- 1Department of Neuroscience, University of Padova, Padova, Italy
- 2De Leo Fund, Research Division, Padova, Italy
- 3Padova University Hospital, Padova, Italy
- 4Padova Neuroscience Center, University of Padova, Padova, Italy
- 5Italian Psychogeriatric Association, Padova, Italy
- 6Australian Institute for Suicide Research and Prevention, Griffith University, Mt Gravatt Campus, Brisbane, QLD, Australia
- 7Slovene Centre for Suicide Research, Primorska University, Koper, Slovenia
Background: People in late adulthood die by suicide at the highest rate worldwide. However, there are still no tools to help predict the risk of death from suicide in old age. Here, we leveraged the Survey of Health, Ageing, and Retirement in Europe (SHARE) prospective dataset to train and test a machine learning model to identify predictors for suicide in late life.
Methods: Of more than 16,000 deaths recorded, 74 were suicides. We matched 73 individuals who died by suicide with people who died by accident, according to sex (28.8% female in the total sample), age at death (67 ± 16.4 years), suicidal ideation (measured with the EURO-D scale), and the number of chronic illnesses. A random forest algorithm was trained on demographic data, physical health, depression, and cognitive functioning to extract essential variables for predicting death from suicide and then tested on the test set.
Results: The random forest algorithm had an accuracy of 79% (95% CI 0.60-0.92, p = 0.002), a sensitivity of.80, and a specificity of.78. Among the variables contributing to the model performance, the three most important factors were how long the participant was ill before death, the frequency of contact with the next of kin and the number of offspring still alive.
Conclusions: Prospective clinical and social information can predict death from suicide with good accuracy in late adulthood. Most of the variables that surfaced as risk factors can be attributed to the construct of social connectedness, which has been shown to play a decisive role in suicide in late life.
Introduction
Suicide among people of old age is a serious public health concern. It is well-known that populations around the world are getting older (1), and this trend sets a growing concern about the need to address the issue of suicide risk among middle-aged and older adults.
Globally, from 1990 to 2017, age-standardized suicide death rates decreased by 32.7% (2), and quality of life and access to health care improved (3). However, suicide rates among individuals aged 65 and over are still the highest among men and women in nearly all regions of the world (2, 4–6). In general, suicide rates tend to increase with advancing age: suicide in old age affects 27.45 individuals per 100,000 population in the age group over 70 years and approximately 17 people per 100,000 inhabitants in the 50-69 age group (5). Moreover, suicide rates are underestimated, especially among older people (7). In addition, the unequivocal attribution of a manner of death to suicide can sometimes be challenging due to a possible attempt of the person to disguise the suicidal intent (e.g., as in some single road traffic deaths (8),) or due to the peculiar circumstances of death (e.g., as in house accidents or falls (3, 7)).
Epidemiologists forecast that, in less than 30 years (9), there may be an almost doubling of the older adult population and a growing percentage of single-nuclear families. This prediction leaves researchers and mental health professionals afraid of an increase in loneliness and dependence, which are factors frequently implicated in suicidal behavior (10, 11). The aging process and death from suicide are two psychosocial phenomena linked by a multidimensional and multifactorial nature (12). Old age is generally associated with a decline in physical and mental functions and an increase in chronic and physical diseases that often result in functional limitations and disabilities (13). Changes in social status and loss of social networks and family support are also commonly experienced as individuals age. These are stressors that can affect the quality of life of individuals and increase vulnerability to mental health problems and suicide risk (14–16). Although several risk factors for suicidal behavior have been identified, practical tools to accurately predict which individuals, especially older people, will attempt or die by suicide are substantially lacking (17). A prevention-oriented risk assessment implies identifying longitudinal predictors that may significantly increase the odds of death from suicide and that can be addressed (i.e., mitigated) to decrease the risk of suicide (18, 19).
Important recommendations to improve suicide risk assessment include considering contextual and sociocultural factors of suicidal behavior (20, 21) and possibly leveraging large amounts of data and machine learning techniques to increase the calculation capacity and the possibility of identifying people at risk (22–24). The combination of all available information (data from questionnaires and socio-demographic and clinical factors) could provide better assessment capabilities for preventing suicide (25–28). Noteworthily, given the higher lethality of suicide attempts in late life [lethal to non-lethal suicide attempt ratio is 1:4 in late life and 1:200 in young adults (29)], there is potentially no room for secondary prevention. Thus, effective primary prevention of suicide is the most meaningful outcome to pursue in this population.
Implementing a machine learning-based predictive approach in suicide prevention offers solutions to the challenges of modeling complex, high-dimensional data with non-linear relationships. Overcoming these challenges with common statistical approaches is non-trivial. In particular, random forest models assess the importance of different features in predicting suicide risk while being less prone to overfitting compared to logistic regression.
In this study, we queried the large prospective dataset from the Survey of Health, Aging, and Retirement in Europe (SHARE) (30, 31), comprising more than 16,000 deaths from different causes (and 74 deaths from suicide), to develop a machine learning algorithm for suicide prediction in older people. We matched the case and control samples by suicide ideation and then implemented a random forest model, as previously done to predict suicide (32, 33). The expedient of matching by suicidal ideation ensures that the model will not rely on this variable. This choice is crucial for two reasons. The first is statistical, as suicidal ideation is only moderately associated with death by suicide (34); thus, the model could heavily bias its classification based on another suicide-related variable; the second is practical, as suicidal ideation (as well as death thoughts/wishes) can remain undisclosed (35, 36) or be denied (37). We hypothesized that social and physical health-related variables would be the most important variables that the algorithm leveraged to predict suicide (13–16). Thus, we aimed at developing a proof-of-concept algorithm as well as testing the association between constructs of social (dis)connectedness and suicide in older adults.
