- 1Department of Bipolar Disorder, Shenzhen Kangning Hospital, Shenzhen Mental Health Center, Shenzhen, Guangdong, China
- 2Department of Cardiology, The Eighth Affiliated Hospital of Sun Yat-Sen University, Shenzhen, Guangzhou, China
- 3Department of Geriatrics, Shenzhen Kangning Hospital, Shenzhen Mental Health Center, Shenzhen, Guangdong, China
- 4Department of Drug Dependence, Shenzhen Kangning Hospital, Shenzhen Mental Health Center, Shenzhen, Guangdong, China
Introduction: Increasing evidence has indicated a connection between bipolar disorder (BD) and arteriosclerosis (AS), yet the specific molecular mechanisms remain unclear. This study aims to investigate the hub genes and molecular pathways for BD with AS.
Methods: BD-related dataset GSE12649 were downloaded from the Gene Expression Omnibus database and differentially expressed genes (DEGs) and key module genes derived from Limma and weighted gene co-expression network analyses (WGCNA) were identified. AS-related genes were sourced from the DisGeNET database, and the overlapping genes between DEGs and AS-related genes were characterized as differentially expressed arteriosclerosis-related genes (DE-ASRGs). The functional enrichment analysis, protein-protein interaction (PPI) network and three machine learning algorithms were performed to explore the hub genes, which were validated with two external validation sets. Additionally, immune infiltration was performed in BD.
Results: Overall, 67 DE-ASRGs were found to be overlapping between the DEGs and AS-related genes. Functional enrichment analysis highlighted the cancer pathways between BD and AS. We identified seven candidate hub genes (CTSD, IRF3, NPEPPS, ST6GAL1, HIF1A, SOX9 and CX3CR1). Eventually, two hub genes (CX3CR1 and ST6GAL1) were identified as BD and AS co-biomarkers by using machine learning algorithms. Immune infiltration had revealed the disorder of immunocytes.
Discussion: This study identified the hub genes CX3CR1 and ST6GAL1 in BD and AS, providing new insights for further research on the bioinformatic mechanisms of BD with AS and contributing to the diagnosis and prevention of AS in psychiatric clinical practice.
1 Introduction
Bipolar disorder (BD) is marked by alternating episodes of depression as well as either mania or hypomania, impacting an estimated 40 million individuals worldwide (1). Bipolar disorder is the 17th leading cause of global burden of diseases (2) and often lead to functional impairment and reduced quality of life (3). Psychiatric and nonpsychiatric medical comorbidities are common in patients with BD and might also contribute to increased mortality, particularly from cardiovascular diseases (CVDs) (4). Individuals diagnosed with bipolar disorder exhibit a notably elevated risk of mortality from CVDs compared to the general population, with a standardized mortality ratio of 1.73 and experience cardiovascular mortality occurring an average of 17 years earlier (1, 5, 6). This elevated risk persists even after considering the high prevalence of cardiovascular risk factors present in individuals with bipolar disorder (7). Evidence from a large epidemiological study suggests that the elevated occurrence and premature onset of CVDs in BD surpasses what can be accounted for by traditional cardiovascular risk factors (8).
Arteriosclerosis (AS) is primarily an aging-related process characterized by increased stiffness in elastic arteries, including the aorta (9). The pathological characteristics of AS include elastin fracture, an increase in collagen fibers, and calcium deposition. AS develops as a result of increased production or progressively greater engagement of stiffer load-bearing elements in the arterial wall, such as collagen (10). Arterial stiffness of AS is a significant risk factor for CVDs and a powerful predictor of CVDs morbidity and mortality (11).
Several studies have investigated the phenomenon between AS and BD. In a study investigating the relationship between arterial stiffness and BD, patients were found to have higher arterial stiffness compared to healthy controls regarding to the elastic modulus of the carotid artery (12). Furthermore, research suggested that patients with BD have greater carotid intima-media thickness before middle age and were at increasing risk of atherosclerosis (13). BD was closely associated with and independently contribute to increasing atherogenic potential (14). Long-term depressive and manic symptom burden, especially the persistence and duration of mood syndromes, has been independently linked to poor endothelial function and impaired vascular function, which lead to subsequent cardiovascular morbidity and mortality (15–17). The chronicity of mood symptoms contributes to vasculopathy in a dose-dependent fashion, and patients with more manic/hypomanic symptoms had poorer endothelial function (17, 18). Besides, lipid abnormalities that contribute to an increased atherogenic potential are implicated in the pathophysiology of BD (19). Underlying pathophysiological factors such as immune-inflammatory abnormalities, hypothalamic-pituitary-adrenal axis and sympathomedullary hyperactivity, increased platelet reactivity, reduced heart rate variability, oxidative stress, and endothelial dysfunction may also contribute to the heightened risk of CVDs (20–22).
Most studies focusing on the relationship between BD and AS are based on clinical investigation and examination, and scarce data are available to explore associated pathological mechanisms and genetic alterations. With the advancement of multi-omics technologies, researchers are now able to uncover new insights into human diseases by identifying biomarkers, pathways, and other approaches, thereby offering novel strategies for disease diagnosis and treatment (23). Genome-wide association studies (GWAS) have identified several genetic variants between BD and CVDs (24, 25). The risk of myocardial infarction has been linked to a genetic variant in the ITIH3–ITIH4 genes, which have also been implicated in the risk of BD (Consortium, 2011). A phenome-wide association study (PheWAS) exploring BD and susceptibility genetic variants across various medical conditions indicated that the genome-wide single nucleotide polymorphism (SNP) rs4765913 in the CACNA1C gene may be linked to an increased risk of “cardiovascular dysgenesis” (25). An existing study of genome wide and candidate gene studies related to cardiometabolic diseases and mood disorders revealed 24 potential pleiotropic genes that are likely to be shared between mood disorders and cardiometabolic diseases risk (26). Moreover, data mining and machine learning techniques have been employed in the study of complex diseases to identify potential biomarkers (27). However, there are limited biologically relevant diagnostic markers available on AS in BD. Exploring new markers and methods to assess AS risk in BD patients could lead to more accurate diagnosis and improved treatment of BD. Investigating the pathogenesis and associations with AS is of considerable interest in the field of psychiatry and this progress requires having better understanding of molecular psychopathology and specific biomarkers (28). Therefore, the present study was conducted to predict the bidirectional hub genes and related pathways between BD and AS using bioinformatics and machine learning algorithms.
2 Methods
2.1 Data collection and preparation
Gene datasets related to bipolar disorder were downloaded from the Gene Expression Omnibus (GEO) database (https://www.ncbi.nlm.nih.gov/geoprofiles/). The inclusion criteria are set as: expression profiles come from the same sample source and should contain enough sample size that at least fifty samples to ensure accuracy. In addition, the test specimens included should be from humans. Finally, two microarray datasets [GSE12649 and GSE5392] derived from prefrontal cortex were downloaded from GEO (Affymetrix GPL96 platform, Affymetrix Human Genome U133A Array). The GSE12649 comprised 33 bipolar disorder samples and 34 control groups, while the GSE5392 contained 30 bipolar disorder samples and 52 control groups. GSE12649 was selected as the training group and GSE5392 as the test group. The expression matrix file of GSE12649 was normalized through the Limma package (29).
Since no microarray data sets detecting gene expression in brain especially in prefrontal cortex among arteriosclerosis, DisGeNET (https://www.disgenet.org/home/) was used to retrieve 2006 arteriosclerosis-related genes (ASRGs) (Supplementary Table S1). The DisGeNET database is a comprehensive gene-associated information platform that integrates experimentally validated data with information from authoritative repositories and scientific literature to present genes and variants linked to human diseases (30). Given that the GEO database lacks datasets directly related to AS, and considering that atherosclerosis is a consequence of arteriosclerosis (10), we have chosen the atherosclerosis dataset GSE100927 as our test group. The whole process flow is shown in Figure 1 and the complete information dataset is given in Table 1.
2.2 Differential gene expression analysis
After preparing the data, differential expression analysis was performed using the limma package in R (version 4.3.1) on the GSE12649 dataset. Differentially expressed genes (DEGs) were identified which were differentially expressed between the BD and control groups. The DEG threshold was set as P value <0.05 and |log2FC (fold change) |>0.2. Next, the difference analysis results were presented using the heatmap and volcano plot. In both plots, blue indicated low expression, and red indicated high. Then, the online Venn diagram tool (http://bioinformatics.psb.ugent.be/webtools/Venn/) was used to obtain the differentially expressed arteriosclerosis-related genes (DE-ASRGs) between DEGs and ASRG.
2.3 Functional enrichment analysis of DE-ASRGs
To further understand the function of the DE-ASRGs, we performed Gene Ontology (GO) enrichment analysis (31) and Kyoto Encyclopedia of Genes and Genomes (KEGG) pathway enrichment analysis (32) using the DAVID (https://david.ncifcrf.gov/). GO is a structured way to represent biological functions in terms of core entities and annotate protein biomarkers in the biological process (BP), cellular composition (CC) and molecular function (MF) levels. KEGG enables the correlation of gene catalogues to system functions at the cellular, species and ecosystem levels, facilitating researchers to understand the signaling pathways in which genes are involved. Based on the analysis, we selected the enrichment analysis results with a count greater than 5 and arranged them in descending order of count to identify the top 10.
2.4 Construction of protein–protein interaction network and identification of candidate hub genes
The DE-ASRGs were analyzed by a search tool for the retrieval of interacting genes/proteins (STRING) (https://www.string-db.org/) to predict the PPI network and to determine the possible relationships between them (confidence level 0.4). The STRING database and Cytoscape software (v3.10.1) completed constructing the PPI network for DE-ASRGs. Gene modules were analyzed and network characteristics of genes were ranked by protein score via the MCODE and CytoHubba plugin, respectively. The CytoHubba plugin was used to score each node gene by 9 randomly selected algorithms, including MNC (Maximum Neighbourhood Component), Degree, MCC (Maximal Clique Centrality), EPC (Edge Percolated Component), Closeness, BottleNeck, Betweenness, Radiality, and Stress. The top 10 genes from each algorithm were used to screen candidate hub genes through the “UpSetR” package.
