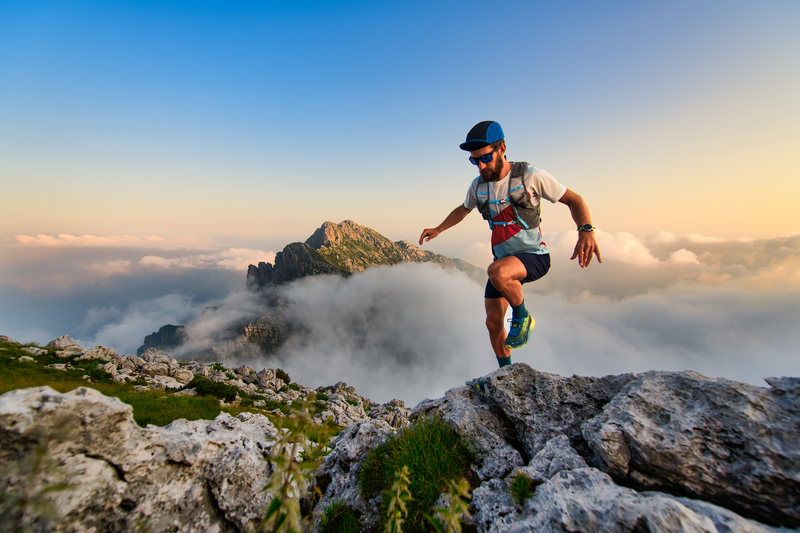
95% of researchers rate our articles as excellent or good
Learn more about the work of our research integrity team to safeguard the quality of each article we publish.
Find out more
ORIGINAL RESEARCH article
Front. Psychiatry , 06 June 2024
Sec. Anxiety and Stress Disorders
Volume 15 - 2024 | https://doi.org/10.3389/fpsyt.2024.1372842
Objective: Although extensive research has explored the link between mental disorders and asthma, the characteristics and patterns of this association are still unclear. Our study aims to examine the genetic causal links between common mental disorders (specifically, anxiety and depression) and asthma.
Methods: We conducted genetic analyses including linkage disequilibrium score regression (LDSC) and bidirectional two-sample Mendelian randomization (MR) analyses, and utilized summary statistics from recent large-scale Genome-Wide Association Studies (GWASs) in European populations, covering sensation of anxiety or depression, anxiety sensation, depression sensation, anxiety disorders, major depression disorder (MDD), and asthma.
Results: LDSC revealed significant genetic correlations among sensation of anxiety or depression, MDD and asthma (P < 0.017), highlighting potential genetic correlation between anxiety disorders and asthma (P < 0.05 yet > 0.017). In bidirectional two-sample MR, inverse-variance weighted (IVW) analyses suggested that genetic liability to asthma was significantly associated with an increased risk of sensation of anxiety or depression (OR = 4.760, 95%CI: 1.645–13.777), and MDD (OR = 1.658, 95%CI: 1.477–1.860). Conversely, IVW analyses indicated that genetic liability to anxiety disorders was not associated with an increased risk of asthma (P > 0.01), nor was genetic liability to asthma associated with an increased risk of anxiety disorders (P > 0.01). Furthermore, no significant genetic causal relationships were observed for other studied traits. Multivariate MR, after adjusting for body mass index and alcohol consumption, further corroborated the independent causal effect of genetic predisposition to MDD on the risk of asthma (OR = 1.460, 95% CI: 1.285–1.660).
Conclusion: Our study establishes MDD as a predisposing factor for asthma. Meanwhile, anxiety disorders are not causal risk factors for asthma, nor is the reverse true. It is recommended to closely monitor asthma symptoms in patients with MDD.
Anxiety and depression, common mental disorders, have significant disability rates (1). Despite the frequent comorbidity of anxiety and depressive disorders (2), they exhibit distinct symptoms. Common symptoms of anxiety include fear, nervousness, and worry (3). Approximately 34% of American adults experience lifelong anxiety (4). Depression, characterized by emotional downturns, social impairments, and motor retardation, significantly limits psychosocial functioning and life quality (5).
Asthma, a chronic non-infectious respiratory condition, is marked by tracheobronchial obstruction and airway hyperresponsiveness (6). The 2015 Global Burden of Disease Study reports that asthma affects 358 million people globally, up 12.6% from 1990, highlighting its emergence as a major public health issue (7). Research shows that 18.1% of adults with asthma also suffer from depression (8). Given the aging demographic, the prevalence of asthma and associated mental health issues is likely to increase (9). Thus, understanding the intricate relationship between anxiety, depression, and asthma is crucial for both disease prevention and complication management.
