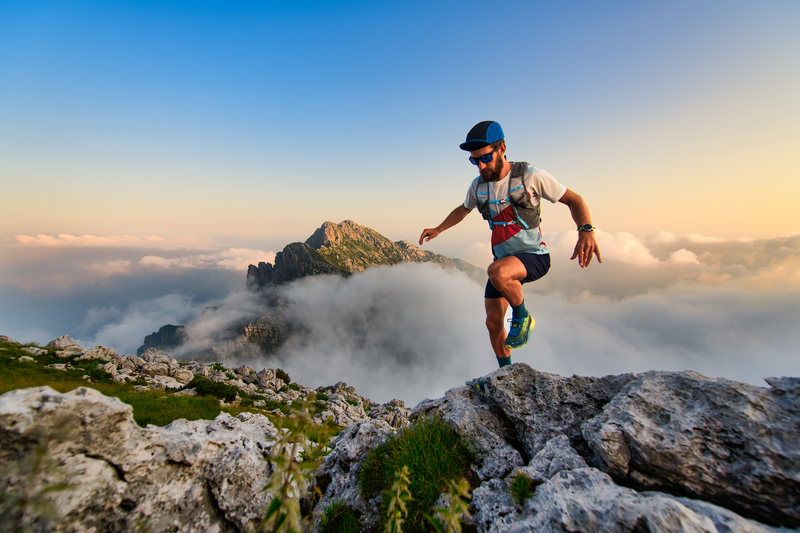
95% of researchers rate our articles as excellent or good
Learn more about the work of our research integrity team to safeguard the quality of each article we publish.
Find out more
ORIGINAL RESEARCH article
Front. Psychiatry , 13 June 2024
Sec. Public Mental Health
Volume 15 - 2024 | https://doi.org/10.3389/fpsyt.2024.1366005
This article is part of the Research Topic Parents with Mental and/or Substance Use Disorders and their Children, Volume III View all 33 articles
Objective: Facial emotion recognition (FER) is a fundamental social skill essential for adaptive social behaviors, emotional development, and overall well-being. FER impairments have been linked to various mental disorders, making it a critical transdiagnostic mechanism influencing the development and trajectory of mental disorders. FER has also been found to play a role in the transgenerational transmission of mental disorders, with the majority of research suggesting FER impairments in children of parents with a mental illness (COPMI). Previous research primarily concentrated on COPMI of parents with internalizing disorders, which does not cover the full spectrum of outpatient mental health service populations. Furthermore, research focuses on varying components of FER by using different assessment paradigms, making it challenging to compare study results. To address these gaps, we comprehensively investigated FER abilities in COPMI using multiple tasks varying in task characteristics.
Methods: We included 189 children, 77 COPMI and 112 children of parents without a diagnosed mental illness (COPWMI), aged 6 to 16 years. We assessed FER using three tasks with varying task demands: an emotional Go/NoGo task, a morphing task, and a task presenting short video sequences depicting different emotions. We fitted separate two-level hierarchical Bayesian models (to account for sibling pairs in our sample) for reaction times and accuracy rates for each task. Good model fit was assured by comparing models using varying priors.
Results: Contrary to our expectations, our results revealed no general FER deficit in COPMI compared to COPWMI. The Bayesian models fitted for accuracy in the morphing task and Go/NoGo task yielded small yet significant effects. However, Bayes factors fitted for the models suggested that these effects could be due to random variations or noise in the data.
Conclusions: Our study does not support FER impairments as a general feature of COPMI. Instead, individual factors, such as the type of parental disorder and the timing of its onset, may play a crucial role in influencing FER development. Future research should consider these factors, taking into account the diverse landscape of parental mental disorders.
Facial emotion recognition (FER) describes the ability to correctly decipher emotional expressions in human faces, which is crucial for adaptive social behaviors, emotional development, and overall well-being (1). This fundamental social skill emerges early in life, with the first precursors presenting in infancy (2–5). FER continues to improve throughout childhood (6) and reaches peak performance in early adulthood before declining again (7). Research suggests that genetic and environmental factors play a role in the development of FER (8–11). Parents are a critical factor in FER development, especially during early childhood, because they shape their children’s emotional environment in direct ways, such as explicit teaching of emotions, as well as indirectly through their own beliefs about emotions and their own FER abilities (12).
