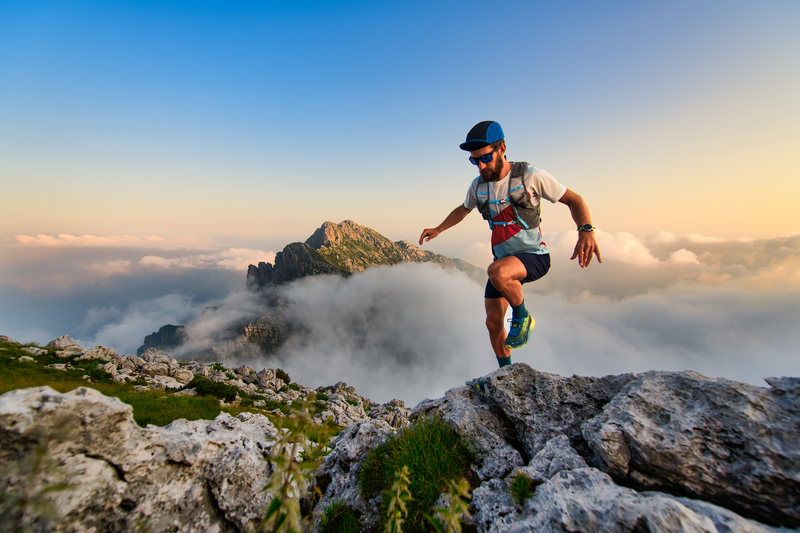
95% of researchers rate our articles as excellent or good
Learn more about the work of our research integrity team to safeguard the quality of each article we publish.
Find out more
ORIGINAL RESEARCH article
Front. Psychiatry , 24 June 2024
Sec. Schizophrenia
Volume 15 - 2024 | https://doi.org/10.3389/fpsyt.2024.1417213
Introduction: Schizophrenia (SCZ) is a severe psychiatric disorder whose pathophysiology remains elusive. Recent investigations have underscored the significance of systemic inflammation, particularly the impact of circulating inflammatory proteins, in SCZ.
Methods: This study explores the potential causal association between certain inflammatory proteins and SCZ. Bidirectional Mendelian randomization (MR) analyses were conducted utilizing data from expansive genome-wide association studies (GWAS). Data regarding circulating inflammatory proteins were sourced from the GWAS Catalog database, encompassing 91 inflammatory cytokines. SCZ-related data were derived from the Finngen database, incorporating 47,696 cases and 359,290 controls. Analytical methods such as inverse variance weighted, MR-Egger, weighted median, simple mode, and weighted mode were employed to evaluate the association between inflammatory cytokines and SCZ. Sensitivity analyses were also performed to affirm the robustness of the results.
Results: Following FDR adjustment, significant associations were observed between levels of inflammatory cytokines, including Fibroblast Growth Factor 5 (OR = 1.140, 95%CI = 1.045, 1.243, p = 0.003, FDR=0.015), C-C Motif Chemokine 4 (OR = 0.888, 95%CI = 0.816, 0.967, p = 0.006, FDR = 0.015), C-X-C Motif Chemokine 1 (OR = 0.833, 95%CI = 0.721, 0.962, p = 0.013, FDR = 0.064), and C-X-C Motif Chemokine 5 (OR = 0.870, 95%CI = 0.778, 0.973, p = 0.015, FDR = 0.074), and the risk of SCZ.
Conclusion: Our results from MR analysis suggest a potential causal link between circulating inflammatory cytokines and SCZ, thereby enriching our understanding of the interactions between inflammation and SCZ. Furthermore, these insights provide a valuable foundation for devising therapeutic strategies targeting inflammation.
Schizophrenia, a pervasive and debilitating psychiatric disorder, affects approximately 1% of the global population and significantly impacts public health as a leading cause of disability and premature mortality (1). This disorder is characterized by a varied spectrum of cognitive, behavioral, and emotional disturbances, devoid of a singular defining symptom. It involves positive symptoms, such as hallucinations and delusions, as well as negative symptoms, including reduced emotional expression, diminished motivation, and social withdrawal.
From an epidemiological standpoint, overwhelming evidence indicates that schizophrenia’s heritability is substantial, with estimates reaching as high as 81% based on extensive twin and family studies (2). The field of genetic epidemiology has experienced remarkable evolution, initially engaging in linkage studies and candidate gene assessments, progressing to genome-wide association studies (GWAS), and currently adopting next-generation sequencing (NGS) methodologies (3). GWAS, in particular, have broadened participant pools and enhanced statistical capabilities to identify common genetic variants linked to schizophrenia. These investigations have identified thousands of unique genetic sites that, together, contribute to a segment of the disorder’s heritability, as represented by single nucleotide polymorphisms (SNPs).