2.5 Screening of target genes by weighted gene co-expression network analysis
The WGCNA was utilized for constructing unsigned co-expression networks for the identification of co-expression modules across samples using the “WGCNA” R package (33). WGCNA is a highly efficient and accurate method for analyzing microarray data, and using this approach can help identify genes associated with diseases. Initially, normalized mRNA expression data were used to perform WGCNA to identify gene co-expression and the correlation between gene modules and clinical characteristics (BD compared to control groups). Samples were examined for missing values and then clustered using the average linkage hierarchical method. Afterward, the optimal values of the weighted parameters of the adjacent functions were obtained using the pickSoftThreshold function and were used as soft thresholds for subsequent network construction. Moreover, a topological overlap matrix (TOM) was devised on the basis of an adjacency matrix, and a dynamic tree-cutting algorithm was used for detecting gene modules with a minimum gene group size of 30 and power = 6. The correlation of each module with the BD was calculated, and the module with P<0.05 was defined as the key module. Finally, we took the intersection between DE-ASRGs and WGCNA-derived key module genes to obtain potential hub gene of AS and BD.
2.6 Machine learning algorithms
To further identify hub genes from the potential candidates, machine learning algorithms were employed. To ensure the repeatability of these algorithms, we set the seed at 2023. The Least Absolute Shrinkage and Selection Operator (LASSO) algorithm was executed using the glmnet package (34), with a tenfold cross-validation was performed to adjust the optimal penalty parameter. The response type was set to binomial, nlambda was set to 100, and alpha was fixed at 1. Moreover, we chose the best lambda value by “lambda.min”. Subsequently, the Random Forest (RF) algorithm (35) was implemented using the randomForest package. We explored the optimal number of random forest trees using cross-validation errors and settled on 500 trees for analysis. The significance of genes in the RF model was evaluated based on mean decrease accuracy and mean decrease Gini. The intersection genes from the top 3 mean decrease accuracy and top 3 mean decrease Gini were identified. Additionally, the support vector machine recursive feature elimination (SVM-RFE) method (36), acting as a vigilant machine learning approach, was employed to select the most relevant variables by eliminating SVM-produced eigenvectors. For the categorization analyses of the screened markers in the BD, the caret package was utilized to conduct the SVM-RFE. The results of SVM-RFE were visualized, and through ten-fold cross-validation, the blue point represented the maximum classification accuracy, highlighting the corresponding gene sets as the most effective diagnostic markers. Only the names of valuable genes used in each machine learning model were included, irrespective of the specific learning model. The hub genes were determined as the intersection genes of valuable genes obtained from the three machine learning algorithms.
SVMs have been shown to exhibit robust classification performance and high accuracy when utilizing feature selection genes in the analysis of microarray expression profiles (37). In this study, SVM was used to evaluate the predictive capability of hub genes as feature genes as follows: 1) the BD datasets including GSE12649 and GSE5392 were separately divided into training (70%) and test (30%) sets; 2) SVM modeling was conducted using gene expression data of hub genes from the training set; 3) optimal SVM hyperparameters were determined through ten-fold cross-validation; 4) model accuracy was assessed using the test set.
2.7 Verification of hub genes
To validate the expression of two hub genes in BD and AS, the t-test was employed to analyze their expression levels. Initially, the expression levels of these hub genes were measured in the dataset GSE12649. Subsequently, two hub genes were validated in the independent datasets GSE5392 and GSE100927. The expression levels of the hub genes were visually represented in boxplots generated using the “ggplot2” package in R. Furthermore, the predictive and discriminatory abilities of the hub genes were evaluated through receiver operating characteristic (ROC) analysis using the “pROC” package, with the area under the curve (AUC) values determined.
2.8 Single gene GSEA analysis
Following the identification of hub genes, single-gene gene set enrichment analysis (GSEA) was conducted to uncover their potential functions, utilizing the “clusterProfiler” package. This analysis was carried out using the human reference genome. Subsequently, enrichplot was utilized to visualize the top 5 activating and inhibiting pathways for each gene in the two disease groups.
2.9 Correlation analysis between infiltrating immune cells and hub genes
For immune infiltration analysis, single-sample gene set enrichment analysis (ssGSEA) was carried out using the “GSVA” package to evaluate the relative infiltration levels of 28 immune cell types in each sample of BD. A comparison of immune cell content between the BD and control groups was conducted using the Wilcoxon test. Furthermore, Spearman correlation analysis between infiltrating immune cells and hub genes was performed using the “corrplot” package in R. The resulting correlations between hub genes and immune cells were visualized using lollipop plots.
2.10 Statistical analysis
R software version 4.2.1 was used to perform statistical analyses. Using the Student’s t-test, continuous variables were compared between two groups. A p-value less than 0.05 was statistically significant.
3 Results
3.1 Screening of differentially expressed genes in BD and identification of arteriosclerosis-related DEGs
After standardizing the microarray results, the differentially expressed genes (DEGs) were screened by “Limma” package (p < 0.05 and |log FC| > 0.2). The GSE12649 dataset contained 436 DEGs, including 201 upregulated genes and 235 downregulated genes (Figures 2A, B). Then, we intersected the resulting data with 2006 ASRGs and a total of 67 differentially expressed arteriosclerosis-related genes (DE-ASRGs) were identified (Figure 2C).
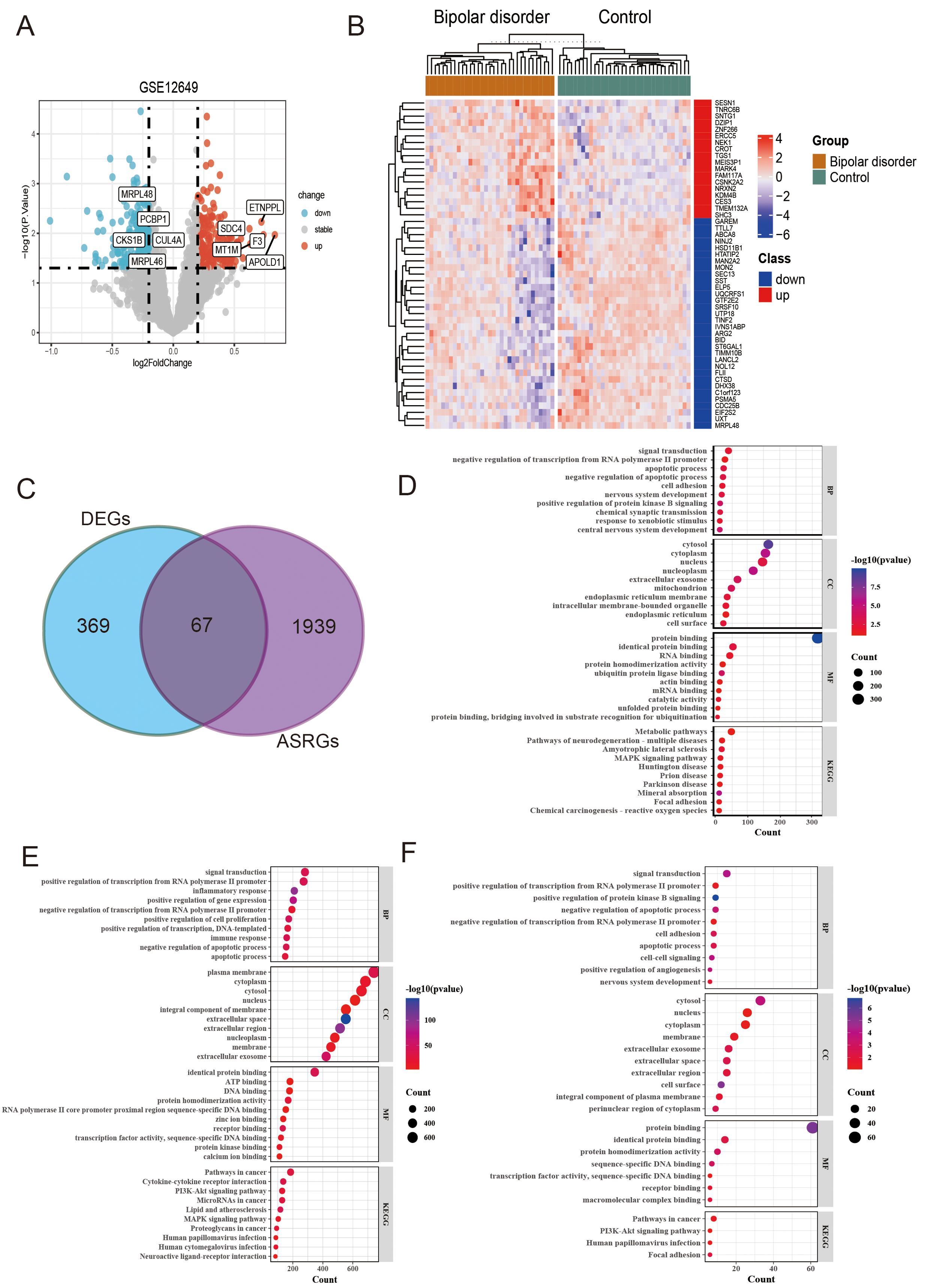
Figure 2. Identification and analysis of differentially expressed genes (DEGs) and differentially expressed arteriosclerosis-related genes (DE-ASRGs) in bipolar disorder (BD). (A) Volcanic map of the DEGs. (B) The expression patterns of top 50 DEGs shown by heatmap. (C) Venn diagram of DE-ASRGs. (D-F) The bubble plot of GO enrichment and KEGG pathway analysis results for DEGs (D), ASRG (E), and DE-ASRGs (F).
3.2 Analysis of the functional characteristics of DE-ASRGs
In order to analyze the biological functions and pathways, perform GO and KEGG pathway enrichment analysis on the DEGs, ASRG, and DE-ASRG genomes using DAVID (Figures 2D-F). Upon comparing the enrichment results of the three genomes, we observed that DE-ASRGs were primarily focused on signal transduction in biological processes. Additionally, negative regulation of apoptotic processes, negative regulation of translation from RNA polymerase II (pol II) promoter, and apoptotic processes were identified as common biological processes across the three genomes. The GO-CC pathways were principally associated with the cytosol, nucleus, and cytoplasm. The GO-MF analysis revealed enrichment in protein binding, identical protein binding and protein homodimerization activity. Additionally, KEGG analysis showed that these DE-ASRGs were enriched in pathways in cancer, PI3K-Akt signaling pathway and human papillomavirus infection (HPV).
