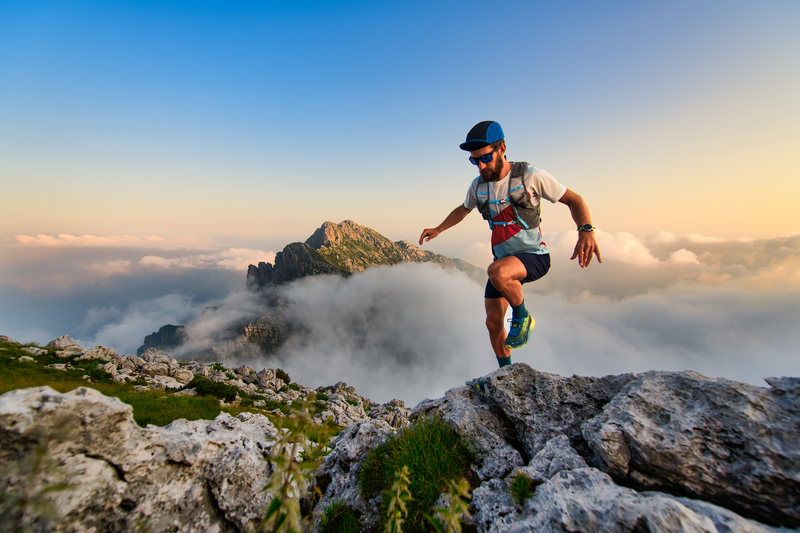
94% of researchers rate our articles as excellent or good
Learn more about the work of our research integrity team to safeguard the quality of each article we publish.
Find out more
METHODS article
Front. Psychiatry , 17 July 2024
Sec. Mood Disorders
Volume 15 - 2024 | https://doi.org/10.3389/fpsyt.2024.1436006
This article is part of the Research Topic Treatment Resistant Depression (TRD): epidemiology, clinic, burden and treatment View all 20 articles
Treatment-Resistant Depression (TRD) poses a substantial health and economic challenge, persisting as a major concern despite decades of extensive research into novel treatment modalities. The considerable heterogeneity in TRD’s clinical manifestations and neurobiological bases has complicated efforts toward effective interventions. Recognizing the need for precise biomarkers to guide treatment choices in TRD, herein we introduce the SelecTool Project. This initiative focuses on developing (WorkPlane 1/WP1) and conducting preliminary validation (WorkPlane 2/WP2) of a computational tool (SelecTool) that integrates clinical data, neurophysiological (EEG) and peripheral (blood sample) biomarkers through a machine-learning framework designed to optimize TRD treatment protocols. The SelecTool project aims to enhance clinical decision-making by enabling the selection of personalized interventions. It leverages multi-modal data analysis to navigate treatment choices towards two validated therapeutic options for TRD: esketamine nasal spray (ESK-NS) and accelerated repetitive Transcranial Magnetic Stimulation (arTMS). In WP1, 100 subjects with TRD will be randomized to receive either ESK-NS or arTMS, with comprehensive evaluations encompassing neurophysiological (EEG), clinical (psychometric scales), and peripheral (blood samples) assessments both at baseline (T0) and one month post-treatment initiation (T1). WP2 will utilize the data collected in WP1 to train the SelecTool algorithm, followed by its application in a second, out-of-sample cohort of 20 TRD subjects, assigning treatments based on the tool’s recommendations. Ultimately, this research seeks to revolutionize the treatment of TRD by employing advanced machine learning strategies and thorough data analysis, aimed at unraveling the complex neurobiological landscape of depression. This effort is expected to provide pivotal insights that will promote the development of more effective and individually tailored treatment strategies, thus addressing a significant void in current TRD management and potentially reducing its profound societal and economic burdens.
It is imperative to improve our therapeutic strategies and provide optimal treatment options for depression. Major Depressive Disorder (MDD) is a substantial contributor to global disability, affecting more than 300 million people (1). Multiple lines of evidence suggest that MDD may stem from various pathophysiological changes (2), including disruptions in glutamatergic function (3). A significant challenge arises as approximately 30-50% of MDD patients exhibit inadequate responses to initial treatment approaches (4). Consequently, these individuals endure distressing symptoms for extended periods, with a significant portion developing treatment-resistant depression (TRD). TRD is operationally defined as the lack of a substantial therapeutic response after two antidepressant trials that are deemed adequate in both duration (specifically, a minimum of 4-6 weeks) and dosage (5). Studies have shown that individuals with TRD have reduced glutamate levels in prefrontal regions (6).
Recently, two rapid-acting interventions gained approval to address TRD: glutamatergic pharmacotherapies, such as esketamine nasal spray (ESK-NS), and non-invasive brain stimulation, specifically repetitive transcranial magnetic stimulation (arTMS), with accelerated protocols being able to exert similar antidepressant effectiveness to standard protocols with a reduced timeframe (7, 8). Both treatments require a significant time investment and are administered in specialized settings, but there is currently insufficient data guiding the choice between them. rTMS can locally modify cortical excitability in specific brain regions, inducing changes in brain circuits typically underactive in MDD (9). In contrast, ESK-NS acts on glutamatergic ionotropic N-methyl-D-aspartate (NMDA) receptors, transiently increasing glutamate release (10). The challenge of identifying personalized interventions for TRD and MDD remains a significant concern, with the absence of tools able to guide treatment selection as a prominent issue. Coupling effective treatments with suitable patients reduces costs, chronicity, and avoidable suffering (4).
Addressing the “treatment-selection” problem requires a deeper understanding of biomarkers in depression: objectively measurable characteristics reflecting underlying biological processes that contribute to heterogeneity of the MDD subtype and predict the therapeutic response (11–13). Resting-state electroencephalography (EEG), a neuroimaging technique known for its high temporal resolution, appears to be a promising approach for response prediction in depressive illness (14). It is a valuable tool to explore neural biomarkers associated with TRD, offering information on neural activity alterations and functional connectivity related to depression. Evidence suggests that EEG-derived biomarkers, such as alpha band asymmetry, altered EEG resting-state B microstate, or EEG functional connectivity patterns, could accurately help predict treatment outcomes (15–17).
Peripheral blood-based biomarkers, such as markers of systemic inflammation (including interleukines: IL-6, IL-8, IL-2p70) and hormone levels (thyroid-stimulating hormone, cortisol, norepinephrine) can also aid in subtyping MDD and predicting treatment response (18).