Inflammatory cytokines are central to the pathophysiology of schizophrenia, contributing significantly to neuroinflammatory processes. These cytokines modulate neuronal receptor activity, affecting neuronal communication and the dynamics of neurotransmitter release and re-uptake. Research has revealed variations in particular inflammatory cytokines in those with schizophrenia, including changes in levels of interleukins (IL) and tumor necrosis factor (TNF) (4). Such variations may be closely related to the genesis of schizophrenia and imply a potential link between irregular cytokine levels and the development of the disease.
IL-6 is one of the most extensively studied cytokines in schizophrenia research to date. Levels of IL-6 in both peripheral blood and cerebrospinal fluid are elevated across all patient groups (including those with first-episode psychosis, clinical high risk or ultra-high risk for psychosis) compared to healthy controls (5–7). Evidence suggests that the variability of IL-6 levels is significantly reduced compared to healthy controls, indicating that elevated IL-6 levels may be a key factor in the immune phenotype of schizophrenia (8). Concentrations of IL-6 in the blood are positively correlated with both negative and positive symptoms, as well as overall psychopathological manifestations and cognitive impairments (9, 10).
Furthermore, numerous studies have found elevated levels of IL-1β in drug-naïve first-episode psychosis patients (11), first-episode psychosis patients in both adults and children (most of whom were on atypical antipsychotic medications (12–14)), and in stable, acutely relapsed, or recovering chronic patients (15, 16). The levels of interleukin factors appear to be associated with the course of the disease and the severity of symptoms. Patients with elevated levels of IL-6, IL-8, and IL-4 tend to have a longer duration of illness and longer hospital stays (17, 18). Moreover, higher levels of IL-6, IL-1β, IL-33, and IL-17 are associated with more severe positive symptoms (19–22). Moreover, as potential biomarkers, inflammatory cytokines provide insight into the clinical state and treatment response of patients. Recent studies indicate that cytokine fluctuations are associated with symptom severity, disease progression, and responsiveness to antipsychotic treatment in schizophrenia. Therefore, cytokines represent promising indicators for early schizophrenia detection, disease monitoring, and treatment outcome assessment (23).
In this study, we perform a comprehensive two-sample Mendelian randomization (MR) analysis to elucidate the causal relationship between 91 inflammation-related biomarkers and the incidence of schizophrenia. Our study rests on three crucial assumptions: 1) the genetic variation selected as the instrumental variable (IV) is genuinely correlated with the exposure of interest; 2) the genetic variation must not be related to any confounding factors; and 3) the genetic variation affects the outcome only through exposure. The objective of this analysis is to uncover the inflammatory underpinnings of schizophrenia and identify potential targets for intervention that may slow down the progression and improve patient outcomes.
Through bidirectional two-sample MR analysis, we examined the causal link between 91 inflammatory cytokines and SCZ. Our approach was based on three core hypotheses: first, instrument variables (IVs) were robustly linked to exposure factors; second, IVs remained unaffected by confounders, whether known or unknown; and third, IVs influenced the outcome factors solely via exposure factors (24). Figure 1 depicts the comprehensive study design. The data leveraged in our research originated from the OPEN GWAS public databases and the Finngen database and it underwent anonymization before publication to ensure the absence of personal or identifiable information.
Figure 1 The main design of the causal relationship between inflammatory cytokines and schizophrenia through directional MR analyses.
The GWAS Catalog, containing entries from GCST90274758 to GCST90274848, provides a comprehensive repository of GWAS statistics for each circulating inflammatory protein (25). The catalog includes extensive data derived from 14,824 European individuals, covering 91 inflammatory cytokines. In selecting schizophrenia-related data from the Finnish database (https://www.finngen.fi/en), we considered factors such as sample size, publication year, the number of SNPs, and ethnicity. The chosen datasets comprise 406,986 participants of European descent, including 47,696 cases and 359,290 controls (Supplementary Table 1).
The cutoff value of 1×10–5 was applied in independent and significant SNPs for each immune trait. Linkage disequilibrium (LD) analysis was conducted using a rigorous threshold (r2 < 0.001) across a 10,000 kb range. To ensure the effect alignment, SNPs were harmonized across exposure and outcome datasets based on allele type. The F-statistic was calculated as F = R2 × (N-2)/(1-R2), with R2 denoting the extent to which the IVs explain the exposure factors and N denoting the sample size of the exposed GWAS data (26). SNPs with an F-statistic less than 10, suggestive of potential weak instrumental variable bias, were removed to mitigate impact on analyses. Subsequently, the resultant SNPs that corresponded with hypotheses were extracted from databases such as IEU OpenGWAS or FinnGen, and associations were ascertained. The final set of SNPs served as the definitive IVs for exposure.
