our assessment, we considered publications on chatbots designed as interventions for depression and did not differentiate between therapeutic and non-therapeutic chatbots, as our goal was to provide a broad overview on the ethical dimensions of integrating sentiment and emotion analysis in chatbots for depression intervention. We identified literature undermining our reflections from a recently conducted review on chatbots for depression (Denecke et al., 2024)1. This procedure may have overlooked aspects. However, since the review was following the PRISMA guidelines, we are confident that it provides an unbiased view on the current landscape of chatbots for depression.
5 Conclusions
In this paper, we assessed the risks associated with sentiment and emotion analysis in chatbots for depression intervention using two assessment tools. We identified several risks that can be grouped into risks related to the capabilities of a chatbot in general, risks associated to the quality of sentiment and emotion analysis, risks associated with the data collection and processing and risks associated with the user behavior. By describing these risks together with possible mitigation strategies, our study aims to raise awareness of the ethical dimensions inherent in decision-making processes during the development of mental health chatbots, and to stimulate discussion within the field. Ethical considerations surrounding the integration of sentiment and emotion analysis into these chatbots have not been sufficiently addressed by the research community. This oversight occurs despite the unique challenges and risks to patient safety posed by these technologies. Consequently, our work is dedicated to elucidating the significance and consequences of ethical and risk factors the integration of sentiment and emotion analysis in chatbots for depression.
In summary, while chatbots with integrated sentiment and emotion analysis can enhance user autonomy and agency by providing personalized, accessible, and confidential support, it is crucial to address potential drawbacks such as dependency, privacy concerns, and the risk of misinterpretation. Balancing these factors is key to leveraging technology in mental health in a way that enhances, rather than diminishes, user autonomy and agency. Further research is needed to disentangle these effects and evaluate how much of the observed benefits are directly attributable to sentiment and emotion analysis versus other components of the chatbot’s design and functionality.
Data availability statement
The original contributions presented in the study are included in the article/Supplementary Material. Further inquiries can be directed to the corresponding author.
Author contributions
KD: Conceptualization, Data curation, Formal analysis, Methodology, Visualization, Writing – original draft, Writing – review & editing. EG: Data curation, Formal analysis, Investigation, Visualization, Writing – original draft, Writing – review & editing.
Funding
The author(s) declare that no financial support was received for the research, authorship, and/or publication of this article.
Acknowledgments
We acknowledge the use of ChatGPT 3.5 for checking grammar for some paragraphs in the paper.
Conflict of interest
The authors declare that the research was conducted in the absence of any commercial or financial relationships that could be construed as a potential conflict of interest.
Publisher’s note
All claims expressed in this article are solely those of the authors and do not necessarily represent those of their affiliated organizations, or those of the publisher, the editors and the reviewers. Any product that may be evaluated in this article, or claim that may be made by its manufacturer, is not guaranteed or endorsed by the publisher.
Supplementary material
The Supplementary Material for this article can be found online at: https://www.frontiersin.org/articles/10.3389/fpsyt.2024.1462083/full#supplementary-material
Footnotes
- ^ Denecke K, Rivera Romero O, Wynn R, Gabarron E. Persuasive chatbot-based interventions for depression: A list of recommendations for improving reporting standards. Front Psychiatry. (2024). Under review.
