- 1Department of Neuroscience, University of Padova, Padua, Italy
- 2Department of Psychiatry and Psychology, Mayo Clinic, Rochester, MN, United States
- 3Bipolar and Depressive Disorders Unit, Hospital Clinic de Barcelona, Barcelona, Spain
- 4Institut d’Investigacions Biomediques August Pi i Sunyer (IDIBAPS), University of Barcelona, Barcelona, Spain
- 5Centro de Investigación Biomédica en Red de Salud Mental (CIBERSAM), Instituto de Salud Carlos III, Madrid, Spain
- 6Department of Medicine, Faculty of Medicine and Health Sciences, Institute of Neurosciences (UBNeuro), University of Barcelona (UB), Barcelona, Spain
- 7Departament de Medicina, Facultat de Medicina i Ciències de la Salut, Universitat de Barcelona (UB), Barcelona, Spain
- 8Department of Psychiatry, University of Utah, Salt Lake City, UT, United States
- 9Department of Psychiatry, Federico II University of Naples, Naples, Italy
- 10SCIENCES lab, Department of Psychiatry, University of Ottawa, Ottawa, ON, Canada
- 11Department of Mental Health, The Ottawa Hospital, Ottawa, ON, Canada
- 12Ottawa Hospital Research Institute (OHRI) Clinical Epidemiology Program University of Ottawa, Ottawa, ON, Canada
- 13School of Epidemiology and Public Health, Faculty of Medicine, University of Ottawa, Ottawa, ON, Canada
- 14Department of Child and Adolescent Psychiatry, Charité Universitätsmedizin, Berlin, Germany
Objective: Bipolar Disorder (BD) is a severe mental illness associated with high rates of general medical comorbidity, reduced life expectancy, and premature mortality. Although BD has been associated with high medical hospitalization, the factors that contribute to this risk remain largely unexplored. We used baseline medical and psychiatric records to develop a supervised machine learning model to predict general medical admissions after discharge from psychiatric hospitalization.
Methods: In this retrospective three-year cohort study of 71 patients diagnosed with BD (mean age=52.19 years, females=56.33%), lasso regression models combining medical and psychiatric records, as well as those using them separately, were fitted and their predictive power was estimated using a leave-one-out cross-validation procedure.
Results: The proportion of medical admissions in patients with BD was higher compared with age- and sex-matched hospitalizations in the same region (25.4% vs. 8.48%). The lasso model fairly accurately predicted the outcome (area under the curve [AUC]=69.5%, 95%C.I.=55–84.1; sensitivity=61.1%, specificity=75.5%, balanced accuracy=68.3%). Notably, pre-existing cardiovascular, neurological, or osteomuscular diseases collectively accounted for more than 90% of the influence on the model. The accuracy of the model based on medical records was slightly inferior (AUC=68.7%, 95%C.I. = 54.6-82.9), while that of the model based on psychiatric records only was below chance (AUC=61.8%, 95%C.I.=46.2–77.4).
Conclusion: Our findings support the need to monitor medical comorbidities during clinical decision-making to tailor and implement effective preventive measures in people with BD. Further research with larger sample sizes and prospective cohorts is warranted to replicate these findings and validate the predictive model.
1 Introduction
Bipolar Disorder (BD) is a severe mental illness that causes significant disability worldwide (1, 2). BD is associated with strikingly high rates of general medical comorbidities (above 90% of cases endorse at least one comorbidity), especially cardiovascular, metabolic, endocrine, respiratory, and musculoskeletal diseases (3, 4). Older age, higher body mass index (BMI), and longer duration of the illness increase the burden of general medical comorbidities among people with a primary diagnosis of BD (5–7), affecting both the course and the treatment outcomes of either of the associated conditions (8).
Accruing evidence underscores the burden of cardiometabolic disease in BD, as highlighted by the higher rates of obesity (46% vs. 37%, Odds Ratio=1.62 [95%C.I. = 1.22–2.15]), elevated systolic blood pressure (35% vs. 19%, OR=2.18 [95%C.I. = 1.55–3.06]) and elevated serum triglyceride levels (37% vs. 26%, OR=1.58 [95%C.I. = 1.13–2.20]) in patients with BD compared to the matched controls (9). Additionally, patients with BD have an increased risk of fatal or non-fatal myocardial infarction or stroke (hazard ratio [HR]=1.54 [95%C.I. = 1.02–2.33]) (10) and an increased risk of major adverse cardiac events (MACE; HR=1.66 [95%C.I. = 1.17–2.28]) (11, 12).
Several factors may contribute to poor physical health among patients with BD, including genetic vulnerability (13, 14), atypical depressive symptoms (15, 16), abnormal feeding behaviors and eating disorders such as binge eating (17, 18), unhealthy lifestyle with reduced physical activity (19, 20), poorer quality diet (21), tobacco smoking (22) and other substance use disorders (23), as well as adverse effects of psychotropic pharmacotherapy, such as weight gain and metabolic syndrome (24–26). Of note, BD is associated with an increased all-cause, suicide, and natural cause-related mortality (27) that is estimated to be twice higher compared to the general population (28) and with a shorter life expectancy, with a recent meta-analysis estimating an average of 13 years of potential life lost compared to the general population (29). Patients with BD die prematurely from multiple causes, including cardiovascular disease, diabetes, chronic obstructive pulmonary disease, influenza or pneumonia, unintentional injuries, and suicide (27, 28, 30). The medical burden in BD is also associated with significant costs, both direct – hospital admissions, use of medical resources – and indirect – loss of work and productivity (31–34).
In addition, previous evidence highlights that discharged psychiatric inpatients are at increased risk of serious adverse outcomes, including all-cause mortality (35). Notably, BD patients are more susceptible to medical hospitalization (36). This underscores the critical need to delve into the complex dynamics of post-hospitalization care for BD patients, emphasizing the imperative for tailored interventions and a comprehensive healthcare approach. Although high hospitalization rates, healthcare costs (37), and increased medical comorbidity are well known in BD patients (4, 14), the factors associated with their increased risk of medical admission after discharge from psychiatric units remain largely unexplored.
In this retrospective observational cohort study, we aimed to identify predictors of medical admissions after discharge from psychiatric hospitalization of people with a primary diagnosis of BD. Given the large number of predictors, we used a machine learning approach that allows the estimation of predictive models from high-dimensional data and automates feature selection while mitigating the bias introduced by manually selecting subsets of potential predictors typically required in traditional regression models (38–40). Compared to standard stepwise regression, this approach provides a more “parsimonious” model, thus avoiding overfitting when multiple predictors are tested.