Observational studies have explored the anxiety, depression, and asthma nexus, but clear patterns remain elusive. An international survey (10) found higher odds ratio (OR) of mental disorders in asthma patients: 1.6 for depression and 1.5 for anxiety (95% CIs: 1.4–1.8 and 1.4–1.7, respectively). Lehto et al. (11) reported associations between asthma and affective traits, with ORs of 1.67 for major depression and 1.45 for anxiety (95% CIs: 1.50–1.86 and 1.30–1.61). A meta-analysis (12) showed higher risks of anxiety symptoms or anxiety disorders in asthma patients (ORs: 1.89 and 2.08, 95% CIs: 1.42–2.52 and 1.70–2.56). These findings highlight the close relationship between asthma and psychological disorder and suggest that mental symptoms and mental disorders are also differentially related to asthma. However, the causal links, especially genetic ones, between anxiety, depression, and asthma are unclear, highlighting the need for more comprehensive, bidirectional studies.
In addition, conventional observational studies often struggle to infer causality accurately due to biases from unmeasured confounding factors and reverse causality. Mendelian randomization (MR) offers an alternative approach to measure causal effects more reliably (13). MR addresses these challenges effectively by leveraging the random genetic assignment during gametogenesis (14). By employing genetically determined variants as instrumental variables (IVs), MR discerns causal links between exposures and outcomes, minimizing risks of confounding and reverse causality (15).
Thus, to investigate the causal nature of this association, we utilized summary statistics from extensive genome-wide association studies (GWASs) and conducted a complexed genetic study comprising: (i) a genetic correlation analysis to determine the shared genetic foundation among anxiety, depression, and asthma; (ii) a bidirectional two-sample MR analysis to explore the causal impact of these relevant traits; and (iii) an inverse-variance weighted (IVW) multivariate MR (MVMR) analysis to account for principal confounders in univariable MR that revealed significant causal connections, as shown in Figure 1. By integrating genetic evidence, our aim was to clarify the nature of the previously established link between anxiety, depression, and asthma. This understanding may offer a scientific foundation for the prevention and treatment strategies of these conditions.
Figure 1 Study Design and Data Source. *In cases where univariable MR indicated a significant causal link. MR, Mendelian randomization; MVMR, multivariable MR; PCG, Psychiatric Genomics Consortium.
Utilizing the IEU database (https://gwas.mrcieu.ac.uk), we gathered GWAS datasets for anxiety and depression, identifying results for sensation states (labeled as “sensation of anxiety or depression”, “anxiety sensation”, and “depression sensation”) and clinical diagnoses (“anxiety disorders” and “major depression disorder”, MDD). This approach enabled us to stratify data based on disease severity, resulting in a hierarchical and progressive categorization of the outcomes. A fundamental aspect of two-sample MR is ensuring minimal or no sample overlap between exposure and outcome data, within acceptable limits (16). Consequently, we chose the latest R10 version of the GWAS datasets for asthma from the FinnGen database (17), released in December 2023.
The GWAS datasets utilized in this study entirely comprise European population samples. Detailed information is provided in Figure 1 and Supplementary Table S1.
No additional ethical approval was needed as all data are publicly accessible.
As for MR, we meticulously chose IVs consisting of independent, genome-wide significant SNPs from exposure and outcome datasets, forming a robust foundation for our MR analysis. To fulfill the three fundamental hypotheses of MR (18), we selected SNPs demonstrating strong correlation with exposure (P < 5×10–8) as instrumental variables for analyzing disease causality. To prevent linkage disequilibrium bias, significant SNPs linked to exposure factors must meet these criteria: r2<0.001 and a genetic distance exceeding 10,000kb. SNPs that don’t meet the independence criteria from confounding factors are excluded. We extracted SNPs related to exposure factors from the GWAS dataset, excluding those directly linked to the outcome (P < 5×10–5) to assure SNPs influence the outcome solely via exposure. To ensure robust results, the exclusion threshold for the Steiger Test has been set to a more stringent level of P < 5×10–5. Ultimately, we coordinated the data ensuring that the effects of SNPs on exposure and outcome were associated with the same allele. In analyzing “depression sensation” and “anxiety disorders” as exposure factors, we applied a less stringent threshold of P < 5×10–6 (19).
Upon finalizing the IVs for analysis, we record key data including allelic effect values (β), standard errors (SE), effect allele frequency (eaf), and P-values. We assessed the strength of the IVs using the F statistic, which is a function of the magnitude and precision of the genetic effect on the trait: F = R2 ×(N - 2)/(1 - R2), where R2 = 2 × eaf × (1 - eaf) × β2, and N is the sample size of the GWAS of SNPs with the trait. We only consider SNPs with an F-value greater than 10 as robust, as this indicates strength against weak instrument bias; those with lower F-values are rejected.
