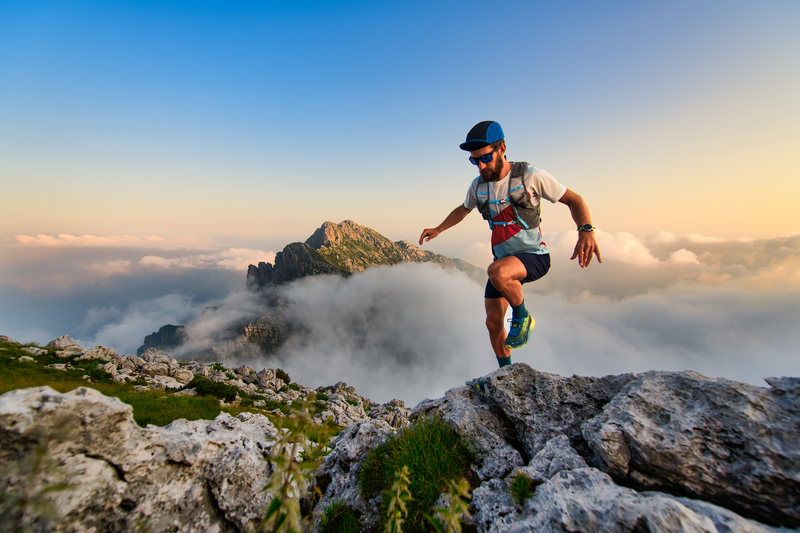
95% of researchers rate our articles as excellent or good
Learn more about the work of our research integrity team to safeguard the quality of each article we publish.
Find out more
ORIGINAL RESEARCH article
Front. Psychiatry , 15 July 2024
Sec. Adolescent and Young Adult Psychiatry
Volume 15 - 2024 | https://doi.org/10.3389/fpsyt.2024.1402680
This article is part of the Research Topic Mind-body medicine and its impacts on psychological networks, quality of life, and health - Volume II View all 39 articles
Background: Anxiety, depression, and sleep problems are prevalent comorbid mental disorders among university students. The World Health Organization (WHO) emphasized a mental health promotion objective, recommending the consideration of protective health-promoting factors in strategies aimed at preventing mental disorders. Integrating theoretically significant constructs (such as protective factors) enhances our comprehension of the intricate mechanisms that underpin mental disorders. This study employed network analysis to first identify core and bridge symptoms within comorbid mental disorders and then explore how health-promoting lifestyles (HPLs) were associated with these disorders. The ultimate goal is to offer health promotion recommendations to enhance students’ quality of life.
Methods: A total of 3,896 qualified university students participated in this study. Anxiety, depression, sleep problems, and HPLs were assessed using the GAD-7, PHQ-9, PSQI, and HPLP-II scales. A Gaussian Graphical Model was used to construct the networks. The Network Comparison Test was applied to determine whether the associations between HPLs and comorbid symptoms vary by gender, educational level, family sibling, and mental health status.
Results: Low energy (PHQ4) had the highest strength centrality, followed by Daytime dysfunction (PSQI7) and Trouble relaxing (GAD4). Five bridge symptoms were identified: Daytime dysfunction (PSQI7), Self-harm even suicide (PHQ9), Sad mood (PHQ2), Low energy (PHQ4), and Feeling afraid (GAD7). Regarding protective HPLs, Physical activity, Spiritual growth, and Stress management generally emerged as the top three central mental health-promoting behaviors.
Conclusion: Targeting core and bridge symptoms with timely and appropriate interventions can alleviate anxiety, depression, and sleep problems in this population. Moreover, promoting physical activity, fostering spiritual growth, and managing stress are likely to significantly enhance the overall mental health of university students.
The mental health of university students has garnered growing attention. Faced with multifaceted pressures such as academics, social interactions, and career choices, more university students are taking leaves of absence or dropping out due to mental disorders (1). The Global Burden of Disease (GBD) Study highlighted the anxiety and depression have emerged as significant mental health challenges on a global scale (2). According to a systematic review conducted in 2022, the prevalence of anxiety and depression among university students has escalated to 39.0% and 33.6%, respectively (3). Importantly, sleep problems often occur concurrently with anxiety and depression. Since the revision of DSM-III-R by the American Psychiatric Association (APA), sleep disorder has been delineated as a distinct and specific category within DSM-IV, DSM-IV-TR, and DSM-5 (4). A meta-analysis among Chinese university students found a 25.7% prevalence of sleep disorders, with 20.3% dissatisfied with sleep quality and 23.6% exhibiting insomnia (5). During episodes of depression, university students suffer from diminished sleep quality, longer sleep onset latency, and increased daytime sleepiness (6). Furthermore, sleep problems and anxiety may be mediated by a pathogenic mechanism involving hyperarousal, influenced by imbalances in neurotransmitters such as cholinergic and gamma-aminobutyric acid (GABA) (7). In short, mental disorders typically co-occur in individuals rather than manifest independently, with those reporting depression and anxiety simultaneously exhibiting more sleep disturbances (8), a phenomenon referred to as comorbidity in clinical research.
Mental disorders also constitute a risk factor for self-harm and even suicide. Data from the International College Student Health Survey showed that approximately one-third of full-time student respondents have experienced at least one DSM-IV mental disorder (9). Compared to the general adult population, university students exhibit significantly higher rates of suicidal ideation (24% vs 9%) and suicide attempts (9% vs 2.7%) (10). Studies have proved that university students face greater challenges in mental health, highlighting the importance of conducting in-depth research on disorders such as anxiety, depression, and sleep. However, previous research on university students’ mental health has primarily relied on total scores from screening scales, often neglecting individual symptoms and the interactions between these symptoms. The network theory of mental disorder (NTMD), as proposed by Borsboom, offers a new perspective to better understand this complexity (11). This theory posits that the persistence of mental disorders are contingent upon the causal relationships among their symptoms. Significant causal relationships may potentially lead to feedback loops among symptoms, perpetuating and exacerbating each other. Using network analysis methods (NAM) in NTMD, symptoms are constructed into an interconnected network where central symptoms are more likely to trigger other symptoms, thereby playing a pivotal role in a disordered state of disease (12). Intervening on central symptoms may accelerate the deactivation of symptom interactions, thus improving peripheral symptoms, which holds considerable practical value (13). Current studies have widely applied this approach to investigate the network structure of mental symptoms among various populations, including the elderly, chronic disease patients, and healthcare workers (14–16).
From a universal health perspective, the World Health Organization (WHO) has proposed a mental health promotion objective, emphasizing that supportive social environment and healthy daily habits can help reduce the incidence of mental illnesses. Moreover, among five primary determinants of human health, lifestyle and behavioral factors contribute 60% (17). Health-promoting lifestyles (HPLs) refer to the multi-dimensional, spontaneous, and sustained daily activities undertaken with the aim of enhancing or promoting individual health and well-being (18). In clinical treatment, promoting the adoption of this adaptive health-promoting lifestyle is also considered a highly cost-effective and potentially applicable non-pharmacological intervention that may alleviate stress and depression (19). It showed that increasing high-intensity physical activity can alleviate the severity of mental health issues or reduce their occurrence among student populations (20). As a factor that promotes better sleep quality, cultivating healthy lifestyle habits is also an effective strategy for improving sleep (21). Certain lifestyles that emphasize mental growth, stress management, and interpersonal relationships are also negatively correlated with anxiety and depression (22). However, the prevalence of healthy behaviors among university students is currently suboptimal, with a considerable number engaging in health-risk behaviors and adopting unhealthy lifestyles (23). Hence, a comprehensive investigation into the correlation between depression, anxiety, sleep problems, and their protective factors (health-promoting lifestyles) among university students is necessary to better enhance their overall health.