2 Materials and methods
2.1 Study cohort
The present retrospective observational cohort study documented inpatients admitted to the psychiatric ward of Padova University Hospital (Padua, Italy) from July 2017 to July 2019, with an established diagnosis of BD according to DSM-IV (i.e., type I, II, or not otherwise specified [NOS] BD, but not cyclothymia). All patients included in this study previously signed a written informed consent for the general use of their data for research purposes anonymously and in aggregate form. In accordance with our local Internal Review Board, the passive review of medical records for this retrospective and naturalistic research study did not require patients to provide further informed consent. This study was carried out in accordance with the guidelines of the Declaration of Helsinki of 1975.
2.2 Assessed variables
Demographic data (i.e., age and sex), anthropometric measures (weight and height), electrocardiograms (ECG), routine blood tests (i.e., cholesterol, glucose, hemoglobin, platelets, potassium, triglycerides, TSH levels and white blood cells count), characteristics of BD (age at onset, and polarity of the index episode, presence of psychotic symptoms during inpatient observation), number of comorbid mental disorders (other than BD), number and type of medical comorbidities (i.e., cancer, cardiovascular, diabetes, hypertension, dyslipidemia, hematological, endocrine, gastrointestinal, gynecological, infectious, renal, hepatic, neurological, osteomuscolar, respiratory, and thyroid disorders), number and type of prescribed medications (i.e., psychiatric: antipsychotics, antidepressants, mood stabilizers, and other psychotropic medications; and non-psychiatric medications), administration of long-acting injectable antipsychotics before admission were gathered for the consecutively admitted patients with BD. A detailed list of the variables collected is available in the Supplementary Material, Supplementary Table 1. We followed up with participants for three years and recorded the first and each subsequent hospitalization for non-psychiatric reasons until the end of the follow-up or the participant’s death.
2.3 Statistical analyses
For descriptive statistics, categorical variables were summarized by frequency, proportions, and percentages, while mean, median, interquartile range (IQR), standard deviation (SD), and confidence intervals (95%CIs) were used for continuous variables. The proportions of medical admissions for patients with BD recruited in the current study were compared with age- and sex-matched hospitalizations in the general population of the same region (Veneto, Italy) with a chi-squared test (χ2) (41). Using variables collected during psychiatric hospitalization (baseline), we attempted to create predictive models of subsequent admission to any general medical ward within the next three years of follow-up. The first model was designed to predict the occurrence of hospitalization in the general medical ward using any collected measures (i.e., biochemical, clinical, ECG-related, psychiatric, and sociodemographic). The second model considered the same outcome and used psychiatric and sociodemographic variables only as predictors. Finally, the third model used medical, including biochemical, clinical, ECG-related, and sociodemographic variables only as predictors. First, we used the “least absolute shrinkage and selection operator” (LASSO) logistic and normal regression models to impute the missing values 20 times (42). This supervised machine learning technique handles the problem of overfitting by including a penalty term that shrinks coefficient estimates toward zero and removes non-critical characteristics from the model. Second, we used another LASSO logistic regression to predict whether a participant would be admitted to any general medical ward during follow-up. We used a leave-one-out cross-validation procedure to subdivide the original sample into two non-overlapping, independent subsamples: the training subsample (in which we fitted the models to impute and the model to predict) and the test subsample (used to check whether the model predicted correctly). We calculated the area under the curve (AUC) and the 95% CIs, sensitivity, specificity, and balanced accuracy (BAC) to estimate the model’s performance. For each variable, the importance scores were obtained by normalizing the absolute values of their coefficients across multiple models and imputations and thresholded as ‘relevant’ using a cut-off of at least 1%. This represents the relative importance or contribution of each predictor to the model. A receiver operating characteristic (ROC) plot was used to show the relationship between sensitivity and specificity for each prediction model. Initially, we aimed to predict the number of hospitalizations using a Poisson LASSO regression but the small number of patients with >1 admission (n=7) did not allow a proper fit of the model. We imputed the missing data, trained the machine learning models, and validated them using R version 4.1.2 (43) and the R package glmnet (44).
3 Results
Seventy-one patients were eligible for inclusion, consented to participate, and completed the three-year follow-up study (see Table 1). Briefly, 45 participants were diagnosed with BD-I, 13 with BD-II, and 13 with BD-NOS. The mean age was 52.19 years old (SD=14.29); 40 participants were female (56.33%). The prevalent polarity was the only baseline characteristic statistically significantly different across different types of BD. Patients with BD-II or BD-NOS had a higher number of depressive episodes than hypomanic episodes. In contrast, patients with BD-I prevalent polarity were more frequently manic (48.9%) than depressive (26.7%) or undetermined (20%). The total number of BD patients admitted to a medical ward was 18: 13 BD-I (with six patients admitted twice or more), 2 BD-II, and 3 BD-NOS (with one admitted three times). The proportion of medical admissions at three-year follow-up in our sample (25.4%) was significantly higher compared with age- and sex-matched hospitalizations (8.48%) in the same region (p<0.001). Five patients (7%) died during follow-up; four for medical reasons (cancer, COVID-19, or unknown) and one for suicide.
3.1 Prediction of hospitalization in any general medical ward using all variables (global model)
In this model, we used 109 baseline variables (i.e., 10 biochemical, 31 clinical, 11 ECG-related, 55 psychiatric, and 2 sociodemographic) to predict the occurrence of medical admission during the three years of follow-up. The model was able to predict the outcome (AUC=69.5%, 95%C.I. = 55-84.1). The sensitivity was 61.1% and the specificity 75.5%, resulting in a BAC=68.3%. A ROC plot is presented in Figure 1. The most relevant variables in the model were cardiovascular disease (importance=59.2%); neurological diseases (importance=24.8%); osteomuscular diseases (importance=7.6%); valproate (importance=3.3%); and age (importance=2.1%) (Figure 2A). The sum of the importance of all remaining variables was 3%.