These biomarkers are easy to measure and have significant potential for practical implementation in routine clinical practice. Additionally, computational phenotyping, which generates research-grade profiles based on clinical presentation and computer-executable algorithms, contributes to a comprehensive understanding of personalized treatment approaches (19).
Machine learning (ML), a subset of artificial intelligence, encompasses diverse algorithms capable of building predictive models based on specific datasets (20). These algorithmic approaches aim to reveal fundamental principles underlying observations without explicit instructions, extracting structured knowledge from extensive datasets (21). A recent review demonstrates that ML technologies and data analytics can be applied at various stages of the patient journey, including detection and diagnosis, prognosis, treatment selection and optimization, outcome monitoring and tracking, and relapse prevention. Furthermore, data-driven ML approaches can identifying subtypes of symptoms and cognitive deficits, enabling model-based phenotyping (22). In this regard, significant progress has been made in the field of oncology. Specifically, it has become possible to robustly predict treatment responders and non-responders by using network-based biomarker expression levels in patients with melanoma, metastatic gastric cancer, and bladder cancer treated with immune checkpoint inhibitors targeting the programmed cell death 1/programmed cell death ligand 1 axis (23). On the other hand, integrating ML methods with extensive electronic health record databases has the potential to facilitate personalized psychiatry (22).
In this area, to fill the gap between the largely unmet needs of TRD and the enormous potential that has been opened by available innovations (e.g., neuroscience techniques, artificial intelligence methods, and advanced therapeutics), computerized tools can be developed, integrating clinical, neurophysiological, and peripheral data to guide treatment selection. These machine-learning methods could overcome the difficulty of treating TRD and its devastating consequences.
This study aims to develop and preliminarily validate a computational system that integrates clinical, electroencephalographical, and peripheral marker data, thus creating a tool to inform the treatment of Treatment-Resistant Depression (TRD) called “SelecTool”. This tool is designed to support clinical decision-making by helping select personalized, tailored interventions. Using a machine-learning analysis of multi-channel data, the SelecTool will guide treatment selection towards ESK-NS or arTMS. This manuscript delineates the study protocol of the SelecTool project, a translational, multicentric investigation encompassing two distinct phases that aim to develop a machine-learning based tool to help guide clinicians in managing TRD.
The project comprises two phases (Figure 1). The first (WP1; see Figure 1) involves the development of SelecTool for treatment orientation towards ESK-NS and arTMS by creating a machine-learning system. This phase includes:
• Prospective evaluation of clinical, electrophysiological, and peripheral biomarkers to predict the antidepressant response to ESK-NS and arTMS (n = 100).
• Integration of the above data with those previously collected from subjects with TRD treated with ESK-NS (n = 50) and arTMS (n = 50).
• Training a computerized system to develop a machine-learning-based tool that guides the treatment selection.
The subsequent stage (WP2; see Figure 1) focuses on the pilot validation of the ESK-NS and arTMS prescription using SelecTool, including the proof-of-concept estimation of SelecTool’s accuracy in an independent cohort (n = 20; out-of-sample validation). In this step, the identified biomarkers guiding treatment will be integrated into the SelecTool model as input data. Using the SelecTool’s output, individuals will undergo nonrandomized assignment to ESK-NS or arTMS interventions. Therefore, the accuracy in determining an increase in the number of responders to treatment will be estimated and compared with the response rates observed in random assignment.
One-hundred and twenty subjects (WP1: 100 subjects; WP2: 20 subjects) who are diagnosed with major depressive episode (both during the course of MDD or bipolar disorder) according to the Diagnostic and Statistical Manual of Mental Disorders, 5th edition (24), will be recruited by three Research Units: the ‘G. d’Annunzio’ University of Chieti, the University of Milano, and the Tor Vergata University of Rome.
The inclusion criteria will be the following: age between 18 and 65; current major depressive episode within at least the past month; TRD, defined as the absence of clinical response despite two or more treatments with antidepressants at adequate doses for 4-6 weeks (5); current stable psychopharmacological therapy for at least 1 month.
The exclusion criteria will be the following: presence of severe organic or neurological comorbidities, any substance use disorder (except nicotine dependence) in the past 6 months, intellectual disability or decline (Mini-Mental State Examination, MMSE < 26); uncontrolled systemic hypertension (specific for safety of ESK-NS treatment); presence of a positive history of seizures in the patient’s history or a first-degree relative (specific for arTMS safety); pregnant and postpartum women.
To refine the processing precision of the tool, we will consolidate the dataset from participants enrolled in the study with clinical data gathered retrospectively. These supplemental data will be obtained from a dedicated TRD dataset, which includes information from subjects who met identical inclusion and exclusion criteria and underwent prior treatment in our centers with ESK-NS (n = 50) and arTMS (n = 50).
The enrolled patients will undergo a comprehensive clinical examination as the initial step. Electrophysiological (EEG recordings) and peripheral biomarkers will be collected during this process. The administration of ESK-NS or arTMS will be determined according to the groupings of participants. Specifically, in the first phase, subjects will be randomly assigned to arTMS or ESK-NS. In the second phase, the extracted treatment-orienting biomarkers will be introduced in the SelecTool model as input data. Based on the SelecTool output, subjects will be assigned to the ESK-NS or arTMS interventions.
Baseline and 1-month follow-up assessments will include neuropsychological and psychiatric evaluations, behavioral assessments, neurophysiological data acquisition, and the collection of peripheral biomarkers.
After one month, the clinical response will be measured by a blind rater based on the MADRS score (> 50% reduction).