MR analyses was performed using a variety of approaches: inverse-variance weighted (IVW), weighted mode, simple mode, weighted median, and MR Egger. The IVW method, utilized as the primary MR technique, integrates the Wald ratios of individual SNPs using meta-analysis. It operates under the assumption that IVs influence the outcomes solely through the exposure, which should yield unbiased causal estimates if horizontal pleiotropy is absent (27). Thus, we utilized the IVW method to provide reliable estimation in our study. To enhance our analytical framework and pinpoint potential biases stemming from ineffective IVs or horizontal pleiotropy, we employed the weighted median and MR-Egger methods. Nevertheless, results from these methods might be less precise due to the susceptibility to the influence of outlier genetic variants, particularly those from the MR-Egger method (28). While the weighted median approach presents lesser bias, it suffers from reduced precision. Despite their lower efficiency, the MR-Egger, simple mode, weighted mode, and weighted median methods were applied in supplementary analyses. This study complies with the Strengthening the Reporting of Observational Studies in Epidemiology using Mendelian Randomization (STROBE-MR) guidelines, as demonstrated by the checklist included in the Supplementary Material (Supplementary Table 7).
A sensitivity analysis was conducted to evaluate potential heterogeneity and pleiotropy. Firstly, IVW and MR-Egger regression were used, and Cochran Q statistics were generated to quantify heterogeneity. Furthermore, the intercept from MR-Egger regression was utilized to investigate horizontal pleiotropy (29). Leave-one-out analysis was carried out to determine the influence of excluding individual SNPs on the aggregate results (30). Scatter plots verifying the horizontal pleiotropy of inflammatory cytokines causally associated with SCZ. Additionally, funnel plots ascertained the integrity of the correlations and confirmed the absence of heterogeneity. All statistical procedures were executed with R software, version 4.3.1. MR analysis was performed employing the “TwoSampleMR” package in R.
Applying a relatively relaxed threshold (p < 1×10–5), we identified independent SNPs for all 91 inflammatory cytokines. Within this scope, 13 to 47 SNPs reached genome-wide significance as IVs for inflammatory cytokines, with F-statistics ranging markedly from 19.51 to 3468.99 (Supplementary Table 6). We found that Fibroblast Growth Factor 5 (FGF5) had an odds ratio (OR) of 1.140 (95% CI: 1.045–1.243, p = 0.003, False Discovery Rate (FDR) = 0.015) according to the IVW method, an OR of 1.189 (95% CI: 1.051–1.345, p = 0.0098) by MR-Egger, an OR of 1.114 (95% CI: 1.012–1.228, p = 0.028) by weighted median, an OR of 1.291 (95% CI: 1.021–1.632, p = 0.041) by simple mode, and an OR of 1.135 (95% CI: 1.028–1.255, p = 0.018) by weighted mode. For C-C Motif Chemokine 4 (CCL4), the IVW method yielded an OR of 0.888 (95% CI: 0.816–0.967, p = 0.006, FDR = 0.015), while the MR-Egger, weighted median, simple mode, and weighted mode indicated an OR of 0.947 (95% CI: 0.841–1.067, p = 0.377), 0.931 (95% CI: 0.826–1.049, p = 0.242), 0.623 (95% CI: 0.455–0.853, p = 0.006), and 0.910 (95% CI: 0.818–1.013, p = 0.095) respectively. Regarding C-X-C Motif Chemokine 1 (CXCL1), the IVW method estimated an OR of 0.833 (95% CI: 0.721–0.962, p = 0.013, FDR = 0.064). Results from additional methods were as follows: MR-Egger with an OR of 0.887 (95% CI: 0.704–1.116, p = 0.316), weighted median at an OR of 0.846 (95% CI: 0.703–1.018, p = 0.076), simple mode with an OR of 0.890 (95% CI: 0.619–1.280, p = 0.536), and weighted mode at an OR of 0.850 (95% CI: 0.711–1.015, p = 0.086). In the case of C-X-C Motif Chemokine 5 (CXCL5), the IVW method calculated an OR of 0.870 (95% CI: 0.778–0.973, p = 0.015, FDR = 0.074). Alternative approach results were: MR-Egger at an OR of 1.004 (95% CI: 0.844–1.194, p = 0.966), weighted median with an OR of 0.970 (95% CI: 0.838–1.123, p = 0.685), simple mode reporting an OR of 0.841 (95% CI: 0.591–1.120, p = 0.347), and weighted mode showing an OR of 0.963 (95% CI: 0.835–1.111, p = 0.611). For Interleukin-24 (IL-24), an OR of 0.780 (95% CI: 0.626–0.970, p = 0.026, FDR = 0.103) was determined by the IVW method. Despite the P value for IL-24 being less than 0.05, its FDR corrected value exceeds the threshold of 0.1. The findings from other methods included: MR-Egger with an OR of 0.992 (95% CI: 0.587–1.675, p = 0.976), weighted median at an OR of 0.725 (95% CI: 0.532–0.988, p = 0.041), simple mode yielding an OR of 0.647 (95% CI: 0.370–1.133, p = 0.147), and weighted mode indicating an OR of 0.688 (95% CI: 0.434–1.090, p = 0.131). These results are visualized in Figure 2 and detailed in Supplementary Table 2.
