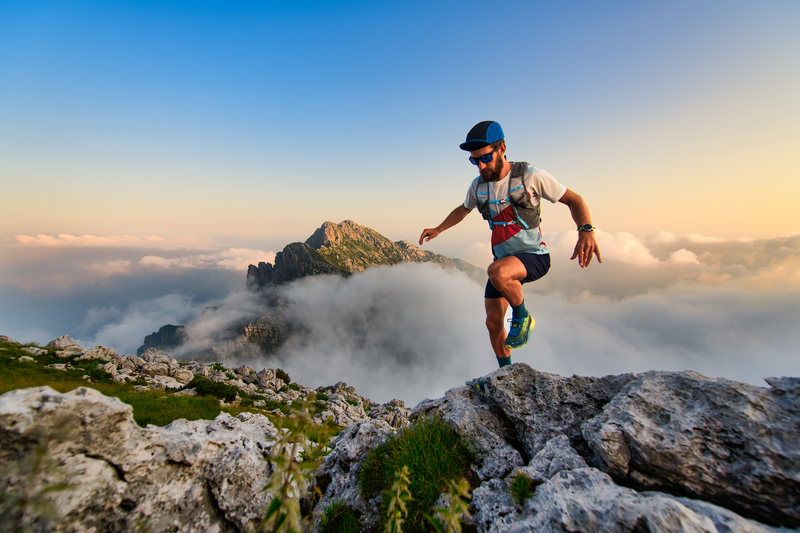
95% of researchers rate our articles as excellent or good
Learn more about the work of our research integrity team to safeguard the quality of each article we publish.
Find out more
HYPOTHESIS AND THEORY article
Front. Psychiatry , 03 September 2024
Sec. Digital Mental Health
Volume 15 - 2024 | https://doi.org/10.3389/fpsyt.2024.1382726
Mental health disorders affect a substantial portion of the global population. Despite preferences for psychotherapy, access remains limited due to various barriers. Digital mental health interventions (DMHIs) have emerged to increase accessibility, yet engagement and treatment completion rates are concerning. Evidence across healthcare where some degree of self-management is required show that treatment engagement is negatively influenced by contextual complexity. This article examines the non-random factors influencing patient engagement in digital and face-to-face psychological therapies. It reviews established models and introduces an adapted version of the Cumulative Complexity Model (CuCoM) as a framework for understanding engagement in the context of digital mental health. Theoretical models like the Fogg Behavior Model, Persuasive System Design, Self-Determination Theory, and Supportive Accountability aim to explain disengagement. However, none adequately consider these broader contextual factors and their complex interactions with personal characteristics, intervention requirements and technology features. We expand on these models by proposing an application of CuCoM’s application in mental health and digital contexts (known as DiCuCoM), focusing on the interplay between patient burden, personal capacity, and treatment demands. Standardized DMHIs often fail to consider individual variations in burden and capacity, leading to engagement variation. DiCuCoM highlights the need for balancing patient workload with capacity to improve engagement. Factors such as life demands, burden of treatment, and personal capacity are examined for their influence on treatment adherence. The article proposes a person-centered approach to treatment, informed by models like CuCoM and Minimally Disruptive Medicine, emphasizing the need for mental healthcare systems to acknowledge and address the unique burdens and capacities of individuals. Strategies for enhancing engagement include assessing personal capacity, reducing treatment burden, and utilizing technology to predict and respond to disengagement. New interventions informed by such models could lead to better engagement and ultimately better outcomes.
Mental ill health adversely affects significant numbers of people worldwide (1), 1 in 5 in any given year (2). Recent data from Australia indicates that 21.5% of the population and 38.8% of young people aged 16-24 years experienced a mental disorder in the previous 12-months. Of these people, 45.1% saw a health professional, 21.3% saw a psychologist and 4.8% accessed a digital mental health service (3). Biological and psychological treatments form the main basis for mental health treatment, but their coverage and ability to substantially reduce disease burden is low (4). While the majority of patients prefer psychotherapy over medication (5), access to these treatments is restrictive due to cost, distance and availability of trained therapists (4). The advent of the internet has helped improve access to psychological treatments (6), overcoming barriers to traditional service provision such as distance, availability, and stigma (7).
Despite these advances, sustained engagement and completion of digital psychological therapy remains a challenge for many patients, where on average, 38.7% do not complete the assigned treatment (8). In comparison, a large scale meta-analysis of engagement in face-to-face settings showed disengagement or dropout rates vary from 17.8% to 37.6% (9). A challenge for the field, however, has been the various definitions and measurement of engagement in both digital or remote settings (10), and face-to-face settings (9). In the digital context, engagement has been defined variously. Within DMHI, engagement is primarily defined by user behaviors such as uptake, sustained use and adherence to the intervention at the intended frequency or duration (11). More specific definitions include the users level of attention, interest and their temporal, emotional and cognitive investment, and include a range of broad actions, including logging in or reading therapeutic content, responding to notifications, and engaging in off-line behavioral change such as behavioral activation (12, 13).
Notwithstanding precise definitions, treatment engagement is critical, as it has also been shown to be a vital mediator and moderator of treatment particularly the quality of that engagement as it relates to the use of specific therapeutic activities that translate to clinical outcomes (14). While engagement with DMHI mediates outcome, various factors have also been shown be associated with engagement itself. Regarding patient or user characteristics, there appear to be common factors associated with disengagement that apply to both digital and face-to-face formats, including lower age, male gender, lower educational status, lower therapist support, among others (9, 15–17). Characteristics associated with engagement are most often reported cross-sectionally at the group level, obscuring the complex and dynamic interactions between these variables over time. Furthermore, studies vary in the number of contextual factors they measure, and rarely capture in the one study the full range of factors known to be associated with psychological treatment disengagement.
While low engagement and engagement variability in both digital and face-to-face mental health is well documented, theories or specific mechanisms that drive this variability has generally been under-researched. In the digital context, various models and theories have been put forward to explain engagement. Some of the prominent models and theories are outlined below.