Linkage disequilibrium score regression (LDSC) has been utilized to assess the overall genetic correlation between “sensation of anxiety or depression”, “anxiety disorders”, “MDD”, and asthma (20). LDSC, grounded in genetic linkage disequilibrium (LD) principles, evaluates the genetic contributions to complex diseases and traits by quantifying LD associations between SNPs and their neighboring SNPs. Genome-wide SNP analysis reveals more about genetic etiologies than MR with selected SNPs (21). Genetic correlation estimates (rg) vary from -1 to +1, where -1 represents a perfect negative correlation, +1 a perfect positive correlation, and values closer to -1 or +1 signify stronger correlations.
We used a bidirectional two-sample MR approach to investigate the genetic causality between anxiety, depression, and asthma. The analysis entailed two steps: first, a forward MR with asthma as the outcome, followed by a reverse MR using asthma as the exposure. Sensitivity analysis using MR Egger and IVW methods validated the results.
Analyses encompassed bidirectional MR via five methods. IVW was the primary method in the absence of horizontal pleiotropy, alongside weighted median, MR-Egger regression, simple mode, and weighted mode. IVW aggregated SNP MR effect estimates for a comprehensive causal effect estimate (22), which was most reliable without horizontal pleiotropy (23) and transformed the data into OR and 95% confidence intervals (CI). MR-PRESSO identifies and corrects horizontal pleiotropy, eliminating outliers (24). Persistent pleiotropy leads to further outlier removal via RadialMR (25). IVW and MR-Egger regression quantified heterogeneity. A leave-one-out sensitivity analysis was conducted, sequentially omitting each SNP to assess its impact on the results.
In cases where univariable MR indicated a significant causal link between mental disorders and asthma, we conducted an IVW-based MVMR analysis (26) to adjust for major confounders including body mass index (BMI) and alcohol consumption (27). Summary-level association results were derived from the largest available GWAS for each phenotype, involving 806,843 (28) and 941,280 (29) individuals respectively. In the presence of heterogeneity in IVW results, the weighted median and MR-Egger methods are employed to yield a more robust causal inference (30).
Analyses utilized “TwoSampleMR” and “MR-PRESSO” in R Software (version 4.3.2) (31, 32). For multiple testing in LDSC, a Bonferroni-adjusted significance threshold was set at P < 0.017 (derived from 0.05/3) (33). Similarly, for multiple testing in two-sample MR, the Bonferroni correction was applied to set the significance threshold at P < 0.01 (0.05/5) (34) as well. P-values below 0.05 yet above the Bonferroni threshold suggest potential associations. In determining causality, a P-value < 0.05 combined with an OR > 1 implies positive genetic causality; conversely, an OR < 1 suggests negative genetic causality. A P-value of < 0.05 was considered statistically significant for MVMR. In MR-PRESSO, a P-valued ≥ 0.05 implies no pleiotropy. In IVW and MR-Egger, a P-value ≥ 0.05 suggests the absence of heterogeneity.
The results of the genetic correlation analyses between overall sensation (sensation of anxiety or depression), two mental disorders (anxiety disorders and MDD), and asthma are shown in Table 1. A significant positive genetic correlation was observed between sensation of anxiety or depression, MDD and asthma (P < 0.017). Additionally, a potential genetic correlation between anxiety disorders and asthma was noted (P < 0.05 yet > 0.017). These findings suggest a shared genetic basis for these complex phenotypes, including those with a potential correlation.
Considering the correlation between overall sensation (sensation of anxiety or depression) and asthma, anxiety sensation and depression sensation were included in the following bidirectional two-sample MR analysis, along with the three traits mentioned above. From the exposure data, we extracted 44, 67, 24, 8, and 44 SNPs, respectively. Ultimately, 8, 13, 22, 6, and 40 SNPs were utilized, each with an F statistic > 10, as illustrated in Figure 2. After excluding outliers for two exposure variables (“anxiety sensation”, “MDD”), no evidence of pleiotropy was observed in the subsequent pleiotropy test. Therefore, MR analysis was performed using IVW as the primary method.