References
1. Boucher EM, Harake NR, Ward HE, Stoeckl SE, Vargas J, Minkel J, et al. Artificially intelligent chatbots in digital mental health interventions: a review. Expert Rev Med Devices. (2021) 18:37–49. doi: 10.1080/17434440.2021.2013200
2. Vaidyam AN, Wisniewski H, Halamka JD, Kashavan MS, Torous JB. Chatbots and conversational agents in mental health: a review of the psychiatric landscape. Can J Psychiatry. (2019) 64:456–64. doi: 10.1177/0706743719828977
3. Lim SM, Shiau CWC, Cheng LJ, Lau Y. Chatbot-delivered psychotherapy for adults with depressive and anxiety symptoms: a systematic review and meta-regression. Behav Ther. (2022) 53:334–47. doi: 10.1016/j.beth.2021.09.007
4. Ahmed A, Hassan A, Aziz S, Abd-Alrazaq AA, Ali N, Alzubaidi M, et al. Chatbot features for anxiety and depression: a scoping review. Health Inf J. (2023) 29:14604582221146719. doi: 10.1177/14604582221146719
5. Martinengo L, Lum E, Car J. Evaluation of chatbot-delivered interventions for selfmanagement of depression: Content analysis. J Affect Disord. (2022) 319:598–607. doi: 10.1016/j.jad.2022.09.028
6. Zhong W, Luo J, Zhang H. The therapeutic effectiveness of artificial intelligence-based chatbots in alleviation of depressive and anxiety symptoms in short-course treatments: A systematic review and meta-analysis. J Affect Disord. (2024) 356:459–69. doi: 10.1016/j.jad.2024.04.057
7. Alanezi F. Assessing the effectiveness of chatgpt in delivering mental health support: a qualitative study. J Multidiscip Healthcare. (2024) 17:461–71. doi: 10.2147/JMDH.S447368
8. Miner AS, Milstein A, Schueller S, Hegde R, Mangurian C, Linos E. Smartphone-based conversational agents and responses to questions about mental health, interpersonal violence, and physical health. JAMA Internal Med. (2016) 176:619–25. doi: 10.1001/jamainternmed.2016.0400
9. Levkovich I, Elyoseph Z. Identifying depression and its determinants upon initiating treatment: Chatgpt versus primary care physicians. Family Med Community Health. (2023) 11(4):e002391. doi: 10.1136/fmch-2023-002391
10. Yang R, Tan TF, Lu W, Thirunavukarasu AJ, Ting DSW, Liu N. Large language models in health care: Development, applications, and challenges. Health Care Sci. (2023) 2:255–63. doi: 10.1002/hcs2.v2.4
11. Sezgin E, Chekeni F, Lee J, Keim S. Clinical accuracy of large language models and google search responses to postpartum depression questions: cross-sectional study. J Med Internet Res. (2023) 25:e49240. doi: 10.2196/49240
12. Farhat F. Chatgpt as a complementary mental health resource: a boon or a bane. Ann Biomed Eng. (2023) 52(5):1111–4. doi: 10.20944/preprints202307.1479.v1
13. Dergaa I, Fekih-Romdhane F, Hallit S, Loch AA, Glenn JM, Fessi MS, et al. Chatgpt is not ready yet for use in providing mental health assessment and interventions. Front Psychiatry. (2024) 14:1277756. doi: 10.3389/fpsyt.2023.1277756
14. Heston TF. Safety of large language models in addressing depression. Cureus. (2023) 15(12):e50729. doi: 10.7759/cureus.50729
15. Nandwani P, Verma R. A review on sentiment analysis and emotion detection from text. Soc network Anal Min. (2021) 11:81. doi: 10.1007/s13278-021-00776-6
17. Benrouba F, Boudour R. Emotional sentiment analysis of social media content for mental health safety. Soc Network Anal Min. (2023) 13:17. doi: 10.1007/s13278-022-01000-9
18. Xu X, Yao B, Dong Y, Gabriel S, Yu H, Hendler J, et al. Mental-llm: Leveraging large language models for mental health prediction via online text data. Arxiv (2023). doi: 10.1145/3643540
19. Yang K, Zhang T, Kuang Z, Xie Q, Ananiadou S. Mentalllama: Interpretable mental health analysis on social media with large language models. arXiv preprint arXiv:2309.13567. (2023). doi: 10.48550/arXiv.2309.13567
20. Chin H, Song H, Baek G, Shin M, Jung C, Cha M, et al. The potential of chatbots for emotional support and promoting mental well-being in different cultures: Mixed methods study. J Med Internet Res. (2023) 25:e51712. doi: 10.2196/51712
21. Sohara Banu A, Nagaveni V, Gowda NC, Yerriswamy T, Gururaj M, Varshitha N. (2023). Well bot—a reinforcement learning and sentiment analysis-based chatbot implementation for mental well-being, in: Reddy V.S., Wang J., Reddy K. (eds) Soft Computing and Signal Processing. ICSCSP 2023. Lecture Notes in Networks and Systems, Springer, Singapore. 864, pp. 471–81. doi: https://doi.org/10.1007/978-981-99-8628-6_40