Figure 2 The forest plot shows the causal associations between inflammatory cytokines and SCZ by forward MR, we mainly used the IVW method. OR < 1 indicates a negative association between exposure and outcome, a noteworthy point that is represented in blue within the graphical data. While OR > 1 indicates a positive association between exposure and outcome, a noteworthy point that is represented in red within the graphical data.
In the reverse MR analysis examining the relationship between SCZ and 91 inflammatory cytokines, we observed the following outcomes: Elevated levels of Leukemia Inhibitory Factor (LIF) were linked to an increased risk of schizophrenia (OR = 1.040, 95% CI: 1.003–1.078, p = 0.033, FDR = 0.165, via IVW). Conversely, reduced levels of Leukemia inhibitory factor receptor (LIF-R) were associated with an augmented risk of schizophrenia (OR = 0.962, 95% CI: 0.929–0.996, p = 0.028, FDR = 0.142, via IVW). Likewise, lower levels of Osteoprotegerin (OPG) correlated with a heightened risk of schizophrenia (OR = 0.969, 95% CI: 0.940–0.999, p = 0.044, FDR = 0.109, via IVW) (refer to Figure 3, Supplementary Table 3). These relationships exhibited neither significant heterogeneity nor evidence of horizontal pleiotropy. However, after FDR adjustment, none of these cytokines remained statistically significant.
Figure 3 The forest plot shows the causal associations between inflammatory cytokines and SCZ by reverse MR, we mainly used the IVW method. OR < 1 indicates a negative association between exposure and outcome, a noteworthy point that is represented in blue within the graphical data. While OR > 1 indicates a positive association between exposure and outcome, a noteworthy point that is represented in red within the graphical data.
To confirm the causal link between inflammatory cytokines and schizophrenia, we conducted sensitivity analyses and detailed the results in the Supplementary Material (Supplementary Tables 4, 5). Q values from MR-Egger and IVW tests indicated no substantial heterogeneity in any significant correlations (all p > 0.05). Additionally, we utilized the MR-Egger intercept and the MR-PRESSO Global test to investigate pleiotropy. Any horizontal pleiotropic outliers identified by MR-PRESSO were removed before re-analysis. Consequently, no evidence of horizontal pleiotropy was found in the association between any inflammatory cytokines and SCZ (all p > 0.05). Subsequent leave-one-out analyses revealed that no individual SNP predominantly influenced the relationship between inflammatory cytokines and SCZ. We illustrated these findings with scatter plot, funnel pot and leave-one analysis (Supplementary Figures S1–S3). Likewise, in reverse analyses, no specific SNP substantially altered the results, as depicted in Supplementary Figures S4, S5.
In this study, we utilized forward and reverse MR analyses which enable an extensive examination of the potential bidirectional causal relationship between inflammatory cytokines and SCZ. The forward MR analysis identified that enhanced levels of particular inflammatory cytokines correlated with a higher risk of SCZ. Conversely, the reverse MR analysis indicated that an elevated risk of SCZ contributed to alterations in the concentrations of certain inflammatory cytokines. These results imply a potential two-way causal relationship, adding complexity to the interplay between inflammatory cytokines and SCZ.
Numerous prior studies have reported that expression levels of inflammatory cytokines play pivotal roles in the pathogenesis and prognosis of SCZ (31). Typically, malignant alterations in SCZ showed abrupt escalations or reductions in the expression levels of these cytokines. Thus, in this study, we intent to profile cytokine and monitor of their expression dynamics to understand the causal relationship between their levels and risk of SCZ. Through screening cytokine data, we initially discovered that abnormal expression of FGF5 might elevate the risk of SCZ. Large-scale genetic association studies have linked SNPs near the FGF gene locus with the emergence of SCZ (32, 33). Additionally, SNPs in the Fibroblast Growth Factor Receptor 1 (FGFR) gene region have demonstrated a statistical correlation with SCZ (34), hinting at possible functional disruptions in the FGF signaling pathway during the development of this disorder. Moreover, postmortem examinations of brain tissues from SCZ patients have revealed variations in FGFR mRNA expression within the hippocampus and additional cerebral regions (35), suggesting FGF signaling dysregulation in individuals diagnosed with SCZ.