3.3 Construction of PPI network of DE-ASRGs and potential hub gene screening
To explore the protein interactions among DE-ASRGs, we performed a PPI network containing 67 nodes and 82 edges with medium confidence (score > 0.4) using the STRING database and visualized the network with Cytoscape software, as shown in Figures 3A, B. Subsequently, the MCODE plugin identified a cluster comprising 5 nodes (CSF1R, HIF1A, TIMP3, ITGA7, SPP1) as illustrated in Figure 3C. The cytoHubba plugin was used to score each node gene by 9 randomly selected algorithms and the top 10 hub genes from each algorithm were identified. Five common genes (CSF1R, SPP1, HIF1A, SOX9, and CX3CR1) were selected from the 9 algorithms, as shown in Figure 3D, using the “UpSetR” package. Further screening of common genes through regression analysis resulted in the identification of three candidate hub genes (HIF1A, SOX9 and CX3CR1) as depicted in Figure 3E.
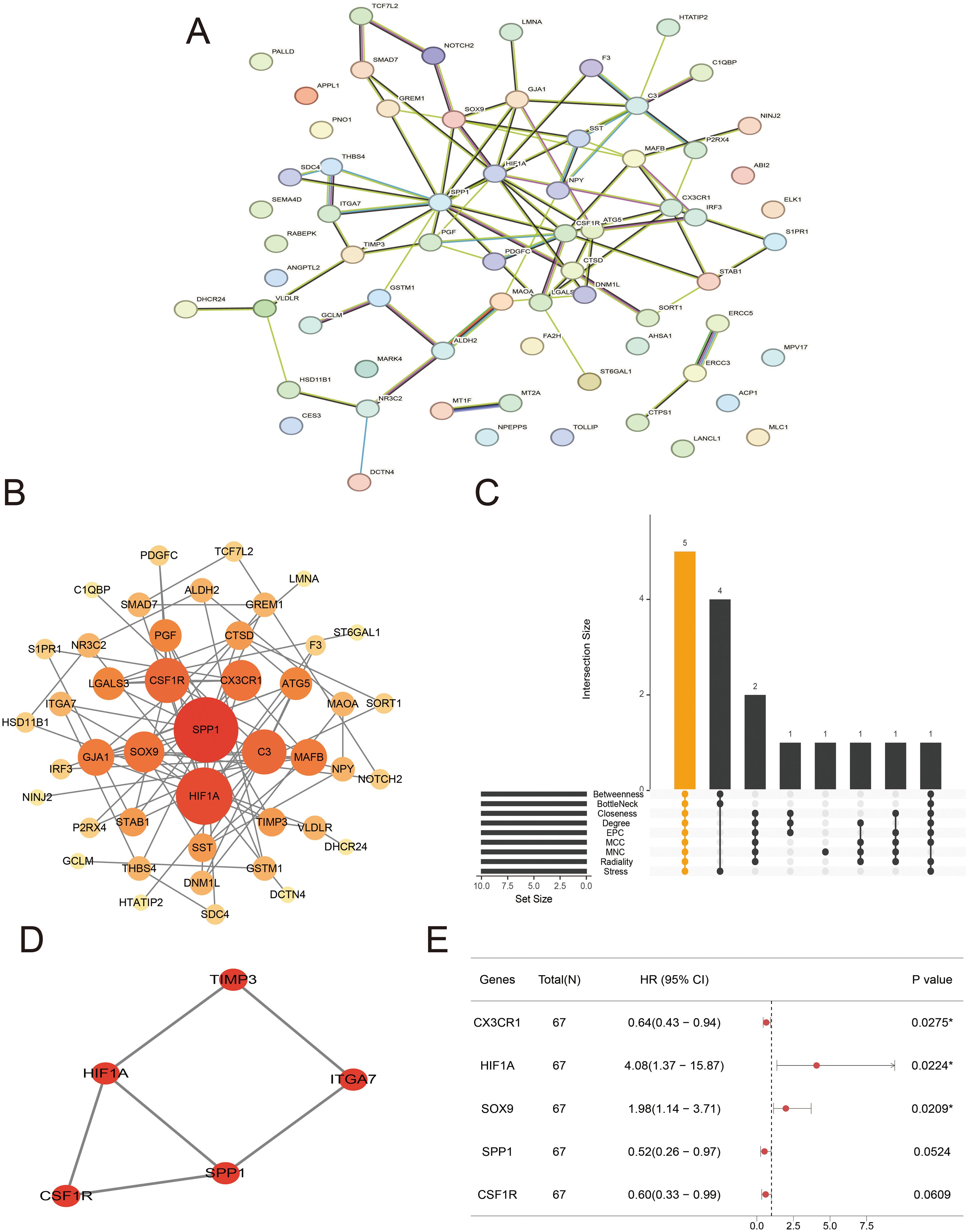
Figure 3. Protein–protein interaction (PPI) network and candidate hub genes. (A, B) Interaction network of differentially expressed arteriosclerosis-related genes (DE-ASRGs) by STRING (A) and Cytoscape (B). (C) The upset plot displaying the common genes among 9 algorithms. (D) Cluster identified by MCODE plugin. (E) Regression analysis of common genes.
3.4 Construction of co-expression networks
In this study, we conducted WGCNA to identify gene modules associated with BD in the GSE12649 dataset. Initially, the dataset underwent outlier screening, followed by clustering of the remaining samples as depicted in Figure 4A. To establish scale-free networks, a soft-threshold power of β = 6 (yielding a scale-free R2 of 0.85) was selected for BD, as shown in Figure 4B. Using a minimum module size of 30, we identified 12 modules, each represented by a unique color (Figure 4C). Heat maps illustrating module-trait relationships based on Pearson correlation coefficients were generated to evaluate the connection between each module and BD (Figure 4D). Among the 11 modules analyzed, the pink module exhibited the strongest correlation with BD (correlation coefficient = -0.29, P = 0.02), encompassing a total of 186 genes. Subsequently, the 186 genes within the pink module were intersected with the DE-ASRGs, resulting in the identification of 4 candidate hub genes (CTSD, IRF3, NPEPPS and ST6GAL1) (Figure 4E). These candidate hub genes were then integrated with those identified through Cytoscape analyses, ultimately yielding a total of 7 candidate hub genes (CTSD, IRF3, NPEPPS, ST6GAL1, HIF1A, SOX9 and CX3CR1).
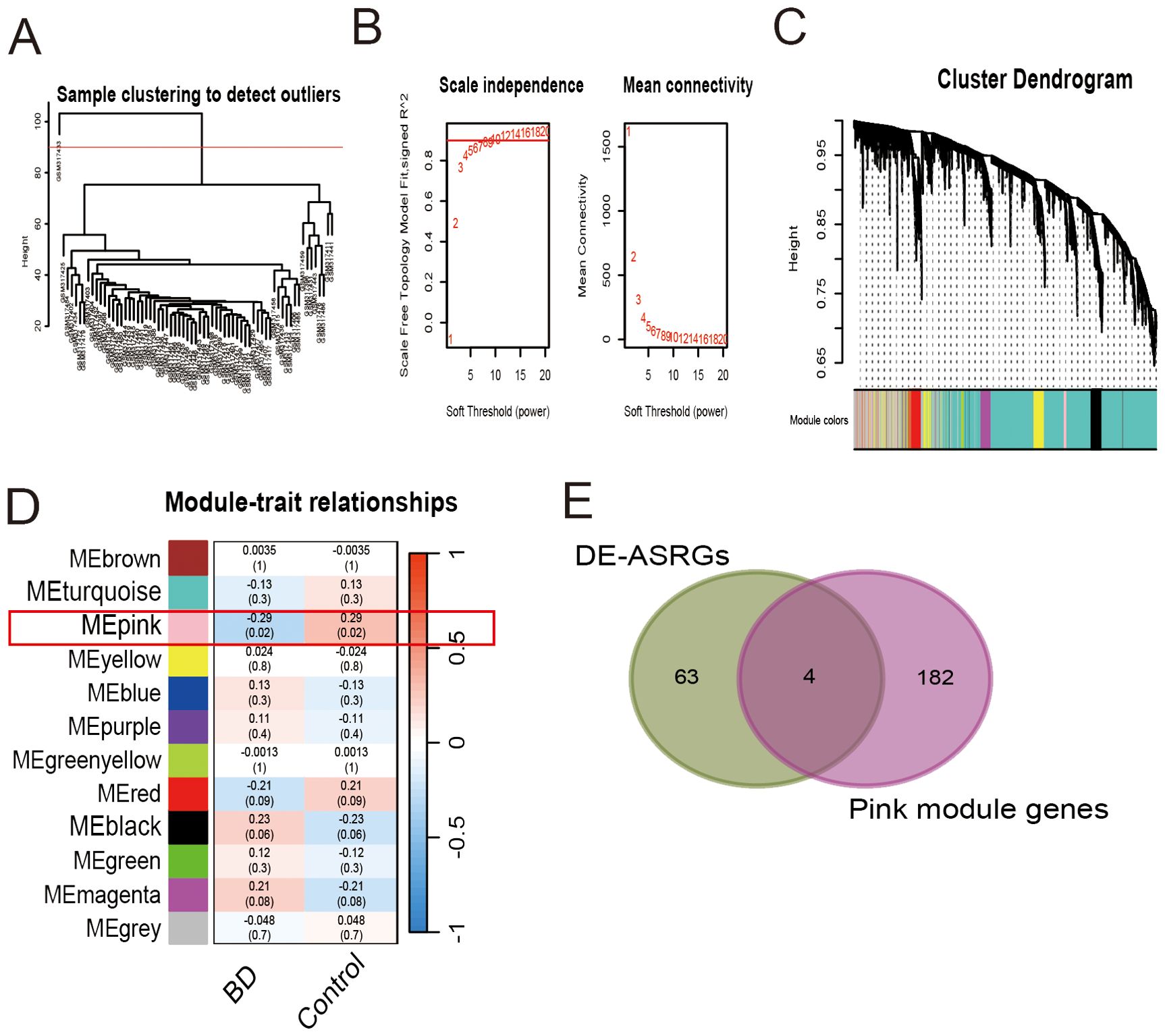
Figure 4. Weighted gene co-expression network analysis for gene set GSE12649. (A) Sample clustering to detect outliers. (B) Scale independence and mean connectivity. (C) Cluster dendrogram. (D) Module-trait relationships. (E) Venn diagram of 4 candidate hub genes.
3.5 Screening hub genes through machine learning
Lasso regression method, random forest and SVM-RFE method were used to further screen the expression matrix of the 7 candidate hub genes. The LASSO regression model was designed based on BD as well as control samples. By analyzing the Lasso coefficient profiles and selecting the optimal tuning parameter, λ was determined to be 0.0168688 (Figure 5A). Subsequently, five candidate genes were identified through the Lasso analysis. Following this, the seven candidate hub genes were fed into the RF classifier, and the top three genes were chosen based on the mean decrease accuracy and mean decrease Gini scale. Three genes were selected as candidate genes from the RF results (Figure 5B). Furthermore, SVM-RFE analysis was performed, revealing that the model incorporating three genes exhibited the best Mean Absolute Error (MAE) and Root Mean Square Error (RMSE) (Figure 5C). By comparing the overlapping genes obtained from three machine learning methods, two hub genes were selected: CX3CR1 and ST6GAL1 (Figure 5D).