Figure 2 The selection process of instrumental variables (IVs). (A) Sensation of anxiety or depression, (B) Anxiety sensation, (C) Depression sensation, (D) Anxiety disorders, (E) Major depression disorder (MDD). A B
C
D
E
We identified a causal relationship between sensation of anxiety or depression, MDD, and asthma. We observed that genetically predicted sensation of anxiety or depression was positively associated with the risk of asthma (OR =4.760, 95%CI: 1.645–13.777, P =0.004), and MDD was also positively associated with the risk of asthma (OR =1.658, 95%CI: 1.477–1.860, P =7.62×10–18). In contrast, we discovered no evidence that genetically predicted anxiety sensation was associated with the risk of asthma (OR = 1.837, 95% CI: 0.745–4.531, P = 0.187), genetically predicted depression sensation was associated with the risk of asthma (OR = 0.917, 95% CI: 0.619–1.357, P = 0.664), or genetically predicted anxiety disorders were associated with the risk of asthma (OR = 1.036, 95% CI: 0.984–1.091, P = 0.178), as displayed in Figure 3. Figure 4 presents the results of the scatter plots for outcomes causally related to asthma. The heterogeneity analysis yielded results indicating uniformity across the IVs, as evidenced in Supplementary Table S2. Furthermore, the robustness of the MR analysis was confirmed through sensitivity analysis employing the leave-one-out method, as shown in Supplementary Figure S1.
Figure 3 The MR analysis results between mental disorders and asthma. In both forward and reverse Mendelian Randomization (MR), Inverse Variance Weighted (IVW) method is primarily employed. (A) The causal effect of anxiety and depression on asthma (forward MR), (B) The causal effect of asthma on anxiety and depression (reverse MR).
Figure 4 Scatter plots of Mendelian Randomization (MR) where exposure is causally related to outcome. The MR analysis employed five methods, namely inverse variance weighted (IVW), weighted median, MR-Egger regression, simple mode, and weighted mode. (A) “Sensation of anxiety or depression” as exposure (B) Major depression disorder (MDD) as exposure.
We extracted 19 SNPs from the exposure data. Finally, 17, 17, 18, 14, and 13 SNPs were employed, each demonstrating an F statistic >10, as depicted in Figure 2. Following the removal of an outlier from the variable associated with MDD as the outcome, no evidence of pleiotropy was observed in the subsequent pleiotropy test. Consequently, the MR analysis proceeded using the IVW method as the primary approach.
The analysis suggested there was no association between genetically predicted asthma and the risk of developing anxiety disorders (OR =1.107, 95%CI: 0.858–1.428, P =0.435). Similarly, our analysis found no significant association between elevated genetically predicted asthma and the increased risk of other mental states, as illustrated in Figure 3. The heterogeneity analysis revealed consistent results among the instrumental variables (Supplementary Table S2). Moreover, the MR analysis’s robustness was substantiated by a sensitivity analysis using the leave-one-out method (Supplementary Figure S2).
Multivariate MR, adjusted for BMI and alcohol consumption, reinforced the independent causal impact of MDD on asthma risk, albeit with a marginally reduced effect size (OR =1.460, 95% CI: 1.285 -1.660, P=7.05×10–9). Given the heterogeneity in IVW results, we applied the MR-Egger and weighted median methods. These methods corroborated the initial findings, showing enhanced results, as outlined in Table 2.
Using comprehensive GWAS data, we systematically examined the bidirectional link between common mental disorders and asthma. Our preliminary results suggested a shared genetic foundation among sensation of anxiety or depression, anxiety disorders, MDD and asthma, including those with a potential correlation. Additionally, our two-sample MR studies indicated a unidirectional causal effect of genetic predisposition to MDD on asthma, which was further affirmed by multivariate MR adjustments. Concurrently, our research also indicated that anxiety disorders are not causal risk factors for asthma, and likewise, the reverse is not true.
Anwar et al. (35), utilizing a phenome-wide association study (PheWAS) followed by MR, and Zhu et al. (18), via generalized summary data MR, both uncovered evidence for the causal effects of MDD on the risk of asthma. Our MR analysis not only is in line with the existing evidence regarding MDD but also distinctively emphasizes its substantial causal impact on asthma, enhancing the previous MR study (34) in three major aspects. Initially, we classified depression into two severity-based categories leading to more precise results. It revealed that specifically the clinical state of depression, which reached the diagnosis of MDD, rather than mere feelings, is causally linked to asthma. Second, the prior MR study’s GWAS data for depression and asthma had a 6.7% sample overlap, posing a risk of type I error. Our study used distinct, updated GWAS datasets, enhancing the validity of our results. Third, our study expanded the MR analysis to include a comprehensive framework with sensitivity analyses for directional pleiotropy and a multivariate MR approach, providing an independent causal assessment. The evidence supporting a causal effect of MDD on asthma is increasingly compelling.