Materials and methods
Dataset curation
The Survey of Health, Aging, and Retirement in Europe (SHARE) (30, 31) is a research infrastructure that collects prospective data on physical, mental, social, and economic well-being and independence in activities of daily living of nationally representative samples of people aged 50 or over in Europe. Participants are residents of 28 European nations and Israel. Sampling bias was addressed by SHARE researchers by sampling SHARE participants using probability selection methods. This dataset is coordinated by the Munich Center for the Economics of Aging (MEA) in collaboration with the English Longitudinal Study of Aging (ELSA) and the U.S. Health and Retirement Study (HRS). Since 2005, the research consortium has collected data on the abovementioned variables in eight “waves.” From the second wave onward, data on the cause, manner, circumstances, and antecedents (up to 1 year before) of death were collected if a participant died in the period between the waves. In such a case, the next-of-kin completed an interview as a proxy interviewee. From wave 2 to wave 9 (excluding wave three for reasons explained below), 16,548 deaths were recorded. Study design, sampling, and data resources for SHARE are described in detail elsewhere (30) and can be found online (https://share-eric.eu/).
Data retrieved from the SHARE dataset were managed with RStudio 4.1.2 (38). First, we grouped all interviews after death for each n-wave. We matched the information collected therein to the health, demographic, and social factors that the participant, who then died, provided in the n-1 wave. We chose the interview preceding death as the baseline for the following reason: each participant, unless they dropped out of the study, had completed at least two interviews (one by themselves, the second by the next of kin had death occurred) and, among participants, the number of completed interviews (waves) could vary enough to make a data analysis plan non-trivial.
Since data on participants’ mental health in wave three were not collected as in other waves, we opted not to consider the deaths that occurred in wave four. We thus obtained a dataset that matched before-death information on mental, physical, social, and economic well-being to the cause of death and its circumstances for each wave from 2005 to 2020. The variables considered in the analysis were demographics and household variables (e.g., household size, help received), antecedents to death, behavioral risks (e.g., smoking), cognitive functioning, financial and economic health, physical health (including measurement of grip strength), mental health (e.g., depressive symptoms). See the Supplementary Material for the complete list of variables (Supplementary Table S1). Given that the baseline measures could have varied from wave to wave, we harmonized the dataset by removing the variables not shared across the waves (all the variables included in the analysis are reported in Supplementary Appendix Table S1).
Statistical analysis
In the seven waves analyzed, 74 deaths by suicide were observed. One of such deaths was later not included in the analysis as the age of death was not collected. We matched the participants who died by suicide one-on-one, by nearest neighbor rule (39), with participants who died in an accident [as in (40, 41)] by gender, age at death, number of chronic physical illnesses, and wish to be dead (present, absent, “do not know” and refuse to report). If data regarding the outcome of interest (i.e., manner of death) or variables on which matching was performed (e.g., suicidal ideation/age at death) were missing, the participant(s) were excluded from further analysis. We used the default and most common matching technique for the nearest neighbor method, propensity score difference (42, 43).
Suicidal ideation was assessed by the interviewer with EURO-D scale item 4: “In the last month, have you felt that you would rather be dead?”. Any mention of suicidal feelings or wishing to be dead was marked as the presence of at least some degree of wish to die. Therefore, active suicidal ideation and passive suicidal ideation were not differentiated in this dataset. The remaining NA values were imputed using median values of the same variable per timepoint (baseline or follow-up) (44). Furthermore, we transformed two variables, the frequency of contact with next-of-kin and the duration of illness, from categorical predictors (e.g., daily contacts = 6, …, weekly contacts = 4) to continuous numeric variables, since we thought that these data would be more informative in a numeric format.
Lastly, the dataset was divided 80/20 into two subsets [train and test (45)]. The larger subset (deaths by suicide = 59; deaths by accident = 58) was used to train a multivariable random forest model in R [(46) see also Methods S1] and thus identify which factors could be leveraged to distinguish death from suicide from death from accident; the remaining subset was used to test the metrics of the model (sensitivity, specificity, overall accuracy, etc.). We opted to employ random forest algorithms because we expected the dataset to contain a large number of potentially useful predictors, with some of them being collinear or interacting with each other in a non-linear fashion. Moreover, random forests deal well with high dimensional data (47). It has also been shown to be effective even with small sample sizes (48). A random forest is an ensemble learning method used for classification and regression tasks in machine learning. In our case, the random forest for classification starts by creating multiple bootstrap samples. For each bootstrap sample, a decision tree is constructed. Instead of considering all features (variables) for splitting at each decision tree node, a random subset of features is chosen. This process makes the trees decorrelated and reduces overfitting.
Once all decision trees have been constructed, predictions are made for each tree. For classification tasks, each tree’s prediction is considered a “vote,” and the class with the most votes becomes the final prediction. Performance metrics to evaluate the performance of a random forest model could be accuracy, sensitivity, and specificity. Data visualization was aided by the randomForestExplainer package (49).
Results
Population characteristics
A total of 146 individuals were included in this analysis (Table 1): half died by suicide, and the other half by accident. Most of the participants lived in Estonia (14.4%), Belgium (10.3%), France (8.9%), Austria (8.2%), Czech Republic (7.5%), and Greece (6.8%), while the rest were from various European countries. Approximately 75% of the deceased were men, and the total sample age of death was 68.05 ± 16.41 years. The next-of-kin who answered the after-death interview was the partner in 40.4% of cases and a son/daughter in 19.2% of cases; notably, a non-relative in approximately 1 out of 4 deceased (a next-of-kin could have also been a neighbor or someone helping in the house). The mean number of children still alive at the participant’s death was 1.90 ± 1.92. Regarding when the deceased had contact with next-of-kin in the last year, in most cases (59.6%), the contact occurred daily. In 1 in 10 cases, the contacts occurred less than once a month or never in the last year.