Furthermore, we also found that increased cytokine levels may exert a protective effect against SCZ. Our results showed that the expression of CXCL1 have changed in SCZ. Previous study has demonstrated that the upregulation of CXCL1 in certain brain regions, particularly where demyelination occurs, enhanced the growth of oligodendrocyte precursor cells. Then, these oligodendrocyte precursor cells developed into oligodendrocytes that contribute to remyelination and potentially inhibit the progression of the disease (36). Moreover, CXCL1 has the capacity to attract neutrophils to the brain, which partially inhibit autoreactive T cell activities, thus slowing SCZ exacerbation (37). In addition to CXCL1, we also identified substantial associations between the risk of SCZ and fluctuations in the levels of various inflammatory proteins, including CCL4, CXCL5, and IL-24. These findings suggest that these proteins might exert protective effects in the context of SCZ.
In the reverse MR analysis, we observed that an increased risk of SCZ contributed to altered levels of specific inflammatory proteins, including LIF and its receptor (LIF-R), and OPG. The LIF gene resides at chromosomal region 22q12.1-q12.2, and has been identified as a susceptibility locus for SCZ. Substantial evidence links the LIF gene and its SNPs with an increased risk of SCZ. And particular variants of the LIF gene are significantly associated with the diagnosis of schizophrenia, especially in patients with hebephrenic SCZ. LIF also plays a role in the normal inflammatory responses of the central and peripheral nervous systems (38), influencing the activity of astrocytes, oligodendrocytes, microglia, and immune cells. Accumulating studies have identified alterations in the expression levels of LIF and its related cytokines in patients with SCZ, which indicates an augmented neuroinflammatory state that indirectly impacts the disease’s pathological progression (39). Moreover, antipsychotic drugs like chlorpromazine can stimulate the LIF-R, affecting serum LIF receptor levels (9). Unfortunately, following the adjustment for FDR, the statistical significance of these cytokines was not maintained. Additional MR investigations are warranted to validate our results as more extensive GWAS datasets become available.
This study presents potential limitations, with the primary constraint being the reliance on GWAS summary datasets for inflammatory protein traits and SCZ, despite these being the most comprehensive datasets available. Variations in sample size, quality control methodologies, and the ethnic diversity of the study populations could introduce potential biases. The datasets utilized in this research are exclusively derived from European cohorts, and the employment of summary-level data precludes the possibility of individual-level analysis. To provide a more comprehensive perspective, future Mendelian Randomization studies utilizing individual-level data are necessary. This limitation hampers our ability to conduct stratified analyses by demographic variables such as race, gender, and age. Furthermore, despite the application of stringent criteria for linkage disequilibrium pruning (r2 < 0.001, distance > 10,000 kb), the selection of SNPs under a less stringent significance threshold (p < 1×10-5) was necessitated due to the limited number of SNPs achieving genome-wide significance. However, the F-statistics for each SNP were greater than 10, indicating the absence of weak instrument bias. We employed a Benjamini-Hochberg FDR threshold of 0.1 to identify results of significance. This FDR approach, while less conservative than the Bonferroni method, offers a more practical balance between the risk of false positives and the pursuit of novel findings. Future larger-scale GWAS are required to identify more genetic loci associated with inflammatory proteins.
In summary, our study has revealed associations between four inflammatory cytokines and the risk of SCZ. Specifically, we highlight FGF5 as a potential pathogenic risk-enhancing inflammatory factor, while CCL4, CXCL1, and CXCL5 are posited as protective candidate inflammatory cytokines for SCZ. Our findings offer new insights into the inflammatory cytokines to reveal the pathogenesis and therapeutic strategies for SCZ. Focusing on FGF5 as a therapeutic target may herald a significant advancement in the treatment of SCZ, paving the way for more effective and tailored therapeutic approaches. To fully understand the potential roles of these inflammatory cytokines and the corresponding drug targets in the development and progression of SCZ, further studies with larger sample sizes and more comprehensive genetic coverage are warranted, taking into account other potential confounding factors. Future investigations should concentrate on corroborating these discoveries and examining the interactions among additional cytokines and SCZ.
The original contributions presented in the study are included in the article/Supplementary Material, further inquiries can be directed to the corresponding author.
Ethical approval was not required for the study involving humans in accordance with the local legislation and institutional requirements. Written informed consent to participate in this study was not required from the participants or the participants’ legal guardians/next of kin in accordance with the national legislation and the institutional requirements.
YL: Writing – original draft, Writing – review & editing. XZ: Writing – original draft, Writing – review & editing.