Subjects will undergo assessments at the screening visit (T0) and one month after initiation of treatment (T1) using a battery of validated psychometric tests (Table 1). At baseline, collection of anamnestic data will include sociodemographic factors, the history of depressive illness, treatment history for the current major depressive episode (MDE), comorbidities, lifetime antidepressant trials, augmentation strategies (such as the combined use of mood stabilizers, benzodiazepines, or antipsychotics), and other therapeutic interventions for treating treatment-resistant depression (TRD). These evaluations will be conducted by qualified psychiatrists, residents in psychiatry or clinical psychologists blinded to the treatment assignment. The primary outcome will be assessed in terms of clinical response, measured by the Montgomery-Åsberg Depression Rating Scale (MADRS; score reduction > 50%) (25). Patients will be evaluated for mood (Hamilton Depression Scale; Young Mania Rating Scale) (26), anhedonia (Snaith-Hamilton Pleasure Scale) (27), temperamental aspects (Temperament Evaluation of Memphis, Pisa and San Diego Autoquestionnaire version) (28); Barratt Impulsiveness Scale version 11; Big Five Questionnaire) (29, 30), anxiety (Hamilton Anxiety Scale) (31), alexithymia (Toronto Alexithymia Scale) (32), general psychiatric symptoms (Brief Psychiatric Rating Scale) (33), traumatic experiences (Childhood Trauma Questionnaire) (34), suicide risk (Columbia-Suicide Severity Rating Scale, Beck Hopelessness Scale) (35, 36), and resilience (Connor-Davidson Resilience Scale) (37). Health-related assessments will use the Clinical Global Impression-Severity scale (CGI-S) (38).
A comprehensive neuropsychological evaluation targeting various cognitive functions will be conducted for all patients. The assessment battery will primarily encompass measures of global cognition (MMSE) (39), attention (sustained spatial attention, Trail Making Test-A [TMT-A]; divided spatial attention, TMT-B; cognitive flexibility, TMT-AB) (40), short- and long-term episodic memory (Babcock Memory test) (41), and executive function (Frontal Assessment Battery) (42).
At T0 and T1, EEG electrical activity will be acquired utilizing a 64-channel EEG system (eego™mylab; ANT Neuro, Hengelo, Netherlands). Resting-state EEG will be recorded with eyes open and closed. Electrooculography and electrocardiography will also be acquired using additional electrodes. The data will undergo pre-processing to eliminate sections of poor quality and channels with unreliable data. Independent component analysis will be applied to eliminate periodic, non-brain signals. EEG analysis aims to identify pertinent and effective electro-neurophysiological biomarkers (at the channel/scalp, source, and source connectivity levels) indicative of treatment response in TRD (Table 2).
Blood samples (15 ml) will be collected at T0 and T1 by forearm venipuncture after an overnight fast. These samples will be stored in BD Vacutainer tubes containing ethylenediaminetetraacetic acid. Serum and plasma will be prepared by centrifugation at 1500 rpm for 10 minutes at 4°C. The serum will be stored in 0.5 ml Eppendorf tubes at -80°C until analysis.
Enzyme-linked immunosorbent assays (ELISAs) will be used to assess systemic inflammation and oxidative stress markers, including C-reactive protein, interleukin-1b (IL-1b), IL-5, IL-6, IL-8, and tumor necrosis factor-alpha (TNF-alpha). The levels of cortisol and adrenocorticotropic hormone will be determined using ELISA. Using specific monoclonal antibodies, the levels of TSH, FT3, and FT4 will be determined. Plasma brain-derived neurotrophic factor (BDNF) and proBDNF levels as biomarkers of synaptic integrity and plasticity will be investigated using ultra-sensitive high-performance single-molecule arrays or conventional ELISA.
During the first phase (WP1), subjects will be randomly assigned in a 1:1 ratio to receive arTMS or ESK-NS. A stratified randomization approach with a four-block size will be implemented to minimize inadvertent bias. Stratification factors will include sex (male, female), age (expected cutoff 50 years old), depression severity (mild/moderate depression, MADRS ≤ 34; severe depression, > 34), and treatment site (Chieti, Milan, and Rome). The randomization process will be carried out by an investigator external to the study.
In the pilot validation phase (WP2), the extracted treatment-guiding biomarkers will be incorporated into the SelecTool model as input data. Subsequently, subjects will be allocated to esketamine or arTMS interventions based on the output of the SelecTool.
All subjects will undergo a comprehensive preliminary visit to assess potential contraindications to treatments. Qualified medical personnel, specifically trained to handle potential side effects and emergencies related to treatments, will administer ESK-NS and arTMS treatments.
Subjects in the ESK-NS group will be administered the drug according to the EMA guidelines (49). It will be supplied in a double-use nasal spray device containing 200 μl of vehicle solution (two sprays), each delivering 28 mg (14 mg ESK-NS base per 100 μl of spray). This dose will be administered twice a week for the first week, followed by 84 mg (three devices) administered twice a week for three weeks, resulting in a total of 1 month of treatment. Before initial administration, patients will be instructed to blow their nose (only before the first device is administered) and then assisted to recline their head 45° (semi-reclined position) during administration to enhance retention of the medication within the nasal cavity. Each ESK-NS session will be conducted by qualified personnel who closely monitor vital parameters (blood pressure, heart rate) before and at 45 and 90 minutes after treatment, following international safety guidelines (50).
Patients in the arTMS group will undergo a 5-day arTMS protocol involving four daily sessions (8). This protocol, developed following the safety guidelines (51) and the principles of accelerated protocols (52), aims to deliver the same number of magnetic pulses as the FDA-approved protocol (53). Stimulation will be performed using a MagPro R30 (MagVenture) system with a B-70 coil targeting the left dorsolateral prefrontal cortex (L-DLPFC), a region approved for TRD treatment (53). The L-DLPFC will be identified using the BEAM F3 method (54), facilitating rapid localization through anthropometric measures. The resting motor threshold will be determined using the evoked potential motor method (55). Each session will adhere to the following parameters, aligning with the FDA-approved standard (53): 10 Hz frequency, 120% resting motor threshold, 40 pulses/train (4 s duration), 26 s inter-train interval, 3000 pulses/session, and a total duration of 35 minutes. This session will be repeated four times within the same day, with a 55-minute interval between sessions (total duration of the cycle session pause: 90 minutes), thus adhering to the accelerated stimulation protocol. The entire protocol will take approximately 5 hours and 5 minutes. Throughout this time, patients will be continuously monitored for side effects. The onset of potential side effects will be evaluated at each stimulation session using a specific and approved scale for rTMS-related side effects (56).
Drawing from the existing literature on the efficacy of arTMS and ESK-NS for Treatment-Resistant Depression (TRD), we anticipate a response rate of approximately 50% for each treatment (52, 57). The sample size was determined using the G*Power 3.1 software, taking into account specific parameters: a substantial effect size of predictors (expected Cohen effect size F = 0.4), power 1-beta = 0.80, one-way, four groups (2x2; treatment: ESK-NS, arTMS; responders and non-responders), and a significance level corrected for multiple comparisons (alpha = 0.001). These calculations resulted in a total sample size of n = 144. Considering a possible imbalance in the allocation of responders (10%) and to mitigate possible dropouts (10%), we increased the total sample size to n = 200.