Recently, researchers have also suggested moving beyond symptom measurement within networks. They propose including not only psychiatric symptoms but also a wider range of theoretically relevant components (such as risk or protective factors), to further understand the complex mechanisms promoting or preventing the development of mental disorders (24, 25). Risk factors provide valuable insights into understanding mental illness, yet the importance of protective factors is equally significant. For example, in a sample of 85 remitted depressed patients, resilience was shown to be an important protective factor, predicting fewer residual depression symptoms and less ruminative thinking (26). Eduardo and colleagues examined the relationship between adolescent suicidal behavior and socio-emotional indicators during adolescence, revealing the potential effectiveness of self-esteem and personal well-being in reducing suicide risk (27). By integrating potential health-promoting lifestyle choices with anxiety, depression, and sleep problems, the network model provides a more comprehensive approach to investigate the protective factors operating at the crossroads between health promotion and the development of mental disorders. Furthermore, it’s worthwhile to consider the variances concerning gender, educational level, familial sibling, and mental health status. Currently, multi-group network analysis has been developed to use the Network Comparison Test (NCT) based on resampling permutation tests to compare global strengths and edge connections between subgroups (28).
Recognizing the complexity of mental health challenges, our first aim is to (a) identify the symptoms that play significant roles in activating, maintaining, and connecting anxiety, depression, and sleep problems within the psychopathology network. Considering the continuously evolution of psychiatric research, our second aim is to (b) explore how comorbid symptoms and protective HPLs are interconnected, discover the central mental health-promoting behaviors, and investigate whether these associations vary by gender, educational level, familial sibling, and mental health status. Ultimately, this may better help educators and healthcare professionals develop evidence-based, more precise health promotion and non-pharmacological intervention strategies to address the mental health problems of university students.
This cross-sectional study, conducted from January 13 to March 18, 2022, was supported by the Psychological Health Education Center at Zhengzhou University. In China, the surveyed location was a Ministry of Education-designated world-class university and a first-class discipline construction university, consistently ranking among the top five nationwide in student enrollment each year, thereby ensuring the representativeness of the survey sample. We employed a multistage sampling method, using seven academic categories (e.g., Medicine, Science, Engineering) as primary units, and degree types (undergraduate and graduate) as secondary units, with stratified sampling throughout. Subsequently, classes served as third-stage units, with cluster sampling randomly selecting students from several classes within each combination of discipline and degree type. Similar to other studies (29, 30), the “Questionnaire Star” program integrated into WeChat was adopted to collect data. An electronic questionnaire was disseminated to 4,952 currently enrolled university students, resulting in the collection and inclusion of 4,698 responses, yielding a response rate of 94.87%. The questionnaire was distributed via the WeChat and Tencent QQ platforms. To ensure data quality, measures implemented: restricting each IP address to a single submission, excluding responses with completion times shorter than 240 seconds or longer than 10 minutes, eliminating incomplete questionnaires, and discarding those with contradictory answers. As a result, a total of 3,896 eligible participants were included. Prior to participation, all subjects provided electronic informed consent. This study received approval from the Ethics Committee of Zhengzhou University (ZZUIRB2022–06).
Power analysis was commonly employed to ascertain the requisite sample size for a study. According to Epskamp and Fried’s research (31), we utilized the netSimulator function to simulate data within a given network model and anticipated network structure, thereby exploring the appropriate sample size necessary for detecting genuine effect sizes. As the network encompasses a greater number of nodes, a larger sample size is necessitated to achieve an equivalent level of reproducibility. Consequently, we conducted data simulations for a network model encompassing comorbid symptoms and health-promoting lifestyles, with the result shown in Supplementary Figure S1. And when N = 1600, the network’s correlation and strength were above 0.9, and the effects on other metrics were also acceptable (above 0.7).
Using the 7-item Generalized Anxiety Disorder Scale (GAD-7) to measure the severity of anxiety symptoms (32). This scale includes seven items that correspond to the DSM-IV criteria for generalized anxiety disorder, with each item rated on a scale from 0 (not at all) to 3 (nearly every day). The total score ranges from 0 to 21, with higher scores representing more severe anxiety symptoms, while a score of 5 or above is classified as mild anxiety. The Chinese adaptation of the GAD-7 has undergone thorough validation, establishing its efficacy as a screening tool for anxiety within the general Chinese population (33).
Depression symptoms were evaluated using the Patient Health Questionnaire-9 (PHQ-9) (34), which is based on the nine Diagnostic and Statistical Manual of Mental Disorders-IV (DSM-IV) criteria for major depression disorder (35). Participants responded to the items on a 4-point Likert scale ranging from 0 (not at all) to 3 (most of the time or always), resulting in a total score ranging from 0 to 27 across the nine items. For PHQ-9 scores, higher total scores represent more severe depression symptoms, with a score of 5 or above indicating mild depression. Extensively validated in the Chinese population (36), the PHQ-9 serves as an effective tool for assessing depression symptoms. Most previous studies have focused on sleep duration and insomnia symptoms (e.g., difficulty falling asleep or staying asleep), while paying little attention to sleep dimensions (e.g., sleep efficiency, daytime dysfunction, and sleep medication) (37). Therefore, we excluded the third item from this scale and employed the Pittsburgh Sleep Quality Index (PSQI) to comprehensively understand the complexity of sleep problems.
The Pittsburgh Sleep Quality Index (PSQI) was used to assess the severity of sleep problems (38). Comprising 19 self-assessment items, the PSQI includes seven dimensions: (1) “Subjective Sleep Quality”; (2) “Sleep Latency”; (3) “Sleep Duration”; (4) “Sleep Efficiency”; (5) “Sleep Disturbance”; (6) “Use of Sleep Medication”; and (7) “Daytime Dysfunction”. In the Chinese population, a global PSQI score exceeding 7 indicates of poor sleep quality, with a sensitivity of 98.3% and specificity of 90.2% (39).
The Health-Promoting Lifestyle Profile (HPLP) was developed to quantify the frequency of participation in health-promoting behaviors (40). The HPLP-II was a revision of the HPLP, consisting of a total of 52 items across six dimensions: interpersonal relationship, health responsibility, stress management, nutrition, physical activity, and spiritual growth. It employed a Likert 4-point scoring system, with higher total scores indicating better health behaviors. The Chinese version of the HPLP-II was revised to include 40 items and has been effectively validated in the Chinese population (41). This version was employed in our survey.
All statistical analyses were performed using the R-Studio program (version 4.3.2). First, descriptive statistical methods were used to summarize demographic information and item scores from each scale. Then, we constructed a comorbid network of anxiety, depression, and sleep problems, in addition to a comprehensive network of comorbid symptoms and their association with health-promoting lifestyles. Network analysis can establish bivariate relationship among multiple variables and reveal the structure and nature of entire complex system of mental disorders caused by the causal interactions among variables (including core components, co-occurrence relationships, and critical nodes), which cannot be explained by regression analysis and latent variable analysis (42).
Before constructing the networks, potential item redundancy was checked using the goldbricker function from the R package networktools (version 1.5.1). Following Jones’ manual (43), if the proportion of significantly different central correlations between two variables and other items is less than 25%, then it can be confirmed that these two items measure the same trait or symptom (i.e., redundancy).
The Pairwise Markov Random Field (PMRF) is commonly employed in cross-sectional studies. Given the continuous nature of our dataset, we utilized the Gaussian Graphical Model (GGM) to estimate a network of partial correlation coefficients (44). The partial correlation network is a type of model based on weighted correlation networks. Partial correlation coefficient denotes the correlation between two nodes while holding all other information in the network constant, hence also referred to as “conditional independence correlations”. To mitigate spurious connections and enhance the network’s comprehensibility, we applied the graphical least absolute shrinkage and selection operator (LASSO) for regularization (45). The Extended Bayesian Information Criterion (EBIC) was used to identify the optimal model fit with a default tuning parameter of 0.5 (46). Additionally, using the Fruchterman-Reingold algorithm to compute the optimal layout of the network, nodes with stronger correlations are positioned closer together, while nodes with weaker correlations are mutually exclusive. For both network estimation and visualization, we relied on the R packages qgraph (version 1.9.8) and bootnet (version 1.5.6) (44, 47). In graphs, each item is represented as a node, and the connections between nodes are termed edges. The thickness of the edges represents the strength of the association, while the color indicates the direction: green for positive correlations and red for negative correlations.