Multiple mechanisms could elucidate the link between MDD and asthma. Depression may cause vagally mediated bronchoconstriction or increased interoceptive sensitivity, accelerating asthma development (36, 37). Chen proposed that depression might intensify airway inflammation in response to environmental triggers (38). Key biological pathways involved are the hypothalamic-pituitary-adrenal (HPA) axis, the sympathetic-adrenal-medullary (SAM) axis, and the sympathetic (SNS) and parasympathetic (PNS) arms of the autonomic nervous system. Depression can activate the SNS, triggering epinephrine release and stimulating noradrenergic fibers in lymphoid tissues. Released catecholamines binding to lymphocytes may enhance humoral responses (39). Concurrently, adrenergic receptors may trigger mast cells to release histamines and activate eosinophils in the airways. Iwata et al. (40) suggested the NOD-like receptor family pyrin domain-containing 3 (NLRP3) inflammasome in inflammatory signal transduction as a potential link between depression and asthma. Secondly, depression might elevate asthma risk through lifestyle factors like smoking and poor health management, and depression may also impact lung function directly, possibly through hyperventilation mechanisms (41, 42). Thirdly, Zhu et al. (34) identified a genetic link between depression and asthma, with an overlap in the POLI gene, suggesting a role for DNA polymerase iota (η) enzyme in this relationship.
A previous study (11) observed a genetic correlation between asthma and depression using LDSC, yet it did not find the connection between asthma and anxiety. On the other hand, Zhu et al. (34) encountered limitations due to a lack of significant SNPs in their anxiety GWAS for adequate MR analysis. Similarly, Ashley et al. (43) applied MR analysis but could not confirm a causal link from asthma to MDD or anxiety. Utilizing updated datasets, our research successfully carried out genetic analysis and identified a potential genetic correlation between asthma and anxiety disorders through LDSC. Furthermore, our study suggests that there is no causal effect of asthma on anxiety disorders, as evident from MR analysis, contrasting with earlier observational studies. Potential unmeasured confounders in earlier studies might explain this discrepancy. Thought there are several possible mechanisms for asthma’s effect on anxiety disorders include: 1) Activation of the mPFC-amygdala circuit by neuroimmune responses (44), and 2) Enhancement of microglia and astrocytes by Th2 inflammatory factors, increasing neural oscillations (45), however, additional research is warranted to solidify this causal association. Additionally, while focusing on shared genetic factors, our study was not able to account for environmental or other non-genetic factors, such as asthma awareness potentially contributing to increased anxiety. Further investigation is required to elucidate the causal dynamics. Moreover, depression and anxiety have overlapping regulatory pathways in asthma pathogenesis, like oxidative stress and inflammation (46), which may influence their causal interrelations.
It’s important to acknowledge several limitations in our study. Firstly, asthma, anxiety, and depression are heterogeneous, with correlations influenced by various factors like gender and age; for example, females with severe asthma often tend to have higher rates of anxiety and depression than males (47). Additionally, the bidirectional relationship between depression, anxiety, and asthma not only exists in adults but also extends to children with asthma (48). Our understanding is still limited, which necessitates future studies with larger sample sizes to conduct more detailed subgroup analysis of these diseases. Second, using European population data to reduce stratification bias may limit generalizability to other populations. Conclusively verifying these relationships demands further analyses, including gene function studies and longitudinal research.
In conclusion, large-scale genetic studies reveal a significant causal effect of MDD on asthma and indicate that anxiety disorders are not causal risk factors for asthma, nor is the reverse true. These findings highlight the importance of MDD prevention in reducing asthma incidence. This research contributes to advancing our understanding of disease prevention and the management of complications.
Publicly available datasets were analyzed in this study. The accession websites and GWAS ID for the datasets can be found in Supplementary Material Table S1. Further inquiries can be directed to the corresponding author.
ZY: Writing – original draft, Writing – review & editing, Software. JC: Funding acquisition, Investigation, Visualization, Writing – review & editing. LG: Project administration, Writing – review & editing. HZ: Visualization, Writing – review & editing. YD: Visualization, Writing – review & editing. GR: Visualization, Writing – review & editing. YM: Visualization, Writing – review & editing. RB: Visualization, Writing – review & editing. XM: Project administration, Writing – review & editing.
The author(s) declare financial support was received for the research, authorship, and/or publication of this article. Innovation Fund of China Academy of Chinese Medical Sciences (No. G12021A00904).
This research acknowledges the contributions of participants and investigators of the FinnGen study, along with researchers providing genome-wide summary statistics. Additionally, special thanks to Aochuan Sun of Beijing University of Traditional Chinese Medicine for his invaluable methodological guidance.
The authors declare that the research was conducted in the absence of any commercial or financial relationships that could be construed as a potential conflict of interest.
All claims expressed in this article are solely those of the authors and do not necessarily represent those of their affiliated organizations, or those of the publisher, the editors and the reviewers. Any product that may be evaluated in this article, or claim that may be made by its manufacturer, is not guaranteed or endorsed by the publisher.