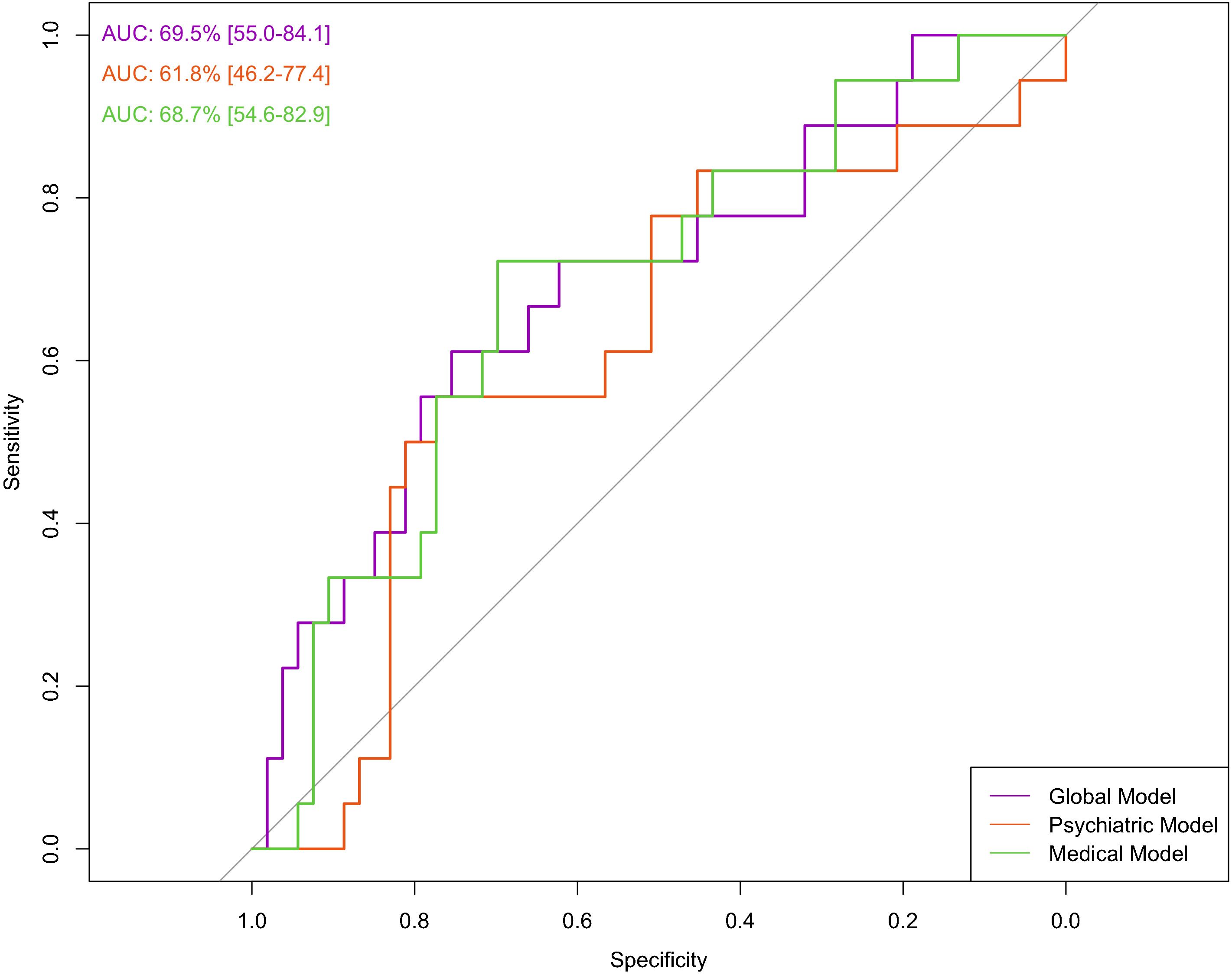
Figure 1. Prediction of hospitalization in any general medical ward using all variables (global model), psychiatric variables (psychiatric model), and medical variables (medical model). The ROC curve shows the relationship between sensitivity and specificity for each prediction model.
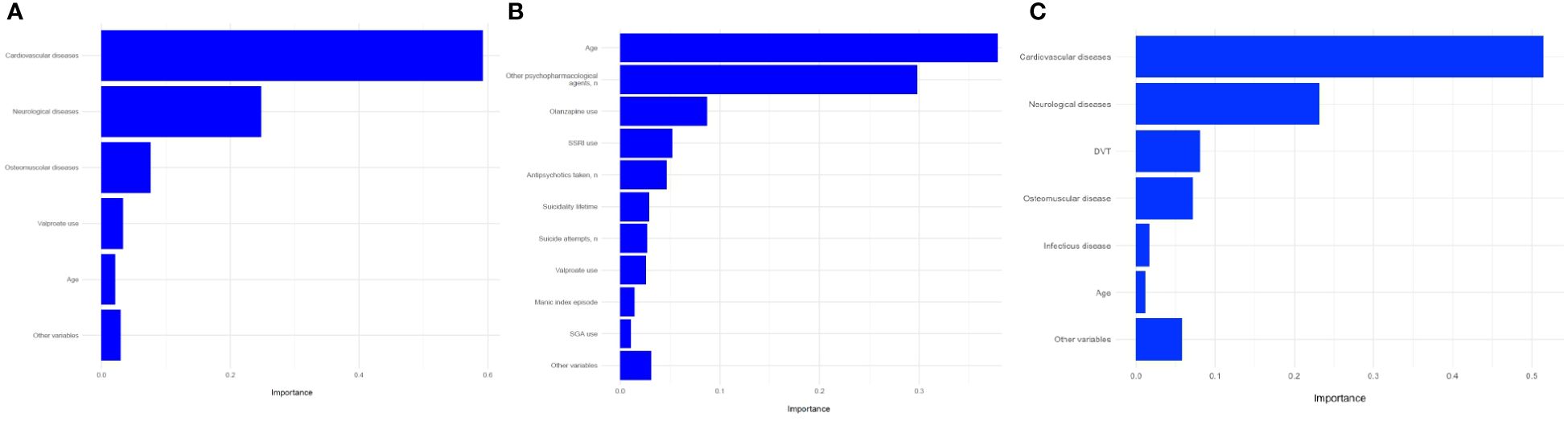
Figure 2. Prediction of hospitalization in any general medical ward using (A) all variables (global model); (B) psychiatric variables (psychiatric model); (C) medical variables (medical model); variable importance. SSRI, Selective serotonin reuptake inhibitor; SGA, Second-generation antipsychotic; DVT, deep vein thrombosis.
3.2 Prediction of hospitalization in any general medical ward using sociodemographic and psychiatric variables (psychiatric model)
In this model, we used psychiatric (n=55) and sociodemographic (n=2) baseline variables only. The model did not predict the outcome (AUC= 61.8%, 95%C.I. = 46.2-77.4). The sensitivity was 55.5%, and the specificity 66.0%, resulting in a BAC of 60.8%. A ROC curve is presented in Figure 1. The most relevant variables of the model were age (importance=37.9%), number of other psychopharmacological agents (importance=29.8%), olanzapine use (importance=8.7%), SSRI use (importance=5.2%), number of antipsychotics (importance=4.7%), suicidality lifetime (importance=2.9%), number of suicide attempts (importance=2.7%), valproate use (importance=2.6%), manic index episode (importance=1.4%), and second-generation antipsychotic use (importance=1%) (Figure 2B). The sum of the importance of all remaining variables was 3.1%.