Quantifying the significance of each node within a network necessitates the computation of centrality metrics, which elucidate the probability that the activation of one node will exert an influence on others (48). Commonly employed measures of node centrality encompass betweenness, closeness, and strength. Nevertheless, prior studies have demonstrated that betweenness and closeness are unreliable for ascertaining node importance (44, 49). Consequently, this study employed strength as the centrality metric. Strength centrality evaluates the aggregate absolute edge weights between a node and all its directly connected nodes, thereby describing the robustness of direct connections between nodes in the absence of intermediary nodes. Hence, nodes with higher strength can be interpreted as more central. Furthermore, bridge centrality metrics (such as bridge strength, bridge closeness, and bridge betweenness) were also evaluated using the bridge function in the R package networktools (version 1.5.1) to identify specific nodes that act as conduits connecting different communities within the network (50). Bridge strength quantifies the total absolute edge weight between a community node (such as “physical activity” in health-promoting lifestyles) and all other nodes outside its community. Finally, the R package mgm (version 1.2–14) was employed to calculate predictability, which indicates the extent to which the variation of a given node in the network can be predicted by the variation of its connected nodes (51). In the network layout, the area within the surrounding cycle of each node represents its predictability value.
We assessed network stability and accuracy using the bootstrap method in the R package bootnet (version 1.5.6), relying on the following three processes. To ensure the stability of strength and bridge strength, we initially implemented a case-dropping bootstrap procedure (44). This involved selectively discarding portions of data without inducing significant alterations in the existing network, thus affirming its stability. Stability was quantified by computing the Correlation Stability Coefficient (CS-C), indicating the maximum sample proportion that could be removed. Typically, a CS-C value of 0.25 or higher is preferred, with values exceeding 0.5 considered particularly desirable (52). Subsequently, to assess the accuracy of edge weights, we employed a non-parametric bootstrap method to derive confidence intervals (CIs) (53). A narrower confidence interval signifies a more reliable network estimation. Finally, to evaluate potential disparities between edges or nodes, we conducted bootstrapped paired differences in edge weights and centralities of different nodes (44).
To explore potential variances in network characteristics related to comorbid symptoms and health-promoting lifestyles among university students based on gender, educational level, family sibling, and mental health status, we classified gender as “Male” and “Female”; education level as “Undergraduate” and “Graduate”; family sibling status as “Only-child” and “Non-only-child”; mental health status as “Non-mentally disordered” and “At least one mental disorder”. Then, we applied a permutation test with 1000 iterations using the R package Network Comparison Test (version 2.2.2) to determine if there are statistical differences in global strength (the absolute sum of all edge weights) as well as network structure (the distributions of edge weights) among subgroups (28). Subsequently, following the adjustment for multiple comparisons using the Bonferroni-Holm correction, the differences in strength at the level of individual edges between both networks were compared.
The demographic characteristics of the overall sample are presented in Table 1. Among the 3,896 participants included in this study, the mean age was 21.92 years (SD = 2.56), with 1,587 (40.7%) being male and 2,309 (59.3%) female. Of these, 1,824 (46.8%) were undergraduates, and 2,072 (53.2%) were graduate students. Altogether, 1,485 (38.1%) reported experiencing anxiety (GAD-7 total score ≥ 5), 1,745 (44.8%) reported experiencing depression (PHQ-9 score ≥ 5), and 629 (16.1%) reported experiencing sleep problems (PSQI total score ≥ 8). Supplementary Table S1 displays the mean, standard deviation (SD), skewness, kurtosis, strength, bridge strength, and predictability of all items across the scales. In both the comorbid network of anxiety, depression, and sleep problems, and the comprehensive network of comorbid symptoms and health-promoting lifestyles, no item was found to be redundant with any other item (i.e., showing less than 25% significantly different correlations).
As illustrated in Figure 1, within the network structure encompassing anxiety, depression, and sleep problems, there are a total of 131 non-zero edges out of 231 possible edges (network density = 0.57), with an average weight of 0.043. The five most prominent edges are all within specific communities: Sleep duration - Sleep efficiency (PSQI3 - PSQI4), Subjective sleep quality - Daytime dysfunction (PSQI1 - PSQI7), Nervousness - Uncontrollable worry (GAD1 - GAD2), Anhedonia - Low energy (PHQ1 - PHQ4), and Abnormal behavior & speech - Self-harm even suicide (PHQ8 - PHQ9) (Supplementary Table S2). Significant edges connecting different communities include Self-harm even suicide - Use of sleep medication (PHQ9 - PSQI6), Low energy - Daytime dysfunction (PHQ4 - PSQI7), and Feeling afraid - Self-harm even suicide (GAD7 - PHQ9) (Supplementary Table S2). Additionally, Excessive worry (GAD3) and Trouble relaxing (GAD4) exhibit the highest predictability (0.747, 0.742), whereas Use of sleep medication (PSQI6) has the lowest predictability (0.173). The average predictability is 0.56, indicating that, on average, more than half of the variance in the nodes can be explained by their adjacent nodes (Supplementary Table S1). Network stability and accuracy tests are shown in Supplementary Figure S4, with both strength and bridge strength centrality being 0.75, exceeding the recommended threshold of 0.5.
The centrality plot (Figure 2A) indicated that Low energy (PHQ4) exhibits the highest strength centrality, followed by Daytime dysfunction (PSQI7) and Trouble relaxing (GAD4), suggesting that these nodes occupy the most significant and influential positions within the comorbidity symptom network. According to Jones’ perspective (50), bridge symptoms are selected using the 80th percentile threshold for bridge centrality. As shown in Figure 2B, Daytime dysfunction (PSQI7), Self-harm even suicide (PHQ9), Sad mood (PHQ2), Low energy (PHQ4), and Feeling afraid (GAD7) exhibit the highest bridge strength, identifying them as the pivotal bridge symptoms linking anxiety, depression, and sleep problems comorbidity. The bootstrapped difference test (Supplementary Figure S4) for node strength and bridge strength further corroborate that these nodes are statistically significantly stronger than other nodes within the network.
Figure 2 Standardized strength and bridge strength centrality of network structure of anxiety, depression and sleep problems among university students (z-scores). (A) strength centrality; (B) bridge strength centrality.
The network of comorbid symptoms and health-promoting lifestyles is illustrated in Figure 3, with stability and accuracy tests presented in Supplementary Figure S5. Regarding strength centrality, Low energy (PHQ4), Daytime dysfunction (PSQI7), and Trouble relaxing (GAD4) remain the most significant and influential comorbid symptoms, while Physical activity, Health responsibility, and Stress management are identified as the top three central HPLs (Supplementary Figure S2). In terms of correlations, Spiritual growth and Physical activity exhibit the most negative edges with comorbid symptoms. Moreover, by evaluating the individual connection between each specific protective HPL node and the wider community of comorbid symptoms, Physical activity demonstrated the highest bridge strength. Specifically, Spiritual growth showed the strongest bridge strength with the depression community, Physical activity with the sleep problems community, and both Health responsibility and Stress management with the anxiety community (Supplementary Table S3; Supplementary Figure S3). However, as Health responsibility showed mostly positive correlations with anxiety symptoms. Thereby, Physical activity, Spiritual growth, and Stress management were identified as the central mental health-promoting behaviors.
Figure 3 Network structure of comorbid symptoms and health-promoting lifestyles among university students.
NCT revealed the invariances of global strength and network structure among the four groups (Supplementary Figures S6–S9). In terms of global strength comparisons across the four subsamples, significant difference was only found in mental health status (non-mentally disordered: 8.72 vs at least one mental disorder: 13.21, p = 0.01). Other comparisons showed no significant differences (male: 13.19 vs female: 12.99, p = 0.634; undergraduate: 12.98 vs graduate: 13.43, p = 0.139; only-child: 13.23 vs non-only-child: 13.52, p = 0.733). Regarding network structure comparisons, significant differences were observed in familial sibling status (M = 0.16, p = 0.030) and mental health status (M = 0.28, p = 0.01); The other two subsamples showed no significant differences (gender: M = 0.13, p = 0.129; educational level: M = 0.12, p = 0.158). Additionally, after applying the Bonferroni-Holm correction, all edge weights in the three subsamples remained non-significant (p > 0.05).