We will develop a machine-learning model to predict the primary treatment outcome and use it for treatment guidance. This model will leverage both neurophysiological data and clinical scores. We also aim to interpret the model and extract the features that influence it. Given the heterogeneous nature of the collected data, an appropriate solution is to opt for ensemble methods, particularly random forest techniques, which have shown suitability for such tasks (19, p. 202) and are relevant for post hoc analysis of results. To provide comprehensive insights, our exploration will not be restricted to random forest techniques; we will also investigate other approaches such as neural networks or support vector classifiers. Dealing with missing values in clinical and psychometric tests is a critical concern, and we will address this using advanced techniques such as multivariate imputation (58, 59). The model parameters and performance will be assessed using nested and shuffle cross-validation, which is recognized as optimal to minimize bias in model error estimations (60). The results’ significance will be evaluated using permutation tests, which are acknowledged as the gold standard for statistical assessments of machine-learning algorithms (61).
This study has received approval from a local Institutional Review Board (C.Et.R.A., approval number: 6/2023). It will follow the principles and recommendations of Good Clinical Practice and the Declaration of Helsinki (World Medical Association, 2013), which offer guidance to physicians engaged in biomedical research with human subjects. The patient will sign the informed consent form, which will be witnessed, dated, and retained by the investigator responsible for recruiting patients into the study.
This research proposal is designed to spearhead an innovative methodology for enhancing clinical decision-making processes in the context of TRD. The aim is to develop and preliminarily validate an advanced computational framework that adeptly consolidates clinical assessments, peripheral biomarkers, and EEG data to address the selection of advanced treatment for TRD. This integration aims to predict treatment outcomes precisely (62), thus facilitating the tailored orientation of therapeutic strategies that reduce unnecessary suffering. To construct this pivotal tool for TRD treatment optimization, we plan to utilize a machine learning algorithm capable of processing complex, multi-dimensional data streams (18, 63).
Machine learning has been employed in the medical sector since the late 1990s, notably in oncology – a principal area of application (20). Within this field, a critical challenge involves the identification of markers that can accurately predict drug responses among diverse groups of cancer patients. A recent study introduced a network-based machine-learning framework capable of generating robust predictions across immune checkpoint inhibitor datasets and pinpointing potential biomarkers (23).
In the area of clinical neurosciences, there is significant potential for benefit from these technological advances, especially considering the nuanced presentation of symptoms characteristic of neurological disorders. A study conducted in 2022 focused on the use of machine learning algorithms to classify subtypes of immune microenvironment and identify unique genes in Alzheimer’s disease. This research highlighted five immune microenvironment-related genes that strongly correlate with pathological markers and reliably predict the disease’s trajectory (64).
The field of psychiatry has also seen considerable advancements through pioneering research efforts. A recent multicenter study applied multimodal machine learning methods, integrating clinical, neurocognitive, structural magnetic resonance imaging, and polygenic risk scores to predict the onset of psychosis in individuals at high clinical risk or with recent-onset depression (65). Furthermore, a recent narrative review investigated the application of machine learning in diagnosing and forecasting schizophrenia, concluding that various machine learning-based models can potentially help healthcare professionals in diagnosing the condition and predicting its clinical presentations and complications (66).
Concerning TRD, a very recent machine learning study has highlighted that characteristics such as profound anhedonia, anxious distress, mixed symptoms, and bipolarity in patients treated with ESK-NS represent factors that predict a positive response and remission. In contrast, the use of benzodiazepines and the severity of depression were associated with delayed responses (67). The levels of accuracy achieved with data exclusively symptom-based do not allow for incorporation into clinical practice and justify the attempt of the SelecTool Project to refine selection methods by integrating other biomarkers.
Given the substantial global health impact of TRD, which doubles the risk of hospitalization and increases the risk of suicide sevenfold compared to treatment-responsive depressed patients (68) our primary objective is to identify treatment approaches that optimize patients’ prospects for recovery. On the one hand, arTMS is a proven intervention for TRD, strongly supported by existing literature, demonstrating response rates of 40–50% and remission rates of 25–30%. (52, 69). On the other hand, in patients treated with ESK-NS, the percentage of remitters has been observed to be less than half (70).
As a result, despite the established antidepressant efficacy of ESK-NS and arTMS, achieving clinical response rates of approximately 50-60% even in real-world studies (8, 71–75), there remains a notable gap in our understanding of their response biomarkers. This proposal is also set to significantly expand our understanding of the complex and heterogeneous nature of the pathophysiology and treatment of MDD. Viewing MDD through the lens of brain connectivity disorders highlights its varied neurobiological foundations, likely related to disparate brain network functionalities (76). Such neurobiological diversity leads to distinct MDD subtypes, each with its unique treatment response profile, particularly to neuromodulation and glutamatergic interventions.
By deepening our understanding of the biomarkers associated with various depression subtypes, including clinical, EEG, and peripheral indicators, we aim to pioneer a patient-centered approach to treatment selection. Given the substantial social, occupational, and physical repercussions associated with TRD, not to mention the increased healthcare costs that make TRD a significant economic burden on healthcare systems (68, p. 201; 77, 78), this research has the potential for considerable social and economic benefits.
In conclusion, this research proposal not only aims to change the approach to treating TRD by leveraging cutting-edge machine learning techniques and comprehensive data analysis, but also aims to shed light on the intricate landscape of the neurobiological underpinnings of depression. Through this endeavor, we anticipate contributing valuable insights that could influence and offer potential advantages for clinical practice, facilitating the development of more effective and personalized treatment regimens. This approach addresses a critical gap in the current management of TRD and potentially alleviating its significant societal and economic impacts.
The original contributions presented in the study are included in the article/supplementary material. Further inquiries can be directed to the corresponding author/s.
The studies involving humans were approved by Comitato Etico Regione Abruzzo (C.Et.R.A.). The studies were conducted in accordance with the local legislation and institutional requirements. The participants provided their written informed consent to participate in this study.