3.3 Prediction of hospitalization in any general medical ward using sociodemographic, biochemical, clinical, and ECG-related variables (medical model)
We used baseline variables related to medical factors in this model (i.e., 10 biochemical, 31 clinical, 11 ECG-related, and 2 sociodemographic). The model was able to predict the outcome (AUC=68.7%, 95%C.I. = 54.6-82.9). Sensitivity was 55.5%, and specificity 71.6%, resulting in a BAC of 63.6%. A ROC curve is presented in Figure 1. The most relevant variables in the model were cardiovascular disease (importance=51.5%), neurological disease (importance=23.2%), deep vein thrombosis (importance=8%), osteomuscular disease (importance=7.1%), infectious disease (importance=1.7%), age (importance=1.2%) (Figure 2C). The sum of the importance of all remaining variables was 5.8%.
4 Discussion
The current three-year retrospective cohort study of 71 patients diagnosed with BD confirmed an increased susceptibility to medical hospitalization aligning with previous reports (36, 45, 46). Using baseline biochemical, clinical, ECG-related, psychiatric, and sociodemographic variables, we developed three supervised machine learning models and two out of three were able to predict general medical admission after a psychiatric exacerbation.
The global model included all variables and showed a fair predictive performance, being able to discriminate those undergoing a medical hospitalization during follow-up with a BAC of 68.3%. The most important variables were the co-occurrence of cardiovascular, neurological, or osteomuscular diseases, which together accounted for more than 90% of the influence on the model.
Again, the medical model, which included all the variables of the global model except the psychiatric ones, was able to predict the outcome, resulting in a BAC of 63.6%. These findings highlight the critical importance of recognizing and addressing medical comorbidity as a significant issue in patients with BD. Indeed, physical health conditions are more frequent in BD compared to the general population, with a two-fold increase in mortality, even after adjusting for age and other sociodemographic factors (28). Additionally, compared to other psychiatric conditions such as schizophrenia spectrum disorders, patients with BD were more likely to have at least one physical comorbidity (47), with specific differences in cardiovascular, nutritional, and metabolic diseases (48). The reasons why these aspects can strongly influence subsequent hospitalizations in people diagnosed with BD may lie in the fact that people with severe mental illness, commonly receive poorer quality of care for physical comorbidities due to several barriers related to patient, treatment, physician, and service (49–51). Therefore, the importance of these variables in the models calls for greater attention to their monitoring, which often appears to be considered of minor relevance (52), to ensure better follow-up of patients and potentially reduce the risk of hospitalizations. Although not as important as the variables discussed so far, the use of valproate appeared to have a modest effect on the outcome of interest. Valproate treatment has been associated with a higher incidence of side effects such as tremors (53), abdominal pain, vomiting (54), dizziness, somnolence (55), or weight gain (55, 56) compared with placebo, teratogenic risk (57), and with increased somnolence or nausea compared with other drugs such as lithium or olanzapine (55, 58). Considering this safety profile, which could worsen a poor physical condition, our results urge caution in the use of this drug. In this light, and also considering the impact of neurological disorders on hospitalizations, the neuroprotective profile associated with the use of lithium seems to be a recommended choice to reduce the physical burden of these patients (59–61).
The psychiatric model included only psychiatric and sociodemographic variables and did not significantly discriminate against those admitted to a general medical ward during follow-up. Notably, while psychopharmacological therapies can have an impact on the physical health of the individual (24), they do not predict a medical hospitalization. Although the reduced model was not significant, age showed an impact of about 40% on overall prediction, thus suggesting a need for greater clinical attention to older patients with BD (62).
Furthermore, in our sample, patients with BD-I were more likely to be admitted to the medical wards (28.9%) than those with BD-II (15.4%) or BD-NOS (23.1%). These findings are consistent with previous reports showing that patients with BD-I had a higher probability of presenting at least one co-occurring chronic medical disorder diagnosed by the physician than patients who screened negative for a manic episode (64.3 vs. 48.5%) (63). Thus, our findings could also be related to the association between chronic medical disorders and the severe course of BD (63). Interestingly, among the psychiatric variables that contributed to the model, current drug treatments, including the type and number of medications, played an important role. Although the overall model did not significantly predict the outcome, we can speculate that specific medications could contribute to an increased risk of medical comorbidities during follow-up (i.e., antipsychotic medications) and cardiovascular and metabolic diseases (12). On the other hand, non-adherence to pharmacological treatment can also increase the risk of all-cause rehospitalization (64, 65). In our sample, during the 3-year retrospective follow-up, we found a mortality rate of 7%, which is consistent with the epidemiological scenarios of the COVID-19 pandemic (66) and the excess mortality rate it caused (67).
Although large evidence in BD has identified being uninsured, the number of previous psychiatric hospitalizations, a younger age, experiencing depressive episodes characterized by prominent neurovegetative features, the number of previous mood episodes, or having lower global functioning and greater severity at discharge as the risk factors for psychiatric readmission (68–72), the predictors associated with a greater probability of general medical hospitalization are less studied and poorly appraised. In our model, although psychiatric and sociodemographic variables contributed to the risk of hospitalization in the global model, they were unable to predict the outcome when the analysis included only these variables. Overall, our findings support the idea that the burden in BD is due not only to sociodemographic and psychiatric conditions but also to general medical problems. They influence specifically medical admission but could also contribute to psychiatric exacerbation. Previous evidence synthesis conducted by Šprah and colleagues (73) supports the hypothesis that patients with mental disorders are at increased risk of psychiatric readmission if they have a co-occurring medical condition, and the same result is confirmed in BD (40, 74).
The current findings should be interpreted in light of some limitations. First, the small sample size did not allow the analysis of other outcomes (such as mortality and survival analysis) and the stratification for the types of BD (75). Larger cohorts are needed to validate the predictive models and enhance their robustness. Second, the retrospective study design may introduce inherent limitations, such as information bias and missing data, which, together with the lack of nested control for BD patients who would not be admitted to a general medical ward, limit our ability to establish causality in the current findings. Third, the regional basis of the study may limit the generalizability of our findings to other regions or healthcare settings. Fourth, the lack of external validation did not allow us to assess a model’s performance and reproducibility. Finally, the exclusion of patients with cyclothymia may limit the comprehensiveness of the current study in representing the entire BD spectrum. Although we decided to exclude patients with cyclothymia to reduce clinical heterogeneity and focus on more severe and homogeneous BD phenotypes, including all BD spectrum disorders could have provided a more complete understanding of the medical comorbidities and hospitalization risks associated with BD.