The Supplementary Material for this article can be found online at: https://www.frontiersin.org/articles/10.3389/fpsyt.2024.1372842/full#supplementary-material
1. GBD 2019 Mental Disorders Collaborators. Global, regional, and national burden of 12 mental disorders in 204 countries and territories, 1990–2019: A systematic analysis for the global burden of disease study 2019. Lancet Psychiatry. (2022) 9:137–50. doi: 10.1016/S2215-0366(21)00395-3
2. Groen RN, Ryan O, Wigman JTW, Riese H, Penninx BWJH, Giltay EJ, et al. Comorbidity between depression and anxiety: Assessing the role of bridge mental states in dynamic psychological networks. BMC Med. (2020) 18:308. doi: 10.1186/s12916-020-01738-z
3. Szuhany KL, Simon NM. Anxiety disorders: A review. Jama. (2022) 328:2431–45. doi: 10.1001/jama.2022.22744
4. Kessler RC, Petukhova M, Sampson NA, Zaslavsky AM, Wittchen HU. Twelve-month and lifetime prevalence and lifetime morbid risk of anxiety and mood disorders in the United States. Int J Meth Psych Res. (2012) 21:169–84. doi: 10.1002/mpr.1359
5. Cruz-Pereira JS, Rea K, Nolan YM, O’Leary OF, Dinan TG, Cryan JF. Depression’s unholy trinity: Dysregulated stress, immunity, and the microbiome. Annu Rev Psychol. (2020) 71:49–78. doi: 10.1146/annurev-psych-122216-011613
6. Stern J, Pier J, Litonjua AA. Asthma epidemiology and risk factors. Semin Immunopathol. (2020) 42:5–15. doi: 10.1007/s00281-020-00785-1
7. GBD 2015 Chronic Respiratory Disease Collaborators. Global, regional, and national deaths, prevalence, disability-adjusted life years, and years lived with disability for chronic obstructive pulmonary disease and asthma, 1990–2015: A systematic analysis for the global burden of disease study 2015. Lancet Respir Med. (2017) 5:691–706. doi: 10.1016/S2213-2600(17)30293-X
8. Moussavi S, Chatterji S, Verdes E, Tandon A, Patel V, Ustun B. Depression, chronic diseases, and decrements in health: Results from the world health surveys. Lancet (London England). (2007) 370:851–8. doi: 10.1016/S0140-6736(07)61415-9
9. Skou ST, Mair FS, Fortin M, Guthrie B, Nunes BP, Miranda JJ, et al. Multimorbidity. Nat Rev Dis Primers. (2022) 8:48. doi: 10.1038/s41572-022-00376-4
10. Scott KM, Von Korff M, Ormel J, Zhang MY, Bruffaerts R, Alonso J, et al. Mental disorders among adults with asthma: Results from the world mental health survey. Gen Hosp Psychiatry. (2007) 29:123–33. doi: 10.1016/j.genhosppsych.2006.12.006
11. Lehto K, Pedersen NL, Almqvist C, Lu Y, Brew BK. Asthma and affective traits in adults: A genetically informative study. Eur Respir J. (2019) 53:1802142. doi: 10.1183/13993003.02142-2018
12. Ye G, Baldwin DS, Hou R. Anxiety in asthma: A systematic review and meta-analysis. Psychol Med. (2021) 51:11–20. doi: 10.1017/S0033291720005097
13. Swanson SA, Tiemeier H, Ikram MA, Hernán MA. Nature as a trialist?: deconstructing the analogy between Mendelian randomization and randomized trials. Epidemiology. (2017) 28:653–9. doi: 10.1097/EDE.0000000000000699
14. Richmond RC, Davey Smith G. Mendelian randomization: concepts and scope. Cold Spring Harb Perspect Med. (2022) 12:a040501. doi: 10.1101/cshperspect.a040501
15. Hu S, Xing H, Wang X, Zhang N, Xu Q. Causal relationships between total physical activity and ankylosing spondylitis: A mendelian randomization study. Front Immunol. (2022) 13:887326. doi: 10.3389/fimmu.2022.887326
16. Burgess S, Davies NM, Thompson SG. Bias due to participant overlap in two-sample Mendelian randomization. Genet Epidemiol. (2016) 40:597–608. doi: 10.1002/gepi.21998
17. Kurki MI, Karjalainen J, Palta P, Sipilä TP, Kristiansson K, Donner KM, et al. FinnGen provides genetic insights from a well-phenotyped isolated population. Nature. (2023) 613:508–18. doi: 10.1038/s41586-022-05473-8
18. Burgess S, Butterworth A, Thompson SG. Mendelian randomization analysis with multiple genetic variants using summarized data. Genet Epidemiol. (2013) 37:658–65. doi: 10.1002/gepi.21758