The author(s) declare financial support was received for the research, authorship, and/or publication of this article. This research was funded by the “Guangdong Province Educational Science Planning Project (Higher Education Special Program)” (grant code: 2023GXJK414), “Young Innovative Talents Project of University of Guangdong Province” (grant code: 2021KQNCX058).
The authors declare that the research was conducted in the absence of any commercial or financial relationships that could be construed as a potential conflict of interest.
All claims expressed in this article are solely those of the authors and do not necessarily represent those of their affiliated organizations, or those of the publisher, the editors and the reviewers. Any product that may be evaluated in this article, or claim that may be made by its manufacturer, is not guaranteed or endorsed by the publisher.
The Supplementary Material for this article can be found online at: https://www.frontiersin.org/articles/10.3389/fpsyt.2024.1417213/full#supplementary-material
CCL4, C-C Motif Chemokine 4; CXCL1, C-X-C Motif Chemokine 1; CXCL5, C-X-C Motif Chemokine 5; FDR, False Discovery Rate; FGF5, Fibroblast Growth Factor 5; FGFR, Fibroblast Growth Factor Receptor GWAS, Genome-Wide Association Studies; IL, Interleukins; IV, Instrumental Variable; LD, Linkage Disequilibrium; LIF, Leukemia Inhibitory Factor; IVW, Inverse-Variance Weighted; MR, Mendelian Randomization; NGS, Next-Generation Sequencing; OPG, Osteoprotegerin; OR, Odds Ratio; SCZ, Schizophrenia; SNPs, Single Nucleotide Polymorphisms; STROBE-MR, Strengthening The Reporting Of Observational Studies In Epidemiology Using Mendelian Randomization; TNF, Tumor Necrosis Factor.
1. Legge SE, Santoro ML, Periyasamy S, Okewole A, Arsalan A, Kowalec K. Genetic architecture of schizophrenia: A review of major advancements. Psychol Med. (2021) 51:2168–77. doi: 10.1017/S0033291720005334
2. Trifu SC, Kohn B, Vlasie A, Patrichi BE. Genetics of schizophrenia (Review). Exp Ther Med. (2020) 20:3462–8. doi: 10.3892/etm.2020.8973
3. Kato H, Kimura H, Kushima I, Takahashi N, Aleksic B, Ozaki N. The genetic architecture of schizophrenia: review of large-scale genetic studies. J Hum Genet. (2023) 68:175–82. doi: 10.1038/s10038–022-01059–4
4. Jiao S, Cao T, Cai H. Peripheral biomarkers of treatment-resistant schizophrenia: genetic, inflammation and stress perspectives. Front Pharmacol. (2022) 13:1005702. doi: 10.3389/fphar.2022.1005702
5. Gallego JA, Blanco EA, Husain-Krautter S, Madeline Fagen E, Moreno-Merino P, Del Ojo-Jimenez JA, et al. Cytokines in cerebrospinal fluid of patients with schizophrenia spectrum disorders: new data and an updated meta-analysis. Schizophr Res. (2018) 202:64–71. doi: 10.1016/j.schres.2018.07.019
6. Wang AK, Miller BJ. Meta-analysis of cerebrospinal fluid cytokine and tryptophan catabolite alterations in psychiatric patients: comparisons between schizophrenia, bipolar disorder, and depression. Schizophr Bull. (2018) 44:75–83. doi: 10.1093/schbul/sbx035
7. Orlovska-Waast S, Kohler-Forsberg O, Brix SW, Nordentoft M, Kondziella D, Krogh J, et al. Cerebrospinal fluid markers of inflammation and infections in schizophrenia and affective disorders: A systematic review and meta-analysis. Mol Psychiatry. (2019) 24:869–87. doi: 10.1038/s41380–018-0220–4
8. Pillinger T, Osimo EF, Brugger S, Mondelli V, McCutcheon RA, Howes OD. A meta-analysis of immune parameters, variability, and assessment of modal distribution in psychosis and test of the immune subgroup hypothesis. Schizophr Bull. (2019) 45:1120–33. doi: 10.1093/schbul/sby160
9. Momtazmanesh S, Zare-Shahabadi A, Rezaei N. Cytokine alterations in schizophrenia: an updated review. Front Psychiatry. (2019) 10:892. doi: 10.3389/fpsyt.2019.00892
10. Dahan S, Bragazzi NL, Yogev A, Bar-Gad M, Barak V, Amital H, et al. The relationship between serum cytokine levels and degree of psychosis in patients with schizophrenia. Psychiatry Res. (2018) 268:467–72. doi: 10.1016/j.psychres.2018.07.041