MP: Writing – original draft, Writing – review & editing. GD: Writing – original draft, Writing – review & editing. BB: Writing – original draft, Writing – review & editing. Gd: Writing – original draft, Writing – review & editing. CC: Writing – original draft, Writing – review & editing. RC: Writing – original draft, Writing – review & editing. GM(7th author): Writing – original draft, Writing – review & editing. OD: Writing – original draft, Writing – review & editing. GM(9th author): Writing – original draft, Writing – review & editing. AD(10th author): Writing – original draft, Writing – review & editing. EB: Writing – original draft, Writing – review & editing. IR: Writing – original draft, Writing – review & editing. AC: Writing – original draft, Writing – review & editing. LP: Writing – original draft, Writing – review & editing. GB: Writing – original draft, Writing – review & editing. MP: Writing – original draft, Writing – review & editing. RG: Writing – original draft, Writing – review & editing. AD(18th author): Writing – original draft, Writing – review & editing. LM: Writing – original draft, Writing – review & editing. FZ: Writing – original draft, Writing – review & editing. BD: Writing – original draft, Writing – review & editing. GM(22nd author): Writing – original draft, Writing – review & editing.
The author(s) declare financial support was received for the research, authorship, and/or publication of this article. This study was supported by the PRIN Research Grant (Code: D53D23013400006).
The authors declare that the research was conducted in the absence of any commercial or financial relationships that could be construed as a potential conflict of interest.
The author(s) declared that they were an editorial board member of Frontiers, at the time of submission. This had no impact on the peer review process and the final decision.
All claims expressed in this article are solely those of the authors and do not necessarily represent those of their affiliated organizations, or those of the publisher, the editors and the reviewers. Any product that may be evaluated in this article, or claim that may be made by its manufacturer, is not guaranteed or endorsed by the publisher.
2. Papp M, Cubała WJ, Swiecicki L, Newman-Tancredi A, Willner P. Perspectives for therapy of treatment-resistant depression. Br J Pharmacol. (2022) 179:4181–200. doi: 10.1111/bph.15596
3. Aleksandrova LR, Phillips AG, Wang YT. Antidepressant effects of ketamine and the roles of AMPA glutamate receptors and other mechanisms beyond NMDA receptor antagonism. J Psychiatry Neurosci. (2017) 42:222–9. doi: 10.1503/jpn.160175
4. McIntyre RS, Alsuwaidan M, Baune BT, Berk M, Demyttenaere K, Goldberg JF, et al. Treatment-resistant depression: Definition, prevalence, detection, management, and investigational interventions. World Psychiatry. (2023) 22:394–412. doi: 10.1002/wps.21120
5. Sforzini L, Worrell C, Kose M, Anderson IM, Aouizerate B, Arolt V, et al. A Delphi-method-based consensus guideline for definition of treatment-resistant depression for clinical trials. Mol Psychiatry. (2022) 27:1286–99. doi: 10.1038/s41380-021-01381-x
6. Kim Y-K, Na K-S. Role of glutamate receptors and glial cells in the pathophysiology of treatment-resistant depression. Prog Neuropsychopharmacol Biol Psychiatry. (2016) 70:117–26. doi: 10.1016/j.pnpbp.2016.03.009
7. Zheng W, Zhang X-Y, Xu R, Huang X, Zheng Y-J, Huang X-B, et al. Adjunctive accelerated repetitive transcranial magnetic stimulation for older patients with depression: A systematic review. Front Aging Neurosci. (2022) 14:1036676. doi: 10.3389/fnagi.2022.1036676
8. Pettorruso M, d’Andrea G, Di Carlo F, De Risio L, Zoratto F, Miuli A, et al. Comparing fast-acting interventions for treatment-resistant depression: An explorative study of accelerated HF-rTMS versus intranasal esketamine. Brain Stimulation: Basic Translational Clin Res Neuromodulation. (2023) 16:1041–3. doi: 10.1016/j.brs.2023.06.003
9. Chou P-H, Lin Y-F, Lu M-K, Chang H-A, Chu C-S, Chang WH, et al. Personalization of repetitive transcranial magnetic stimulation for the treatment of major depressive disorder according to the existing psychiatric comorbidity. Clin Psychopharmacol Neuroscience. (2021) 19:190–205. doi: 10.9758/cpn.2021.19.2.190
10. D’Andrea G, Pettorruso M, Lorenzo GD, Mancusi G, McIntyre RS, Martinotti G. Rethinking ketamine and esketamine action: Are they antidepressants with mood-stabilizing properties? Eur Neuropsychopharmacol. (2023) 70:49–55. doi: 10.1016/j.euroneuro.2023.02.010
11. Drysdale AT, Grosenick L, Downar J, Dunlop K, Mansouri F, Meng Y, et al. Resting-state connectivity biomarkers define neurophysiological subtypes of depression. Nat Med. (2017) 23:28–38. doi: 10.1038/nm.4246
12. Gadad BS, Jha MK, Czysz A, Furman JL, Mayes TL, Emslie MP, et al. Peripheral biomarkers of major depression and antidepressant treatment response: Current knowledge and future outlooks. J Affect Disord. (2018) 233:3–14. doi: 10.1016/j.jad.2017.07.001
13. D’Onofrio AM, Pizzuto DA, Batir R, Perrone E, Cocciolillo F, Cavallo F, et al. Dopaminergic dysfunction in the left putamen of patients with major depressive disorder. J Affect Disord. (2024) 357:107–15. doi: 10.1016/j.jad.2024.04.044