Regarding the duration of the illness before death, the most frequent answers were one year or more (24%) and less than one month (59.6%). Regarding the total time spent hospitalized, 90.4% of the sample stayed in hospital between 1 and 4 weeks in the last year. Sensitivity analysis for groups of illnesses and reasons for hospital stays could not be conducted because of the reduced sample size and/or unavailability of further details. More than half of the sample were visited by their GP at least once in the year before death, and approximately 68% took medication for any physical or mental illness. Difficulties in activities of daily living (ADL) were present in 68.5% of the sample. Regarding long-term diseases, 83.6% of the sample reported having at least one. Only 6 participants (4.1%) reported making any mention of suicidal feelings or the wish to be dead. In contrast, 32.9% of the sample denied such feelings. For 63% of the sample, the interviewer did not report if suicidal ideation was present/absent or if the participant did not know or refused to answer.
Regarding the time gap between the last in-person interview with the participant and the end-of-life interview with the next-of-kin (due to the death of the participant), only one year passed for most death occurrences (43 deaths by suicide and 45 accidents). Two years passed for 11 deaths by accident and ten deaths by suicide (next-of-kin interviews that took place at waves 3 and 9); three years for 17 deaths by accident and 20 by suicide (next-of-kin interviews that took place at waves 2 and 5). Furthermore, 71.2% of the participants did not have a will at the time of death.
No significant differences emerged between participants who died by suicide and those who died by accident, except for the length of illness before death: participants who died by suicide (37%) had been ill for more than one year, while these figures were significantly lower for people who died by accident (11%), who, on the other hand, had a more recent illness onset (82.2% in the previous month compared to 37% of those who died by suicide). Before implementing the random forest model, we conducted a univariate analysis to discern which factors differed between the two population samples before the one-on-one matching (Supplementary-Table S2) and after the matching (Table 1). Before matching, people who died by suicide (n=73) differed significantly from people who died in accidents (n=420) in age at death (69.09 ± 11.78 and 76.72 ± 11.72 respectively – t = 5.041, df = 95.563, p-value <.001) and duration of illness before death (longer duration of illness for people who died by suicide – X-squared = 89.74, df = 5, p <.001). After one-to-one-matching, which included matching also based on age at death, only the duration of the illness before death was still significantly different (X-squared = 33.308, df = 4, p <.001). In the univariate analysis, no other statistically significant differences between the two samples emerged before or after one-on-one matching in the variables of interest.
Random forest model
We implemented a random forest algorithm to determine which factors reported at wave n-1 (henceforth “baseline”) or retrospective information collected from the next-of-kin during the end-of-life interview could be leveraged to improve the prediction of death from suicide at wave n.
The algorithm was trained on 80% of the sample to predict two possible outcomes: death from suicide and death from accident. Using the split mentioned above, the algorithm was asked to correctly categorize 58 deaths by accidents and 59 deaths by suicide. At the training level, the overall classification error (out-of-bag estimate of error - OOB) was 33.33% (s.d. = 3.3%), with no striking difference between the misclassification of death from accidents or death from suicide: 39 of 59 deaths by suicide were correctly categorized (error rate 33.89%); out of 58 deaths by accidents, 39 were correctly identified as such (error rate 32.75%). The most important variables (as measured via accuracy and Gini decrease) to tell apart deaths by accident and death from suicide were the following (Figure 1A): the relationship between the next-of-kin and the decedent (the next-of-kin of people who died by suicide was more likely to be a non-relative), the total length of hospitalization in the previous year, and if any access to the hospital was necessary for therapy administration (the need for hospitalization increased the risk of suicide, especially shorter-length hospitalizations), difficulties in the activities of daily living, how many hours of help the decedent needed (the fewer hours of help needed, the higher the risk of suicide) if they owned a home and had a will. The three most important variables identified by the algorithm were: longer duration of illness, less than daily contact with the next-of-kin, and less than three children still alive, which were all independent predictors of suicide. Moreover, we found relevant interactions between the duration of the illness, the number of children still alive, and the frequency of contact with the next of kin (Figure 1B). In particular, a longer-than-one-month illness duration increases the risk of suicide with respect to a shorter length; this risk could be further exacerbated if the participant had fewer than three children still alive (on the other hand, having three or more children mitigated the probability of suicide regardless of the longer-than-one-month illness duration). A similar interaction was present throughout the duration of the illness and contact with the next-of-kin (Figure 1C). Noteworthily, less than daily contact with the next-of-kin increased the risk of suicide. Lastly, we found an interaction between the frequency of contact with the next-of-kin and the number of children still alive (Figure 1D). The algorithm identified an increased risk of suicide with a reduced frequency of contact and a smaller number of children still alive.