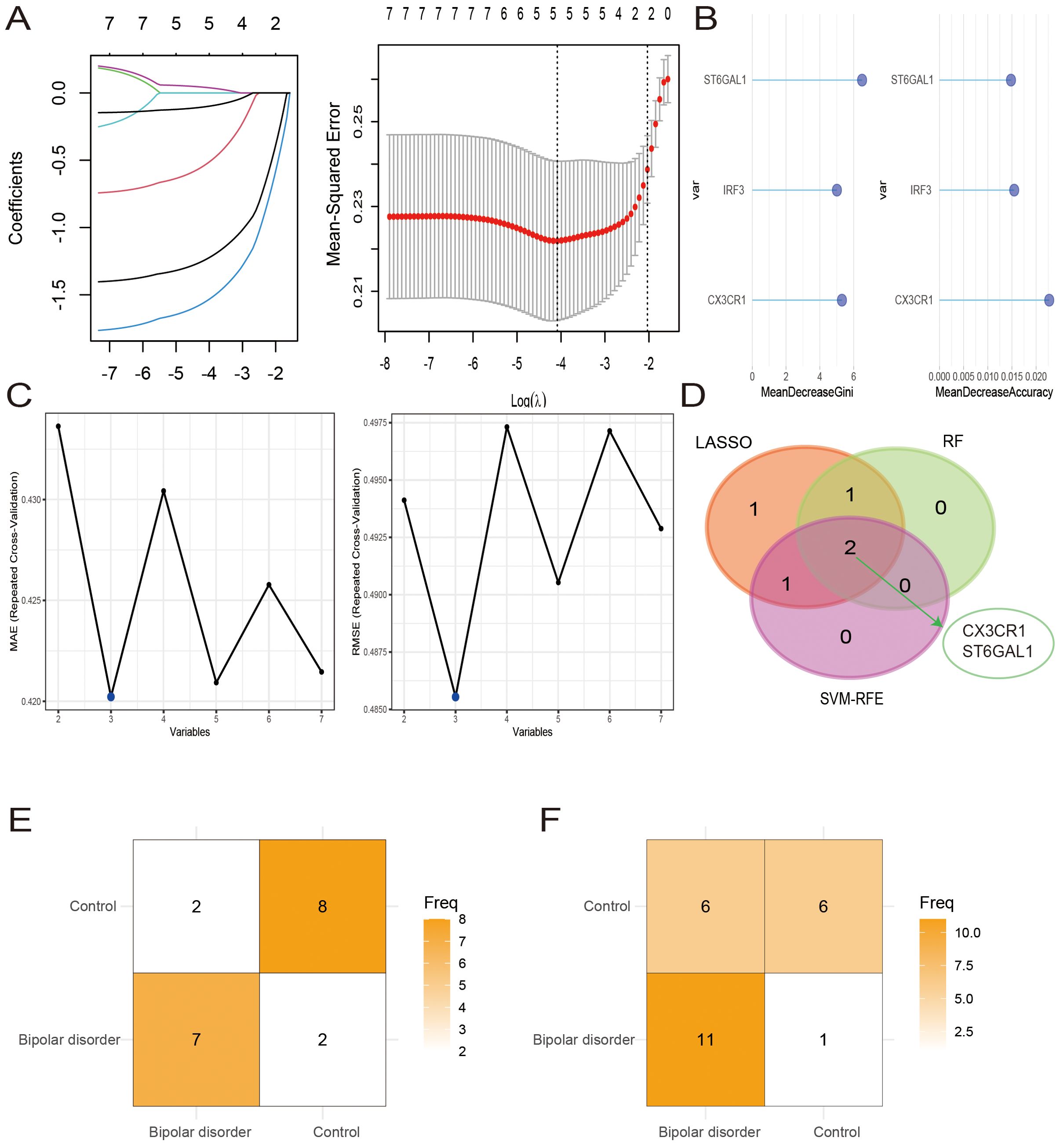
Figure 5. Hub cross-talk gene screening. (A-C) Results of Least Absolute Shrinkage and Selection Operator (LASSO) (A), Random Forest (RF) (B), and support vector machine recursive feature elimination (SVM-RFE) (C) analysis. (D) Venn diagram of 2 hub genes. (E, F) The confusion matrices for the model’s validation on the GSE12649 (E) and GSE5392 (F) datasets.
We developed SVM models using the BD datasets GSE12649 and GSE5392 to assess the predictive capability of the two hub genes, CX3CR1 and ST6GAL1. The model’s performance on the GSE12649 test set showed an accuracy of 78.90% and a Kappa of 0.58. For the GSE5392 test set, the SVM model achieved an accuracy of 70.83% and a Kappa of 0.42. The SVM results are detailed in Figures 5E, F, as well as in Table 2.
3.6 The expression analysis and ROC curve analysis of hub genes
We investigated the expression of these two genes in the GSE12649 dataset and GSE5392 validation dataset, comparing BD and control samples. As shown in Figure 6A, CX3CR1 and ST6GAL1 showed a significantly lower expression in BD, so as the GSE5392 validation dataset (Figures 7A, C). Likewise, we used the AS dataset GSE100927 for validation, and our findings revealed that the expression level of hub genes was higher in the AS group (Figure 7E). ROC analysis was used to verify the specificity and sensitivity of the two hub genes for BD and AS diagnosis. The AUC areas of the two hub genes in GSE12649 and GSE5392 were around 0.7, indicating their diagnostic value (Figures 7B, D). In GSE100927 dataset, the AUC areas of two hub genes were > 0.8, showing that the hub genes had good diagnostic values.
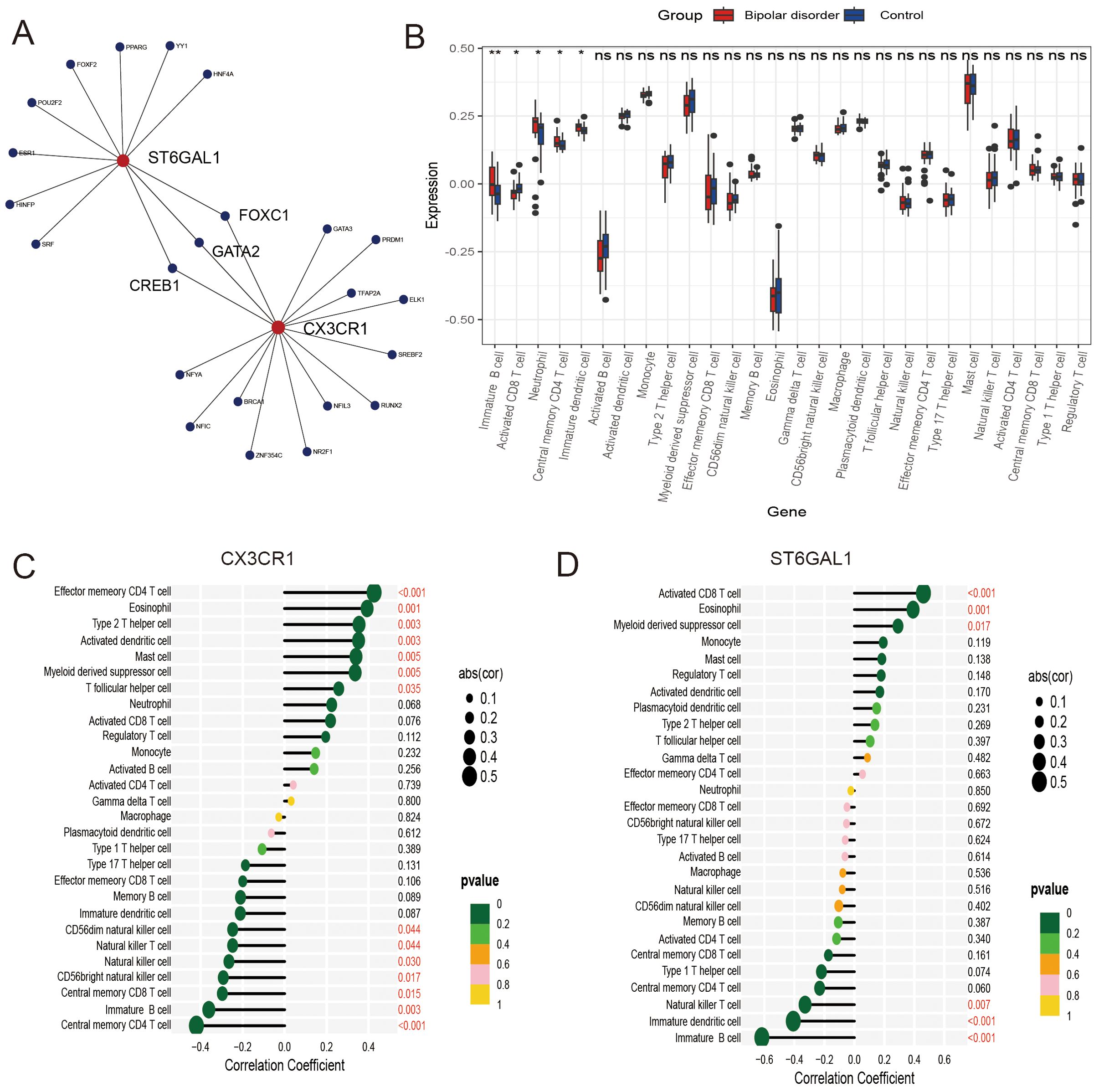
Figure 6. Regulatory networks and immune infiltration analysis for hub genes. (A) Transcription factor-key gene interaction network. (B) The difference in immune infiltration between bipolar disorder samples and control samples. (C, D) Correlation between CX3CR1 and ST6GAL1 with immune infiltrating cells.