The Fogg Behavior Model (FBM) provides a structured framework for understanding human behavior in the context of technology use and digital engagement (18). The model aims to support behavior change through persuasive design, and asserts that behavior is a product of three factors: motivation, ability, and triggers, and that for a person to perform a target behavior, they must be sufficiently motivated, have the ability to perform the behavior, and be triggered to perform the behavior (18). This model is particularly useful in the design of digital health technologies, where engaging users effectively requires understanding and influencing their motivations, enhancing their ability to use the technology often by making the technology easy to use, and providing timely and appropriate prompts or reminders. Persuasive system design (PSD) builds upon FBM to describe technology design techniques that can motivate behavior change and user engagement, comprising of 28 persuasive strategies grouped into four categories—primary task support, dialogue support, system credibility support, and social support (19). The evidence on how effective these approached are in improving engagement is limited. In one recent review, a negative association between PSD features and engagement (as measured by completion rate) was observed (20). Another found mixed evidence that a specific strategy or group of strategies is relatively more efficacious in promoting engagement than others (21).
Self Determination Theory (SDT) is one of the most prominent and empirically supported theories of human motivation that has demonstrated efficacy in predicting motivated behavior in multiple contexts and populations and for a variety of health behaviors such as physical activity, healthy eating, and smoking cessation (22). The quality of motivation is influenced by the extent to which individuals experience support for three basic psychological needs: autonomy, competence, and relatedness. Therefore, behaviors or messages from agents that support the satisfaction of these needs are likely to promote autonomous motivation and sustained behavior change within the individual, while those that do not may undermine autonomous motivation and lead to negative outcomes, such as disengagement or poor adherence.
In acknowledging the value of support in improving engagement and adherence with psychological interventions, Mohr and colleagues constructed the Supportive Accountability (SA) model (23), which is particularly relevant in the context of remote interventions where there is limited direct human interaction. Support emphasizes the encouragement, guidance and feedback from a trained coach or therapist. Accountability refers to the user justifying their actions or inactions to another person such as the coach or therapist. Both SDT and SA emphasize the importance of interpersonal interactions and relationships on motivation and engagement.
The above theories and models are widely used and referenced when developing digital interventions, though each when used on its own has limitations when applied to the use of digital interventions to treat mental ill health. For example, in their extensive review of engagement with DMHI, Borghouts and colleagues (17) coded 3 categories of barriers and facilitators: 1. user characteristics, including demographics such as age and education, personality traits, mental health status and severity, beliefs, technology experience and skills and life integration, 2. program characteristics including type of content, perceived fit, perceived usefulness, level of guidance or support, social connectedness, perceived impact or effectiveness of the intervention, and 3. technology and environment characteristics which include technology related factors, technical issues, usability and delivery platform, privacy and confidentiality, social influence by external others, and implementation factors. Based on this evidence, it’s clear that a more comprehensive and holistic models of digital psychological treatment engagement are required. Models that consider the complex interaction between personal capacity and the burdensomeness of the technologies and interventions themselves. For example, each of the above models do not adequately explain the complex interacting relationship between the personal resources of the patient/user, the burden of their illness, the burden of their current life circumstance and the burden placed upon them by the DMHI (including both the technology and the intervention itself). In the following section we outline an approach to conceptualizing treatment engagement and adherence used more widely in chronic disease research that has direct relevance for digital psychological interventions.
The cumulative complexity model (CuCoM) (24) was developed in the context of multimorbidity and chronic conditions. It is a person-centered functional complexity model that emphasizes the interplay between a patient’s workload of demands (encompassing the burdens associated with doing the ‘work’ or treatment and the other contextual burdens operating in the person’s day-to-day life) and their capacity (comprising the capacity reducing impact of the illness itself as well as personal resource scarcity) to manage this workload (Figure 1). While excessive workload or low capacity in isolation may contribute to poor access or nonadherence to an intervention, CuCoM argues that it is the imbalance between the two which is the primary driver poor engagement and subsequent poor outcomes (24). Over time, especially if treatments are ineffective, the burden of illness (BOI) and the burden of treatment (BOT) act as feedback loops, further eroding capacity and adding additional workload and demands, resulting in a cumulative cycle of patient complexity (24). CuCoM has gained research attention and clinical application in relation to chronic disease management (25–29), but has yet to be applied in mental health or digital mental health contexts. The following section outlines the core components of an adapted version of the CuCoM, the Digital Cumulative Complexity Model (DiCuCoM) as they each relate to digital psychological interventions. Table 1 outlines the key similarities and differences between DiCuCoM and other the other prominent theories and models of digital mental health user engagement outlined above.
Figure 1. The digital cumulative complexity model (DiCuCoM) adapted for digital mental health interventions, highlighting the isolated and cumulative impact of workload and capacity as they interact over time.
Table 1. Similarities and difference of the DiCuCoM with prominent other theories of models of DMHI user engagement.
Being a patient can be hard work (30–32). In addition to the burden of illness (BOI) experienced by the individual (expanded on below), individuals may experience concurrent burdens in the ordinary demands of daily living (life demands). When seeking treatment for a mental health problem, there is additional burden or work placed on the patient (or a user of a digital intervention). This is known as the Burden of Treatment (BOT). Both impact complexity through their toll on the persons time, effort, and attention (24).
There is evidence that highlights the relationship between high burden life demands and engagement in DMHI (33). Work commitments, family problems, moving house (34), financial concerns or ‘life chaos’ (defined as numerous commitments or unstable living arrangements) (35), being unemployed (36), and experiencing a greater number of these psychosocial stressors in daily life (16) are all associated with reduced engagement.