11. Marcinowicz P, Wiedlocha M, Zborowska N, Debowska W, Podwalski P, Misiak B, et al. A meta-analysis of the influence of antipsychotics on cytokines levels in first episode psychosis. J Clin Med. (2021) 10:2488. doi: 10.3390/jcm10112488
12. Lesh TA, Careaga M, Rose DR, McAllister AK, Van de Water J, Carter CS, et al. Cytokine alterations in first-episode schizophrenia and bipolar disorder: relationships to brain structure and symptoms. J Neuroinflamm. (2018) 15:165. doi: 10.1186/s12974–018-1197–2
13. Zhang T, Zeng J, Wei Y, Ye J, Tang X, Xu L, et al. Changes in Inflammatory Balance Correlates with Conversion to Psychosis among Individuals at Clinical High-Risk: A Prospective Cohort Study. Psychiatry Res. (2022) 318:114938. doi: 10.1016/j.psychres.2022.114938
14. Dunleavy C, Elsworthy RJ, Upthegrove R, Wood SJ, Aldred S. Inflammation in first-episode psychosis: the contribution of inflammatory biomarkers to the emergence of negative symptoms, a systematic review and meta-analysis. Acta Psychiatr Scand. (2022) 146:6–20. doi: 10.1111/acps.13416
15. Frydecka D, Krzystek-Korpacka M, Lubeiro A, Stramecki F, Stanczykiewicz B, Beszlej JA, et al. Profiling inflammatory signatures of schizophrenia: A cross-sectional and meta-analysis study. Brain Behav Immun. (2018) 71:28–36. doi: 10.1016/j.bbi.2018.05.002
16. Zhu F, Zhang L, Liu F, Wu R, Guo W, Ou J, et al. Altered serum tumor necrosis factor and interleukin-1beta in first-episode drug-naive and chronic schizophrenia. Front Neurosci. (2018) 12:296. doi: 10.3389/fnins.2018.00296
17. Zhang T, Zeng J, Wei Y, Ye J, Tang X, Xu L, et al. Changes in inflammatory markers in clinical high risk of developing psychosis. Neuropsychobiology. (2023) 82:104–16. doi: 10.1159/000528770
18. Halstead S, Siskind D, Amft M, Wagner E, Yakimov V, Shih-Jung Liu Z, et al. Alteration patterns of peripheral concentrations of cytokines and associated inflammatory proteins in acute and chronic stages of schizophrenia: A systematic review and network meta-analysis. Lancet Psychiatry. (2023) 10:260–71. doi: 10.1016/S2215–0366(23)00025–1
19. Borovcanin MM, Janicijevic SM, Jovanovic IP, Gajovic N, Arsenijevic NN, Lukic ML. Il-33/St2 pathway and galectin-3 as a new analytes in pathogenesis and cardiometabolic risk evaluation in psychosis. Front Psychiatry. (2018) 9:271. doi: 10.3389/fpsyt.2018.00271
20. Romeo B, Brunet-Lecomte M, Martelli C, Benyamina A. Kinetics of cytokine levels during antipsychotic treatment in schizophrenia: A meta-analysis. Int J Neuropsychopharmacol. (2018) 21:828–36. doi: 10.1093/ijnp/pyy062
21. Ouyang L, Li D, Li Z, Ma X, Yuan L, Fan L, et al. Il-17 and Tnf-Beta: predictive biomarkers for transition to psychosis in ultra-high risk individuals. Front Psychiatry. (2022) 13:1072380. doi: 10.3389/fpsyt.2022.1072380
22. Reale M, Costantini E, Greig NH. Cytokine imbalance in schizophrenia. From research to clinic: potential implications for treatment. Front Psychiatry. (2021) 12:536257. doi: 10.3389/fpsyt.2021.536257
23. Shnayder NA, Khasanova AK, Strelnik AI, Al-Zamil M, Otmakhov AP, Neznanov NG, et al. Cytokine imbalance as a biomarker of treatment-resistant schizophrenia. Int J Mol Sci. (2022) 23:11324. doi: 10.3390/ijms231911324
24. Levin MG, Burgess S. Mendelian randomization as a tool for cardiovascular research: A review. JAMA Cardiol. (2024) 9:79–89. doi: 10.1001/jamacardio.2023.4115
25. Zhao JH, Stacey D, Eriksson N, Macdonald-Dunlop E, Hedman AK, Kalnapenkis A, et al. Genetics of circulating inflammatory proteins identifies drivers of immune-mediated disease risk and therapeutic targets. Nat Immunol. (2023) 24:1540–51. doi: 10.1038/s41590-023-01588-w
26. Shim H, Chasman DI, Smith JD, Mora S, Ridker PM, Nickerson DA, et al. A multivariate genome-wide association analysis of 10 ldl subfractions, and their response to statin treatment, in 1868 Caucasians. PLoS One. (2015) 10:e0120758. doi: 10.1371/journal.pone.0120758