Impaired FER is linked to various mental disorders in both children and adults (13), such as internalizing disorders (14–16), schizophrenia (17), borderline personality disorder (18), and externalizing disorders (19). It is therefore considered to be an important transdiagnostic feature relevant to the development and course of mental illness (20). FER also serves as a foundational element for further emotion processing, regulation, empathy, and, consequently, proficient social interaction (9)—each of which is compromised across a spectrum of disorders as well (20). Beyond being a transdiagnostic feature in mental illness, research shows that abnormal FER predates the onset and development of mental disorders (9, 21–23).
FER research has gained popularity over the past years, with assessment methods becoming more diverse. While early research on FER focused solely on identifying emotions in static pictures, more recent studies use a broader variety of assessment paradigms. This evolution is in part due to improvements in assessment technologies but also theoretically based as emotional expressions in natural contexts are often swift and subtle, meaning static pictures are not as ecologically valid as other stimulus types (24, 25). However, as the landscape of studies on FER becomes more diverse regarding study design and employed tasks, the results also become more challenging to compare as task characteristics have a significant influence on study outcomes (26, 27). One important factor to consider is the outcome measure used to assess FER. Some studies focus on accuracy rates, while others focus on reaction speed or sensitivity in FER assessment. These measures likely represent distinct components of FER and rely on different cognitive processes (26–28). Differences in the outcome measures also come with different practical implications: Because facial expressions change rapidly and are often subtle in realistic contexts, results measuring sensitivity toward emotional expressions might be more relevant than accuracy rates when deriving clinical implications from research findings. Furthermore, factors such as stimulus differences (e.g., ethnicity or sex of the faces), contextual clues, and response requirements can influence study outcomes (27). In addition, the answer formats differ across studies. While in some studies participants are asked to name the presented emotion without any prompt, other studies rely on forced-choice formats. Another critical difference between studies is whether the stimuli are static or contain motion. Because emotional expressions in human faces are dynamic, it can be argued that results derived from static stimuli display lower ecological validity than results gained with dynamic stimuli.
Taken together, FER is a relevant feature in the context of mental disorders and has gained popularity as a research subject in recent years. However, the diversity in study design and task characteristics must be considered when interpreting and comparing study outcomes.
Children of parents with a mental illness (COPMI) exhibit higher subclinical internalizing and externalizing symptom rates (29), compared to children of parents without a mental illness (COPWMI), and have a significantly elevated risk of mental disorder development (20, 30, 31). Considering parents’ vital role in shaping their children’s emotional environment in various ways (12) in conjunction with the significance of FER in the context of mental illness, it is plausible to assume FER impairments in COPMI.
However, research on FER in COPMI yields heterogeneous results. While the majority of studies suggest FER impairments in COPMI to varying extents, a few studies found FER improvements for specific emotions compared to COPMWI. Research on FER in children of parents with depression provides evidence supporting the idea of FER deficits (21, 32). Joormann et al. (32) assessed FER in daughters of mothers with a history of depression using facial stimuli morphing from a neutral to an emotional facial expression (morphing task). They found daughters of mothers with a history of depression to make more errors in identifying anger and to require higher intensity to identify sad facial expressions accurately. Mannie et al. (21) add to this body of evidence. In their study, young adults who had a biological parent with a history of depression performed significantly slower in an emotional categorization task. Furthermore, research found that FER deficits among COPMI were discernible from an early age (33–35). In a study by Székely et al. (33), depressive symptoms at any time point during the child’s life significantly predicted impaired accuracy in an emotion identification task using static pictures in preschoolers. Meiser et al. (34) found aligning evidence, stating that preschool-aged children whose mothers had suffered from postpartum depression or anxiety disorders performed significantly worse on emotion labeling tasks. Also, FER impairments do not seem to be specific to children of parents with depression, because research found FER impairments in children of parents with other disorders as well (6, 24, 36–41). Hanford et al. (37) assessed FER in children of parents diagnosed with bipolar disorder using a task presenting emotional expressions in static faces at different intensities. They included symptomatic as well as asymptomatic teenage children in their study. Here, children of parents diagnosed with bipolar disorder made more errors across emotions regardless of their own diagnostic status. Sharma et al. (38) add to these findings because they found unaffected school-aged children of parents diagnosed with bipolar disorder to conduct more errors in overall emotion recognition and specifically in the recognition of fear on a static picture task. In a study by Bilodeau et al. (6), the existing evidence was expanded to children of parents with panic disorder. They assessed FER in unaffected children of parents with a current or past history of panic disorder and found those children made more errors in recognizing fear and anger as well as sadness. Horton et al. (40) noted that children of parents with diagnosed schizophrenia show lower accuracy as well as recognition speed across all emotions and for fear specifically.