22. Devaram S. Empathic chatbot: Emotional intelligence for mental health well-being. ArXiv abs/2012.09130. (2020). doi: 10.48550/arXiv.2012.09130
23. Maida E, Moccia M, Palladino R, Borriello G, Affinito G, Clerico M, et al. Chatgpt vs. neurologists: a cross-sectional study investigating preference, satisfaction ratings and perceived empathy in responses among people living with multiple sclerosis. J Neurol. (2024) 271(7):4057–66. doi: 10.1007/s00415-024-12328-x
24. Sharma A, Lin IW, Miner AS, Atkins DC, Althoff T. Human–ai collaboration enables more empathic conversations in text-based peer-to-peer mental health support. Nat Mach Intell. (2023) 5:46–57. doi: 10.1038/s42256-022-00593-2
25. Katirai A. Ethical considerations in emotion recognition technologies: a review of the literature. AI Ethics. (2023), 1–22. doi: 10.1007/s43681-023-00307-3
26. Wang C, Liu S, Yang H, Guo J, Wu Y, Liu J. Ethical considerations of using chatgpt in health care. J Med Internet Res. (2023) 25:e48009. doi: 10.2196/48009
27. Coghlan S, Leins K, Sheldrick S, Cheong M, Gooding P, D’Alfonso S. To chat or bot to chat: Ethical issues with using chatbots in mental health. Digital Health. (2023) 9:20552076231183542. doi: 10.1177/20552076231183542
28. Mohammad SM. Practical and ethical considerations in the effective use of emotion and sentiment lexicons. arXiv preprint arXiv:2011.03492. (2020). doi: 10.48550/arXiv.2011.03492
29. Straw I. Ethical implications of emotion mining in medicine. Health Policy Technol. (2021) 10:191–5. doi: 10.1016/j.hlpt.2020.11.006
31. Denecke K, May R, Gabarron E, Lopez-Campos GH. Assessing the potential risks of digital therapeutics (dtx): the dtx risk assessment canvas. J Personalized Med. (2023) 13:1523. doi: 10.3390/jpm13101523
32. Hardebolle C, Macko V, Ramachandran V, Holzer A, Jermann P. Digital ethics canvas: A guide for ethical risk assessment and mitigation in the digital domain. (2023). doi: 10.21427/9WA5-ZY95
34. Kotlarsky J, Oshri I, Sekulic N. Digital sustainability in information systems research: Conceptual foundations and future directions. J Assoc Inf Syst. (2023) 24:936–52. doi: 10.17705/1jais.00825
35. Giunti G, Isomursu M, Gabarron E, Solad Y. Designing depression screening chatbots. Stud Health Technol Inf. (2021) 284:259–63. doi: 10.3233/SHTI210719
36. May R, Denecke K. Extending patient education with claire: An interactive virtual reality and voice user interface application. EC-TEL. (2020), 482–6. doi: 10.1007/978-3-030-57717-9_49
37. Denecke K, Vaaheesan S, Arulnathan A. A mental health chatbot for regulating emotions (sermo)-concept and usability test. IEEE Trans Emerging Topics Computing. (2020) 9:1170–82. doi: 10.1109/TETC.2020.2974478
38. Denecke K, Lutz-Hochreutener S, Pöpel A, May R. Self-anamnesis with a conversational user interface: Concept and usability study. Methods Inf Med. (2018) 57:243–52. doi: 10.1055/s-0038-1675822
39. Denecke K, May R, Deng Y. Towards emotion-sensitive conversational user interfaces in healthcare applications. Stud Health Technol Inf. (2019) 264:1164–8. doi: 10.3233/SHTI190409
40. Denecke K, May R. Investigating conversational agents in healthcare: Application of a technical-oriented taxonomy. Proc Comput Sci. (2023) 219:1289–96. doi: 10.1016/j.procs.2023.01.413
41. Denecke K, Cihoric N, Reichenpfader D. Designing a digital medical interview assistant for radiology. Stud Health Technol Inf. (2023) 301:60–6. doi: 10.3233/SHTI230012
42. Denecke K. Framework for guiding the development of high-quality conversational agents in healthcare. Healthcare (MDPI). (2023) 11:1061. doi: 10.3390/healthcare11081061
43. Liu H, Peng H, Song X, Xu C, Zhang M. Using ai chatbots to provide self-help depression interventions for university students: A randomized trial of effectiveness. Internet Interventions. (2022) 27:100495. doi: 10.1016/j.invent.2022.100495
44. Rani K, Vishnoi H, Mishra M. (2023). A mental health chatbot delivering cognitive behavior therapy and remote health monitoring using nlp and ai, in: 2023 International Conference on Disruptive Technologies (ICDT). 313–7. doi: 10.1109/ICDT57929.2023.10150665