In conclusion, to our knowledge, this is the first study that specifically aimed to investigate predictors associated with medical admissions after discharge from psychiatric hospitalization in a cohort of BD, and although risk factors for hospitalization in psychiatric settings are well known, factors influencing medical hospitalization have not been studied extensively. Although the model we propose to describe medical hospitalization in patients with BD is in the development stage, the current study raises the need to further examine and carefully monitor medical comorbidities during clinical judgments in people with BD. Future research should employ prospective study designs with larger, multicenter cohorts to enhance the generalizability of our findings and provide more robust evidence regarding causality while better controlling for potential confounders. More prospective studies are needed to replicate and validate this model, as well as to quantify the risk of medical admission after discharge from the psychiatric ward in this vulnerable population to tailor effective preventive measures.
Data availability statement
The raw data supporting the conclusions of this article will be made available by the authors, without undue reservation.
Ethics statement
The studies involving humans were approved by the local Internal Review Board. The passive review of medical records for this retrospective and naturalistic research study did not require patients to provide a further informed consent. The studies were conducted in accordance with the local legislation and institutional requirements.
Author contributions
AM: Conceptualization, Data curation, Formal analysis, Methodology, Writing – original draft, Writing – review & editing. MP: Formal analysis, Methodology, Writing – original draft, Writing – review & editing. ML: Data curation, Investigation, Writing – original draft, Writing – review & editing. NM: Data curation, Formal analysis, Writing – original draft, Writing – review & editing. ED: Data curation, Investigation, Writing – review & editing. RC: Data curation, Investigation, Writing – review & editing. NN: Supervision, Writing – review & editing. MF: Supervision, Writing – review & editing. MV: Supervision, Writing – review & editing. MAF: Supervision, Writing – review & editing. EV: Supervision, Writing – review & editing. MS: Supervision, Writing – review & editing. JR: Formal analysis, Methodology, Supervision, Writing – review & editing. FS: Conceptualization, Methodology, Supervision, Writing – review & editing.
Funding
The author(s) declare financial support was received for the research, authorship, and/or publication of this article. Open Access funding provided by Università degli Studi di Padova | University of Padua, Open Science Committee.
Conflict of interest
MS received honoraria/has been a consultant for AbbVie, Angelini, Lundbeck, Otsuka. EV has received grants and served as consultant, advisor or CME speaker for the following entities: ABBiotics, AbbVie, Adamed, Angelini, Biogen, Beckley-Psytech, Biohaven, Boehringer-Ingelheim, Celon Pharma, Compass, Dainippon Sumitomo Pharma, Ethypharm, Ferrer, Gedeon Richter, GH Research, Glaxo-Smith Kline, HMNC, Idorsia, Johnson & Johnson, Lundbeck, Luye Pharma, Medincell, Merck, Newron, Novartis, Orion Corporation, Organon, Otsuka, Roche, Rovi, Sage, Sanofi-Aventis, Sunovion, Takeda, Teva, and Viatris, outside the submitted work. MAF received grant support from Assurex Health and Mayo Foundation, received CME travel and honoraria from Carnot Laboratories and American Physician Institute, and has Financial Interest/Stock ownership/Royalties from Chymia LLC. JR has received CME honoraria from Inspira Networks for a machine learning course promoted by Adamed, outside the submitted work.
The remaining authors declare that the research was conducted in the absence of any commercial or financial relationships that could be construed as a potential conflict of interest.
The author(s) declared that they were an editorial board member of Frontiers, at the time of submission. This had no impact on the peer review process and the final decision.
Publisher’s note
All claims expressed in this article are solely those of the authors and do not necessarily represent those of their affiliated organizations, or those of the publisher, the editors and the reviewers. Any product that may be evaluated in this article, or claim that may be made by its manufacturer, is not guaranteed or endorsed by the publisher.
Supplementary material
The Supplementary Material for this article can be found online at: https://www.frontiersin.org/articles/10.3389/fpsyt.2024.1435199/full#supplementary-material
References
1. World Health Organization, World Bank. World report on disability. Geneva, Switzerland: World Health Organization (2011). p. 325.
2. Lopez AD, Murray CCJL. The global burden of disease, 1990-2020. Nat Med. (1998) 4:1241–3. doi: 10.1038/3218
3. Forty L, Ulanova A, Jones L, Jones I, Gordon-Smith K, Fraser C, et al. Comorbid medical illness in bipolar disorder. Br J Psychiatry. (2014) 205:465–72. doi: 10.1192/bjp.bp.114.152249
4. Sylvia LG, Shelton RC, Kemp DE, Bernstein EE, Friedman ES, Brody BD, et al. Medical burden in bipolar disorder: findings from the Clinical and Health Outcomes Initiative in Comparative Effectiveness for Bipolar Disorder study (Bipolar CHOICE). Bipolar Disord. (2015) 17:212–23. doi: 10.1111/bdi.12243
5. Gomes FA, Magalhães PV, Magee T, Brietzke E, Kunz M, Kapczinski F. Clinical correlates of high burden of general medical comorbidities in patients with bipolar disorder. J Affect Disord Rep. (2020) 1:100001. doi: 10.1016/j.jadr.2020.100001
6. Stubbs B, Vancampfort D, Solmi M, Veronese N, Fornaro M. How common is bipolar disorder in general primary care attendees? A systematic review and meta-analysis investigating prevalence determined according to structured clinical assessments. Aust N Z J Psychiatry. (2016) 50:631–9. doi: 10.1177/0004867415623857
7. Chen M, Jiang Q, Zhang L. The prevalence of bipolar disorder in autoimmune disease: a systematic review and meta-analysis. Ann Palliat Med. (2021) 10:35061–361. doi: 10.21037/apm
8. Ramasubbu R, Beaulieu S, Taylor VH, Schaffer A, McIntyre RS. The CANMAT task force recommendations for the management of patients with mood disorders and comorbid medical conditions: Diagnostic, assessment, and treatment principles. Ann Of Clin Psychiatry. (2012) 24:82–90.