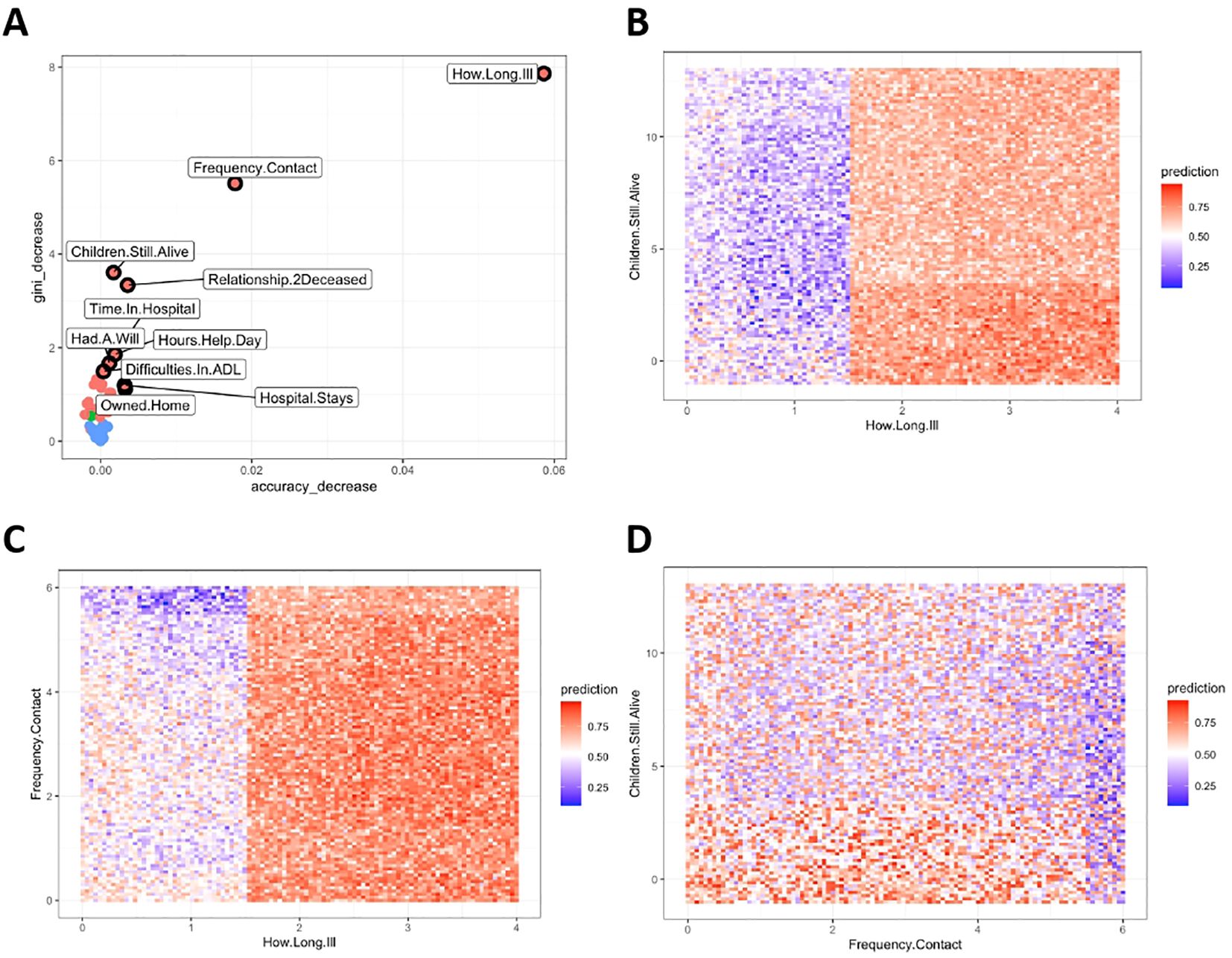
Figure 1. Predictors distinguishing death from suicide from death from accident. ADL, Activities of Daily Living. Gini Importance = This index estimates how much a random forest relies on a particular feature in classification. In particular, a decrease of Gini Importance measures how much a variable helps in the correct classification of cases (by assessing the loss of purity if that variable is excluded from the analysis). It measures the average gain of purity by splits of a given variable. If the variable (e.g., duration of illness) is useful, it splits mixed labeled nodes (mixed group of participants that died by suicide or accident) into pure single class nodes (two groups, one with people who died by suicide and one with people who died by accident). Accuracy decrease = It indicates the loss of model performance without each variable (excluded from the analysis one at the time). (A) The plot displays variables according to their contribution to the model’s performance, as determined by metrics such as accuracy decrease and Gini decrease. Variables closer to the upper right corner have a greater impact to model performance, indicating their higher importance in distinguishing between outcomes (e.g., deaths by suicide vs. accidents). For instance, removing “Duration of Illness – How.Long.Ill” decreases the model’s accuracy by 0.06 (6%) and its Gini index by 8 (in range 0-100). (B) This panel illustrates the predicted probability of death by suicide, influenced by the duration of illness and the number of children still alive. The color gradient from 0 (deep blue) to 1 (red) represents increasing suicide risk. For example, the lower right corner, where the probability approaches 1, indicates a high likelihood of classifying a death by suicide if the duration of illness is long and the number of children is low. The illness duration is categorized as follows: 1 = less than one month, 2 = 1-6 months, 3 = more than six months, 4 = a year or more. A longer duration typically increases risk, but this can be mitigated if the individual has three or more children alive. (C) This plot shows how suicide risk varies with the duration of illness and the frequency of contact with the next-of-kin. The frequency ranges from 0 (=never) to 6 (=daily), with 2 representing about once a month and 4 about once a week. Higher frequencies of contact, especially daily, generally indicated a reduced risk of suicide. However, this protective effect diminishes with longer illness durations. (D) This panel examines the relationship between contact frequency with next-of-kin and the number of children still alive on suicide risk. No distinct patterns are evident, but the data suggest that lower contact frequency and fewer children are associated with increased suicide risk.
By leveraging the above variables, the random forest algorithm was required to categorize the deaths of the remaining 20% of the sample. Eleven of the 15 deaths by accidents were correctly identified. Regarding deaths from suicide, the prediction was corrected for 12 out of 14 deaths. This classification yielded an overall accuracy of 0.79 (CI [0.60 – 0.92], p = 0.002) with a sensitivity of.80 and a specificity of.78.