14. Widge AS, Bilge MT, Montana R, Chang W, Rodriguez CI, Deckersbach T, et al. Electroencephalographic biomarkers for treatment response prediction in major depressive illness: A meta-analysis. Am J Psychiatry. (2019) 176:44–56. doi: 10.1176/appi.ajp.2018.17121358
15. Arns M, Bruder G, Hegerl U, Spooner C, Palmer DM, Etkin A, et al. EEG alpha asymmetry as a gender-specific predictor of outcome to acute treatment with different antidepressant medications in the randomized iSPOT-D study. Clin Neurophysiol. (2016) 127:509–19. doi: 10.1016/j.clinph.2015.05.032
16. Vellante F, Ferri F, Baroni G, Croce P, Migliorati D, Pettoruso M, et al. Euthymic bipolar disorder patients and EEG microstates: A neural signature of their abnormal self experience? J Affect Disord. (2020) 272:326–34. doi: 10.1016/j.jad.2020.03.175
17. Benschop L, Vanhollebeke G, Li J, Leahy RM, Vanderhasselt M-A, Baeken C. Reduced subgenual cingulate-dorsolateral prefrontal connectivity as an electrophysiological marker for depression. Sci Rep. (2022) 12:16903. doi: 10.1038/s41598-022-20274-9
18. Jani BD, McLean G, Nicholl BI, Barry SJE, Sattar N, Mair FS, et al. Risk assessment and predicting outcomes in patients with depressive symptoms: A review of potential role of peripheral blood based biomarkers. Front Hum Neurosci. (2015) 9:18. doi: 10.3389/fnhum.2015.00018
19. Dadi K, Varoquaux G, Houenou J, Bzdok D, Thirion B, Engemann D. Population modeling with machine learning can enhance measures of mental health. GigaScience. (2021) 10. doi: 10.1093/gigascience/giab071
20. Nichols JA, Herbert Chan HW, Baker MAB. Machine learning: Applications of artificial intelligence to imaging and diagnosis. Biophys Rev. (2019) 11:111–8. doi: 10.1007/s12551-018-0449-9
21. Bzdok D, Altman N, Krzywinski M. Statistics versus machine learning. Nat Methods. (2018) 15:233–4. doi: 10.1038/nmeth.4642
22. Chen ZS, Kulkarni P, Galatzer-Levy IR, Bigio B, Nasca C, Zhang Y. Modern views of machine learning for precision psychiatry. Patterns. (2022) 3:100602. doi: 10.1016/j.patter.2022.100602
23. Kong J, Ha D, Lee J, Kim I, Park M, Im S-H, et al. Network-based machine learning approach to predict immunotherapy response in cancer patients. Nat Commun. (2022) 13:3703. doi: 10.1038/s41467-022-31535-6
24. APA. Diagnostic and Statistical Manual. Arlington: American Psychiatric Association (2013). doi: 10.1176/appi.books.9780890425596
25. Montgomery SA, Asberg M. A new depression scale designed to be sensitive to change. Br J Psychiatry : J Ment Sci. (1979) 134:382–9. doi: 10.1192/bjp.134.4.382
26. Hamilton M. A rating scale for depression. J Neurology Neurosurgery Psychiatry. (1960) 23:56–62. doi: 10.1136/jnnp.23.1.56
27. Snaith RP, Hamilton M, Morley S, Humayan A, Hargreaves D, Trigwell P. A scale for the assessment of hedonic tone the Snaith-Hamilton Pleasure Scale. Br J Psychiatry : J Ment Sci. (1995) 167:99–103. doi: 10.1192/bjp.167.1.99
28. Elias LR, Köhler CA, Stubbs B, Maciel BR, Cavalcante LM, Vale AMO, et al. Measuring affective temperaments: A systematic review of validation studies of the Temperament Evaluation in Memphis Pisa and San Diego (TEMPS) instruments. J Affect Disord. (2017) 212:25–37. doi: 10.1016/j.jad.2017.01.023
29. Reise SP, Moore TM, Sabb FW, Brown AK, London ED. The Barratt Impulsiveness Scale-11: Reassessment of its structure in a community sample. psychol Assess. (2013) 25:631–42. doi: 10.1037/a0032161
30. Widiger TA, Crego C. The Five Factor Model of personality structure: An update. World Psychiatry : Off J World Psychiatr Assoc (WPA). (2019) 18:271–2. doi: 10.1002/wps.20658
31. Hamilton M. The assessment of anxiety states by rating. Br J Med Psychol. (1959) 32:50–5. doi: 10.1111/j.2044-8341.1959.tb00467.x
32. Bagby M, Taylor GJ, Ryan D. Toronto alexithymia scale: relationship with personality and psychopathology measures. Psychother Psychosomatics. (2010) 45:207–15. doi: 10.1159/000287950
33. Zanello A, Berthoud L, Ventura J, Merlo MCG. The Brief Psychiatric Rating Scale (version 4.0) factorial structure and its sensitivity in the treatment of outpatients with unipolar depression. Psychiatry Res. (2013) 210:626–33. doi: 10.1016/j.psychres.2013.07.001
34. Bernstein DP, Stein JA, Newcomb MD, Walker E, Pogge D, Ahluvalia T, et al. Development and validation of a brief screening version of the Childhood Trauma Questionnaire. Child Abuse Negl. (2003) 27:169–90. doi: 10.1016/S0145-2134(02)00541-0
35. Salvi J. Calculated decisions: columbia-suicide severity rating scale (C-SSRS). Emergency Med Pract. (2019) 21:CD3–4.
36. Pettorruso M, D’Andrea G, Martinotti G, Cocciolillo F, Miuli A, Di Muzio I, et al. Hopelessness, dissociative symptoms, and suicide risk in major depressive disorder: clinical and biological correlates. Brain Sci. (2020) 10. doi: 10.3390/brainsci10080519
37. Connor KM, Davidson JRT. Development of a new resilience scale: The Connor-Davidson Resilience Scale (CD-RISC). Depression Anxiety. (2003) 18:76–82. doi: 10.1002/(ISSN)1520-6394
38. Busner J, Targum SD. The clinical global impressions scale: Applying a research tool in clinical practice. Psychiatry (Edgmont (Pa.: Township)). (2007) 4:28–37.