19. Zhou Q, Jin X, Li H, Wang Q, Tao M, Wang J, et al. Cholesterol and low-density lipoprotein as a cause of psoriasis: results from bidirectional mendelian randomization. J Eur Acad Dermatol Venereol. (2024) 38:710–8. doi: 10.1111/jdv.19670
20. Bulik-Sullivan B, Finucane HK, Anttila V, Gusev A, Day FR, Loh PR, et al. An atlas of genetic correlations across human diseases and traits. Nat Genet. (2015) 47:1236–41. doi: 10.1038/ng.3406
21. Li GHY, Cheung CL, Chung AKK, Cheung BMY, Wong ICK, Fok MLY, et al. Evaluation of bi-directional causal association between depression and cardiovascular diseases: a Mendelian randomization study. Psychol Med. (2022) 52:1765–76. doi: 10.1017/S0033291720003566
22. Ellingjord-Dale M, Papadimitriou N, Katsoulis M, Yee C, Dimou N, Gill D, et al. Coffee consumption and risk of breast cancer: A Mendelian randomization study. PloS One. (2021) 16:e0236904. doi: 10.1371/journal.pone.0236904
23. Huang S, Tian F, Yang X, Fang S, Fan Y, Bao J. Physical activity and systemic lupus erythematosus among European populations: A two-sample Mendelian randomization study. Front Genet. (2022) 12:784922. doi: 10.3389/fgene.2021.784922
24. Sang N, Gao RC, Zhang MY, Wu ZZ, Wu ZG, Wu GC. Causal relationship between sleep traits and risk of systemic Lupus erythematosus: A two-sample Mendelian randomization study. Front Immunol. (2022) 13:918749. doi: 10.3389/fimmu.2022.918749
25. Bowden J, Spiller W, Del Greco MF, Sheehan N, Thompson J, Minelli C, et al. Improving the visualization, interpretation and analysis of two-sample summary data Mendelian randomization via the Radial plot and Radial regression. Int J Epidemiol. (2018) 47:2100–0. doi: 10.1093/ije/dyy265
26. Burgess S, Thompson SG. Multivariable Mendelian randomization: the use of pleiotropic genetic variants to estimate causal effects. Am J Epidemiol. (2015) 181:251–60. doi: 10.1093/aje/kwu283
27. Mikkelsen H, Landt EM, Benn M, Nordestgaard BG, Dahl M. Causal risk factors for asthma in mendelian randomization studies: A systematic review and meta-analysis. Clin Trans Allergy. (2022) 12:e12207. doi: 10.1002/clt2.12207
28. 23andMe Research Team, HUNT All-In Psychiatry, Liu M, Jiang Y, Wedow R, Li Y, et al. Association studies of up to 1.2 million individuals yield new insights into the genetic etiology of tobacco and alcohol use. Nat Genet. (2019) 51:237–44. doi: 10.1038/s41588-018-0307-5
29. Pulit SL, Stoneman C, Morris AP, Wood AR, Glastonbury CA, Tyrrell J, et al. Meta-analysis of genome-wide association studies for body fat distribution in 694 649 individuals of European ancestry. Hum Mol Genet. (2019) 28:166–74. doi: 10.1093/hmg/ddy327
30. Rees JMB, Wood AM, Burgess S. Extending the MR-Egger method for multivariable Mendelian randomization to correct for both measured and unmeasured pleiotropy. Stat Med. (2017) 36:4705–18. doi: 10.1002/sim.7492
31. Yavorska OO, Burgess S. MendelianRandomization: An R package for performing mendelian randomization analyses using summarized data. Int J Epidemiol. (2017) 46:1734–9. doi: 10.1093/ije/dyx034
32. Patel A, Ye T, Xue H, Lin Z, Xu S, Woolf B, et al. MendelianRandomization v0.9.0: Updates to an R package for performing mendelian randomization analyses using summarized data. Wellcome Open Res. (2023) 8:449. doi: 10.12688/wellcomeopenres
33. Armstrong RA. When to use the bonferroni correction. Ophthalmic Physiol Opt: J Br Coll Ophthalmic Opt (Optom). (2014) 34:502–8. doi: 10.1111/opo.12131
34. Zhu Z, Zhu X, Liu CL, Shi H, Shen S, Yang Y, et al. Shared genetics of asthma and mental health disorders: A large-scale genome-wide cross-trait analysis. Eur Respir J. (2019) 54:1901507. doi: 10.1183/13993003.01507-2019
35. Mulugeta A, Zhou A, King C, Hyppönen E. Association between major depressive disorder and multiple disease outcomes: a phenome-wide Mendelian randomisation study in the UK Biobank. Mol Psychiatry. (2020) 25:1469–76. doi: 10.1038/s41380-019-0486-1