45. Inkster B, Sarda S, Subramanian V. An empathy-driven, conversational artificial intelligence agent (wysa) for digital mental well-being: real-world data evaluation mixed-methods study. JMIR mHealth uHealth. (2018) 6:e12106. doi: 10.2196/12106
46. Dosovitsky G, Pineda BS, Jacobson NC, Chang C, Escoredo M, Bunge EL. Artificial intelligence chatbot for depression: descriptive study of usage. JMIR Formative Res. (2020) 4:e17065. doi: 10.2196/17065
47. Beatty C, Malik T, Meheli S, Sinha C. Evaluating the therapeutic alliance with a free-text cbt conversational agent (wysa): a mixed-methods study. Front Digital Health. (2022) 4:847991. doi: 10.3389/fdgth.2022.847991
48. Kop R, Hoogendoorn M, Klein MC. (2014). A personalized support agent for depressed patients: Forecasting patient behavior using a mood and coping model, in: 2014 IEEE/WIC/ACM International Joint Conferences on Web Intelligence (WI) and Intelligent Agent Technologies (IAT), Warsaw, Poland. 302–309. doi: 10.1109/WI-IAT.2014.181
49. Klos MC, Escoredo M, Joerin A, Lemos VN, Rauws M, Bunge EL. Artificial intelligence–based chatbot for anxiety and depression in university students: pilot randomized controlled trial. JMIR formative Res. (2021) 5:e20678. doi: 10.2196/20678
50. Nicol G, Wang R, Graham S, Dodd S, Garbutt J. Chatbot-delivered cognitive behavioral therapy in adolescents with depression and anxiety during the covid-19 pandemic: feasibility and acceptability study. JMIR Formative Res. (2022) 6:e40242. doi: 10.2196/40242
51. Patel F, Thakore R, Nandwani I, Bharti SK. (2019). Combating depression in students using an intelligent chatbot: a cognitive behavioral therapy, in: 2019 IEEE 16th India Council International Conference (INDICON) Rajkot, India. 1–4. doi: 10.1109/INDICON47234.2019.9030346
52. Fitzpatrick KK, Darcy A, Vierhile M. Delivering cognitive behavior therapy to young adults with symptoms of depression and anxiety using a fully automated conversational agent (woebot): a randomized controlled trial. JMIR Ment Health. (2017) 4:e7785. doi: 10.2196/mental.7785
53. He Y, Yang L, Zhu X, Wu B, Zhang S, Qian C, et al. Mental health chatbot for young adults with depressive symptoms during the covid-19 pandemic: single-blind, three-arm randomized controlled trial. J Med Internet Res. (2022) 24:e40719. doi: 10.2196/40719
54. Leo AJ, Schuelke MJ, Hunt DM, Miller JP, Areán PA, Cheng AL. Digital mental health intervention plus usual care compared with usual care only and usual care plus in-person psychological counseling for orthopedic patients with symptoms of depression or anxiety: cohort study. JMIR formative Res. (2022) 6:e36203. doi: 10.2196/36203
55. Fulmer R, Joerin A, Gentile B, Lakerink L, Rauws M. Using psychological artificial intelligence (tess) to relieve symptoms of depression and anxiety: randomized controlled trial. JMIR Ment Health. (2018) 5:e9782. doi: 10.2196/mental.9782
56. Dosovitsky G, Bunge E. Development of a chatbot for depression: adolescent perceptions and recommendations. Child Adolesc Ment Health. (2023) 28:124–7. doi: 10.1111/camh.12627
57. Sharma B, Puri H, Rawat D. (2018). Digital psychiatry-curbing depression using therapy chatbot and depression analysis, in: 2018 Second International Conference on Inventive Communication and Computational Technologies (ICICCT) Coimbatore, India. 627–631. doi: 10.1109/ICICCT.2018.8472986
58. van Cuylenburg HC, Ginige T. (2021). Emotion guru: a smart emotion tracking application with ai conversational agent for exploring and preventing depression, in: 2021 International Conference on UK-China Emerging Technologies (UCET). Chengdu, China. 1–6. doi: 10.1109/UCET54125.2021.9674993
59. Forman-Hoffman VL, Pirner MC, Flom M, Kirvin-Quamme A, Durden E, Kissinger JA, et al. Engagement, satisfaction, and mental health outcomes across different residential subgroup users of a digital mental health relational agent: exploratory single-arm study. JMIR Formative Res. (2023) 7:e46473. doi: 10.2196/46473
60. Bunge EL. Artificial intelligence chatbot for depression in youth: Development, usage, and outcomes. In: 2020 Virtual Meeting. AACAP, THE SCIENTIFIC PROCEEDINGS of the 67th Annual Meeting of the American Academy of Child & Adolescent Psychiatry12 October 2020 - 24 October 2020. (2020).