In the present study, we evaluated the core and bridge symptoms within comorbid network of anxiety, depression, and sleep problems, and identified central mental health-promoting behaviors. Consistent with epidemiological findings in university student populations, at least one in three individuals reported anxiety or depression, and one in every five to six individuals experienced sleep problems. Investigating the relationship between sleep problems and anxiety and depression from sleep dimensions (e.g., sleep efficiency, daytime dysfunction) provides a deep understanding of the complexity of mental health. Health promotion involves enabling individuals to achieve optimal physical and mental well-being, live under healthy habits, and make choices conducive to health (54). By applying a more comprehensive network approach, we can intricately analyze the associations between health-protective factors and specific mental disorders. In the following, we discuss the results of the networks.
Low energy (PHQ4) exhibited the highest strength centrality, indicating its crucial role in activating and sustaining the comorbid network of depression, anxiety, and sleep problems. This phenomenon was also widely observed among Chinese nurses and nursing students and closely associated with Anhedonia (PHQ1) (55, 56). Low energy, manifesting as fatigue or exhaustion, is a core feature of depression (57). During the late stages of the COVID-19 pandemic, the prevalence of fatigue among nursing students reached 67.3% (58). Evidence suggested that this may be associated with the somatization of psychological distress, particularly prevalent among Asian adolescents. This cultural tendency favors physical expression over verbal expression as a coping strategy (55). The cumulative impact of intense academic pressures, limited recreational and social activities, and inadequate sleep on their physical and mental health often manifested as a state of low energy. Ariel et al. found that low energy as a crucial predictor across multiple domains of health and functional impairment, corroborating our findings (59). It may also serve as an early indicator of anhedonia. Neurological evidence indicated that energy depletion may inhibit the neural systems responsible for enhancing the reception of rewarding stimuli (60). Furthermore, as the second most central symptom, Daytime dysfunction (PSQI7) showed strong edge connectivity with Low energy (PHQ4), serving as bridge symptoms. This supported findings from studies on Macau residents, migrant Filipino domestic workers, and clinicians with depression symptoms (61–63). It has been identified as a prominent bridge symptom linking childhood trauma, sleep disorders, and depression in adolescents (64). Exploratory research into sleep structure revealed that sleep problems could be categorized into nocturnal symptoms and daytime consequences, both contributing to dissatisfaction with sleep quantity or quality. However, interference with daytime functioning was centrally positioned and may be a critical outcome measure in insomnia treatment (65). Related studies indicated that students with low energy are more sensitive to stressors, amplifying the negative consequences of insomnia rather than the experience of insomnia itself (66, 67). The clinical significance of Trouble relaxing (GAD4) has been well-documented in insomnia and psychopathology (59). Notably, trouble relaxing was highly correlated with restlessness and clusters within the psychomotor community. Our results also showed that these symptoms have the highest predictability within the network, corroborating Bai et al.’s finding that difficulty relaxing ranks second in EI, with restlessness showing the highest BEI after sleep problems (63). The inability to relax as a core nexus symptom in comorbid PTSD, depression, and anxiety among trauma-exposed samples (68). Outside the classroom and internship settings, interventions such as mindfulness-based approaches and relaxation exercises can alleviate students’ physical and mental stress (69).
Node bridge strength centrality may provide insights for identifying critical bridge symptoms in the connection and progression of mental disorders. Beyond low energy and daytime dysfunction, Self-harm even suicide (PHQ9), Sad mood (PHQ2), and Feeling afraid (GAD7) also emerged as bridge symptoms within the current network. As a global health concern, Self-harm even suicide (PHQ9) has consistently been recognized as a key intervention target within adolescent mental disorders (70). Numerous scholars have probed into the nexus between adolescent mental health and suicidal ideation, revealing anxiety as a prospective predictor of suicidal ideation; over 50% of completed suicides and 20%-48% of suicide attempts are attributed to depression (71). We also identified that within the depression community, suicidal ideation is most strongly associated with abnormal behavior & speech (i.e., psychomotor agitation and impulsivity). Among adults experiencing major depression episodes, risk-taking behavior and psychomotor impulsivity were believed to facilitate the transition from suicidal ideation to suicide attempts (72). Our study also identified Feeling afraid (GAD7) as another significant bridge symptom, closely related to suicidal ideation. Epidemiological investigations signify that 75% of lifetime mental disorders manifest before the age of 24, with anxiety disorders typically emerging from early adolescence to young adulthood (73). The factors unique to college students, such as student debt, employment uncertainty, academic pressure, and separation from family, showed that feeling afraid doubles the likelihood of suicidal ideation among those experiencing anxiety symptoms (74). Additionally, Sad mood (PHQ2) played a distinct bridge role in linking anxiety disorders, as corroborated by a bayesian network of anxiety-depression bridges (75). Stigma toward mental illnesses could also lead to the emergence of adolescent emotional problems (76).
The “Healthy China Action (2019–2030)” plan, formulated by the Healthy China Initiative Committee emphasizes the “big health” concept, integrating prevention and treatment through 15 major health promotion actions, shifting the focus from disease-centric to health-centric approaches. This offered valuable insights for our research. We found that physical activity occupied a central position in the network, potentially at the intersection of mental disorders and health. It remained the most prominent health node when quantifying the degree to which specific health-promoting behaviors correlate with changes in comorbid symptoms. Specifically, physical activity showed a significant negative correlation with sleep problems, potentially reducing the use of hypnotics, alleviating daytime dysfunction, and improving subjective sleep quality. Numerous scholars have corroborated our findings. It enhanced dopamine levels, altering responses to emotional events, and serving as a stress relief mechanism to help individuals return to pre-stress levels more quickly (77, 78). Moreover, adhering to scientific and effective physical exercise can regulate serum cortisol to optimal levels, boost immunity, and serum cortisol is closely linked to the body’s circadian rhythm (79). Studies also indicated that while body temperature decreases when falling asleep, evening exercise initially raises deep body temperature and accelerates the rate of temperature decline, thereby improving sleep (80). It’s noteworthy that whether a U-shaped relationship exists between physical activity and mental health (81), or the optimal frequency and intensity, requires further research to ascertain.
Spiritual growth can significantly reduce levels of depression. It’s strongly negatively correlated with self-harm even suicide, and worthlessness, marking these as the strongest negative edges within the network. Spiritual growth encompasses pursuing long-term life goals, experiencing oneself as positively developing and changing, gaining a sense of belonging, and maximizing the potential for a healthy life through goal-directed efforts (41, 82). Importantly, signs of depression are also characteristic of a lack of spirituality, Leung found that spiritual development significantly reduced depression, anxiety, and stress in adolescents while enhancing their self-confidence (83). It has been observed that young individuals experiencing depression often exhibit intrusive rumination, characterized by involuntary and repetitive contemplation of negative thoughts, which exacerbates their suffering (84). In contrast, spiritual growth can facilitate deliberate rumination, where individuals purposefully reflect on and analyze traumatic events to understand their meaning and impact, thereby promoting psychological growth (85). Additionally, self-efficacy is closely related to spiritual growth and can be viewed as an outward manifestation of it. It also serves as a mediator through which spiritual growth positively influences mental health, significantly reducing symptoms of depression, anxiety, and externalizing behaviors (86). As the third major health-promoting node, stress management showed a significant negative correlation with anxiety symptoms, alleviating trouble relaxing and restlessness. In this study, trouble relaxing was identified as a core symptom of anxiety, and restlessness was closely linked to it. This illustrates how stress management, by targeting core symptoms, can effectively and directly mitigate anxiety. Adolescents who frequently employ stress management strategies and adopt more positive coping and defense mechanisms exhibit fewer anxiety symptoms over time, underscoring the importance of incorporating stress management techniques into mental health programs (87).