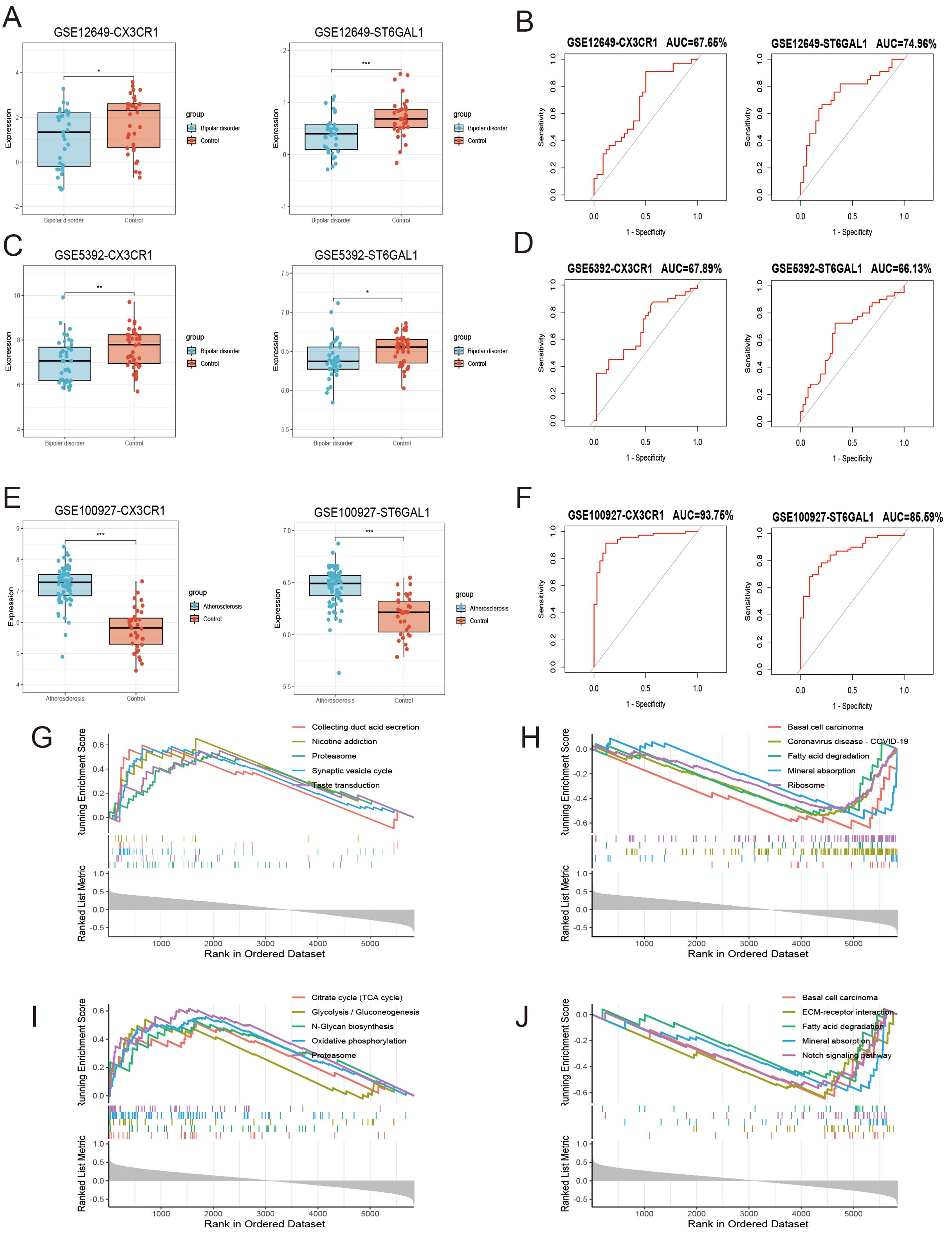
Figure 7. Hub gene expression level, receiver operating characteristic (ROC) analysis and the functions of hub genes including CX3CR1 and ST6GAL1. (A, C, E) Expression levels of hub genes in GSE12639 (A), GSE5392 (C), GSE100927 (E). (B, D, F) The ROC curve analysis of hub genes in GSE12649 (B), GSE5392 (D), GSE100927 (F). (G-J) The activating and inhibiting pathways of CX3CR1 (G, H) and ST6GAL1 (I, J) using GSEA analysis.
3.7 Single-gene GSEA analysis and identification of transcription factors
Single-gene GSEA analysis was performed on these two hub genes to obtain the related pathways of each gene. The results showed its correlation with proteasome, basal cell carcinoma, fatty acid degradation, mineral absorption and so on (Figures 7G-J). The interaction network consisted of two hub genes and 23 TFs (Transcription Factors) (Figure 6A). ST6GAL1 was regulated by eleven TFs, while CX3CR1 was regulated by fifteen TFs. Moreover, FOXC1, GATA2, and CREB1 were found to interact with both hub genes, indicating a potential close interaction between these TFs and the hub genes.
3.8 Correlation analysis between hub genes and immune cells
To further understand the involvement of the hub genes in immune infiltration, we conducted Spearman correlation analysis to investigate the potential relationships between these hub genes and immune cell infiltration. The results showed significantly higher levels of immature B cells, neutrophils, central memory CD4 T cells, and immature dendritic cells, as well as significantly lower levels of activated CD8 T cells in the BD group compared to the control group, as seen in the box plot (Figure 6B). Our findings regarding the hub genes, CX3CR1 and ST6GAL1, were consistently exhibited significantly positive correlations with eosinophils, while demonstrating significantly negative correlations with immature B cells (Figures 6C, D).
4 Discussion
BD is a chronic and disabling affective disorder with significant high rate of physical comorbidities. Taking into account efficacious psychopharmacological treatment and adequate follow-up regarding physical comorbidities is critical for reducing the cardiovascular disease risk in patients with BD. BD is known to be a risk factor for accelerated early CVDs (20). Characterized by arterial stiffness, AS may play a role in increasing the risk of CVDs in patients with BD and should be further investigated (12). While the clinical relationship between BD and AS has been well-discussed (12, 18, 38), the pathogenesis underlying this relationship are not fully understood. Therefore, it is essential to explore the molecular changes of these two diseases and provided a theoretical basis for the in-depth understanding of the pathogenesis.
In our study, we identified 67 overlapping DE-ASRGs between BD patients and healthy controls, based on analysis of microarray datasets. Our GO functional enrichment analyses revealed that these DE-ASRGs were closely linked to various biological processes, including signal transduction, positive and negative regulation of translation from RNA pol II promoter, apoptotic processes, cell adhesion, and positive regulation of protein kinase B signaling. These findings indicated that the DE-ASRGs played crucial roles in intracellular signaling and regulatory processes, influencing cell survival, proliferation, and adaptation. Furthermore, our KEGG enrichment analysis showed that the DE-ASRGs were predominantly associated with the cancer pathway, PI3K-Akt signaling pathway, HPV, and focal adhesion. Recent studies have found that the PI3K-Akt pathway plays a crucial role in cerebrovascular diseases, metabolic syndrome, and mental disorders. The PI3K-Akt pathway can serve as a therapeutic target for brain aging and neurodegenerative changes (39). One study suggests that there may be a connection between bipolar disorder and obesity through the PI3K-Akt pathway (40). The PI3K-Akt pathway and focal adhesion, as potential genetic defects in BD and therapeutic targets of lithium, play a role in axonal growth and neuronal development (41). Previous studies have found that lithium is a protective factor for CVDs (42). Based on the founding of this study, the PI3K-Akt pathway and focal adhesion may be potential targets for lithium to reduce the risk of CVDs in BD patients. Interestingly, considering the correlation between PI3K-Akt signaling pathway (43, 44), HPV (45), and focal adhesion (46) with cancer, it could be inferred that cancer pathways may play an important role between BD and AS.
By comprehensive analysis of gene expression profiles, CX3CR1 and ST6GAL1 were identified as two key hub genes between BD and AS. CX3CR1, a crucial chemokine receptor in the G protein-coupled receptor superfamily (47), is the only proinflammatory leukocyte receptor specific for the chemokine fractalkine (CX3CL1) (48), and the preservation of normal CX3CL1/CX3CR1 signaling seems to be essential for normal brain function. The expression of CX3CR1 is largely restricted to microglia within the brain parenchyma, where they play a role in remodeling neuronal circuits, serving as resident phagocytic cells involved in immune-mediated defense mechanisms, clearing damaged cell debris, and contributing to the regulation of homeostatic synaptic plasticity (49, 50). In the absence of normal CX3CL1/CX3CR1 signaling, aberrant microglial activation and elevated microglial proinflammatory activity could increase neurotoxicity, since CX3CL1/CX3CR1 signaling decreases the overproduction of inducible nitric oxide synthase, interleukin (IL)-1β, tumor necrosis factor-α (TNF-α), IL-6, and mediators of oxidative stress (48, 51). As one of the chemokines, CX3CL1 can regulate the population of central nervous system (CNS) tissue with peripherally derived cell types by relying on the activation of the CX3CR1 receptor and inhibiting the migration of astrocytes through the PI3K activity (52). Previous findings supported the notion that CX3CL1/CX3CR1 communication serves as an “off” signal, maintaining microglia in a “resting” state (53, 54).
CX3CR1 has been shown to be associated with neurodegenerative disorders and to exhibit neuroprotective effects (48). Recent conceptualizations characterize BD as a neurodegenerative disorder, marked by the progressive deterioration of brain volumes and accelerated brain aging (55). Consistent with the results of this study, significantly reduced CX3CR1 transcript level was observed in patients with BD relative to controls (56). Another study suggested that psychiatric therapy activates CX3CR1 (40), which indicated that psychiatric therapy can play a therapeutic role in reducing inflammatory response. It was speculated that the relationship between CX3CR1 and BD may be attributed to abnormal neuroinflammatory conditions. For the role of CX3CR1 in AS, several lines of evidence implicated CX3CL1/CX3CR1 in the pathogenesis of vascular inflammation injury (47). It could be observed that CX3CL1/CX3CR1 was activated by inflammatory stimuli, including TNF-a, IFN-γ, and LPS (57). CX3CL1 could act as a classical chemoattractant for T lymphocytes (58), and dendritic cells (59), which was consistent with our immune infiltration results. As chemokines and adhesion molecules, CX3CL1/CX3CR1 can directly mediate the interaction between inflammatory cells and vascular cells, and promote the plaque formation and development (47). Considering that the decrease of CX3CR1 in CNS will promote the occurrence of inflammation, the inflammatory situation may be a common physiological mechanism of BD and AS. Consistent with previous studies (60, 61), CX3CR1 had been shown to be upregulated in the AS validation group in this study. Interestingly, CX3CR1 exhibited opposite changes in BD and AS, which may be due to differences in sampling locations and the differential expression levels in the brain and vascular cells.