Discussion
In this study, we queried the Survey of Health, Ageing, and Retirement in Europe (SHARE) dataset to reveal the predictors of death from suicide in late adulthood. We applied a supervised machine learning (random forest) algorithm to automatically extract the variables deemed important in discerning death from suicide from death from accident after appropriate population matching according to sex, age of death, number of physical illnesses, and suicidal ideation. Our analyses returned several physical and social health variables that are central in distinguishing accidental death from suicide. These variables included the duration of illness, the frequency of contact with the next-of-kin, the number of children, the time spent in the hospital in the last year, the hours of help per day needed, the presence of difficulties in the activities of daily living, the relationship between the next-of-kin and the decedent. We showed that great importance is placed on the duration of the illness (Figure 1): illnesses lasting more than a month in the year before death were more likely to predict death from suicide than death from accident, and longer-term illnesses (i.e., > one year) posit a higher risk of suicide. Nevertheless, the data from this sample seem to indicate that death from suicide was more probable with a duration of illness of 1-6 months. We argue that this should be considered at least in light of the age of the deceased and the nature of the illness itself: rapid-onset illnesses that hinder the daily activities of individuals or require extensive medical care with no apparent prospect of recovery (50), challenge daily living, and could further exacerbate a deterioration in physical or mental conditions of older people. Contact with healthcare providers has already been shown to be more likely to occur less than a month before death from suicide in older adults than in younger adults (51), probably correlated with a greater need for care. Although detailed analyses would be essential to determine the impact of specific illnesses on suicide, this was unfeasible, as it would have required a larger sample size. However, by leveraging the SHARE dataset, other authors reported that specific system diseases are more likely to be associated with suicidal ideation (52), although no correlation between suicidal ideation and death from suicide could be drawn (53). The most important factors were the frequency of contact with the next-of-kin (who also completed an end-of-life interview by proxy), their relationship with the decedent, and the number of children the individual had before death. In this sample, the next-of-kin of the individuals who died by suicide was a non-relative 31.5% of the time, with respect to 19.2% for those who died by accident, indicating a lesser presence of family members in the life of those who died by suicide. All these variables are related to the participant’s social connections before death and indicate the role of interactions with family members (41). The role that family might play in suicide prevention has previously been evidenced, specifically by reducing feelings of loneliness, increasing belongingness, and possibly reducing anxiety and depressive symptoms (16). Here, we report that there could be a “dose threshold” for the frequency of contact with next-of-kin, above which the probability of death from suicide could be diminished. Specifically, we showed that people who had daily or multiple contacts a week with their next-of-kin had a reduced likelihood of dying by suicide, also considering the duration of the disease and the number of children still alive. The algorithm also identified that participants who had no children alive at their death were more likely to have died by suicide than those with children, particularly those with three or more. To further corroborate the hypothesis of the pivotal role of family support in suicide prevention in late life, the algorithm identified the relationship between the next-of-kin and the decedent as a predictor in differentiating death from suicide from death from accident. Few other studies investigated the link between loneliness and death from suicide (54), and a recent meta-analysis also highlighted that no studies published up to 2020 included suicide death as a distinct outcome measure (10). However, most of the published literature indicates a moderate to strong association between loneliness and non-lethal suicidal behaviors. These findings presented herein evidenced that factors related to loneliness may be a predictor of death from suicide, at least in older adults, as postulated by recent theories of suicide (55, 56). Further studies are needed to corroborate our findings in other geographical areas.
Limitations
Although this analysis is based on a prospective, harmonized dataset, it is worth noting that this data survey was not conceptualized for studying suicidal behaviors. Therefore, some important variables related to this phenomenon were not collected. For example, we highlight that no measures for grief, which was shown to be an important factor for suicide death (41, 57), were available; in addition, there was no direct information on previous or current mental disorders among the participants, but only data on current mood could be drawn from the EURO-D scale. Moreover, suicidal ideation was not thoroughly assessed with specific questions aimed at knowing if the respondent had the intention or plan to end their life, or, on the other hand, the frequency and intrusiveness of those thoughts. In particular, we highlight that for more than half of the sample, data on suicide ideation is either missing or the participant did not know if they had suicidal thoughts/refused to answer. This could be interpreted in light of the difficulty that elderly people experience in disclosing the wish to die. However, no strong conclusions can be made regarding the reason for missing data at this point. Similarly, details regarding the circumstances and dynamics of the accidents that resulted in the death of a person were not available. Therefore, it cannot be certainly excluded that some deaths by accidents were not suicide attempts. However, such events tend to be single-car accidents (less than 3% of all road fatalities are thought to be suicides (58)). In this sample, only three people (4.1%) who died by accident died outside of their house/hospital (compared to 14 people (19.2%) who died by suicide), making the chances of misclassification negligible. Furthermore, end-of-life interviews were conducted with the help of the next-of-kin and, obviously, in a retrospective manner. This likely implied recall bias. In addition, respondents might have reported purposefully inflated/deflated figures regarding the decedent’s care to deal with the interviewers’ desirability/sense of righteousness, although it would be impossible to prove if this has ever occurred.
Second, death from suicide represents approximately 1% of all deaths. Given the rarity of the event, thousands of deaths had to be recorded to have enough data to draw decently solid conclusions. In this dataset, 74 suicides were registered out of 16,548 deaths. Although this sample size might prove sufficient to evince strong associations and contributions of socio-demographic and clinical factors to suicide, some nuances might not be evidenced: for example, it was unfeasible to conduct further analysis on the role of the duration of illnesses of specific systems (e.g., cardiovascular, respiratory, etc.) on suicide. Moreover, some data were not reported for all participants and had to be imputed. This is a typical case for large datasets.
Lastly, it should be noted that the statistical approach presented herein differentiates deaths by suicide from deaths by accident based on the quality and quantity of data provided, regardless of previous findings or authors’ views.
Conclusions
We employed a machine learning algorithm to demonstrate the predictors of late-life suicide in a large longitudinal European cohort. When tested, the random forest algorithm yielded an overall accuracy of 0.79 (CI [0.60 – 0.92], p = 0.002). It highlighted that the most important variables used to discern deaths by suicide from deaths by accident were social connectedness-related (frequency of contact with the next-of-kin, the relationship with the next-of-kin, the number of children still alive) and physical illness-related (duration of illness before deaths, length of hospitalization in the 12 months preceding deaths, difficulties in the activities of daily living). The findings presented here provide a hierarchical importance of predictors for late-life suicide and highlight social connection and physical health as critical variables for assessing suicide risk. Replication of these findings and deepening our understanding of these predictors through experts by experience (i.e., survivors of near-lethal suicide attempts) will be instrumental in designing accurate prediction models and tailored interventions for suicide prevention.