In addition to whatever burdens an individual experiences in their daily life and from their illness, DMHI themselves require work and therefore carry subjective burden. Compared to face-to-face treatments, DMHI have been highly successful at reducing BOT, saving time and financial resources to physically attend appointments for example. However other treatment burdens remain, both in navigating the technology itself (digital burden) and the work of engaging in cognitive and behavioral change (therapy burden). Examples include reading lengthy and cognitively demanding content (33), practicing cognitive or behavioral skills that trigger negative emotional states (33), completing numerous or lengthy questionnaires, setting time aside to practice skills (therapy burden) (37); and experiencing technical issues (38), poor usability (37), difficulty logging on, difficulty navigating the platform, content that is delivered in non-preferred formats (audio-visual versus text alone), content that is difficult to read (digital burden) (17, 39, 40).
Capacity can be thought of as the sum-total of resources and abilities that a patient can draw on to access care, use care, and enact self-care. Every structure or system (including patients) has a maximum performable workload that is determined by its unique capacity (32). Capacity is affected by the availability or scarcity of personal resources, be they intrinsic (such as physical, mental, personal attitudes and beliefs, personality, intelligence, literacy) or extrinsic (such as socio-economic resources, social supports, education level, income, amount of free time). Furthermore, capacity is negatively affected by the burdens associated with their disease or illness itself (BOI). The interaction between personal resources and BOI affect the person’s ability or readiness to do the ‘work’ of treatment (24). Importantly, capacity varies between individuals; some persevere despite tremendous workloads; others falter even when relatively unencumbered (24). According to Shippe et al. (24), measurable attributes of capacity include the following: 1. amount/magnitude (e.g., greater/lesser symptoms, finances, or social support); 2. controllability (some factors, such as literacy, are more responsive to personal efforts than others, such as pain); 3. extensiveness or scope of impact (symptoms may have limited or widespread effects on functioning).
In the context of DMHI, personal capacity has consistently been directly or indirectly implicated in engagement. Indirectly, personal or demographic factors such as younger age (16, 41), being male (42–44) (17, 39), lower education level (15, 17). These factors have an indirect effect, partly because factors such as age are correlates to other abilities that affect capacity, such as underdeveloped executive functioning skills such as self-monitoring and organization (45), and increased disease and lifestyle risk factor burdens that accompany adolescence (46, 47). Direct factors include possessing conscientious personality traits (48, 49), possessing negative personal beliefs about treatment including stigma (35) (17, 50), possessing low rates of mental health literacy (17, 51); having little free time (17, 52). Socioeconomic status may also be an indirect indicator of capacity as it is defined as a rough measure of the relative material resources or nonmaterial resources a person may have, including education, occupational prestige, and neighborhood quality (53). As a correlate of reduced capacity, SES has been shown to be associated with improved physical health and lower rates of heart disease, stroke, cancer, diabetes, and many other serious illnesses as well as lifespan (54). Mental health also increases with SES, with progressively less depression, anxiety, and psychosis at higher levels of SES (55–57).
Burden of illness (BOI) factors associated with reduced DMHI engagement include higher symptom severity or complexity (16, 17, 41, 43), physical illness (52), and the lack of motivation inherent in conditions such as depression (52). The stage or severity of illness in young adults (58, 59) has also been shown to negatively affect treatment engagement in face-to-face services, especially in those with greater symptom severity, functional impairment and ambiguous syndromes (60, 61). While not specifically examined to date, it is possible that different symptoms or symptom combinations may also have distinctive negative effects on personal capacity and subsequently treatment engagement. For example, the symptom of amotivation may reduce the capacity of an individual to do behavioral activation tasks, hyperactivity may make it difficult to do mindfulness activities, rigid and fixed beliefs may make cognitive challenging difficult and so on.
The core assertion of the model is that where there is an imbalance between the person’s workload and their capacity to meet those demands, poor engagement and subsequently poor outcomes ensue (24). Collectively, a person’s capacity may or may not reach that required to meet the prerequisites of sustained engagement in any psychological treatment. When workload exceeds capacity, people feel the effects of treatment burden and this can lead to de-prioritization of various aspects of care and ultimately result in poor fidelity to treatment programs and to treatment failures (28, 30, 62–64). An example is reading content in a DMHI. The act of reading is considered work and people vary in their capacity to complete this work. Those with high reading ability will not experience this work as burdensome. Those with reading difficulties will find that the reading workload exceeds their reading capacity and will find this burdensome, leading to disengagement. Furthermore, people experiencing this imbalance may disengage or clinically deteriorate, leading service providers to provide more care, often unintentionally fueling burden and creating a perpetuating cycle of increasing complexity. Approaches like stepped care models or adaptive interventions increase the intensity and nature of treatment in response poor engagement or poor treatment outcome (65) but in doing so, may increase the burden of treatment in a potentially already ‘overloaded’ person. This is why most stepped care models recommend greater levels of professional support as a means to increase capacity in the face of increase illness and treatment burden (65).
Given these complexities, multiple interactions may shape patient demands, capacity, and the interplay between them (Figure 1). Even though there is significant evidence of both burden and capacity related factors in isolation influencing engagement, limited research has been conducted on these complex interactions over time. Yet it is their interaction at the individual level which is most likely to be predictive of engagement and subsequent outcome, which may explain the variability in findings when these factors are studied in isolation. The goal is to balance the recommended treatment workload with the unique capacity of the individual to avoid overload and to adapt this balance over the course of care. Engagement research, however, rarely examines how these complex combinations interact, which inhibits our ability to design, test and deliver more appropriate interventions.
The workload-capacity imbalance is most apparent in standardized DMHI (and other psychological treatments for that matter), where all users receive the same intervention regardless of their capacity. While useful for research standardization and fidelity, these ‘one-size-fits-all’ approaches disregard individual variation in illness burden and personal resources. Treatment demands are fixed and usually specify a set amount of time to spend, a set amount to read, what tasks to complete, and the frequency and length of support appointments to participate in. The result as outlined above is poor engagement for large numbers of participants in these standardized programs.