The NCT analysis and quantification of centrality indices have confirmed the universal applicability of stress management, physical activity, and spiritual growth as key health-promoting factors for students. Nonetheless, some distinctions emerged when comparing the intensity levels of health-promoting behaviors’ effects on mental health symptoms across subgroups. Regarding gender, we found no association between stress management and anxiety in males, while physical activity significantly reduced anxiety and improved sleep quality. This implies that men often employ ineffective strategies for managing stress. Traditional masculine traits, which emphasize strength and control, condition men to suppress vulnerable emotions like fear and sadness, a habit persisting into adulthood (88). Additionally, a report found that men more frequently engage in regular exercise compared to women (89). For graduate students, stress management was the most central health-promoting behavior, surpassing physical activity. Among only-children, stress management strategies were more effective in alleviating anxiety compared to those with siblings. We hypothesize that for individuals experiencing greater and more complex stress, targeted stress management strategies are more direct and effective in reducing psychological issues in the short term. Our findings align with epidemiological surveys on students’ mental health (90–92). A higher proportion of women (41.14%) than men (33.71%) experienced anxiety. Anxiety severity was higher among graduate students (8.32) compared to undergraduates (8.09), and higher among only-children (8.75) compared to those with siblings (8.03). Notably, maintaining health responsibility (i.e., continuously attending to physical and mental health and remaining vigilant for potential illnesses) can counterintuitively exacerbate anxiety among women and graduate students. While this represents a form of health responsibility, over attention to health issues in high-stress populations can amplify anxiety, a phenomenon known as “health anxiety”. When classified by clinical scores, university students with at least one mental disorder exhibited significantly higher network density (0.44) compared to those without mental disorders (0.33). This elevated connectivity reflects the complexity and interconnection of mental disorders. Moreover, within the network of students experiencing mental disorders, stronger associations were observed among Abnormal behavior & speech - Self-harm even suicide, Spiritual growth - Worthlessness, and Stress management - Restlessness. Self-harm even suicide emerged as the second most central symptom node, following Low energy. Consistent with study on female nursing students (55), this finding suggested that psychomotor symptoms (such as psychomotor agitation/impulsivity and restlessness) and worthlessness may trigger connections within the network and significantly predict suicidal ideation. This also indirectly supports the notion that targeted interventions in stress management and spiritual growth are more directly effective under severe mental disorders.
This study, based on Chinese university students, employed network analysis to identify core and bridge symptoms in three prevalent mental disorders. It uniquely integrated health-promoting behaviors into the network, exploring their associations with mental disorders, and identifying optimal nodes for health promotion, aligning with the WHO’s mental health promotion objective. The analysis revealed that central health-promoting behaviors predominantly impact the core and bridge symptoms of mental disorders, thereby preventing their development. Network comparison tests were conducted to further understand the differential effects or generalizability of these behaviors within the student population. Moreover, the HPLP-II questionnaire, chosen for its validated advantage in assessing self-maintenance health behaviors in daily life among the Chinese population, proved beneficial.
Despite these strengths, several limitations must be acknowledged. Firstly, the cross-sectional design of the study precludes causal inferences; longitudinal data are required to elucidate the complex mechanisms underlying the interactions between symptoms and health-promoting behaviors. Secondly, future research should incorporate risk factors to gain a comprehensive understanding, as risk factors may exacerbate mental disorders while protective factors might counteract these effects. Thirdly, our study was conducted in a single comprehensive university; despite efforts to mitigate bias, caution is warranted when generalizing the findings. Finally, it must be recognized that all sample information in this study was self-reported, which may affect the accuracy of the analysis.
In conclusion, university students are a high-risk group for mental disorders, with at least one in three reporting anxiety or depression, and one in every five to six experiencing sleep problems. Network analysis of the three common mental disorders revealed core symptoms: Low energy (PHQ4), Daytime dysfunction (PSQI7), and Trouble relaxing (GAD4). Bridge symptoms: Daytime dysfunction (PSQI7), Self-harm even suicide (PHQ9), Sad mood (PHQ2), Low energy (PHQ4), and Feeling afraid (GAD7). These symptoms are crucial in activating, maintaining, and interconnecting comorbid conditions. By incorporating health-promoting lifestyles (HPLs) into the network, we explored how protective HPLs are associated with these disorders and examined variations by gender, educational level, family sibling, and mental health status. Our findings highlighted the universal applicability of physical activity, spiritual growth, and stress management as the top health-promoting nodes for students. This study provided insights into common mental disorders and influential health lifestyles, which can aid educators and healthcare professionals in developing evidence-based, non-pharmacological intervention strategies to better promote mental health among university students.
The original contributions presented in the study are included in the article/Supplementary Material. Further inquiries can be directed to the corresponding author.
The studies involving humans were approved by The Ethics Committee of Zhengzhou University. The studies were conducted in accordance with the local legislation and institutional requirements. The participants provided their written informed consent to participate in this study.
ZZ: Writing – review & editing, Writing – original draft, Visualization, Validation, Supervision, Software, Resources, Methodology, Investigation, Formal analysis. CS: Funding acquisition, Data curation, Conceptualization, Writing – review & editing, Visualization, Supervision, Resources, Project administration. PZ: Writing – review & editing, Visualization, Supervision, Software, Methodology, Data curation. LW: Writing – review & editing, Visualization, Validation, Supervision, Project administration, Funding acquisition. QZ: Writing – review & editing, Visualization, Supervision, Project administration, Investigation, Funding acquisition. YG: Writing – review & editing, Visualization, Supervision, Resources, Investigation. LG: Writing – review & editing, Visualization, Supervision, Resources, Project administration. YL: Writing – review & editing, Visualization, Supervision, Investigation. PW: Writing – review & editing, Visualization, Supervision, Funding acquisition. BH: Writing – review & editing, Validation, Supervision, Data curation. ML: Writing – review & editing, Validation, Supervision. JD: Writing – review & editing, Validation, Supervision. YW: Writing – review & editing, Validation, Supervision. ZW: Writing – review & editing, Validation, Supervision. YQ: Writing – review & editing, Data curation, Supervision, Project administration.
The author(s) declare financial support was received for the research, authorship, and/or publication of this article. This study was supported by the Henan Province Science and Technology Research Project (No. 232102311023, 242102311135, 242102310234, 232102310052).
We thank all those who participated in the survey.
The authors declare that the research was conducted in the absence of any commercial or financial relationships that could be construed as a potential conflict of interest.
All claims expressed in this article are solely those of the authors and do not necessarily represent those of their affiliated organizations, or those of the publisher, the editors and the reviewers. Any product that may be evaluated in this article, or claim that may be made by its manufacturer, is not guaranteed or endorsed by the publisher.