9. Cuellar-Barboza AB, Cabello-Arreola A, Winham SJ, Colby C, Romo-Nava F, Nunez NA, et al. Body mass index and blood pressure in bipolar patients: Target cardiometabolic markers for clinical practice. J Affect Disord. (2021) 282:637–43. doi: 10.1016/j.jad.2020.12.121
10. Prieto ML, Schenck LA, Kruse JL, Klaas JP, Chamberlain AM, Bobo WV, et al. Long-term risk of myocardial infarction and stroke in bipolar I disorder: A population-based Cohort Study. J Affect Disord. (2016) 194:120–7. doi: 10.1016/j.jad.2016.01.015
11. Foroughi M, Inojosa JRM, Lopez-Jimenez F, Saeidifard F, Suarez L, Stokin GB, et al. Association of bipolar disorder with major adverse cardiovascular events: A population-based historical cohort study. Psychosom Med. (2022) 84:97–103. doi: 10.1097/PSY.0000000000001017
12. Correll CU, Solmi M, Veronese N, Bortolato B, Rosson S, Santonastaso P, et al. Prevalence, incidence and mortality from cardiovascular disease in patients with pooled and specific severe mental illness: a large-scale meta-analysis of 3,211,768 patients and 113,383,368 controls. World Psychiatry. (2017) 16:163–80. doi: 10.1002/wps.20420
13. Amare AT, Schubert KO, Klingler-Hoffmann M, Cohen-Woods S, Baune BT. The genetic overlap between mood disorders and cardiometabolic diseases: a systematic review of genome wide and candidate gene studies. Transl Psychiatry. (2017) 7:e1007. doi: 10.1038/tp.2016.261
14. Miola A, De Filippis E, Veldic M, Ho AMC, Winham SJ, Mendoza M, et al. The genetics of bipolar disorder with obesity and type 2 diabetes. J Affect Disord. (2022) 313:222–31. doi: 10.1016/j.jad.2022.06.084
15. Calkin CV, Gardner DM, Ransom T, Alda M. The relationship between bipolar disorder and type 2 diabetes: more than just co-morbid disorders. Ann Med. (2013) 45:171–81. doi: 10.3109/07853890.2012.687835
16. Łojko D, Buzuk G, Owecki M, Ruchała M, Rybakowski JK. Atypical features in depression: Association with obesity and bipolar disorder. J Affect Disord. (2015) 185:76–80. doi: 10.1016/j.jad.2015.06.020
17. McElroy SL, Crow S, Biernacka JM, Winham S, Geske J, Barboza ABC, et al. Clinical phenotype of bipolar disorder with comorbid binge eating disorder. J Affect Disord. (2013) 150:981–6. doi: 10.1016/j.jad.2013.05.024
18. McElroy SL, Crow S, Blom TJ, Biernacka JM, Winham SJ, Geske J, et al. Prevalence and correlates of DSM-5 eating disorders in patients with bipolar disorder. J Affect Disord. (2016) 191:216–21. doi: 10.1016/j.jad.2015.11.010
19. Vancampfort D, Firth J, Schuch FB, Rosenbaum S, Mugisha J, Hallgren M, et al. Sedentary behavior and physical activity levels in people with schizophrenia, bipolar disorder and major depressive disorder: a global systematic review and meta-analysis. World Psychiatry. (2017) 16:308–15. doi: 10.1002/wps.20458
20. Croatto G, Vancampfort D, Miola A, Olivola M, Fiedorowicz JG, Firth J, et al. The impact of pharmacological and non-pharmacological interventions on physical health outcomes in people with mood disorders across the lifespan: An umbrella review of the evidence from randomised controlled trials. Mol Psychiatry. (2023) 28:369–90. doi: 10.1038/s41380-022-01770-w
21. Gardea-Resendez M, Winham SJ, Romo-Nava F, Cuellar-Barboza A, Clark MM, Andreazza AC, et al. Quantification of diet quality utilizing the rapid eating assessment for participants-shortened version in bipolar disorder: Implications for prospective depression and cardiometabolic studies. J Affect Disord. (2022) 310:150–5. doi: 10.1016/j.jad.2022.05.037
22. Li XH, An FR, Ungvari GS, Ng CH, Chiu HFK, Wu PP, et al. Prevalence of smoking in patients with bipolar disorder, major depressive disorder and schizophrenia and their relationships with quality of life. Sci Rep. (2017) 7:8430. doi: 10.1038/s41598-017-07928-9
23. Hunt GE, Malhi GS, Cleary M, Lai HMX, Sitharthan T. Prevalence of comorbid bipolar and substance use disorders in clinical settings, 1990-2015: Systematic review and meta-analysis. J Affect Disord. (2016) 206:331–49. doi: 10.1016/j.jad.2016.07.011
24. Correll CU, Detraux J, De Lepeleire J, De Hert M. Effects of antipsychotics, antidepressants and mood stabilizers on risk for physical diseases in people with schizophrenia, depression and bipolar disorder. World Psychiatry. (2015) 14:119–36. doi: 10.1002/wps.v14.2
25. Dragioti E, Radua J, Solmi M, Gosling CJ, Oliver D, Lascialfari F, et al. Impact of mental disorders on clinical outcomes of physical diseases: an umbrella review assessing population attributable fraction and generalized impact fraction. World Psychiatry. (2023) 22:86–104. doi: 10.1002/wps.21068
26. Kerrison RS, Jones A, Peng J, Price G, Verne J, Barley EA, et al. Inequalities in cancer screening participation between adults with and without severe mental illness: results from a cross-sectional analysis of primary care data on English Screening Programmes. Br J Cancer. (2023) 129:81–93. doi: 10.1038/s41416-023-02249-3
27. Biazus TB, Beraldi GH, Tokeshi L, Rotenberg L de S, Dragioti E, Carvalho AF, et al. All-cause and cause-specific mortality among people with bipolar disorder: a large-scale systematic review and meta-analysis. Mol Psychiatry. (2023) 28:2508–24. doi: 10.1038/s41380-023-02109-9
28. Crump C, Sundquist K, Winkleby MA, Sundquist J. Comorbidities and mortality in bipolar disorder: a Swedish national cohort study. JAMA Psychiatry. (2013) 70:931–9. doi: 10.1001/jamapsychiatry.2013.1394
29. Chan JKN, Tong CCHY, Wong CSM, Chen EYH, Chang WC. Life expectancy and years of potential life lost in bipolar disorder: systematic review and meta-analysis. Br J Psychiatry. (2022) 221:567–76. doi: 10.1192/bjp.2022.19
30. Hayes JF, Miles J, Walters K, King M, Osborn DPJ. A systematic review and meta-analysis of premature mortality in bipolar affective disorder. Acta Psychiatr Scand. (2015) 131:417–25. doi: 10.1111/acps.12408