Developed alongside the CuCoM, the Minimally Disruptive Medicine (MDM) model (30) advocates for an approach to healthcare that seeks to tailor treatment to the realities of patients’ lives, minimizing the burden of treatment while maximizing patient capacity. The core principle of MDM is to carefully calibrate the treatment and broader healthcare workload imposed on patients, ensuring it does not overwhelm their capacity. MDM aims to achieve a balance by considering the demands of treatment in the context of the patient’s life and responsibilities, striving for the best clinical outcomes with the least possible impact on a patient’s daily functioning and quality of life (66). This model is person-centered, recognizing the importance of patient goals and preferences, and seeking to avoid the treatment or health system-related exacerbation of health issues due to overwhelming intervention and management regimens (32). For example, self-managing a chronic disease is estimated to demand two hours of a patient’s attention and effort per day (67).
There is an urgent need to develop new psychological treatments and models to minimize burden and ensure engagement in mental health interventions. Models that are more nuanced, context-sensitive, and responsive to individual variation in capacities and burdens. This approach aligns with the ethos of MDM by ensuring that interventions, be they face-to-face or digital, do not inadvertently exacerbate disparities by imposing a one-size-fits-all treatment regime that ignores the complexities of patients’ lives. Figure 2 outlines a person (user)-centered approach to providing psychological interventions to patients that considers burden, capacity in addition to traditional disease/problem assessment and intervention.
Figure 2. A model for addressing patient complexity to facilitate sustained engagement in psychological interventions.
Next, we outline two strategies that can be used to provide and develop more personalized psychological interventions derived from the DiCuCoM.
Shared decision making and informed consent are critical before commencing any health intervention (68) following an adequately comprehensive assessment of the user at entry (Figure 2). Users should have a complete understanding of the potential benefits, burdens, demands, harms and risks of starting a proposed intervention. These kinds of discussions with MHPs are valued by users of DMHI (69). Indeed, a recent meta-analysis showed that synchronous contact before the digital intervention commenced significantly improved intervention adherence and outcome (70). For example, if the intervention requires an 8-week enrolment, weekly reading, regularly questionnaire completion and regular telephone contact with the clinician, these requirements should be stated clearly up front, and the user themselves will decide as to their capacity to engage in the suggested work to obtain their desired goal. Just like other mental health interventions, DMHIs are not suitable for all. It is important that the intended user’s assessed capacity is balanced against the fixed burden of the intended intervention, and that this is openly and transparently discussed and consented to prior to commencement. If this were to occur, less users might start these interventions, but conceivably more would engage. At present, we have many users who fail to complete an intervention, which either maintains or exacerbates their burden of illness, and can lead to ‘failure demand’, where failing to adequately treat the condition the first time creates later downstream demand on services (64, 71).
Providing additional professional support has been associated with improved engagement (39). A meta-analysis of 40 studies with a total of 7313 participants found an overall completion rate of 43%, with completion rates increasing with greater levels of support: 26% without support, 62% administrative support only, and 72% for therapeutic support (40). Studies have also explored the role of social support systems in psychological treatments, including peer support groups, community-based programs, and social networking (72, 73). These interventions aim to provide emotional and practical support, reducing feelings of isolation, shape more positive attitudes toward intervention and provide additional encouragement or motivation to do the work of therapy (74). Providing additional social supports may also be warranted, such as access to career consultants or services (75), information or direction to services that can assist with financial counselling, domestic violence, housing and accommodation. Digital Navigators are becoming increasingly popular as a way to support MHP and users with digital literacy, workflow integration (for clinicians) and engagement, although further work is required to standardize the scope of these roles (76).
As outlined, standardized DMHI have an inequitable effect on user engagement, simply because there is significant variability in the degree of contextual burden and internal capacity across users. To increase DMHI engagement and subsequent outcome, future research should explore ways of reducing treatment burden and offering supportive technologies that make treatment easier to access and complete. In a recent systematic review, young people reported that interventions are more likely to be used if they are low effort, fun, relaxing, easy to navigate, and fit into daily routine (39).
‘Effort-Optimized Interventions’ have been recommended as a way to reduce the effort required to engage in therapeutic activities, among other things, such as setting graded tasks and setting dynamically tailored tasks (77). Further, they argue that commerce and social media sectors use A/B testing paradigms (randomized controlled experiments where two versions of a variable such as a feature are compared) to evaluate small platform changes that lead to large improvements in engagement, and that similar approaches can be used in reducing effort in DMHIs (77). In this way creating a wider range of interventions that vary by effort, burden, or difficulty, and testing them for engagement and effectiveness, will provide users with more choice in selecting interventions that fit them in the context of their personal capacities. While developing these new interventions, users can rate and provide feedback on the level of difficulty or workload experienced for the whole DMHI, over and above technology usability alone. Researchers could identify gaps in their existing interventions and develop new ways of delivering them that carry less treatment burden. Recent examples include reducing word counts in iCBT programs (78), and single-module, ultra-brief versions of much longer programs (79), both proved to be as effective as their longer-form counterparts. ‘Just in Time Adaptive Interventions’ are another example of a new DMHI delivery technique that requires little user effort, and have been shown to be engaging and effective (80). Maintaining effectiveness while reducing effort is crucial. Knowing which are the core components that absolutely must be retained will be of critical research focus. Precision psychological interventions (81, 82) aim to differentiate the essential and non-essential components of treatment and pare back interventions to the core components when there is imbalance, which may result highly personalized interventions.
Research into the development of enhanced measurement-based care and routine outcome monitoring that captures metrics beyond symptoms (including capacity and burden metrics) is another avenue through which engagement might be improved. Disengagement is often determined in retrospect, after a DMHI was due to be completed. We do not yet have systems that can predict disengagement in near or real time, which reduces our ability to respond and intervene early to avoid treatment failure. Regularly measuring and feeding-back user progress to clinicians and users themselves, might ensure treatments are more efficiently targeted, potentially reducing unnecessary intervention, and tailoring the therapy more closely to user needs. Further research using machine learning or artificial intelligence might explore a broader range of burden, capacity and maintenance factors and the nature of their relationship with uptake, engagement, and outcome, which may inspire the development of better targeted, more precise, and more personalized interventions. These strategies can be offered to patients prospectively from the point at which they access the DMHI and may help identify subgroups of patients who are less likely to engage in treatment, based on characteristics they report at assessment, to facilitate a discussion about the appropriateness of the specific intervention.