The Supplementary Material for this article can be found online at: https://www.frontiersin.org/articles/10.3389/fpsyt.2024.1402680/full#supplementary-material
1. Ramon-Arbues E, Gea-Caballero V, Granada-Lopez JM, Juarez-Vela R, Pellicer-Garcia B, Anton-Solanas I. The prevalence of depression, anxiety and stress and their associated factors in college students. Int J Environ Res Public Health. (2020) 17. doi: 10.3390/ijerph17197001
2. Yang X, Fang Y, Chen H, Zhang T, Yin X, Man J, et al. Global, regional and national burden of anxiety disorders from 1990 to 2019: results from the global burden of disease study 2019. Epidemiol Psychiatr Sci. (2021) 30:e36. doi: 10.1017/S2045796021000275
3. Li W, Zhao Z, Chen D, Peng Y, Lu Z. Prevalence and associated factors of depression and anxiety symptoms among college students: a systematic review and meta-analysis. J Child Psychol Psychiatry. (2022) 63:1222–30. doi: 10.1111/jcpp.13606
4. Gauld C, Lopez R, Morin CM, Maquet J, Mcgonigal A, Geoffroy PA, et al. Why do sleep disorders belong to mental disorder classifications? A network analysis of the "sleep-wake disorders" section of the dsm-5. J Psychiatr Res. (2021) 142:153–59. doi: 10.1016/j.jpsychires.2021.07.050
5. Li L, Wang YY, Wang SB, Zhang L, Li L, Xu DD, et al. Prevalence of sleep disturbances in chinese university students: a comprehensive meta-analysis. J Sleep Res. (2018) 27:e12648. doi: 10.1111/jsr.12648
6. Wang Y, Guang Z, Zhang J, Han L, Zhang R, Chen Y, et al. Effect of sleep quality on anxiety and depression symptoms among college students in China's xizang region: the mediating effect of cognitive emotion regulation. Behav Sci (Basel). (2023) 13. doi: 10.3390/bs13100861
7. Oh CM, Kim HY, Na HK, Cho KH, Chu MK. The effect of anxiety and depression on sleep quality of individuals with high risk for insomnia: a population-based study. Front Neurol. (2019) 10:849. doi: 10.3389/fneur.2019.00849
8. Deng J, Zhou F, Hou W, Silver Z, Wong CY, Chang O, et al. The prevalence of depressive symptoms, anxiety symptoms and sleep disturbance in higher education students during the covid-19 pandemic: a systematic review and meta-analysis. Psychiatry Res. (2021) 301:113863. doi: 10.1016/j.psychres.2021.113863
9. Auerbach RP, Mortier P, Bruffaerts R, Alonso J, Benjet C, Cuijpers P, et al. Who world mental health surveys international college student project: prevalence and distribution of mental disorders. J Abnorm Psychol. (2018) 127:623–38. doi: 10.1037/abn0000362
10. Mason B. Mental health concerns for college students: self-harm, suicidal ideation, and substance use disorders. Prim Care. (2023) 50:47–55. doi: 10.1016/j.pop.2022.10.007
11. Borsboom D. A network theory of mental disorders. World Psychiatry. (2017) 16:5–13. doi: 10.1002/wps.20375
12. Contreras A, Nieto I, Valiente C, Espinosa R, Vazquez C. The study of psychopathology from the network analysis perspective: a systematic review. Psychother Psychosom. (2019) 88:71–83. doi: 10.1159/000497425
13. Lunansky G, Naberman J, van Borkulo CD, Chen C, Wang L, Borsboom D. Intervening on psychopathology networks: evaluating intervention targets through simulations. Methods. (2022) 204:29–37. doi: 10.1016/j.ymeth.2021.11.006
14. Zhang P, Wang L, Zhou Q, Dong X, Guo Y, Wang P, et al. A network analysis of anxiety and depression symptoms in Chinese disabled elderly. J Affect Disord. (2023) 333:535–42. doi: 10.1016/j.jad.2023.04.065
15. Wang N, Wang M, Xin X, Zhang T, Wu H, Huang X, et al. Exploring the relationship between anxiety, depression, and sleep disturbance among hiv patients in China from a network perspective. Front Psychiatry. (2021) 12:764246. doi: 10.3389/fpsyt.2021.764246
16. Chen C, Li F, Liu C, Li K, Yang Q, Ren L. The relations between mental well-being and burnout in medical staff during the covid-19 pandemic: a network analysis. Front Public Health. (2022) 10:919692. doi: 10.3389/fpubh.2022.919692
17. Wang H. A discourse on the balance of life: key factors in health and longevity for the elderly. J Beneficial Readings Drug Inf Med Advices. (2009) 5):40–2.
18. Hua Y, Wang B, Wallen GR, Shao P, Ni C, Hua Q. Health-promoting lifestyles and depression in urban elderly Chinese. PloS One. (2015) 10:e117998. doi: 10.1371/journal.pone.0117998
19. Zhu M, Zhao J, Zhu X, Cheng Q, Zhang S, Kong L. Effects of health-promoting lifestyle on late-onset depression in older adults: mediating effect of meaning in life and interleukin-6 (IL-6). Psychol Res Behav Manag. (2023) 16:5159–68. doi: 10.2147/PRBM.S441277
20. Hoying J, Melnyk BM, Hutson E, Tan A. Prevalence and correlates of depression, anxiety, stress, healthy beliefs, and lifestyle behaviors in first-year graduate health sciences students. Worldviews Evid Based Nurs. (2020) 17:49–59. doi: 10.1111/wvn.12415
21. Zhang S, Zhang N, Wang S, Hong J, Li F, Guo H, et al. Circadian rhythms and sleep quality among undergraduate students in China: the mediating role of health-promoting lifestyle behaviours. J Affect Disord. (2023) 333:225–32. doi: 10.1016/j.jad.2023.04.077
22. Kelly DL, Yang GS, Starkweather AR, Siangphoe U, Alexander-Delpech P, Lyon DE. Relationships among fatigue, anxiety, depression, and pain and health-promoting lifestyle behaviors in women with early-stage breast cancer. Cancer Nurs. (2020) 43:134–46. doi: 10.1097/NCC.0000000000000676
23. Cheng Z, Qiu-yu P, Wei P, Dong-mei X. Investigation and analysis on health lifestyle and behavior of undergraduates. J Xichang Coll (Natural Sci Edition). (2016) 30:112–16. doi: 10.16104/j.issn.1673-1891.2016.02.032
24. van der Lee ML, Schellekens M. Capturing the complexity of mental disorders in the medically ill: the network approach on behavioral medicine. Transl Behav Med. (2020) 10:812–16. doi: 10.1093/tbm/ibz019
25. Schellekens M, Wolvers M, Schroevers MJ, Bootsma TI, Cramer A, van der Lee ML. Exploring the interconnectedness of fatigue, depression, anxiety and potential risk and protective factors in cancer patients: a network approach. J Behav Med. (2020) 43:553–63. doi: 10.1007/s10865-019-00084-7
26. Hoorelbeke K, Van den Bergh N, Wichers M, Koster E. Between vulnerability and resilience: a network analysis of fluctuations in cognitive risk and protective factors following remission from depression. Behav Res Ther. (2019) 116:1–09. doi: 10.1016/j.brat.2019.01.007
27. Fonseca-Pedrero E, Al-Halabi S, Perez-Albeniz A, Debbane M. Risk and protective factors in adolescent suicidal behaviour: a network analysis. Int J Environ Res Public Health. (2022) 19. doi: 10.3390/ijerph19031784
28. van Borkulo CD, van Bork R, Boschloo L, Kossakowski JJ, Tio P, Schoevers RA, et al. Comparing network structures on three aspects: a permutation test. Psychol Methods. (2023) 28:1273–85. doi: 10.1037/met0000476
29. McTeague LM, Rosenberg BM, Lopez JW, Carreon DM, Huemer J, Jiang Y, et al. Identification of common neural circuit disruptions in emotional processing across psychiatric disorders. Am J Psychiatry. (2020) 177:411–21. doi: 10.1176/appi.ajp.2019.18111271
30. Sun D, Yang D, Li Y, Zhou J, Wang W, Wang Q, et al. Psychological impact of 2019 novel coronavirus (2019-nCoV) outbreak in health workers in China. Epidemiol Infect. (2020) 148:e96. doi: 10.1017/S0950268820001090
31. Epskamp S, Fried EI. A tutorial on regularized partial correlation networks. Psychol Methods. (2018) 23:617–34. doi: 10.1037/met0000167
32. Spitzer RL, Kroenke K, Williams JB, Lowe B. A brief measure for assessing generalized anxiety disorder: the gad-7. Arch Intern Med. (2006) 166:1092–97. doi: 10.1001/archinte.166.10.1092
33. Gong Y, Zhou H, Zhang Y, Zhu X, Wang X, Shen B, et al. Validation of the 7-item generalized anxiety disorder scale (gad-7) as a screening tool for anxiety among pregnant Chinese women. J Affect Disord. (2021) 282:98–103. doi: 10.1016/j.jad.2020.12.129
34. Kroenke K, Spitzer RL, Williams JB. The phq-9: validity of a brief depression severity measure. J Gen Intern Med. (2001) 16:606–13. doi: 10.1046/j.1525-1497.2001.016009606.x
35. Spitzer RL, Williams JB, Kroenke K, Hornyak R, McMurray J. Validity and utility of the prime-md patient health questionnaire in assessment of 3000 obstetric-gynecologic patients: the prime-md patient health questionnaire obstetrics-gynecology study. Am J Obstet Gynecol. (2000) 183:759–69. doi: 10.1067/mob.2000.106580
36. Wang W, Bian Q, Zhao Y, Li X, Wang W, Du J, et al. Reliability and validity of the chinese version of the patient health questionnaire (PHQ-9) in the general population. Gen Hosp Psychiatry. (2014) 36:539–44. doi: 10.1016/j.genhosppsych.2014.05.021
37. Luo J, Bei DL, Zheng C, Jin J, Yao C, Zhao J, et al. The comorbid network characteristics of anxiety and depressive symptoms among Chinese college freshmen. BMC Psychiatry. (2024) 24:297. doi: 10.1186/s12888-024-05733-z
38. Buysse DJ, Reynolds CR, Monk TH, Berman SR, Kupfer DJ. The Pittsburgh sleep quality index: a new instrument for psychiatric practice and research. Psychiatry Res. (1989) 28:193–213. doi: 10.1016/0165-1781(89)90047-4
39. Liu X, Tang M, Hu L, Wang A, Wu H, Zao G, et al. Study on reliability and validity of the Pittsburgh sleep quality index. Chin J Psychiatry. (1996) 29:103–07.