31. Wyatt RJ, Henter I. An economic evaluation of manic-depressive illness–1991. Soc Psychiatry Psychiatr Epidemiol. (1995) 30:213–9. doi: 10.1007/BF00789056
32. Johnson RE, McFarland BH. Lithium use and discontinuation in a health maintenance organization. Am J Psychiatry. (1996) 153:993–1000. doi: 10.1176/ajp.153.8.993
33. Goetzel RZ, Hawkins K, Ozminkowski RJ, Wang S. The health and productivity cost burden of the “top 10” physical and mental health conditions affecting six large U.S. employers in 1999. J Occup Environ Med. (2003) 45:5–14. doi: 10.1097/00043764-200301000-00007
34. Centorrino F, Mark TL, Talamo A, Oh K, Chang J. Health and economic burden of metabolic comorbidity among individuals with bipolar disorder. J Clin Psychopharmacol. (2009) 29:595–600. doi: 10.1097/JCP.0b013e3181bef8a6
35. Walter F, Carr MJ, Mok PLH, Antonsen S, Pedersen CB, Appleby L, et al. Multiple adverse outcomes following first discharge from inpatient psychiatric care: a national cohort study. Lancet Psychiatry. (2019) 6:582–9. doi: 10.1016/S2215-0366(19)30180-4
36. Davydow DS, Ribe AR, Pedersen HS, Fenger-Grøn M, Cerimele JM, Vedsted P, et al. Serious mental illness and risk for hospitalizations and rehospitalizations for ambulatory care-sensitive conditions in Denmark: A nationwide population-based cohort study. Med Care. (2016) 54:90–7. doi: 10.1097/MLR.0000000000000448
37. Bessonova L, Ogden K, Doane MJ, O’sullivan AK, Tohen M. The economic burden of bipolar disorder in the United States: A systematic literature review. Clinicoecon Outcomes Res. (2020) 12:481–97. doi: 10.2147/CEOR.S259338
38. Tibshirani R. Regression shrinkage and selection via the lasso. J R Stat Soc Ser B (Methodological). (1996) 58:267–88. doi: 10.1111/j.2517-6161.1996.tb02080.x
39. Madigan D, Ryan PB, Schuemie M, Stang PE, Overhage JM, Hartzema AG, et al. Evaluating the impact of database heterogeneity on observational study results. Am J Epidemiol. (2013) 178:645–51. doi: 10.1093/aje/kwt010
40. Edgcomb J, Shaddox T, Hellemann G, Brooks JO. High-risk phenotypes of early psychiatric readmission in bipolar disorder with comorbid medical illness. Psychosomatics. (2019) 60:563–73. doi: 10.1016/j.psym.2019.05.002
41. ISTAT. Multipurpose Survey on Households: Aspects of Daily Life (2023). Available online at: https://www.istat.it/en/archivio/129934 (Accessed January 17, 2024).
42. Solanes A, Mezquida G, Janssen J, Amoretti S, Lobo A, González-Pinto A, et al. Combining MRI and clinical data to detect high relapse risk after the first episode of psychosis. Schizophrenia. (2022) 8:1–9. doi: 10.1038/s41537-022-00309-w
43. R Core Team. R: A language and environment for statistical computing. Vienna, Austria: R Foundation for Statistical Computing (2022). Available at: https://www.R-project.org/.
44. Friedman J, Hastie T, Tibshirani R. Regularization paths for generalized linear models via coordinate descent. J Stat Softw. (2010) 33:1. doi: 10.18637/jss.v033.i01
45. Dembek C, Mackie de M, Modi K, Zhu Y, Niu X, Grinnell T. The economic and humanistic burden of bipolar disorder in adults in the United States. Ann Gen Psychiatry. (2023) 22:13. doi: 10.1186/s12991-023-00440-7
46. Leung N, Bernacki SE, Kalia N, Bernacki EJ, Almeida JRC. Long-term healthcare utilization and the cost of bipolar disorder among participants in a large employer’s health benefit plan. J Occup Environ Med. (2022) 64:E124–30. doi: 10.1097/JOM.0000000000002465
47. Goldman ML, Mangurian C, Corbeil T, Wall MM, Tang F, Haselden M, et al. Medical comorbid diagnoses among adult psychiatric inpatients. Gen Hosp Psychiatry. (2020) 66:16–23. doi: 10.1016/j.genhosppsych.2020.06.010
48. Mariano A, Di Lorenzo G, Jannini TB, Santini R, Bertinelli E, Siracusano A, et al. Medical comorbidities in 181 patients with bipolar disorder vs. Schizophrenia and related psychotic disorders: findings from a single-center, retrospective study from an acute inpatients psychiatric unit. Front Psychiatry. (2021) 12:702789. doi: 10.3389/fpsyt.2021.702789
49. De Hert M, Cohen D, Bobes J, Cetkovich-Bakmas M, Leucht S, Ndetei DM, et al. Physical illness in patients with severe mental disorders. II. Barriers to care, monitoring and treatment guidelines, plus recommendations at the system and individual level. World Psychiatry. (2011) 10:138–51. doi: 10.1002/j.2051-5545.2011.tb00036.x
50. Solmi M, Fiedorowicz J, Poddighe L, Delogu M, Miola A, Høye A, et al. Disparities in screening and treatment of cardiovascular diseases in patients with mental disorders across the world: systematic review and meta-analysis of 47 observational studies. Am J Psychiatry. (2021) 178:793–803. doi: 10.1176/appi.ajp.2021.21010031
51. Solmi M, Firth J, Miola A, Fornaro M, Frison E, Fusar-Poli P, et al. Disparities in cancer screening in people with mental illness across the world versus the general population: prevalence and comparative meta-analysis including 4 717 839 people. Lancet Psychiatry. (2020) 7:52–63. doi: 10.1016/S2215-0366(19)30414-6
52. Kupfer DJ. The increasing medical burden in bipolar disorder. JAMA. (2005) 293:2528–30. doi: 10.1001/jama.293.20.2528
53. Cipriani A, Reid K, Young AH, Macritchie K, Geddes J. Valproic acid, valproate and divalproex in the maintenance treatment of bipolar disorder. Cochrane Database Syst Rev. (2013) 2013:CD003196. doi: 10.1002/14651858.CD003196.pub2
54. Jochim J, Rifkin-Zybutz RP, Geddes J, Cipriani A. Valproate for acute mania. Cochrane Database Syst Rev. (2019) 10:CD004052. doi: 10.1002/14651858.CD004052.pub2
55. Kishi T, Ikuta T, Matsuda Y, Sakuma K, Okuya M, Nomura I, et al. Pharmacological treatment for bipolar mania: a systematic review and network meta-analysis of double-blind randomized controlled trials. Mol Psychiatry. (2022) 27:1136–44. doi: 10.1038/s41380-021-01334-4