27. Hemani G, Zheng J, Elsworth B, Wade KH, Haberland V, Baird D, et al. The Mr-base platform supports systematic causal inference across the human phenome. Elife. (2018) 7:e34408. doi: 10.7554/eLife.34408
28. Sanderson E. Multivariable Mendelian randomization and mediation. Cold Spring Harb Perspect Med. (2021) 11:a038984. doi: 10.1101/cshperspect.a038984
29. Burgess S, Bowden J, Fall T, Ingelsson E, Thompson SG. Sensitivity analyses for robust causal inference from Mendelian randomization analyses with multiple genetic variants. Epidemiology. (2017) 28:30–42. doi: 10.1097/EDE.0000000000000559
30. Wu F, Huang Y, Hu J, Shao Z. Mendelian randomization study of inflammatory bowel disease and bone mineral density. BMC Med. (2020) 18:312. doi: 10.1186/s12916–020-01778–5
31. Ermakov EA, Mednova IA, Boiko AS, Buneva VN, Ivanova SA. Chemokine dysregulation and neuroinflammation in schizophrenia: A systematic review. Int J Mol Sci. (2023) 24:2215. doi: 10.3390/ijms24032215
32. Talaei A, Farkhondeh T, Forouzanfar F. Fibroblast growth factor: promising target for schizophrenia. Curr Drug Targets. (2020) 21:1344–53. doi: 10.2174/1389450121666200628114843
33. Klimaschewski L, Claus P. Fibroblast growth factor signalling in the diseased nervous system. Mol Neurobiol. (2021) 58:3884–902. doi: 10.1007/s12035–021-02367–0
34. Narla ST, Lee YW, Benson CA, Sarder P, Brennand KJ, Stachowiak EK, et al. Common developmental genome deprogramming in schizophrenia - role of integrative nuclear fgfr1 signaling (Infs). Schizophr Res. (2017) 185:17–32. doi: 10.1016/j.schres.2016.12.012
35. Parthasarathy G, Pattison MB, Midkiff CC. The Fgf/Fgfr system in the microglial neuroinflammation with Borrelia burgdorferi: likely intersectionality with other neurological conditions. J Neuroinflamm. (2023) 20:10. doi: 10.1186/s12974-022-02681-x
36. Ermakov EA, Melamud MM, Buneva VN, Ivanova SA. Immune system abnormalities in schizophrenia: an integrative view and translational perspectives. Front Psychiatry. (2022) 13:880568. doi: 10.3389/fpsyt.2022.880568
37. Korbecki J, Gassowska-Dobrowolska M, Wojcik J, Szatkowska I, Barczak K, Chlubek M, et al. The importance of cxcl1 in physiology and noncancerous diseases of bone, bone marrow, muscle and the nervous system. Int J Mol Sci. (2022) 23:4205. doi: 10.3390/ijms23084205
38. Metcalfe SM. Neuroprotective immunity: leukaemia inhibitory factor (Lif) as guardian of brain health. Med Drug Discovery. (2019) 2:100006. doi: 10.1016/j.medidd.2019.100006
Keywords: schizophrenia, inflammatory cytokines, Mendelian randomization, immunity, psychiatry disorder
Citation: Li Y-T and Zeng X (2024) Circulating inflammatory cytokines influencing schizophrenia: a Mendelian randomization study. Front. Psychiatry 15:1417213. doi: 10.3389/fpsyt.2024.1417213
Received: 14 April 2024; Accepted: 10 June 2024;
Published: 24 June 2024.
Edited by:
Sergi Papiol, Ludwig Maximilian University of Munich, GermanyReviewed by:
Tamilanban T., SRM Institute of Science and Technology, IndiaCopyright © 2024 Li and Zeng. This is an open-access article distributed under the terms of the Creative Commons Attribution License (CC BY). The use, distribution or reproduction in other forums is permitted, provided the original author(s) and the copyright owner(s) are credited and that the original publication in this journal is cited, in accordance with accepted academic practice. No use, distribution or reproduction is permitted which does not comply with these terms.
*Correspondence: Xuezhen Zeng, emVuZ3h6aDdAbWFpbC5zeXN1LmVkdS5jbg==
Disclaimer: All claims expressed in this article are solely those of the authors and do not necessarily represent those of their affiliated organizations, or those of the publisher, the editors and the reviewers. Any product that may be evaluated in this article or claim that may be made by its manufacturer is not guaranteed or endorsed by the publisher.
Research integrity at Frontiers
Learn more about the work of our research integrity team to safeguard the quality of each article we publish.