Data availability statement
Publicly available datasets were analyzed in this study. This data can be found here: https://share-eric.eu/data/data-access.
Ethics statement
Ethical approval was not required for the study involving humans in accordance with the local legislation and institutional requirements. Written informed consent to participate in this study was not required from the participants or the participants’ legal guardians/next of kin in accordance with the national legislation and the institutional requirements.
Author contributions
NM: Conceptualization, Data curation, Formal analysis, Investigation, Methodology, Software, Visualization, Writing – original draft, Writing – review & editing. JZ: Writing – original draft, Writing – review & editing. FS: Conceptualization, Supervision, Writing – review & editing. DD: Supervision, Writing – review & editing.
Funding
The author(s) declare financial support was received for the research, authorship, and/or publication of this article. Open Access funding provided by Università degli Studi di Padova | University of Padua, Open Science Committee.
Conflict of interest
The authors declare that the research was conducted in the absence of any commercial or financial relationships that could be construed as a potential conflict of interest.
Publisher’s note
All claims expressed in this article are solely those of the authors and do not necessarily represent those of their affiliated organizations, or those of the publisher, the editors and the reviewers. Any product that may be evaluated in this article, or claim that may be made by its manufacturer, is not guaranteed or endorsed by the publisher.
Supplementary material
The Supplementary Material for this article can be found online at: https://www.frontiersin.org/articles/10.3389/fpsyt.2024.1455247/full#supplementary-material
References
1. United Nations, Department of Economic and Social Affairs, Population Division. World Population Prospects 2022: Summary of Results. (2022). Available online at: https://www.un.org/development/desa/pd/content/World-Population-Prospects-2022 (Accessed April 22, 2024).
2. Naghavi M. Global, regional, and national burden of suicide mortality 1990 to 2016: systematic analysis for the Global Burden of Disease Study 2016. BMJ. (2019) l94:1–13. doi: 10.1136/bmj.l94
3. De Leo D. Late-life suicide in an aging world. Nat Aging. (2022) 2:7–12. doi: 10.1038/s43587-021-00160-1
4. Lapierre S, Erlangsen A, Waern M, De Leo D, Oyama H, Scocco P, et al. A systematic review of elderly suicide prevention programs. Crisis. (2011) 32:88–98. doi: 10.1027/0227-5910/a000076
5. Global Burden of Disease Collaborative Network. Global Burden of Disease Study 2017 (GBD 2017) Burden by Risk 1990-2017. (2018).
6. World Health Organization (WHO). Preventing suicide: A global imperative. Available online at: https://www.who.int/publications/i/item/9789241564779 (Accessed April 22, 2024).
7. De Leo D, Arnautovska U. Prevention and treatment of suicidality in older adults. In: O’Connor RC, Pirkis J, editors. The International Handbook of Suicide Prevention, 1st ed. UK: Wiley (2016). p. 323–45. doi: 10.1002/9781118903223.ch18
8. Pompili M, Serafini G, Innamorati M, Montebovi F, Palermo M, Campi S, et al. Car accidents as a method of suicide: A comprehensive overview. Forensic Sci Int. (2012) 223:1–9. doi: 10.1016/j.forsciint.2012.04.012
9. Organisation for Economic Co-operation and Development. Going for growth 2021: shaping a vibrant recovery(2021). Available online at: https://www.oecd.org/newsroom/release-of-oecd-s-going-for-growth-2021-shaping-a-vibrant-recovery.htm (Accessed March 13, 2024).
10. McClelland H, Evans JJ, Nowland R, Ferguson E, O’Connor RC. Loneliness as a predictor of suicidal ideation and behaviour: a systematic review and meta-analysis of prospective studies. J Affect Disord. (2020) :274:880–96. doi: 10.1016/j.jad.2020.05.004
11. Wand A, Verbeek H, Hanon C, De Mendonça Lima CA, Rabheru K, Peisah C. Is suicide the end point of ageism and human rights violations? Am J Geriatric Psychiatry. (2021) 29:1047–52. doi: 10.1016/j.jagp.2021.05.025
12. De Leo D, Giannotti AV. Suicide in late life: A viewpoint. Prev Med. (2021) 152:106735. doi: 10.1016/j.ypmed.2021.106735
13. Eisenberger NI. The pain of social disconnection: examining the shared neural underpinnings of physical and social pain. Nat Rev Neurosci. (2012) 13:421–34. doi: 10.1038/nrn3231
14. Chen Y, Feeley TH. Social support, social strain, loneliness, and well-being among older adults: An analysis of the Health and Retirement Study*. J Soc Pers Relationships. (2014) 31:141–61. doi: 10.1177/0265407513488728
15. Choi NG, DiNitto DM, Marti CN, Conwell Y. Physical health problems as a late-life suicide precipitant: examination of coroner/medical examiner and law enforcement reports. Gerontologist. (2019) 59:356–67. doi: 10.1093/geront/gnx143
16. Santini ZI, Jose PE, York Cornwell E, Koyanagi A, Nielsen L, Hinrichsen C, et al. Social disconnectedness, perceived isolation, and symptoms of depression and anxiety among older Americans (NSHAP): a longitudinal mediation analysis. Lancet Public Health. (2020) 5:e62–70. doi: 10.1016/S2468-2667(19)30230-0
17. Franklin JC, Ribeiro JD, Fox KR, Bentley KH, Kleiman EM, Huang X, et al. Risk factors for suicidal thoughts and behaviors: A meta-analysis of 50 years of research. Psychol Bull. (2017) 143:187–232. doi: 10.1037/bul0000084
18. Pisani AR, Murrie DC, Silverman MM. Reformulating suicide risk formulation: from prediction to prevention. Acad Psychiatry. (2016) 40:623–9. doi: 10.1007/s40596-015-0434-6
19. Pisani AR, Murrie DC, Silverman M, Turner K. Prevention-oriented risk formulation. In: Pompili M, editor. Suicide Risk Assessment and Prevention. Springer International Publishing, Cham (2022). p. 119–49. doi: 10.1007/978-3-030-42003-1_13
20. Fleischmann A, Bertolote JM, De Leo D, Wasserman D. Instruments used in SUPRE-MISS: The WHO Multisite Intervention Study on Suicidal Behaviours. In: Wasserman D, Wasserman C, editors. Oxford Textbook of Suicidology and Suicide Prevention, 1st ed. Oxford University Press, Oxford (2009). p. 313–6. Available at: https://academic.oup.com/book/24371/chapter/187264273.