39. Measso G, Cavarzeran F, Zappalà G, Lebowitz BD, Crook TH, Pirozzolo FJ, et al. The mini-mental state examination: Normative study of an Italian random sample. Dev Neuropsychol. (1993) 9:77–85. doi: 10.1080/87565649109540545
40. Giovagnoli AR, Del Pesce M, Mascheroni S, Simoncelli M, Laiacona M, Capitani E. Trail making test: Normative values from 287 normal adult controls. Ital J Neurological Sci. (1996) 17:305–9. doi: 10.1007/BF01997792
41. Carlesimo GA, Caltagirone C, Gainotti G. The Mental Deterioration Battery: Normative data, diagnostic reliability and qualitative analyses of cognitive impairment. The Group for the Standardization of the Mental Deterioration Battery. Eur Neurol. (1996) 36:378–84. doi: 10.1159/000117297
42. Appollonio I, Leone M, Isella V, Piamarta F, Consoli T, Villa ML, et al. The Frontal Assessment Battery (FAB): Normative values in an Italian population sample. Neurological Sci. (2005) 26:108–16. doi: 10.1007/s10072-005-0443-4
43. Coan JA, Allen JJB. Frontal EEG asymmetry as a moderator and mediator of emotion. Biol Psychol. (2004) 67:7–49. doi: 10.1016/j.biopsycho.2004.03.002
44. Nusslock R, Walden K, Harmon-Jones E. Asymmetrical frontal cortical activity associated with differential risk for mood and anxiety disorder symptoms: An RDoC perspective. Int J Psychophysiology. (2015) 98:249–61. doi: 10.1016/j.ijpsycho.2015.06.004
45. Murphy M, Whitton AE, Deccy S, Ironside ML, Rutherford A, Beltzer M, et al. Abnormalities in electroencephalographic microstates are state and trait markers of major depressive disorder. Neuropsychopharmacology. (2020) 45:2030–7. doi: 10.1038/s41386-020-0749-1
46. Pizzagalli DA, Webb CA, Dillon DG, Tenke CE, Kayser J, Goer F, et al. Pretreatment rostral anterior cingulate cortex theta activity in relation to symptom improvement in depression: A randomized clinical trial. JAMA Psychiatry. (2018) 75:547–54. doi: 10.1001/jamapsychiatry.2018.0252
47. Fox MD, Buckner RL, White MP, Greicius MD, Pascual-Leone A. Efficacy of transcranial magnetic stimulation targets for depression is related to intrinsic functional connectivity with the subgenual cingulate. Biol Psychiatry. (2012) 72:595–603. doi: 10.1016/j.biopsych.2012.04.028
48. Rolle CE, Fonzo GA, Wu W, Toll R, Jha MK, Cooper C, et al. Cortical connectivity moderators of antidepressant vs placebo treatment response in major depressive disorder: secondary analysis of a randomized clinical trial. JAMA Psychiatry. (2020) 77:397–408. doi: 10.1001/jamapsychiatry.2019.3867
50. McIntyre RS, Rosenblat JD, Nemeroff CB, Sanacora G, Murrough JW, Berk M, et al. Synthesizing the evidence for ketamine and esketamine in treatment-resistant depression: An international expert opinion on the available evidence and implementation. Am J Psychiatry. (2021) 178:383–99. doi: 10.1176/appi.ajp.2020.20081251
51. Rossi S, Antal A, Bestmann S, Bikson M, Brewer C, Brockmöller J, et al. Safety and recommendations for TMS use in healthy subjects and patient populations, with updates on training, ethical and regulatory issues: Expert Guidelines. Clin Neurophysiol. (2021) 132:269–306. doi: 10.1016/j.clinph.2020.10.003
52. Miron J-P, Jodoin VD, Lespérance P, Blumberger DM. Repetitive transcranial magnetic stimulation for major depressive disorder: Basic principles and future directions. Ther Adv Psychopharmacol. (2021) 11:20451253211042696. doi: 10.1177/20451253211042696
53. McClintock SM, Reti IM, Carpenter LL, McDonald WM, Dubin M, Taylor SF, et al. Consensus recommendations for the clinical application of repetitive transcranial magnetic stimulation (rTMS) in the treatment of depression. J Clin Psychiatry. (2018) 79, Fascicolo 1. doi: 10.4088/JCP.16cs10905
54. Beam W, Borckardt JJ, Reeves ST, George MS. An efficient and accurate new method for locating the F3 position for prefrontal TMS applications. Brain Stimulation. (2009) 2:50–4. doi: 10.1016/j.brs.2008.09.006
55. Bestmann S, Krakauer JW. The uses and interpretations of the motor-evoked potential for understanding behaviour. Exp Brain Res. (2015) 233:679–89. doi: 10.1007/s00221-014-4183-7
56. Rossi S, Hallett M, Rossini PM, Pascual-Leone A, Avanzini G, Bestmann S, et al. Safety, ethical considerations, and application guidelines for the use of transcranial magnetic stimulation in clinical practice and research. Clin Neurophysiol. (2009) 120:2008–39. doi: 10.1016/j.clinph.2009.08.016
57. Jones RR, Freeman MP, Kornstein SG, Cooper K, Daly EJ, Canuso CM, et al. Efficacy and safety of esketamine nasal spray by sex in patients with treatment-resistant depression: Findings from short-term randomized, controlled trials. Arch Women’s Ment Health. (2022) 25:313–26. doi: 10.1007/s00737-021-01185-6
58. Josse J, Prost N, Scornet E, Varoquaux G. On the consistency of supervised learning with missing values. (2019). pp. 1–43.