40. Walker SN, Sechrist KR, Pender NJ. The health-promoting lifestyle profile: development and psychometric characteristics. Nurs Res. (1987) 36:76–81. doi: 10.1097/00006199-198703000-00002
41. Cao W, Guo Y, Ping W, Zheng J. Development and psychometric tests of a Chinese version of the HPLP-II scales. Chin J Dis Control Prev. (2016) 20:286–89. doi: 10.16462/j.cnki.zhjbkz.2016.03.018
42. McNally RJ. Network analysis of psychopathology: controversies and challenges. Annu Rev Clin Psychol. (2021) 17:31–53. doi: 10.1146/annurev-clinpsy-081219-092850
43. Xu X, Xie T, Zhou N, Shi G, Wen J, Wang J, et al. Network analysis of pgd, ptsd and insomnia symptoms in chinese shidu parents with pgd. Eur J Psychotraumatol. (2022) 13:2057674. doi: 10.1080/20008198.2022.2057674
44. Epskamp S, Borsboom D, Fried EI. Estimating psychological networks and their accuracy: a tutorial paper. Behav Res Methods. (2018) 50:195–212. doi: 10.3758/s13428-017-0862-1
45. Friedman J, Hastie T, Tibshirani R. Sparse inverse covariance estimation with the graphical lasso. Biostatistics. (2008) 9:432–41. doi: 10.1093/biostatistics/kxm045
46. van Borkulo CD, Borsboom D, Epskamp S, Blanken TF, Boschloo L, Schoevers RA, et al. A new method for constructing networks from binary data. Sci Rep. (2014) 4:5918. doi: 10.1038/srep05918
47. Epskamp S, Cramer AOJ, Waldorp LJ, Schmittmann VD, Borsboom D. Qgraph : network visualizations of relationships in psychometric data. J Stat Softw. (2012) 48:1–18. doi: 10.18637/jss.v048.i04
48. Opsahl T, Agneessens F, Skvoretz J. Node centrality in weighted networks: generalizing degree and shortest paths. Soc Networks. (2010) 32:245–51. doi: 10.1016/j.socnet.2010.03.006
49. Bringmann LF, Elmer T, Epskamp S, Krause RW, Schoch D, Wichers M, et al. What do centrality measures measure in psychological networks? J Abnorm Psychol. (2019) 128:892–903. doi: 10.1037/abn0000446
50. Jones PJ, Ma R, McNally RJ. Bridge centrality: a network approach to understanding comorbidity. Multivariate Behav Res. (2021) 56:353–67. doi: 10.1080/00273171.2019.1614898
51. Haslbeck J, Waldorp LJ. How well do network models predict observations? On the importance of predictability in network models. Behav Res Methods. (2018) 50:853–61. doi: 10.3758/s13428-017-0910-x
52. Costenbader E, Valente TW. The stability of centrality measures when networks are sampled. Soc Networks. (2003) 25:283–307. doi: 10.1016/S0378-8733(03)00012-1
53. Marchetti I. Hopelessness: a network analysis. Cognit Ther Res. (2019) 43:611–19. doi: 10.1007/s10608-018-9981-y
54. Brundtland GH. Statement by Dr Gro Harlem Brundtland, director general who, to the fifth global conference on health promotion, Mexico City, 5 June 2000. Health Promot Int. (2001) 16:95–8. doi: 10.1093/heapro/16.1.95
55. Ren L, Wang Y, Wu L, Wei Z, Cui LB, Wei X, et al. Network structure of depression and anxiety symptoms in chinese female nursing students. BMC Psychiatry. (2021) 21:279. doi: 10.1186/s12888-021-03276-1
56. Peng P, Liang M, Wang Q, Lu L, Wu Q, Chen Q. Night shifts, insomnia, anxiety, and depression among chinese nurses during the covid-19 pandemic remission period: a network approach. Front Public Health. (2022) 10:1040298. doi: 10.3389/fpubh.2022.1040298
57. Zhao Y, Liang K, Qu D, He Y, Wei X, Chi X. The longitudinal features of depressive symptoms during the covid-19 pandemic among Chinese college students: a network perspective. J Youth Adolesc. (2023) 52:2031–44. doi: 10.1007/s10964-023-01802-w
58. Liu S, Xi HT, Zhu QQ, Ji M, Zhang H, Yang BX, et al. The prevalence of fatigue among Chinese nursing students in post-covid-19 era. Peerj. (2021) 9:e11154. doi: 10.7717/peerj.11154
59. Bard HA, O'Driscoll C, Miller CB, Henry AL, Cape J, Espie CA. Insomnia, depression, and anxiety symptoms interact and individually impact functioning: a network and relative importance analysis in the context of insomnia. Sleep Med. (2023) 101:505–14. doi: 10.1016/j.sleep.2022.12.005
60. Heitmann H, Andlauer TFM, Korn T, Muhlau M, Henningsen P, Hemmer B, et al. Fatigue, depression, and pain in multiple sclerosis: how neuroinflammation translates into dysfunctional reward processing and anhedonic symptoms. Multiple Sclerosis: Clin Lab Res. (2022) 28:1020–27. doi: 10.1177/1352458520972279
61. Garabiles MR, Lao CK, Xiong Y, Hall BJ. Exploring comorbidity between anxiety and depression among migrant Filipino domestic workers: a network approach. J Affect Disord. (2019) 250:85–93. doi: 10.1016/j.jad.2019.02.062
62. Cai H, Zhao YJ, Xing X, Tian T, Qian W, Liang S, et al. Network analysis of comorbid anxiety and insomnia among clinicians with depressive symptoms during the late stage of the covid-19 pandemic: a cross-sectional study. Nat Sci Sleep. (2022) 14:1351–62. doi: 10.2147/NSS.S367974
63. Bai W, Zhao YJ, Cai H, Sha S, Zhang Q, Lei SM, et al. Network analysis of depression, anxiety, insomnia and quality of life among macau residents during the covid-19 pandemic. J Affect Disord. (2022) 311:181–88. doi: 10.1016/j.jad.2022.05.061
64. Guo W, Zhao Y, Chen H, Liu J, Chen X, Tang H, et al. The bridge symptoms of childhood trauma, sleep disorder and depressive symptoms: a network analysis. Child Adolesc Psychiatry Ment Health. (2023) 17. doi: 10.1186/s13034-023-00635-6
65. Bai W, Zhao Y, An F, Zhang Q, Sha S, Cheung T, et al. Network analysis of insomnia in chinese mental health professionals during the covid-19 pandemic: a cross-sectional study. Nat Sci Sleep. (2021) 13:1921–30. doi: 10.2147/NSS.S326880
66. Zhang X, Gao F, Kang Z, Zhou H, Zhang J, Li J, et al. Perceived academic stress and depression: the mediation role of mobile phone addiction and sleep quality. Front Public Health. (2022) 10:760387. doi: 10.3389/fpubh.2022.760387
67. Liu Q, Luo D, Haase JE, Guo Q, Wang XQ, Liu S, et al. The experiences of health-care providers during the covid-19 crisis in China: a qualitative study. Lancet Glob Health. (2020) 8:e790–98. doi: 10.1016/S2214-109X(20)30204-7
68. Price M, Legrand AC, Brier ZMF, Hebert-Dufresne L. The symptoms at the center: examining the comorbidity of posttraumatic stress disorder, generalized anxiety disorder, and depression with network analysis. J Psychiatr Res. (2019) 109:52–8. doi: 10.1016/j.jpsychires.2018.11.016
69. Orosa-Duarte A, Mediavilla R, Munoz-Sanjose A, Palao A, Garde J, Lopez-Herrero V, et al. Mindfulness-based mobile app reduces anxiety and increases self-compassion in healthcare students: a randomised controlled trial. Med Teach. (2021) 43:686–93. doi: 10.1080/0142159X.2021.1887835
70. Gore FM, Bloem PJ, Patton GC, Ferguson J, Joseph V, Coffey C, et al. Global burden of disease in young people aged 10-24 years: a systematic analysis. Lancet. (2011) 377:2093–102. doi: 10.1016/S0140-6736(11)60512-6