61. Khawaja Z, Bélisle-Pipon J-C. Your robot therapist is not your therapist: understanding the role of ai-powered mental health chatbots. Front Digital Health. (2023) 5:1278186. doi: 10.3389/fdgth.2023.1278186
62. Maharjan R, Doherty K, Rohani DA, Bækgaard P, Bardram JE. Experiences of a speech-enabled conversational agent for the self-report of well-being among people living with affective disorders: an in-the-wild study. ACM Trans Interactive Intelligent Syst (TiiS). (2022) 12:1–29. doi: 10.1145/3484508
63. Yang S, Lee J, Sezgin E, Bridge J, Lin S. Clinical advice by voice assistants on postpartum depression: cross-sectional investigation using apple siri, amazon alexa, google assistant, and microsoft cortana. JMIR mHealth uHealth. (2021) 9:e24045. doi: 10.2196/24045
64. van der Schyff EL, Ridout B, Amon KL, Forsyth R, Campbell AJ. Providing self-led mental health support through an artificial intelligence–powered chat bot (leora) to meet the demand of mental health care. J Med Internet Res. (2023) 25:e46448. doi: 10.2196/46448
65. Guingrich R, Graziano MS. Chatbots as social companions: How people perceive consciousness, human likeness, and social health benefits in machines. arXiv preprint arXiv:2311.10599. (2023). doi: 10.48550/arXiv.2311.10599
66. Laurenceau I, Louis JD, Gilbert JE. (2022). Examining bias in sentiment analysis algorithms interacting with emojis with skin tone modifiers, in: Stephanidis, C., Antona, M., Ntoa, S. (eds) HCI International 2022 Posters. HCII 2022. Communications in Computer and Information Science, 1582. Springer, Cham. doi: https://doi.org/10.1007/978-3-031-06391-6_70
67. Kiritchenko S, Mohammad S. Examining gender and race bias in two hundred sentiment analysis systems. In: Nissim M, Berant J, Lenci A, editors. Proceedings of the Seventh Joint Conference on Lexical and Computational Semantics. Association for Computational Linguistics, New Orleans, Louisiana (2018). p. 43–53. doi: 10.18653/v1/S18-2005
68. Elliott R, Bohart AC, Watson JC, Murphy D. Therapist empathy and client outcome: An updated meta-analysis. Psychotherapy. (2018) 55:399. doi: 10.1037/pst0000175
69. Blease C, Torous J. Chatgpt and mental healthcare: balancing benefits with risks of harms. BMJ Ment Health. (2023) 26(1):e300884. doi: 10.1136/bmjment-2023-300884
70. Daws R. Medical chatbot using openai’s gpt-3 told a fake patient to kill themselves. AI News. (2020). Available at: https://www.artificialintelligence-news.com/news/medical-chatbot-openai-gpt3-patient-kill-themselves/.
71. El Atillah I. Man ends his life after an ai chatbot ‘encouraged’him to sacrifice himself to stop climate change. euronews. com. (2023). Available at: https://www.euronews.com/next/2023/03/31/man-ends-his-life-after-an-ai-chatbot-encouraged-him-to-sacrifice-himself-to-stop-climate-.
Keywords: depression, mental health, emotion, sentiment, emotion analysis, chatbot, conversational agent, ethics
Citation: Denecke K and Gabarron E (2024) The ethical aspects of integrating sentiment and emotion analysis in chatbots for depression intervention. Front. Psychiatry 15:1462083. doi: 10.3389/fpsyt.2024.1462083
Received: 09 July 2024; Accepted: 18 October 2024;
Published: 14 November 2024.
Edited by:
Jasmine M. Noble, Mood Disorders Society of Canada, CanadaCopyright © 2024 Denecke and Gabarron. This is an open-access article distributed under the terms of the Creative Commons Attribution License (CC BY). The use, distribution or reproduction in other forums is permitted, provided the original author(s) and the copyright owner(s) are credited and that the original publication in this journal is cited, in accordance with accepted academic practice. No use, distribution or reproduction is permitted which does not comply with these terms.
*Correspondence: Elia Gabarron, ZWxpYS5nYWJhcnJvbkBoaW9mLm5v