59. Little R, Rubin D. Statistical Analysis with Missing Data. 3rd ed. Wiley Online Library (2019). doi: 10.1002/SERIES1345
60. Varoquaux G. Cross-validation failure: Small sample sizes lead to large error bars. NeuroImage. (2018) 180:68–77. doi: 10.1016/j.neuroimage.2017.06.061
61. Combrisson E, Jerbi K. Exceeding chance level by chance: The caveat of theoretical chance levels in brain signal classification and statistical assessment of decoding accuracy. J Neurosci Methods. (2015) 250:126–36. doi: 10.1016/j.jneumeth.2015.01.010
62. Marzetti L, Basti A, Chella F, D’Andrea A, Syrjälä J, Pizzella V. Brain functional connectivity through phase coupling of neuronal oscillations: A perspective from magnetoencephalography. Front Neurosci. (2019) 13:964. doi: 10.3389/fnins.2019.00964
63. Beijers L, Wardenaar KJ, van Loo HM, Schoevers RA. Data-driven biological subtypes of depression: Systematic review of biological approaches to depression subtyping. Mol Psychiatry. (2019) 24:888–900. doi: 10.1038/s41380-019-0385-5
64. Lai Y, Lin P, Lin F, Chen M, Lin C, Lin X, et al. Identification of immune microenvironment subtypes and signature genes for Alzheimer’s disease diagnosis and risk prediction based on explainable machine learning. Front Immunol. (2022) 13:1046410. doi: 10.3389/fimmu.2022.1046410
65. Koutsouleris N, Dwyer DB, Degenhardt F, Maj C, Urquijo-Castro MF, Sanfelici R, et al. Multimodal machine learning workflows for prediction of psychosis in patients with clinical high-Risk syndromes and recent-Onset depression. JAMA Psychiatry. (2021) 78:195–209. doi: 10.1001/jamapsychiatry.2020.3604
66. Gashkarimov VR, Sultanova RI, Efremov IS, Asadullin AR. Machine learning techniques in diagnostics and prediction of the clinical features of schizophrenia: A narrative review. Consortium Psychiatricum. (2023) 4:43–53. doi: 10.17816/CP.202343
67. Pettorruso M, Guidotti R, d’Andrea G, De Risio L, D’Andrea A, Chiappini S, et al. Predicting outcome with Intranasal Esketamine treatment: A machine-learning, three-month study in Treatment-Resistant Depression (ESK-LEARNING). Psychiatry Res. (2023) 327:115378. doi: 10.1016/j.psychres.2023.115378
68. Amos TB, Tandon N, Lefebvre P, Pilon D, Kamstra RL, Pivneva I, et al. Direct and indirect cost burden and change of employment status in treatment-resistant depression: A matched-cohort study using a US commercial claims database. J Clin Psychiatry. (2018) 79. doi: 10.4088/JCP.17m11725
69. D’Andrea G, Mancusi G, Santovito MC, Marrangone C, Martino F, Santorelli M, et al. Investigating the role of maintenance TMS protocols for major depression: systematic review and future perspectives for personalized interventions. J Personalized Med. (2023) 13. doi: 10.3390/jpm13040697
70. Sapkota A, Khurshid H, Qureshi IA, Jahan N, Went TR, Sultan W, et al. Efficacy and safety of intranasal esketamine in treatment-resistant depression in adults: A systematic review. Cureus. (2021) 13. doi: 10.7759/cureus.17352
71. Chiappini S, D’Andrea G, De Filippis S, Di Nicola M, Andriola I, Bassetti R, et al. Esketamine in treatment-resistant depression patients comorbid with substance-use disorder: A viewpoint on its safety and effectiveness in a subsample of patients from the REAL-ESK study. Eur Neuropsychopharmacol. (2023) 74:15–21. doi: 10.1016/j.euroneuro.2023.04.011
72. d’Andrea G, Chiappini S, McIntyre RS, Stefanelli G, Carullo R, Andriola I, et al. ). Investigating the effectiveness and tolerability of intranasal esketamine among older adults with treatment-resistant depression (TRD): A post-hoc analysis from the REAL-ESK study group. Am J Geriatric Psychiatry. (2023). doi: 10.1016/j.jagp.2023.06.016
73. d’Andrea G, Pettorruso M, Di Lorenzo G, Rhee TG, Chiappini S, Carullo R, et al. The rapid antidepressant effectiveness of repeated dose of intravenous ketamine and intranasal esketamine: A post-hoc analysis of pooled real-world data. J Affect Disord. (2024) 348:314–22. doi: 10.1016/j.jad.2023.12.038
74. Martinotti G, Vita A, Fagiolini A, Maina G, Bertolino A, Dell’Osso B, et al. Real-world experience of esketamine use to manage treatment-resistant depression: A multicentric study on safety and effectiveness (REAL-ESK study). J Affect Disord. (2022) 319:646–54. doi: 10.1016/j.jad.2022.09.043
75. Martinotti G, Dell’Osso B, Di Lorenzo G, Maina G, Bertolino A, Clerici M, et al. Treating Bipolar Depression with Esketamine: Safety and Effectiveness data from a naturalistic multicentric study on Esketamine in Bipolar versus Unipolar Treatment-Resistant Depression. Bipolar Disord. (2023). doi: 10.1111/bdi.13296
76. Li BJ, Friston K, Mody M, Wang HN, Lu HB, Hu DW. A brain network model for depression: From symptom understanding to disease intervention. CNS Neurosci Ther. (2018) 24:1004–19. doi: 10.1111/cns.12998
77. Perrone V, Sangiorgi D, Andretta M, Ducci G, Forti B, Francesa Morel PC, et al. Healthcare resource consumption and related costs of patients estimated with treatment-resistant depression in Italy. ClinicoEconomics Outcomes Res: CEOR. (2021) 13:629–35. doi: 10.2147/CEOR.S314111
Keywords: transcranial magnetic stimulation (rTMS), esketamine nasal spray, machine-learning (ML) algorithms, treatment resistant depression (TRD), endophenotypes
Citation: Pettorruso M, Di Lorenzo G, Benatti B, d’Andrea G, Cavallotto C, Carullo R, Mancusi G, Di Marco O, Mammarella G, D’Attilio A, Barlocci E, Rosa I, Cocco A, Padula LP, Bubbico G, Perrucci MG, Guidotti R, D’Andrea A, Marzetti L, Zoratto F, Dell’Osso BM and Martinotti G (2024) Overcoming treatment-resistant depression with machine-learning based tools: a study protocol combining EEG and clinical data to personalize glutamatergic and brain stimulation interventions (SelecTool Project). Front. Psychiatry 15:1436006. doi: 10.3389/fpsyt.2024.1436006
Received: 21 May 2024; Accepted: 01 July 2024;
Published: 17 July 2024.
Edited by:
Michele Fornaro, University of Naples Federico II, ItalyReviewed by:
Mariusz Stanisław Wiglusz, Medical University of Gdansk, PolandCopyright © 2024 Pettorruso, Di Lorenzo, Benatti, d’Andrea, Cavallotto, Carullo, Mancusi, Di Marco, Mammarella, D’Attilio, Barlocci, Rosa, Cocco, Padula, Bubbico, Perrucci, Guidotti, D’Andrea, Marzetti, Zoratto, Dell’Osso and Martinotti. This is an open-access article distributed under the terms of the Creative Commons Attribution License (CC BY). The use, distribution or reproduction in other forums is permitted, provided the original author(s) and the copyright owner(s) are credited and that the original publication in this journal is cited, in accordance with accepted academic practice. No use, distribution or reproduction is permitted which does not comply with these terms.
*Correspondence: Giacomo d’Andrea, Z2lhY29tby5kYW5kcmVhMTk5M0BnbWFpbC5jb20=
Disclaimer: All claims expressed in this article are solely those of the authors and do not necessarily represent those of their affiliated organizations, or those of the publisher, the editors and the reviewers. Any product that may be evaluated in this article or claim that may be made by its manufacturer is not guaranteed or endorsed by the publisher.
Research integrity at Frontiers
Learn more about the work of our research integrity team to safeguard the quality of each article we publish.