ST6GAL1 has anti-inflammatory effects by catalyzing sialylation of other molecules including receptors, lectins, and cytokines (62, 63). The lack of ST6GAL1 represents an excessive inflammatory response, with higher levels of neutrophilic and eosinophilic response (62, 64). As we found in this study, ST6GAL1 levels were lower than normal in BD patients, which may have contributed to the excessive of inflammation in BD patients. Large scale association analysis identified ST6GAL1 as a protective effect on CVDs occurrence (65). ST6GAL1 is strongly expressed in large blood vessels, including the aorta, and related to the angiogenic process (66). A previous study had shown that the overexpression of ST6GAL1 strongly inhibits monocyte-transendothelial migration, suggesting that ST6GAL1 could be a potential target for atherosclerosis prevention and treatment (67). However, the role of ST6GAL1 between BD and AS is not yet clear, and more research is needed to elucidate the direct relationship between them. Given that both conditions entail abnormal immune responses, the interplay between CX3CR1 and ST6GAL1 may serve as a key mechanism to understand the association between BD and AS.
It should be noted that two hub genes both related to cancer, which were identified as the key pathway through KEGG enrichment analysis in this study. CX3CL1/CX3CR1 has a tumor-suppressive activity by recruiting antitumoral immune cells such as NK and T cells into the tumor microenvironment to control tumor growth (68). Previous study observed that CX3CR1 ectopic expression improved the recruitment of adoptively transferred T cells toward CX3CL1-generated cancers, leading to the augmentation of T-cell infiltration and reduction of tumor growth (69). In addition, CX3CL1/CX3CR1 axis (70) and ST6GAL1 (71) has been confirmed to mediates several cellular functions, including activation of PI3K-Akt. ST6GAL1 has become increasingly dominant in sialyltransferase activity, which are implicated in cancer (72). ST6GAL1 is known to promote growth, survival, and metastasis, and it is upregulated in various types of cancer (including pancreatic, prostate, breast, and ovarian cancer) (73–76), while being downregulated in hepatocellular carcinoma (77). ST6GAL1 expression is thought to be associated with increased invasiveness and metastasis (78). Knockout ST6GAL1, lacking the α2,6-sialylation enzyme, is shown to exhibit impaired tumor angiogenesis through enhanced endothelial apoptosis (79). Patients with BD are more likely to develop malignant cancer than the general population, which may imply a genetic overlap in neurodevelopment and malignancy pathogenesis (80). Besides, AS are major risk factors for cancer (81). Therefore, the cancer pathway may serve as a bridge between BD and AS.
It is important to mention that, BD has been linked to clinical signs of accelerated aging, potentially explaining its association with age-related medical conditions including cancer (82). Aging is characterized by the functional decline of the immune system and is the primary risk factor for infectious diseases, CVDs, cancer, and neurodegenerative disorders (83). Given that arterial stiffness in AS is often considered a signal of vascular aging (11), it is possible that aging could be a co-pathogenesis of the two diseases. Further research on the biological mechanisms of aging and cancer between BD and AS is needed. Furthermore, both CX3CR1 and ST6GAL1 have been linked to cognitive impairment (84, 85). Among the co-regulated TFs, CREB1 has been identified as a risk factor for cognitive impairment in patients with BD (86). Given the unambiguous correlation between BD and AS and cognitive impairment (87, 88), cognition may serve as a critical connecting symptom between BD and AS.
5 Limitation
While we have speculated on the potential association between BD and AS based on bioinformatics analysis, there are some limitations to consider. Firstly, the absence of confounding variables in the GSE12649 dataset made it difficult to assess the stability of the differential analysis. Secondly, the DisGeNET database utilized in this study allows users to obtain genes relevant to particular diseases (89). However, this approach may filter out some potentially valuable molecules. In addition, the different sampling locations limit the interpretability of hub genes. Finally, considering feature selection algorithms appear to possess poor reproducibility in different datasets, wet experiments are needed to further verify the predictions.
6 Conclusions
In the present study, we utilized bioinformatic techniques including three machine learning approaches to identify 2 hub genes, CX3CR1 and ST6GAL1, which were both significantly related to BD and AS. Furthermore, we uncovered that the co-pathogenesis of the two diseases lies in the cancer-related pathways through GSEA analysis. Overall, the newly discovered diagnostic genes and potential molecular mechanisms in this study offer new clinical insights and guidance for diagnosing and treating BD and AS patients. However, further experimentation is needed to confirm the conclusions.
Data availability statement
The datasets presented in this study can be found in online repositories. The names of the repository/repositories and accession number(s) can be found in the article/Supplementary Material. Further inquiries can be directed to the corresponding author/s.
Ethics statement
Ethical approval was not required for the studies on humans in accordance with the local legislation and institutional requirements because only commercially available established cell lines were used.
Author contributions
XBZ: Conceptualization, Formal Analysis, Visualization, Writing – original draft, Writing – review & editing. XZZ: Data curation, Methodology, Project administration, Writing – original draft, Writing – review & editing. YZ: Investigation, Validation, Writing – original draft. CC: Resources, Visualization, Writing – review & editing. EJ: Supervision, Writing – original draft, Writing – review & editing.
Funding
The author(s) declare financial support was received for the research, authorship, and/or publication of this article. This work was supported by the Shenzhen Fund for Guangdong Provincial High-level Clinical Key Specialties (No. SZGSP013) and Shenzhen Key Medical Discipline Construction Fund (No.SZXK043).
Acknowledgments
The authors would like to thank the GEO, DAVID, DisGeNET, STRING databases for the availability of the data.
Conflict of interest
The authors declare that the research was conducted in the absence of any commercial or financial relationships that could be construed as a potential conflict of interest.
Publisher’s note
All claims expressed in this article are solely those of the authors and do not necessarily represent those of their affiliated organizations, or those of the publisher, the editors and the reviewers. Any product that may be evaluated in this article, or claim that may be made by its manufacturer, is not guaranteed or endorsed by the publisher.
Supplementary material
The Supplementary Material for this article can be found online at: https://www.frontiersin.org/articles/10.3389/fpsyt.2024.1392437/full#supplementary-material
References
1. Nierenberg AA, Agustini B, Köhler-Forsberg O, Cusin C, Katz D, Sylvia LG, et al. Diagnosis and treatment of bipolar disorder: A review. Jama. (2023) 330:1370–80. doi: 10.1001/jama.2023.18588
2. Vigo D, Thornicroft G, Atun R. Estimating the true global burden of mental illness. Lancet Psychiatry. (2016) 3:171–8. doi: 10.1016/S2215-0366(15)00505-2
3. Oldis M, Murray G, Macneil CA, Hasty MK, Daglas R, Berk M, et al. Trajectory and predictors of quality of life in first episode psychotic mania. J Affect Disord. (2016) 195:148–55. doi: 10.1016/j.jad.2016.02.018
4. Vieta E, Berk M, Schulze TG, Carvalho AF, Suppes T, Calabrese JR, et al. Bipolar disorders. Nat Rev Dis Primers. (2018) 4:18008. doi: 10.1038/nrdp.2018.8
5. Hayes JF, Miles J, Walters K, King M, Osborn DP. A systematic review and meta-analysis of premature mortality in bipolar affective disorder. Acta Psychiatr Scand. (2015) 131:417–25. doi: 10.1111/acps.12408
6. Correll CU, Solmi M, Veronese N, Bortolato B, Rosson S, Santonastaso P, et al. Prevalence, incidence and mortality from cardiovascular disease in patients with pooled and specific severe mental illness: a large-scale meta-analysis of 3,211,768 patients and 113,383,368 controls. World Psychiatry. (2017) 16:163–80. doi: 10.1002/wps.20420
7. Fiedorowicz JG, Solomon DA, Endicott J, Leon AC, Li C, Rice JP, et al. Manic/hypomanic symptom burden and cardiovascular mortality in bipolar disorder. Psychosom Med. (2009) 71:598–606. doi: 10.1097/PSY.0b013e3181acee26
8. Goldstein BI, Schaffer A, Wang S, Blanco C. Excessive and premature new-onset cardiovascular disease among adults with bipolar disorder in the US NESARC cohort. J Clin Psychiatry. (2015) 76:163–9. doi: 10.4088/JCP.14m09300
9. Murakami T. Atherosclerosis and arteriosclerosis. Hypertens Res. (2023) 46:1810–1. doi: 10.1038/s41440-023-01284-0
10. Mitchell GF, Powell JT. Arteriosclerosis: A primer for "In focus" Reviews on arterial stiffness. Arterioscler Thromb Vasc Biol. (2020) 40:1025–7. doi: 10.1161/ATVBAHA.120.314208