56. Yildiz A, Siafis S, Mavridis D, Vieta E, Leucht S. Comparative efficacy and tolerability of pharmacological interventions for acute bipolar depression in adults: a systematic review and network meta-analysis. Lancet Psychiatry. (2023) 10:693–705. doi: 10.1016/S2215-0366(23)00199-2
57. Anmella G, Vieta E. Teratogenicity of valproate: Further reasons for action. Eur Neuropsychopharmacol. (2023) 80:25–6. doi: 10.1016/j.euroneuro.2023.11.008
58. McKnight RF, de la Motte de Broöns de Vauvert SJGN, Chesney E, Amit BH, Geddes J, Cipriani A. Lithium for acute mania. Cochrane Database Syst Rev. (2019) 6:CD004048. doi: 10.1002/14651858.CD004048.pub4
59. Puglisi-Allegra S, Ruggieri S, Fornai F. Translational evidence for lithium-induced brain plasticity and neuroprotection in the treatment of neuropsychiatric disorders. Transl Psychiatry. (2021) 11:366. doi: 10.1038/s41398-021-01492-7
60. Chen S, Underwood BR, Jones PB, Lewis JR, Cardinal RN. Association between lithium use and the incidence of dementia and its subtypes: A retrospective cohort study. PloS Med. (2022) 19e1003941. doi: 10.1371/journal.pmed.1003941
61. Velosa J, Delgado A, Finger E, Berk M, Kapczinski F, de Azevedo Cardoso T. Risk of dementia in bipolar disorder and the interplay of lithium: a systematic review and meta-analyses. Acta Psychiatr Scand. (2020) 141:510–21. doi: 10.1111/acps.13153
62. Soreca I, Fagiolini A, Frank E, Houck PR, Thompson WK, Kupfer DJ. Relationship of general medical burden, duration of illness and age in patients with bipolar I disorder. J Psychiatr Res. (2008) 42:956–61. doi: 10.1016/j.jpsychires.2007.10.009
63. McIntyre RS, Konarski JZ, Soczynska JK, Wilkins K, Panjwani G, Bouffard B, et al. Medical comorbidity in bipolar disorder: implications for functional outcomes and health service utilization. Psychiatr Serv. (2006) 57:1140–4. doi: 10.1176/ps.2006.57.8.1140
64. Hassan M, Lage MJ. Risk of rehospitalization among bipolar disorder patients who are nonadherent to antipsychotic therapy after hospital discharge. Am J Health Syst Pharm. (2009) 66:358–65. doi: 10.2146/ajhp080374
65. Samalin L, Belzeaux R. Why does non-adherence to treatment remain a leading cause of relapse in patients with bipolar disorder? Eur Neuropsychopharmacol. (2023) 73:16–8. doi: 10.1016/j.euroneuro.2023.04.012
66. Fornaro M, De Prisco M, Billeci M, Ermini E, Young AH, Lafer B, et al. Implications of the COVID-19 pandemic for people with bipolar disorders: A scoping review. J Affect Disord. (2021) 295:740–51. doi: 10.1016/j.jad.2021.08.091
67. Mathieu E, Ritchie H, Rodés-Guirao L, Appel C, Giattino C, Hasell J, et al. Coronavirus Pandemic (COVID-19) (2023). Available online at: OurWorldInData.org.
68. Perlick DA, Rosenheck RA, Clarkin JF, Sirey JA, Raue P. Symptoms predicting inpatient service use among patients with bipolar affective disorder. Psychiatr Serv. (1999) 50:806–12. doi: 10.1176/ps.50.6.806
69. Kessing LV, Hansen MG, Andersen PK. Course of illness in depressive and bipolar disorders. Naturalistic study, 1994-1999. Br J Psychiatry. (2004) 185:372–7. doi: 10.1192/bjp.185.5.372
70. Patel NC, Crismon ML, Pondrom M. Rehospitalization rates of patients with bipolar disorder discharged on a mood stabilizer versus a mood stabilizer plus an atypical or typical antipsychotic. J Behav Health Serv Res. (2005) 32:438–45. doi: 10.1007/BF02384203
71. Woo YS, Bahk WM, Jung YE, Jeong JH, Lee HB, Won SH, et al. One-year rehospitalization rates of patients with first-episode bipolar mania receiving lithium or valproate and adjunctive atypical antipsychotics. Psychiatry Clin Neurosci. (2014) 68:418–24. doi: 10.1111/pcn.12145
72. Hamilton JE, Passos IC, De Azevedo Cardoso T, Jansen K, Allen M, Begley CE, et al. Predictors of psychiatric readmission among patients with bipolar disorder at an academic safety-net hospital. Aust N Z J Psychiatry. (2016) 50:584–93. doi: 10.1177/0004867415605171
73. Šprah L, Dernovšek MZ, Wahlbeck K, Haaramo P. Psychiatric readmissions and their association with physical comorbidity: A systematic literature review. BMC Psychiatry. (2017) 17:2. doi: 10.1186/s12888-016-1172-3
74. Amann BL, Radua J, Wunsch C, König B, Simhandl C. Psychiatric and physical comorbidities and their impact on the course of bipolar disorder: A prospective, naturalistic 4-year follow-up study. Bipolar Disord. (2017) 19:225–34. doi: 10.1111/bdi.12495
Keywords: bipolar disorder, comorbidity, premature mortality, general medicine admission, machine learning, clinical decision-making
Citation: Miola A, De Prisco M, Lussignoli M, Meda N, Dughiero E, Costa R, Nunez NA, Fornaro M, Veldic M, Frye MA, Vieta E, Solmi M, Radua J and Sambataro F (2024) Prediction of medical admissions after psychiatric inpatient hospitalization in bipolar disorder: a retrospective cohort study. Front. Psychiatry 15:1435199. doi: 10.3389/fpsyt.2024.1435199
Received: 19 May 2024; Accepted: 16 July 2024;
Published: 03 September 2024.
Edited by:
Gábor Gazdag, Jahn Ferenc Dél-Pesti Kórház és Rendelőintézet, HungaryReviewed by:
Francesco Monaco, Azienda Sanitaria Locale Salerno, ItalyChristoph Born, Klinikum am Weissenhof, Germany
Copyright © 2024 Miola, De Prisco, Lussignoli, Meda, Dughiero, Costa, Nunez, Fornaro, Veldic, Frye, Vieta, Solmi, Radua and Sambataro. This is an open-access article distributed under the terms of the Creative Commons Attribution License (CC BY). The use, distribution or reproduction in other forums is permitted, provided the original author(s) and the copyright owner(s) are credited and that the original publication in this journal is cited, in accordance with accepted academic practice. No use, distribution or reproduction is permitted which does not comply with these terms.
*Correspondence: Fabio Sambataro, fabio.sambataro@unipd.it