This article proposes a person-centered model of patient complexity in which clinical and social factors accumulate and interact to shape uptake, engagement, and adherence to DMHIs. Integrating prior literature in other domains of healthcare, and complementary to existing models of DMHI engagement, the DiCuCoM emphasizes individual-level functions whereby complicating factors of workload, capacity, and treatment and illness burdens influence engagement. The focus on function facilitates a cohesive, generalizable framework with practical applicability to digital and other psychological interventions. The model also guides improvements and advances DMHI design and practice. Overall, the DiCuCoM is intended to stimulate innovations in research and practice that respect the clinical importance of workload-capacity imbalances and its effect on engagement equity. By deliberately considering the burden of treatment, DMHIs can evolve to mitigate systemic inequities, thereby supporting more equitable access to and engagement with mental health care across diverse user groups.
The original contributions presented in the study are included in the article/supplementary material. Further inquiries can be directed to the corresponding author.
SC: Conceptualization, Writing – original draft, Writing – review & editing. MA-J: Writing – review & editing.
The author(s) declare that no financial support was received for the research, authorship, and/or publication of this article.
The authors declare that the research was conducted in the absence of any commercial or financial relationships that could be construed as a potential conflict of interest.
All claims expressed in this article are solely those of the authors and do not necessarily represent those of their affiliated organizations, or those of the publisher, the editors and the reviewers. Any product that may be evaluated in this article, or claim that may be made by its manufacturer, is not guaranteed or endorsed by the publisher.
1. Vos T, Abajobir AA, Abate KH, Abbafati C, Abbas KM, Abd-Allah F, et al. Global, regional, and national incidence, prevalence, and years lived with disability for 328 diseases and injuries for 195 countries, 1990–2016: a systematic analysis for the Global Burden of Disease Study 2016. Lancet. (2017) 390:1211–59. doi: 10.1016/S0140-6736(17)32154-2
2. Steel Z, Marnane C, Iranpour C, Chey T, Jackson JW, Patel V, et al. The global prevalence of common mental disorders: a systematic review and meta-analysis 1980–2013. Int J Epidemiol. (2014) 43:476–93. doi: 10.1093/ije/dyu038
3. Australian Bureau Statistics. National Study of Mental Health and Wellbeing, Canberra. Australian Bureau of Statistics (2020-2022). Available online at: https://www.abs.gov.au/statistics/health/mental-health/national-study-mental-health-and-wellbeing/2020-2022.
4. Holmes EA, Ghaderi A, Harmer CJ, Ramchandani PG, Cuijpers P, Morrison AP, et al. The Lancet Psychiatry Commission on psychological treatments research in tomorrow’s science. Lancet Psychiatry. (2018) 5:237–86. doi: 10.1016/S2215-0366(17)30513-8
5. McHugh RK, Whitton SW, Peckham AD, Welge JA, Otto MW. Patient preference for psychological vs pharmacologic treatment of psychiatric disorders: a meta-analytic review. J Clin Psychiatry. (2013) 74:13979. doi: 10.4088/JCP.12r07757
6. Andersson G, Titov N, Dear BF, Rozental A, Carlbring P. Internet-delivered psychological treatments: from innovation to implementation. World Psychiatry. (2019) 18:20–8. doi: 10.1002/wps.20610
7. Andersson G, Titov N. Advantages and limitations of Internet-based interventions for common mental disorders. World Psychiatry. (2014) 13:4–11. doi: 10.1002/wps.v13.1
8. Etzelmueller A, Vis C, Karyotaki E, Baumeister H, Titov N, Berking M, et al. Effects of internet-based cognitive behavioral therapy in routine care for adults in treatment for depression and anxiety: Systematic review and meta-analysis. J Med Internet Res. (2020) 22:e18100. doi: 10.2196/18100
9. Swift JK, Greenberg RP. Premature discontinuation in adult psychotherapy: A meta-analysis. J consulting Clin Psychol. (2012) 80:547. doi: 10.1037/a0028226
10. Torous J, Michalak EE, O’Brien HL. Digital health and engagement—looking behind the measures and methods. JAMA network Open. (2020) 3:e2010918–e. doi: 10.1001/jamanetworkopen.2020.10918
11. Lipschitz JM, Pike CK, Hogan TP, Murphy SA, Burdick KE. The engagement problem: a review of engagement with digital mental health interventions and recommendations for a path forward. Curr Treat Options Psychiatry. (2023) 10:119–35. doi: 10.1007/s40501-023-00297-3
12. Nahum-Shani I, Shaw SD, Carpenter SM, Murphy SA, Yoon C. Engagement in digital interventions. Am Psychol. (2022) 77:836–52. doi: 10.1037/amp0000983
13. Perski O, Blandford A, West R, Michie S. Conceptualising engagement with digital behaviour change interventions: a systematic review using principles from critical interpretive synthesis. Transl Behav Med. (2017) 7:254–67. doi: 10.1007/s13142-016-0453-1
14. Baumel A. Therapeutic activities as a link between program usage and clinical outcomes in digital mental health interventions: a proposed research framework. J Technol Behav Sci. (2022) 7:234–9. doi: 10.1007/s41347-022-00245-7
15. Schmidt ID, Forand NR, Strunk DR. Predictors of dropout in Internet-based cognitive behavioral therapy for depression. Cogn Ther Res. (2019) 43:620–30. doi: 10.1007/s10608-018-9979-5
16. Cross SP, Karin E, Staples LG, Bisby MA, Ryan K, Duke G, et al. Factors associated with treatment uptake, completion, and subsequent symptom improvement in a national digital mental health service. Internet Interv. (2022) 27:100506. doi: 10.1016/j.invent.2022.100506
17. Borghouts J, Eikey E, Mark G, De Leon C, Schueller SM, Schneider M, et al. Barriers to and facilitators of user engagement with digital mental health interventions: systematic review. J Med Internet Res. (2021) 23:e24387. doi: 10.2196/24387
18. Fogg BJ ed. A behavior model for persuasive design. In: Proceedings of the 4th international Conference on Persuasive Technology. Persuasive’09, April 26-29, Claremont, California, USA.