21. De Leo D. Ageism and suicide prevention. Lancet Psychiatry. (2018) 5:192–3. doi: 10.1016/S2215-0366(17)30472-8
22. Ruder TD, Hatch GM, Ampanozi G, Thali MJ, Fischer N. Suicide announcement on facebook. Crisis. (2011) 32:280–2. doi: 10.1027/0227-5910/a000086
23. Oquendo MA, Baca-Garcia E, Artés-Rodríguez A, Perez-Cruz F, Galfalvy HC, Blasco-Fontecilla H, et al. Machine learning and data mining: strategies for hypothesis generation. Mol Psychiatry. (2012) 17:956–9. doi: 10.1038/mp.2011.173
24. Corbitt-Hall DJ, Gauthier JM, Troop-Gordon W. Suicidality disclosed online: using a simulated facebook task to identify predictors of support giving to friends at risk of self-harm. Suicide Life Threat Behav. (2019) 49:598–613. doi: 10.1111/sltb.12461
25. Blasco-Fontecilla H, Delgado-Gomez D, Legido-Gil T, De Leon J, Perez-Rodriguez MM, Baca-Garcia E. Can the holmes-rahe social readjustment rating scale (SRRS) be used as a suicide risk scale? An exploratory study. Arch Suicide Res. (2012) 16:13–28. doi: 10.1080/13811118.2012.640616
26. Stefansson J, Nordström P, Runeson B, Åsberg M, Jokinen J. Combining the Suicide Intent Scale and the Karolinska Interpersonal Violence Scale in suicide risk assessments. BMC Psychiatry. (2015) 15:226. doi: 10.1186/s12888-015-0607-6
27. Bakhiyi CL, Calati R, Guillaume S, Courtet P. Do reasons for living protect against suicidal thoughts and behaviors? A systematic review of the literature. J Psychiatr Res. (2016) 77:92–108. doi: 10.1016/j.jpsychires.2016.02.019
28. Kim DW, Jeong KY, Kim KS. Psychological scales as predictors of emergency department hospitalizations in suicide attempters. Am J Emerg Med. (2018) 36:93–9. doi: 10.1016/j.ajem.2017.07.038
29. Conwell Y, Van Orden K, Caine ED. Suicide in older adults. Psychiatr Clin North Am. (2011) 34:451–68. doi: 10.1016/j.psc.2011.02.002
30. Börsch-Supan A, Brandt M, Hunkler C, Kneip T, Korbmacher J, Malter F, et al. Data resource profile: the survey of health, ageing and retirement in Europe (SHARE). Int J Epidemiol. (2013) 42:992–1001. doi: 10.1093/ije/dyt088
31. Bergmann M, Kneip T, De Luca G, Scherpenzeel A. Survey Participation in the Survey of Health, Ageing and Retirement in Europe (SHARE), Wave. (2022), Based on Release 8.0.0. SHARE Working Paper Series 81-2022, Germany.
32. Walsh CG, Ribeiro JD, Franklin JC. Predicting risk of suicide attempts over time through machine learning. Clin Psychol Sci. (2017) 5:457–69. doi: 10.1177/2167702617691560
33. Belsher BE, Smolenski DJ, Pruitt LD, Bush NE, Beech EH, Workman DE, et al. Prediction models for suicide attempts and deaths: A systematic review and simulation. JAMA Psychiatry. (2019) 76:642. doi: 10.1001/jamapsychiatry.2019.0174
34. McHugh CM, Corderoy A, Ryan CJ, Hickie IB, Large MM. Association between suicidal ideation and suicide: meta-analyses of odds ratios, sensitivity, specificity and positive predictive value. BJPsych Open. (2019) 5:e18. doi: 10.1192/bjo.2018.88
35. Cukrowicz KC, Duberstein PR, Vannoy SD, Lin EH, Unützer J. What factors determine disclosure of suicide ideation in adults 60 and older to a treatment provider? Suicide Life Threat Behav. (2014) 44:331–7. doi: 10.1111/sltb.12075
36. Hallford DJ, Rusanov D, Winestone B, Kaplan R, Fuller-Tyszkiewicz M, Melvin G. Disclosure of suicidal ideation and behaviours: A systematic review and meta-analysis of prevalence. Clin Psychol Rev. (2023) 101:102272. doi: 10.1016/j.cpr.2023.102272
37. Berman AL. Risk factors proximate to suicide and suicide risk assessment in the context of denied suicide ideation. Suicide Life Threat Behav. (2018) 48:340–52. doi: 10.1111/sltb.12351
38. R Core Team. R: A language and environment for statistical computing. Vienna, Austria: R Foundation for Statistical Computing (2019).
39. Ho DE, Imai K, King G, Stuart EA. MatchIt: nonparametric preprocessing for parametric causal inference. J Stat Soft. (2011) 42:1–28. http://www.jstatsoft.org/v42/i08/.