71. Yang T, He Y, Wu L, Ren L, Lin J, Wang C, et al. The relationships between anxiety and suicidal ideation and between depression and suicidal ideation among chinese college students: a network analysis. Heliyon. (2023) 9:e20938. doi: 10.1016/j.heliyon.2023.e20938
72. Wang L, He CZ, Yu YM, Qiu XH, Yang XX, Qiao ZX, et al. Associations between impulsivity, aggression, and suicide in chinese college students. BMC Public Health. (2014) 14:551. doi: 10.1186/1471-2458-14-551
73. Kessler RC, Berglund P, Demler O, Jin R, Merikangas KR, Walters EE. Lifetime prevalence and age-of-onset distributions of DSM-IV disorders in the national comorbidity survey replication. Arch Gen Psychiatry. (2005) 62:593–602. doi: 10.1001/archpsyc.62.6.593
74. Moskow DM, Lipson SK, Tompson MC. Anxiety and suicidality in the college student population. J Am Coll Health. (2024) 72:881–88. doi: 10.1080/07448481.2022.2060042
75. Wang Y, Li Z, Cao X. Investigating the network structure and causal relationships among bridge symptoms of comorbid depression and anxiety: a Bayesian network analysis. J Clin Psychol. (2024) 80:1271–85. doi: 10.1002/jclp.23663
76. Wasil AR, Gillespie S, Park SJ, Venturo-Conerly KE, Osborn TL, DeRubeis RJ, et al. Which symptoms of depression and anxiety are most strongly associated with happiness? A network analysis of Indian and Kenyan adolescents. J Affect Disord. (2021) 295:811–21. doi: 10.1016/j.jad.2021.08.087
77. Bernstein EE, McNally RJ. Acute aerobic exercise helps overcome emotion regulation deficits. Cognit Emot. (2017) 31:834–43. doi: 10.1080/02699931.2016.1168284
78. Zhang Y, Liu J, Zhang Y, Ke L, Liu R. Interactive compensation effects of physical activity and sleep on mental health: a longitudinal panel study among Chinese college students during the covid-19 pandemic. Int J Environ Res Public Health. (2022) 19. doi: 10.3390/ijerph191912323
79. Li Y, Guo K. Research on the relationship between physical activity, sleep quality, psychological resilience, and social adaptation among Chinese college students: a cross-sectional study. Front Psychol. (2023) 14:1104897. doi: 10.3389/fpsyg.2023.1104897
80. Sejbuk M, Mironczuk-Chodakowska I, Witkowska AM. Sleep quality: a narrative review on nutrition, stimulants, and physical activity as important factors. Nutrients. (2022) 14. doi: 10.3390/nu14091912
81. Grasdalsmoen M, Eriksen HR, Lonning KJ, Sivertsen B. Physical exercise, mental health problems, and suicide attempts in university students. BMC Psychiatry. (2020) 20:175. doi: 10.1186/s12888-020-02583-3
82. Celik DO, Haney MO. The relationship between depression, healthy lifestyle behaviors and internet addiction: a cross-sectional study of the athlete university students in Turkey. Front Psychiatry. (2023) 14:1222931. doi: 10.3389/fpsyt.2023.1222931
83. Leung CH, Pong HK. Cross-sectional study of the relationship between the spiritual wellbeing and psychological health among university students. PloS One. (2021) 16:e249702. doi: 10.1371/journal.pone.0249702
84. Rezaie L, Norouzi E, Bratty AJ, Khazaie H. Better sleep quality and higher physical activity levels predict lower emotion dysregulation among persons with major depression disorder. BMC Psychol. (2023) 11:171. doi: 10.1186/s40359-023-01213-3
85. Zeng W, Zeng Y, Xu Y, Huang D, Shao J, Wu J, et al. The influence of post-traumatic growth on college students' creativity during the covid-19 pandemic: the mediating role of general self-efficacy and the moderating role of deliberate rumination. Front Psychol. (2021) 12:665973. doi: 10.3389/fpsyg.2021.665973
86. Sahebi Z, Barkhordari-Sharifabad M. Spiritual care competency and its relationship with clinical self-efficacy in nursing students. BMC Med Educ. (2023) 23:937. doi: 10.1186/s12909-023-04937-3
87. Pawlaczyk M, Siembida J, Balaj K, Rajewska-Rager A. The assessment of stress level, anxiety, depressive symptoms, and defense mechanisms among polish and English medical students. Ann Gen Psychiatry. (2020) 19:29. doi: 10.1186/s12991-020-00274-7
88. Hudson N, Law C, Culley L, Mitchell H, Denny E, Norton W, et al. Men, chronic illness and healthwork: accounts from male partners of women with endometriosis. Sociol Health Illn. (2020) 42:1532–47. doi: 10.1111/1467-9566.13144
89. Masina T, Madzar T, Musil V, Milosevic M. Differences in health-promoting lifestyle profile among Croatian medical students according to gender and year of study. Acta Clin Croat. (2017) 56:84–91. doi: 10.20471/acc.2017.56.01.13
90. Evans TM, Bira L, Gastelum JB, Weiss LT, Vanderford NL. Evidence for a mental health crisis in graduate education. Nat Biotechnol. (2018) 36:282–84. doi: 10.1038/nbt.4089
91. Gao W, Ping S, Liu X. Gender differences in depression, anxiety, and stress among college students: a longitudinal study from China. J Affect Disord. (2020) 263:292–300. doi: 10.1016/j.jad.2019.11.121
Keywords: anxiety, depression, sleep problems, network analysis, health-promoting lifestyles, university students
Citation: Sun C, Zhu Z, Zhang P, Wang L, Zhang Q, Guo Y, Guo L, Li Y, Wang P, Hu B, Liu M, Duan J, Wang Y, Wang Z and Qin Y (2024) Exploring the interconnections of anxiety, depression, sleep problems and health-promoting lifestyles among Chinese university students: a comprehensive network approach. Front. Psychiatry 15:1402680. doi: 10.3389/fpsyt.2024.1402680
Received: 18 March 2024; Accepted: 26 June 2024;
Published: 15 July 2024.
Edited by:
Steffen Schulz, Charité University Medicine Berlin, GermanyReviewed by:
Pu Peng, Central South University, ChinaCopyright © 2024 Sun, Zhu, Zhang, Wang, Zhang, Guo, Guo, Li, Wang, Hu, Liu, Duan, Wang, Wang and Qin. This is an open-access article distributed under the terms of the Creative Commons Attribution License (CC BY). The use, distribution or reproduction in other forums is permitted, provided the original author(s) and the copyright owner(s) are credited and that the original publication in this journal is cited, in accordance with accepted academic practice. No use, distribution or reproduction is permitted which does not comply with these terms.
*Correspondence: Ying Qin, cWlueUB6enUuZWR1LmNu
Disclaimer: All claims expressed in this article are solely those of the authors and do not necessarily represent those of their affiliated organizations, or those of the publisher, the editors and the reviewers. Any product that may be evaluated in this article or claim that may be made by its manufacturer is not guaranteed or endorsed by the publisher.
Research integrity at Frontiers
Learn more about the work of our research integrity team to safeguard the quality of each article we publish.