19. Oinas-Kukkonen H, Harjumaa M. Key Issues, Process Model and System Features1. In: Routledge handbook of policy design. New York: Routledge (2018).
20. Wu A, Scult MA, Barnes ED, Betancourt JA, Falk A, Gunning FM. Smartphone apps for depression and anxiety: a systematic review and meta-analysis of techniques to increase engagement. NPJ Digital Med. (2021) 4:20. doi: 10.1038/s41746-021-00386-8
21. Gan DZ, McGillivray L, Larsen ME, Christensen H, Torok M. Technology-supported strategies for promoting user engagement with digital mental health interventions: A systematic review. Digital Health. (2022) 8:20552076221098268. doi: 10.1177/20552076221098268
22. Ryan RM, Deci EL. Self-determination theory: Basic psychological needs in motivation, development, and wellness. New York: Guilford Publications (2017).
23. Mohr D, Cuijpers P, Lehman K. Supportive accountability: a model for providing human support to enhance adherence to eHealth interventions. J Med Internet Res. (2011) 13:e1602. doi: 10.2196/jmir.1602
24. Shippee ND, Shah ND, May CR, Mair FS, Montori VM. Cumulative complexity: a functional, patient-centered model of patient complexity can improve research and practice. J Clin Epidemiol. (2012) 65:1041–51. doi: 10.1016/j.jclinepi.2012.05.005
25. Demain S, Goncalves A-C, Areia C, Oliveira R, Marcos AJ, Marques A, et al. Living with, managing and minimising treatment burden in long term conditions: a systematic review of qualitative research. PloS One. (2015) 10:e0125457. doi: 10.1371/journal.pone.0125457
26. Skou ST, Mair FS, Fortin M, Guthrie B, Nunes BP, Miranda JJ, et al. Multimorbidity. Nat Rev Dis Primers. (2022) 8:48. doi: 10.1038/s41572-022-00376-4
27. May CR, Eton DT, Boehmer K, Gallacher K, Hunt K, MacDonald S, et al. Rethinking the patient: using Burden of Treatment Theory to understand the changing dynamics of illness. BMC Health Serv Res. (2014) 14:1–11. doi: 10.1186/1472-6963-14-281
28. Mair FS, May CR. Thinking about the burden of treatment. Br Med J. (2014) 349: 1–2. doi: 10.1136/bmj.g6680
29. Rosbach M, Andersen JS. Patient-experienced burden of treatment in patients with multimorbidity–a systematic review of qualitative data. PloS One. (2017) 12:e0179916. doi: 10.1371/journal.pone.0179916
30. May C, Montori VM, Mair FS. We need minimally disruptive medicine. Bmj. (2009) 339:b2803. doi: 10.1136/bmj.b2803
31. Institute of Medicine (IOM). Crossing the Quality Chasm: A New Health System for the Twenty-first Century. Washington: National Academy Press (2001).
32. Leppin AL, Montori VM, Gionfriddo MR. Minimally disruptive medicine: A pragmatically comprehensive model for delivering care to patients with multiple chronic conditions. Healthcare. (2015) 3:50–63. doi: 10.3390/healthcare3010050
33. Johansson O, Michel T, Andersson G, Paxling B. Experiences of non-adherence to Internet-delivered cognitive behavior therapy: A qualitative study. Internet Interventions. (2015) 2:137–42. doi: 10.1016/j.invent.2015.02.006
34. Proudfoot J, Goldberg D, Mann A, Everitt B, Marks I, Gray J. Computerized, interactive, multimedia cognitive-behavioural program for anxiety and depression in general practice. psychol Med. (2003) 33:217–27. doi: 10.1017/S0033291702007225
35. Moskalenko MY, Hadjistavropoulos HD, Katapally TR. Barriers to patient interest in Internet-based cognitive behavioral therapy: Informing e-health policies through quantitative analysis. Health Policy Technol. (2020) 9:139–45. doi: 10.1016/j.hlpt.2020.04.004
36. Gunn J, Cameron J, Densley K, Davidson S, Fletcher S, Palmer V, et al. Uptake of mental health websites in primary care: Insights from an Australian longitudinal cohort study of depression. Patient Educ Couns. (2018) 101:105–12. doi: 10.1016/j.pec.2017.07.008
37. Cerga-Pashoja A, Doukani A, Gega L, Walke J, Araya R. Added value or added burden? A qualitative investigation of blending internet self-help with face-to-face cognitive behaviour therapy for depression. Psychother Res. (2020) 30:998–1010. doi: 10.1080/10503307.2020.1720932
38. Gerhards S, Abma T, Arntz A, De Graaf L, Evers S, Huibers M, et al. Improving adherence and effectiveness of computerised cognitive behavioural therapy without support for depression: a qualitative study on patient experiences. J Affect Disord. (2011) 129:117–25. doi: 10.1016/j.jad.2010.09.012
39. Garrido S, Millington C, Cheers D, Boydell K, Schubert E, Meade T, et al. What works and what doesn’t work? A systematic review of digital mental health interventions for depression and anxiety in young people. Front Psychiatry. (2019) 10. doi: 10.3389/fpsyt.2019.00759
40. Richards D, Richardson T. Computer-based psychological treatments for depression: a systematic review and meta-analysis. Clin Psychol Rev. (2012) 32:329–42. doi: 10.1016/j.cpr.2012.02.004
41. Edmonds M, Hadjistavropoulos H, Schneider L, Dear B, Titov N. Who benefits most from therapist-assisted internet-delivered cognitive behaviour therapy in clinical practice? Predictors of symptom change and dropout. J Anxiety Disord. (2018) 54:24–32. doi: 10.1016/j.janxdis.2018.01.003
42. Kramer J, Conijn B, Oijevaar P, Riper H. Effectiveness of a web-based solution-focused brief chat treatment for depressed adolescents and young adults: randomized controlled trial. J Med Internet Res. (2014) 16:e3261. doi: 10.2196/jmir.3261