40. De La Vega Sánchez D, Guija JA, Pérez-Moreno P, Kelly SA, Santos M, Oquendo MA, et al. Association of religious activity with male suicide deaths. Suicide Life Threat Behav. (2020) 50:449–60. doi: 10.1111/sltb.12600
41. De Leo D, Draper BM, Snowdon J, Kõlves K. Suicides in older adults: A case–control psychological autopsy study in Australia. J Psychiatr Res. (2013) 47:980–8. doi: 10.1016/j.jpsychires.2013.02.009
42. Stuart EA. Matching methods for causal inference: A review and a look forward. Statist Sci. (2010) 25. doi: 10.1214/09-STS313.full
43. Thoemmes FJ, Kim ES. A systematic review of propensity score methods in the social sciences. Multivariate Behav Res. (2011) 46:90–118. doi: 10.1080/00273171.2011.540475
44. Berkelmans GFN, Read SH, Gudbjörnsdottir S, Wild SH, Franzen S, van der Graaf Y, et al. Population median imputation was noninferior to complex approaches for imputing missing values in cardiovascular prediction models in clinical practice. J Clin Epidemiol. (2022) 145:70–80. doi: 10.1016/j.jclinepi.2022.01.011
45. Guyon I. A scaling law for the validation-set training-set size ratio. Berkeley, California (1997).
47. Han S, Kim H, Lee YS. Double random forest. Mach Learn. (2020) 109:1569–86. doi: 10.1007/s10994-020-05889-1
48. Qi Y. Random forest for bioinformatics. In: Zhang C, Ma Y, editors. Ensemble Machine Learning. Springer New York, New York, NY (2012). p. 307–23. doi: 10.1007/978-1-4419-9326-7_11
49. Paluszynska A, Biecek P, Jiang Y. randomForestExplainer: explaining and visualizing random forests in terms of variable importance(2020). Available online at: https://CRAN.R-project.org/package=randomForestExplainer (Accessed March 19, 2024).
50. Cho J, Lee WJ, Moon KT, Suh M, Sohn J, Ha KH, et al. Medical care utilization during 1 year prior to death in suicides motivated by physical illnesses. J Prev Med Public Health. (2013) 46:147–54. doi: 10.3961/jpmph.2013.46.3.147
51. Luoma JB, Martin CE, Pearson JL. Contact with mental health and primary care providers before suicide: A review of the evidence. AJP. (2002) 159:909–16. doi: 10.1176/appi.ajp.159.6.909
52. Lutz J, Morton K, Turiano NA, Fiske A. Health conditions and passive suicidal ideation in the survey of health, ageing, and retirement in Europe. GERONB. (2016) 71:936–46. doi: 10.1093/geronb/gbw019
53. Lukaschek K, Engelhardt H, Baumert J, Ladwig KH. No correlation between rates of suicidal ideation and completed suicides in Europe: analysis of 49,008 participants (55+ Years) based on the survey of health, ageing and retirement in Europe (SHARE). Eur Psychiatr. (2015) 30:874–9. doi: 10.1016/j.eurpsy.2015.07.009
54. Shaw RJ, Cullen B, Graham N, Lyall DM, Mackay D, Okolie C, et al. Living alone, loneliness and lack of emotional support as predictors of suicide and self-harm: A nine-year follow up of the UK Biobank cohort. J Affect Disord. (2021) 279:316–23. doi: 10.1016/j.jad.2020.10.026
55. Chu C, Buchman-Schmitt JM, Stanley IH, Hom MA, Tucker RP, Hagan CR, et al. The interpersonal theory of suicide: A systematic review and meta-analysis of a decade of cross-national research. Psychol Bull. (2017) 143:1313–45. doi: 10.1037/bul0000123
56. O’Connor RC, Kirtley OJ. The integrated motivational–volitional model of suicidal behaviour. Phil Trans R Soc B. (2018) 373:20170268. doi: 10.1098/rstb.2017.0268
57. Harwood DMJ, Hawton K, Hope T, Harriss L, Jacoby R. Life problems and physical illness as risk factors for suicide in older people: a descriptive and case-control study. Psychol Med. (2006) 36:1265–74. doi: 10.1017/S0033291706007872
Keywords: suicide, old adults, belongingness, social connection, illness duration
Citation: Meda N, Zammarrelli J, Sambataro F and De Leo D (2024) Late-life suicide: machine learning predictors from a large European longitudinal cohort. Front. Psychiatry 15:1455247. doi: 10.3389/fpsyt.2024.1455247
Received: 26 June 2024; Accepted: 23 August 2024;
Published: 17 September 2024.
Edited by:
Vincenzo De Luca, University of Toronto, CanadaReviewed by:
José Ignacio Nazif-Munoz, Université de Sherbrooke, CanadaIsabel Heinrich, Johannes Gutenberg University Mainz, Germany
Deana Davalos, Colorado State University, United States
Khethelo Richman Xulu, University of the Witwatersrand, South Africa
Dusan Kolar, Queen’s University, Canada
Casimiro Cabrera Abreu, Queen’s University, Canada
William Sulis, McMaster University, Canada
Olusegun Baiyewu, University of Ibadan, Nigeria
Copyright © 2024 Meda, Zammarrelli, Sambataro and De Leo. This is an open-access article distributed under the terms of the Creative Commons Attribution License (CC BY). The use, distribution or reproduction in other forums is permitted, provided the original author(s) and the copyright owner(s) are credited and that the original publication in this journal is cited, in accordance with accepted academic practice. No use, distribution or reproduction is permitted which does not comply with these terms.
*Correspondence: Fabio Sambataro, fabio.sambataro@unipd.it
†ORCID: Nicola Meda, orcid.org/0000-0003-1114-3632
Josephine Zammarrelli, orcid.org/0000-0003-1760-5324
Diego De Leo, orcid.org/0000-0001-8255-6480
Fabio Sambataro, orcid.org/0000-0003-2102-416X