36. Wright R. Stress and atopic disorders. J Allergy Clin Immunol. (2005) 116:1301–6. doi: 10.1016/j.jaci.2005.09.050
37. Wright RJ, Rodriguez M, Cohen S. Review of psychosocial stress and asthma: an integrated biopsychosocial approach. Thorax. (1998) 53:1066–74. doi: 10.1136/thx.53.12.1066
38. Chen E, Miller GE. Stress and inflammation in exacerbations of asthma. Brain Behav Immun. (2007) 21:993–9. doi: 10.1016/j.bbi.2007.03.009
39. Marshall GD, Agarwal SK. Stress, immune regulation, and immunity: applications for asthma. Allergy Asthma Proc. (2000) 21:241–6. doi: 10.2500/108854100778248917
40. Iwata M, Ota KT, Duman RS. The inflammasome: Pathways linking psychological stress, depression, and systemic illnesses. Brain Behavior Immunity. (2013) 31:105–14. doi: 10.1016/j.bbi.2012.12.008
41. Chan A, Yii A, Tay CK, Lapperre T, Tan LL, Yeoh F, et al. The impact of anxiety and depression on asthma-related health outcomes: a prospective study. Allergy Immunol. (2015), PA5097. doi: 10.1183/13993003.congress-2015.PA5097
42. Cleveland Clinic. Smoking & asthma: effects, prevention. Available online at: https://my.clevelandclinic.org/health/articles/4584-smoking–asthma.
43. Budu-Aggrey A, Joyce S, Davies NM, Paternoster L, Munafò MR, Brown SJ, et al. Investigating the causal relationship between allergic disease and mental health. Clin Exp Allergy: J Br Soc Allergy Clin Immunol. (2021) 51:1449–58. doi: 10.1111/cea.14010
44. Liu WZ, Zhang WH, Zheng ZH, Zou JX, Liu XX, Huang SH, et al. Identification of a prefrontal cortex-to-amygdala pathway for chronic stress-induced anxiety. Nat Commun. (2020) 11:2221. doi: 10.1038/s41467-020-15920-7
45. Gholami-Mahtaj L, Mooziri M, Dehdar K, Abdolsamadi M, Salimi M, Raoufy MR. ACC-BLA functional connectivity disruption in allergic inflammation is associated with anxiety. Sci Rep. (2022) 12:2731. doi: 10.1038/s41598-022-06748-w
46. Jiang M, Qin P, Yang X. Comorbidity between depression and asthma via immune-inflammatory pathways: A meta-analysis. J Affect Disord. (2014) :166:22–9. doi: 10.1016/j.jad.2014.04.027
47. Anastasia P, Eleni T, Eleftheria M, Xenia N, Eygenia P, Kyriakos S, et al. Depression levels influence the rate of asthma exacerbations in females. J Personalized Med. (2021) 11:586. doi: 10.3390/jpm11060586
Keywords: mendelian randomization, causality, asthma, mental disorder, anxiety, depression
Citation: Yan Z, Chen J, Guo L, Zhang H, Ding Y, Ren G, Mao Y, Bai R and Ma X (2024) Genetic analyses of the bidirectional associations between common mental disorders and asthma. Front. Psychiatry 15:1372842. doi: 10.3389/fpsyt.2024.1372842
Received: 18 January 2024; Accepted: 17 May 2024;
Published: 06 June 2024.
Edited by:
Drozdstoy Stoyanov, Plovdiv Medical University, BulgariaReviewed by:
Youqian Zhang, Yangtze University, ChinaCopyright © 2024 Yan, Chen, Guo, Zhang, Ding, Ren, Mao, Bai and Ma. This is an open-access article distributed under the terms of the Creative Commons Attribution License (CC BY). The use, distribution or reproduction in other forums is permitted, provided the original author(s) and the copyright owner(s) are credited and that the original publication in this journal is cited, in accordance with accepted academic practice. No use, distribution or reproduction is permitted which does not comply with these terms.
*Correspondence: Xiaochang Ma, bWF4aWFvY2hhbmdAeDI2My5uZXQ=
†These authors have contributed equally to this work and share first authorship
Disclaimer: All claims expressed in this article are solely those of the authors and do not necessarily represent those of their affiliated organizations, or those of the publisher, the editors and the reviewers. Any product that may be evaluated in this article or claim that may be made by its manufacturer is not guaranteed or endorsed by the publisher.
Research integrity at Frontiers
Learn more about the work of our research integrity team to safeguard the quality of each article we publish.