43. Neil A, Batterham P, Christensen H, Bennett K, Griffiths K. Predictors of adherence by adolescents to a cognitive behavior therapy website in school and community-based settings. J Med Internet Res. (2009) 11:e1050. doi: 10.2196/jmir.1050
44. van der Zanden R, Kramer J, Gerrits R, Cuijpers P. Effectiveness of an online group course for depression in adolescents and young adults: a randomized trial. J Med Internet Res. (2012) 14:e2033. doi: 10.2196/jmir.2033
45. Anderson VA, Anderson P, Northam E, Jacobs R, Catroppa C. Development of executive functions through late childhood and adolescence in an Australian sample. Dev Neuropsychol. (2001) 20:385–406. doi: 10.1207/S15326942DN2001_5
46. Mokdad AH, Forouzanfar MH, Daoud F, Mokdad AA, El Bcheraoui C, Moradi-Lakeh M, et al. Global burden of diseases, injuries, and risk factors for young people’s health during 1990–2013: a systematic analysis for the Global Burden of Disease Study 2013. Lancet. (2016) 387:2383–401. doi: 10.1016/S0140-6736(16)00648-6
47. Patel V, Flisher AJ, Hetrick S, McGorry P. Mental health of young people: a global public-health challenge. Lancet. (2007) 369:1302–13. doi: 10.1016/S0140-6736(07)60368-7
48. Cillessen L, van de Ven MO, Compen FR, Bisseling EM, van der Lee ML, Speckens AE. Predictors and effects of usage of an online mindfulness intervention for distressed cancer patients: Usability study. J Med Internet Res. (2020) 22:e17526. doi: 10.2196/17526
49. Gulliver A, Calear AL, Sunderland M, Kay-Lambkin F, Farrer LM, Batterham PJ. Predictors of acceptability and engagement in a self-guided online program for depression and anxiety. Internet Interventions. (2021) 25:100400. doi: 10.1016/j.invent.2021.100400
50. Clement S, Schauman O, Graham T, Maggioni F, Evans-Lacko S, Bezborodovs N, et al. What is the impact of mental health-related stigma on help-seeking? A systematic review of quantitative and qualitative studies. psychol Med. (2015) 45:11–27. doi: 10.1017/S0033291714000129
51. Coles ME, Ravid A, Gibb B, George-Denn D, Bronstein LR, McLeod S. Adolescent mental health literacy: Young people’s knowledge of depression and social anxiety disorder. J Adolesc Health. (2016) 58:57–62. doi: 10.1016/j.jadohealth.2015.09.017
52. Klein B, Meyer D, Austin DW, Kyrios M. Anxiety online—a virtual clinic: preliminary outcomes following completion of five fully automated treatment programs for anxiety disorders and symptoms. J Med Internet Res. (2011) 13:e1918. doi: 10.2196/jmir.1918
53. Farah MJ. The neuroscience of socioeconomic status: Correlates, causes, and consequences. Neuron. (2017) 96:56–71. doi: 10.1016/j.neuron.2017.08.034
54. Adler NE, Stewart J. Health disparities across the lifespan: meaning, methods, and mechanisms. Ann New York Acad Sci. (2010) 1186:5–23. doi: 10.1111/j.1749-6632.2009.05337.x
55. Kessler RC, Berglund P, Demler O, Jin R, Merikangas KR, Walters EE. Lifetime prevalence and age-of-onset distributions of DSM-IV disorders in the National Comorbidity Survey Replication. Arch Gen Psychiatry. (2005) 62:593–602. doi: 10.1001/archpsyc.62.6.593
56. Lorant V, Deliège D, Eaton W, Robert A, Philippot P, Ansseau M. Socioeconomic inequalities in depression: a meta-analysis. Am J Epidemiol. (2003) 157:98–112. doi: 10.1093/aje/kwf182
57. McLaughlin KA, Costello EJ, Leblanc W, Sampson NA, Kessler RC. Socioeconomic status and adolescent mental disorders. Am J Public Health. (2012) 102:1742–50. doi: 10.2105/AJPH.2011.300477
58. Cross SP, Hermens DF, Scott EM, Ottavio A, McGorry PD, Hickie IB. A clinical staging model for early intervention youth mental health services. Psychiatr Serv. (2014) 65:939–43. doi: 10.1176/appi.ps.201300221
59. Shah JL, Scott J, McGorry PD, Cross SPM, Keshavan MS, Nelson B, et al. Transdiagnostic clinical staging in youth mental health: a first international consensus statement. World Psychiatry. (2020) 19:233–42. doi: 10.1002/wps.20745
60. Cross SPM, Hermens DF, Scott J, Salvador-Carulla L, Hickie IB. Differential impact of current diagnosis and clinical stage on attendance at a youth mental health service. Early Interv Psychiatry. (2017) 11:255–62. doi: 10.1111/eip.12319
61. Cross SP, Hermens DF, Hickie IB. Treatment patterns and short-term outcomes in an early intervention youth mental health service. Early Interv Psychiatry. (2016) 10:88–97. doi: 10.1111/eip.12191
62. Heckman BW, Mathew AR, Carpenter MJ. Treatment burden and treatment fatigue as barriers to health. Curr Opin Psychol. (2015) 5:31–6. doi: 10.1016/j.copsyc.2015.03.004
63. Tran V-T, Barnes C, Montori VM, Falissard B, Ravaud P. Taxonomy of the burden of treatment: a multi-country web-based qualitative study of patients with chronic conditions. BMC Med. (2015) 13:115. doi: 10.1186/s12916-015-0356-x
64. Rock D, Cross SP. Regional planning for meaningful person-centred care in mental health: context is the signal not the noise. Epidemiol Psychiatr Sci. (2020) 29:e104. doi: 10.1017/S2045796020000153
65. Cross SP, Hickie I. Transdiagnostic stepped care in mental health. Public Health Res Pract. (2017) 27:1–5. doi: 10.17061/phrp2721712
66. Boehmer KR, Shippee ND, Beebe TJ, Montori VM. Pursuing minimally disruptive medicine: disruption from illness and health care-related demands is correlated with patient capacity. J Clin Epidemiol. (2016) 74:227–36. doi: 10.1016/j.jclinepi.2016.01.006