11. Boutouyrie P, Chowienczyk P, Humphrey JD, Mitchell GF. Arterial stiffness and cardiovascular risk in hypertension. Circ Res. (2021) 128:864–86. doi: 10.1161/CIRCRESAHA.121.318061
12. Kılıçaslan AK, Emir BS, Yildiz S, Kılıçaslan G, Kurt O. Arterial stiffness in patients with bipolar disorder. Clin Psychopharmacol Neurosci. (2023) 21:516–25. doi: 10.9758/cpn.22.1009
13. Tsai SY, Shen RS, Kuo CJ, Chen PH, Chung KH, Hsiao CY, et al. The association between carotid atherosclerosis and treatment with lithium and antipsychotics in patients with bipolar disorder. Aust N Z J Psychiatry. (2020) 54:1125–34. doi: 10.1177/0004867420952551
14. Bortolasci CC, Vargas HO, Vargas Nunes SO, De Melo LG, De Castro MR, Moreira EG, et al. Factors influencing insulin resistance in relation to atherogenicity in mood disorders, the metabolic syndrome and tobacco use disorder. J Affect Disord. (2015) 179:148–55. doi: 10.1016/j.jad.2015.03.041
15. Rajagopalan S, Brook R, Rubenfire M, Pitt E, Young E, Pitt B. Abnormal brachial artery flow-mediated vasodilation in young adults with major depression. Am J Cardiol. (2001) 88:196–198, a197. doi: 10.1016/S0002-9149(01)01623-X
16. Rybakowski JK, Wykretowicz A, Heymann-Szlachcinska A, Wysocki H. Impairment of endothelial function in unipolar and bipolar depression. Biol Psychiatry. (2006) 60:889–91. doi: 10.1016/j.biopsych.2006.03.025
17. Fiedorowicz JG, Coryell WH, Rice JP, Warren LL, Haynes WG. Vasculopathy related to manic/hypomanic symptom burden and first-generation antipsychotics in a sub-sample from the collaborative depression study. Psychother Psychosom. (2012) 81:235–43. doi: 10.1159/000334779
18. Schmitz SL, Abosi OJ, Persons JE, Sinkey CA, Fiedorowicz JG. Impact of mood on endothelial function and arterial stiffness in bipolar disorder. Heart Mind (Mumbai). (2018) 2:78–84. doi: 10.4103/hm.hm_20_19
19. Nunes SO, Piccoli De Melo LG, Pizzo De Castro MR, Barbosa DS, Vargas HO, Berk M, et al. Atherogenic index of plasma and atherogenic coefficient are increased in major depression and bipolar disorder, especially when comorbid with tobacco use disorder. J Affect Disord. (2015) 172:55–62. doi: 10.1016/j.jad.2014.09.038
20. Goldstein BI, Carnethon MR, Matthews KA, Mcintyre RS, Miller GE, Raghuveer G, et al. Major depressive disorder and bipolar disorder predispose youth to accelerated atherosclerosis and early cardiovascular disease: A scientific statement from the American heart association. Circulation. (2015) 132:965–86. doi: 10.1161/CIR.0000000000000229
21. Marshe VS, Pira S, Mantere O, Bosche B, Looper KJ, Herrmann N, et al. C-reactive protein and cardiovascular risk in bipolar disorder patients: A systematic review. Prog Neuropsychopharmacol Biol Psychiatry. (2017) 79:442–51. doi: 10.1016/j.pnpbp.2017.07.026
22. Morris G, Berk M, Walder K, O'neil A, Maes M, Puri BK. The lipid paradox in neuroprogressive disorders: Causes and consequences. Neurosci Biobehav Rev. (2021) 128:35–57. doi: 10.1016/j.neubiorev.2021.06.017
23. Wang T, Rentería ME, Tian Z, Peng J. Editorial: Data mining and statistical methods for knowledge discovery in diseases based on multimodal omics, volume II. Front Genet. (2023) 14:1270862. doi: 10.3389/fgene.2023.1270862
24. Mühleisen TW, Leber M, Schulze TG, Strohmaier J, Degenhardt F, Treutlein J, et al. Genome-wide association study reveals two new risk loci for bipolar disorder. Nat Commun. (2014) 5:3339. doi: 10.1038/ncomms4339
25. Prieto ML, Ryu E, Jenkins GD, Batzler A, Nassan MM, Cuellar-Barboza AB, et al. Leveraging electronic health records to study pleiotropic effects on bipolar disorder and medical comorbidities. Transl Psychiatry. (2016) 6:e870. doi: 10.1038/tp.2016.138
26. Amare AT, Schubert KO, Klingler-Hoffmann M, Cohen-Woods S, Baune BT. The genetic overlap between mood disorders and cardiometabolic diseases: a systematic review of genome wide and candidate gene studies. Transl Psychiatry. (2017) 7:e1007. doi: 10.1038/tp.2016.261
27. Wang T, Rentería ME, Peng J. Editorial: data mining and statistical methods for knowledge discovery in diseases based on multimodal omics. Front Genet. (2022) 13:895796. doi: 10.3389/fgene.2022.895796
28. Vieta E. The bipolar maze: a roadmap through translational psychopathology. Acta Psychiatr Scand. (2014) 129:323–7. doi: 10.1111/acps.12270
29. Ritchie ME, Phipson B, Wu D, Hu Y, Law CW, Shi W, et al. limma powers differential expression analyses for RNA-sequencing and microarray studies. Nucleic Acids Res. (2015) 43:e47. doi: 10.1093/nar/gkv007
30. Piñero J, Bravo À., Queralt-Rosinach N, Gutiérrez-Sacristán A, Deu-Pons J, Centeno E, et al. DisGeNET: a comprehensive platform integrating information on human disease-associated genes and variants. Nucleic Acids Res. (2017) 45:D833–d839. doi: 10.1093/nar/gkw943
31. Chen L, Zhang YH, Wang S, Zhang Y, Huang T, Cai YD. Prediction and analysis of essential genes using the enrichments of gene ontology and KEGG pathways. PLoS One. (2017) 12:e0184129. doi: 10.1371/journal.pone.0184129
32. Kanehisa M, Furumichi M, Tanabe M, Sato Y, Morishima K. KEGG: new perspectives on genomes, pathways, diseases and drugs. Nucleic Acids Res. (2017) 45:D353–d361. doi: 10.1093/nar/gkw1092
33. Langfelder P, Horvath S. WGCNA: an R package for weighted correlation network analysis. BMC Bioinf. (2008) 9:559. doi: 10.1186/1471-2105-9-559
34. Friedman J, Hastie T, Tibshirani R. Regularization paths for generalized linear models via coordinate descent. J Stat Softw. (2010) 33:1–22. doi: 10.18637/jss.v033.i01
35. Blanchet L, Vitale R, Van Vorstenbosch R, Stavropoulos G, Pender J, Jonkers D, et al. Constructing bi-plots for random forest: Tutorial. Anal Chim Acta. (2020) 1131:146–55. doi: 10.1016/j.aca.2020.06.043
36. Huang ML, Hung YH, Lee WM, Li RK, Jiang BR. SVM-RFE based feature selection and Taguchi parameters optimization for multiclass SVM classifier. ScientificWorldJournal. (2014) 2014:795624. doi: 10.1155/2014/795624
37. Noble WS. What is a support vector machine? Nat Biotechnol. (2006) 24:1565–7. doi: 10.1038/nbt1206-1565
38. Sodhi SK, Linder J, Chenard CA, Miller Del D, Haynes WG, Fiedorowicz JG. Evidence for accelerated vascular aging in bipolar disorder. J Psychosom Res. (2012) 73:175–9. doi: 10.1016/j.jpsychores.2012.06.004
39. Fakhri S, Iranpanah A, Gravandi MM, Moradi SZ, Ranjbari M, Majnooni MB, et al. Natural products attenuate PI3K/Akt/mTOR signaling pathway: A promising strategy in regulating neurodegeneration. Phytomedicine. (2021) 91:153664. doi: 10.1016/j.phymed.2021.153664
40. Kambey PA, Kodzo LD, Serojane F, Oluwasola BJ. The bi-directional association between bipolar disorder and obesity: Evidence from Meta and bioinformatics analysis. Int J Obes (Lond). (2023) 47:443–52. doi: 10.1038/s41366-023-01277-6
41. Ou AH, Rosenthal SB, Adli M, Akiyama K, Akula N, Alda M, et al. Lithium response in bipolar disorder is associated with focal adhesion and PI3K-Akt networks: a multi-omics replication study. Transl Psychiatry. (2024) 14:109. doi: 10.1038/s41398-024-02811-4
42. Tsai SY, Kuo CJ, Sajatovic M, Huang YJ, Chen PH, Chung KH. Lithium exposure and chronic inflammation with activated macrophages and monocytes associated with atherosclerosis in bipolar disorder. J Affect Disord. (2022) 314:233–40. doi: 10.1016/j.jad.2022.07.024
43. Noorolyai S, Shajari N, Baghbani E, Sadreddini S, Baradaran B. The relation between PI3K/AKT signalling pathway and cancer. Gene. (2019) 698:120–8. doi: 10.1016/j.gene.2019.02.076
44. He Y, Sun MM, Zhang GG, Yang J, Chen KS, Xu WW, et al. Targeting PI3K/Akt signal transduction for cancer therapy. Signal Transduct Target Ther. (2021) 6:425. doi: 10.1038/s41392-021-00828-5
45. Rahangdale L, Mungo C, O'connor S, Chibwesha CJ, Brewer NT. Human papillomavirus vaccination and cervical cancer risk. Bmj. (2022) 379:e070115. doi: 10.1136/bmj-2022-070115
46. Zhang Z, Li J, Jiao S, Han G, Zhu J, Liu T. Functional and clinical characteristics of focal adhesion kinases in cancer progression. Front Cell Dev Biol. (2022) 10:1040311. doi: 10.3389/fcell.2022.1040311
47. Liu H, Jiang D. Fractalkine/CX3CR1 and atherosclerosis. Clin Chim Acta. (2011) 412:1180–6. doi: 10.1016/j.cca.2011.03.036
48. Subbarayan MS, Joly-Amado A, Bickford PC, Nash KR. CX3CL1/CX3CR1 signaling targets for the treatment of neurodegenerative diseases. Pharmacol Ther. (2022) 231:107989. doi: 10.1016/j.pharmthera.2021.107989
49. Ransohoff RM, Cardona AE. The myeloid cells of the central nervous system parenchyma. Nature. (2010) 468:253–62. doi: 10.1038/nature09615
50. Schafer DP, Lehrman EK, Kautzman AG, Koyama R, Mardinly AR, Yamasaki R, et al. Microglia sculpt postnatal neural circuits in an activity and complement-dependent manner. Neuron. (2012) 74:691–705. doi: 10.1016/j.neuron.2012.03.026
51. Lyons A, Lynch AM, Downer EJ, Hanley R, O'sullivan JB, Smith A, et al. Fractalkine-induced activation of the phosphatidylinositol-3 kinase pathway attentuates microglial activation in vivo and in vitro. J Neurochem. (2009) 110:1547–56. doi: 10.1111/j.1471-4159.2009.06253.x
52. Sheridan GK, Murphy KJ. Neuron-glia crosstalk in health and disease: fractalkine and CX3CR1 take centre stage. Open Biol. (2013) 3:130181. doi: 10.1098/rsob.130181
53. Biber K, Neumann H, Inoue K, Boddeke HW. Neuronal 'On' and 'Off' signals control microglia. Trends Neurosci. (2007) 30:596–602. doi: 10.1016/j.tins.2007.08.007