67. Jowsey T, Yen L. Time spent on health related activities associated with chronic illness: a scoping literature review. BMC Public Health. (2012) 12:1–12. doi: 10.1186/1471-2458-12-1044
68. Slade M. Implementing shared decision making in routine mental health care. World Psychiatry. (2017) 16:146–53. doi: 10.1002/wps.20412
69. Fisher A, Corrigan E, Cross S, Ryan K, Staples L, Tan R, et al. Decision-making about uptake and engagement with digital mental health services: a qualitative exploration of service user perspectives. Clin Psychol. (2023) 28:1–12. doi: 10.1080/13284207.2023.2279657
70. Krieger T, Bur OT, Weber L, Wolf M, Berger T, Watzke B, et al. Human contact in internet-based interventions for depression: A pre-registered replication and meta-analysis of randomized trials. Internet Interventions. (2023) 32:100617. doi: 10.1016/j.invent.2023.100617
72. Ridout B, Campbell A. The use of social networking sites in mental health interventions for young people: systematic review. J Med Internet Res. (2018) 20:e12244. doi: 10.2196/12244
73. Rice S, O’Bree B, Wilson M, McEnery C, Lim MH, Hamilton M, et al. Leveraging the social network for treatment of social anxiety: pilot study of a youth-specific digital intervention with a focus on engagement of young men. Internet Interventions. (2020) 20:100323. doi: 10.1016/j.invent.2020.100323
74. Cross S, Nicholas J, Mangelsdorf S, Valentine L, Baker S, McGorry P, et al. Developing a theory of change for a digital youth mental health service (Moderated online social therapy): mixed methods knowledge synthesis study. JMIR Form Res. (2023) 7:e49846. doi: 10.2196/49846
75. Simmons MB, Nicholas J, Chinnery G, O’Sullivan S, D’Alfonso S, Bendall S, et al. The youth online training and employment system: Study protocol for a randomized controlled trial of an online vocational intervention for young people with mental ill health. Early Intervention Psychiatry. (2021) 15:1602–11. doi: 10.1111/eip.13100
76. Perret S, Alon N, Carpenter-Song E, Myrick K, Thompson K, Li S, et al. Standardising the role of a digital navigator in behavioural health: a systematic review. Lancet Digital Health. (2023) 5:e925–e32. doi: 10.1016/S2589-7500(23)00152-8
77. Baumel A, Muench FJ. Effort-optimized intervention model: framework for building and analyzing digital interventions that require minimal effort for health-related gains. J Med Internet Res. (2021) 23:e24905. doi: 10.2196/24905
78. Karlsson-Good M, Kaldo V, Lundberg L, Kraepelien M, Anthony SA, Holländare F. Increasing the accessibility to internet-based cognitive behavioural therapy for depression: A single-blind randomized controlled trial of condensed versus full-text versions. Internet Interventions. (2023) 34:100678. doi: 10.1016/j.invent.2023.100678
79. Bisby MA, Balakumar T, Scott AJ, Titov N, Dear BF. An online therapist-guided ultra-brief treatment for depression and anxiety: a randomized controlled trial. psychol Med. (2023) 54:1–12. doi: 10.1017/S003329172300260X
80. Bell I, Arnold C, Gilbertson T, D’Alfonso S, Castagnini E, Chen N, et al. A personalized, transdiagnostic smartphone intervention (Mello) targeting repetitive negative thinking in young people with depression and anxiety: pilot randomized controlled trial. J Med Internet Res. (2023) 25:e47860. doi: 10.2196/47860
81. Acarturk C, Cuijpers P, Purgato M, Singh R. Moving beyond a ‘one-size-fits-all’ rationale in global mental health: prospects of a precision psychology paradigm. Epidemiol Psychiatr Sci. (2021) 30:e63. doi: 10.1017/S2045796021000500
Keywords: digital mental health, engagement, treatment burden, patient capacity, minimally disruptive medicine, cumulative complexity model, person-centered care
Citation: Cross SP and Alvarez-Jimenez M (2024) The digital cumulative complexity model: a framework for improving engagement in digital mental health interventions. Front. Psychiatry 15:1382726. doi: 10.3389/fpsyt.2024.1382726
Received: 06 February 2024; Accepted: 14 August 2024;
Published: 03 September 2024.
Edited by:
Michael Patrick Schaub, University of Zurich, SwitzerlandReviewed by:
J. Carola Pérez, University for Development, ChileCopyright © 2024 Cross and Alvarez-Jimenez. This is an open-access article distributed under the terms of the Creative Commons Attribution License (CC BY). The use, distribution or reproduction in other forums is permitted, provided the original author(s) and the copyright owner(s) are credited and that the original publication in this journal is cited, in accordance with accepted academic practice. No use, distribution or reproduction is permitted which does not comply with these terms.
*Correspondence: Shane P. Cross, c2hhbmUuY3Jvc3NAb3J5Z2VuLm9yZy5hdQ==
Disclaimer: All claims expressed in this article are solely those of the authors and do not necessarily represent those of their affiliated organizations, or those of the publisher, the editors and the reviewers. Any product that may be evaluated in this article or claim that may be made by its manufacturer is not guaranteed or endorsed by the publisher.
Research integrity at Frontiers
Learn more about the work of our research integrity team to safeguard the quality of each article we publish.