54. Milior G, Lecours C, Samson L, Bisht K, Poggini S, Pagani F, et al. Fractalkine receptor deficiency impairs microglial and neuronal responsiveness to chronic stress. Brain Behav Immun. (2016) 55:114–25. doi: 10.1016/j.bbi.2015.07.024
55. Zovetti N, Rossetti MG, Perlini C, Brambilla P, Bellani M. Brain ageing and neurodegeneration in bipolar disorder. J Affect Disord. (2023) 323:171–5. doi: 10.1016/j.jad.2022.11.066
56. Padmos RC, Hillegers MH, Knijff EM, Vonk R, Bouvy A, Staal FJ, et al. A discriminating messenger RNA signature for bipolar disorder formed by an aberrant expression of inflammatory genes in monocytes. Arch Gen Psychiatry. (2008) 65:395–407. doi: 10.1001/archpsyc.65.4.395
57. Ludwig A, Berkhout T, Moores K, Groot P, Chapman G. Fractalkine is expressed by smooth muscle cells in response to IFN-gamma and TNF-alpha and is modulated by metalloproteinase activity. J Immunol. (2002) 168:604–12. doi: 10.4049/jimmunol.168.2.604
58. Foussat A, Coulomb-L'hermine A, Gosling J, Krzysiek R, Durand-Gasselin I, Schall T, et al. Fractalkine receptor expression by T lymphocyte subpopulations and in vivo production of fractalkine in human. Eur J Immunol. (2000) 30:87–97. doi: 10.1002/(ISSN)1521-4141
59. Dichmann S, Herouy Y, Purlis D, Rheinen H, Gebicke-Härter P, Norgauer J. Fractalkine induces chemotaxis and actin polymerization in human dendritic cells. Inflammation Res. (2001) 50:529–33. doi: 10.1007/PL00000230
60. Wong BW, Wong D, Mcmanus BM. Characterization of fractalkine (CX3CL1) and CX3CR1 in human coronary arteries with native atherosclerosis, diabetes mellitus, and transplant vascular disease. Cardiovasc Pathol. (2002) 11:332–8. doi: 10.1016/S1054-8807(02)00111-4
61. Combadière C, Potteaux S, Gao JL, Esposito B, Casanova S, Lee EJ, et al. Decreased atherosclerotic lesion formation in CX3CR1/apolipoprotein E double knockout mice. Circulation. (2003) 107:1009–16. doi: 10.1161/01.CIR.0000057548.68243.42
62. Jones MB, Nasirikenari M, Feng L, Migliore MT, Choi KS, Kazim L, et al. Role for hepatic and circulatory ST6Gal-1 sialyltransferase in regulating myelopoiesis. J Biol Chem. (2010) 285:25009–17. doi: 10.1074/jbc.M110.104406
63. Liu Z, Swindall AF, Kesterson RA, Schoeb TR, Bullard DC, Bellis SL. ST6Gal-I regulates macrophage apoptosis via α2-6 sialylation of the TNFR1 death receptor. J Biol Chem. (2011) 286:39654–62. doi: 10.1074/jbc.M111.276063
64. Nasirikenari M, Segal BH, Ostberg JR, Urbasic A, Lau JT. Altered granulopoietic profile and exaggerated acute neutrophilic inflammation in mice with targeted deficiency in the sialyltransferase ST6Gal I. Blood. (2006) 108:3397–405. doi: 10.1182/blood-2006-04-014779
65. Saade S, Cazier JB, Ghassibe-Sabbagh M, Youhanna S, Badro DA, Kamatani Y, et al. Large scale association analysis identifies three susceptibility loci for coronary artery disease. PloS One. (2011) 6:e29427. doi: 10.1371/journal.pone.0029427
66. Javerzat S, Franco M, Herbert J, Platonova N, Peille AL, Pantesco V, et al. Correlating global gene regulation to angiogenesis in the developing chick extra-embryonic vascular system. PloS One. (2009) 4:e7856. doi: 10.1371/journal.pone.0007856
67. Zhang J, Liu Y, Deng X, Chen L, Yang X, Yu C. ST6GAL1 negatively regulates monocyte transendothelial migration and atherosclerosis development. Biochem Biophys Res Commun. (2018) 500:249–55. doi: 10.1016/j.bbrc.2018.04.053
68. Lu X. Structure and function of ligand CX3CL1 and its receptor CX3CR1 in cancer. Curr Med Chem. (2022) 29:6228–46. doi: 10.2174/0929867329666220629140540
69. Siddiqui I, Erreni M, Van Brakel M, Debets R, Allavena P. Enhanced recruitment of genetically modified CX3CR1-positive human T cells into Fractalkine/CX3CL1 expressing tumors: importance of the chemokine gradient. J Immunother Cancer. (2016) 4:21. doi: 10.1186/s40425-016-0125-1
70. Rivas-Fuentes S, Salgado-Aguayo A, Arratia-Quijada J, Gorocica-Rosete P. Regulation and biological functions of the CX3CL1-CX3CR1 axis and its relevance in solid cancer: A mini-review. J Cancer. (2021) 12:571–83. doi: 10.7150/jca.47022
71. Irons EE, Lau JTY. Systemic ST6Gal-1 is a pro-survival factor for murine transitional B cells. Front Immunol. (2018) 9 2150. doi: 10.3389/fimmu.2018.02150
72. Garnham R, Scott E, Livermore KE, Munkley J. ST6GAL1: A key player in cancer. Oncol Lett. (2019) 18:983–9. doi: 10.3892/ol.2019.10458
73. Dall'olio F. The sialyl-alpha2,6-lactosaminyl-structure: biosynthesis and functional role. Glycoconj J. (2000) 17:669–76. doi: 10.1023/A:1011077000164
74. Wei A, Fan B, Zhao Y, Zhang H, Wang L, Yu X, et al. ST6Gal-I overexpression facilitates prostate cancer progression via the PI3K/Akt/GSK-3β/β-catenin signaling pathway. Oncotarget. (2016) 7:65374–88. doi: 10.18632/oncotarget.v7i40
75. Hsieh CC, Shyr YM, Liao WY, Chen TH, Wang SE, Lu PC, et al. Elevation of β-galactoside α2,6-sialyltransferase 1 in a fructoseresponsive manner promotes pancreatic cancer metastasis. Oncotarget. (2017) 8:7691–709. doi: 10.18632/oncotarget.v8i5
76. Wichert B, Milde-Langosch K, Galatenko V, Schmalfeldt B, Oliveira-Ferrer L. Prognostic role of the sialyltransferase ST6GAL1 in ovarian cancer. Glycobiology. (2018) 28:898–903. doi: 10.1093/glycob/cwy065
77. Liu R, Cao X, Liang Y, Li X, Jin Q, Li Y, et al. Downregulation of ST6GAL1 promotes liver inflammation and predicts adverse prognosis in hepatocellular carcinoma. J Inflammation Res. (2022) 15:5801–14. doi: 10.2147/JIR.S385491
78. Antony P, Rose M, Heidenreich A, Knüchel R, Gaisa NT, Dahl E. Epigenetic inactivation of ST6GAL1 in human bladder cancer. BMC Cancer. (2014) 14:901. doi: 10.1186/1471-2407-14-901
79. Imamaki R, Ogawa K, Kizuka Y, Komi Y, Kojima S, Kotani N, et al. Glycosylation controls cooperative PECAM-VEGFR2-β3 integrin functions at the endothelial surface for tumor angiogenesis. Oncogene. (2018) 37:4287–99. doi: 10.1038/s41388-018-0271-7
80. Chen MH, Tsai SJ, Su TP, Li CT, Lin WC, Cheng CM, et al. Cancer risk in patients with bipolar disorder and unaffected siblings of such patients: A nationwide population-based study. Int J Cancer. (2022) 150:1579–86. doi: 10.1002/ijc.33914
81. Dana PM, Sadoughi F, Mobini M, Shafabakhsh R, Chaichian S, Moazzami B, et al. Molecular and biological functions of melatonin in endometrial cancer. Curr Drug Targets. (2020) 21:519–26. doi: 10.2174/1389450120666190927123746
82. Fries GR, Zamzow MJ, Andrews T, Pink O, Scaini G, Quevedo J. Accelerated aging in bipolar disorder: A comprehensive review of molecular findings and their clinical implications. Neurosci Biobehav Rev. (2020) 112:107–16. doi: 10.1016/j.neubiorev.2020.01.035
83. Watanabe R, Hashimoto M. Aging-related vascular inflammation: giant cell arteritis and neurological disorders. Front Aging Neurosci. (2022) 14:843305. doi: 10.3389/fnagi.2022.843305
84. Lee E, Giovanello KS, Saykin AJ, Xie F, Kong D, Wang Y, et al. Single-nucleotide polymorphisms are associated with cognitive decline at Alzheimer's disease conversion within mild cognitive impairment patients. Alzheimers Dement (Amst). (2017) 8:86–95. doi: 10.1016/j.dadm.2017.04.004
85. Puntambekar SS, Moutinho M, Lin PB, Jadhav V, Tumbleson-Brink D, Balaji A, et al. CX3CR1 deficiency aggravates amyloid driven neuronal pathology and cognitive decline in Alzheimer's disease. Mol Neurodegener. (2022) 17:47. doi: 10.1186/s13024-022-00545-9
86. Wang X, Zhang G, Lu W, Zhang Y, Fan W, Tang W, et al. Common variants in CREB1 gene confer risk for bipolar disorder in Han Chinese. Asian J Psychiatr. (2021) 59:102648. doi: 10.1016/j.ajp.2021.102648
87. Solé B, Jiménez E, Torrent C, Reinares M, Bonnin CDM, Torres I, et al. Cognitive impairment in bipolar disorder: treatment and prevention strategies. Int J Neuropsychopharmacol. (2017) 20:670–80. doi: 10.1093/ijnp/pyx032
88. Blevins BL, Vinters HV, Love S, Wilcock DM, Grinberg LT, Schneider JA, et al. Brain arteriolosclerosis. Acta Neuropathol. (2021) 141:1–24. doi: 10.1007/s00401-020-02235-6
Keywords: bipolar disorder, arteriosclerosis, bioinformatics, hub genes, CX3CR1, ST6GAL1
Citation: Zheng X, Zhang X, Zhang Y, Chen C and Ji E (2024) Identification of significant biomarkers for predicting the risk of bipolar disorder with arteriosclerosis based on integrative bioinformatics and machine learning. Front. Psychiatry 15:1392437. doi: 10.3389/fpsyt.2024.1392437
Received: 04 April 2024; Accepted: 19 August 2024;
Published: 03 September 2024.
Edited by:
Tao Wang, Northwestern Polytechnical University, ChinaReviewed by:
Junwei Han, Harbin Medical University, ChinaMassimo Tusconi, University of Cagliari, Italy
Copyright © 2024 Zheng, Zhang, Zhang, Chen and Ji. This is an open-access article distributed under the terms of the Creative Commons Attribution License (CC BY). The use, distribution or reproduction in other forums is permitted, provided the original author(s) and the copyright owner(s) are credited and that the original publication in this journal is cited, in accordance with accepted academic practice. No use, distribution or reproduction is permitted which does not comply with these terms.
*Correspondence: Erni Ji, 38346840@qq.com; Xiabing Zheng, 824381788@qq.com
†These authors have contributed equally to this work and share first authorship