However, as mentioned above, a few studies yielded contrasting results. Lopez-Duran et al. (42) assessed FER in children whose parents have a documented history of childhood-onset depression via a morphing task. They found that boys, but not girls, of parents with a history of depression require lower intensity levels to correctly identify sadness in a morphing task. Burkhouse et al. (43) assessed FER in children of mothers with a history of depression using a forced-choice emotion identification task. They found that children of mothers with a history of depression exhibit increased sensitivity in detecting sad faces and reduced sensitivity in detecting happy faces only if the children carried a specific allele in their oxytocin receptor gene. Children who did not have this genetic predisposition did not show any differences in FER compared to the control group. Joormann et al. (44) added to this body of evidence because they found that daughters of mothers with depression display selective attention toward negative facial expressions, while daughters of healthy mothers exhibited selective attention to positive facial expressions after a negative mood induction paradigm. The “stress acceleration hypothesis” explains these results. It proposes FER improvements for specific emotions in COPMI as an adaptive survival strategy to a negative environment (45). According to this theory, it can be beneficial for children of parents with depression to be sensitive toward sad facial expressions, because they are a relevant social cue in their home environment and may be connected to certain expectations regarding the child’s behavior. However, the studies mentioned above only show FER improvements for specific emotions and in specific contexts (e.g., children with certain genetic predispositions), suggesting that FER improvements are not a general feature related to parental mental illness.
Taken together, while a few studies find FER improvements for specific emotions and/or in specific contexts (42–45), most research suggests FER impairments in COPMI (6, 8, 10, 11, 21, 32–34, 36–41). However, due to differences in study designs and employed tasks, it remains unclear whether these deficits are specific to certain emotions, tasks, or stimuli. Furthermore, almost all research on FER in COPMI focuses on children of parents with internalizing disorders. However, this is not representative of populations in the mental healthcare system. To expand existing findings and to ensure generalizability for populations assessing outpatient mental health services, it is essential to assess a more heterogeneous group of COPMI regarding parental disorder type.
Thus, our study employs different tasks with varying task demands in a COPMI sample representative for populations assessing outpatient mental health services to get deeper insights into FER in COPMI. In line with the majority of research, we hypothesized that COPMI would show impairments in FER across all three tasks. Specifically, we hypothesized that COPMI would show lower accuracy rates and higher reaction times in an emotional Go/NoGo task (H1). Second, we expected that COPMI would show lower accuracy rates and higher reaction times in a morphing task (H2). Third, we expected lower accuracy rates in correctly naming emotions in a non-speeded task presenting emotional video sequences (H3).
The present study was conducted in a cross-sectional setting. Participants took part in a questionnaire assessment as well as a lab assessment. See Section 2.5. for more details. The present study is part of a randomized controlled multicenter study of a preventive intervention for COPMI in Germany (COMPARE-family) and its add-on project COMPARE-emotion). The projects are described in detail in the study protocols (46, 47). The local ethics committee approved the study, and informed consent was given by each parent and child participating in COMPARE-family and COMPARE-emotion.
The inclusion criteria were as follows: a) children between 6 and 16 years of age for COPMI, b) a parent with a mental illness according to the Diagnostic and Statistical Manual of Mental Disorders (DSM-5) (48) assessed via a semistructured clinical interview and for COPWMI, and c) parents without a current or past mental disorder and without current or past psychotherapeutic treatment. The exclusion criteria were a) insufficient German language skills of children and parents; b) for COPMI, severe impairment of the children requiring comprehensive treatment; c) a parent ongoing outpatient or inpatient treatment or continuous use of benzodiazepines; and d) for COPWMI, a TCBCL ≥62.
COPMI were recruited as part of the COMPARE-family project (46, 47). Parents with a mental illness were primarily recruited through university outpatient clinics in Gießen and Bochum in Germany. In Gießen, families were additionally contacted via letters to families with children in the corresponding age range provided by the local registry office, public advertisement, information material in inpatient psychiatric clinics and psychotherapeutic practices, and the University’s internal mailing list. COPWMI were recruited in Gießen and Dortmund as part of the add-on project COMPARE-emotion via the respective research group’s database of former study participants, mailings of families with children in the corresponding age range, social media advertisement, and public advertisement in schools and daycare centers.
SES was assessed according to the Studie zur Gesundheit von Kindern und Jugendlichen in Deutschland (KiGGS) study (49). The SES index ranges between 3.0 and 21.0 and considers education, professional qualifications, status, and net household income. The index can be used as a metric variable or grouped into low, middle, and high SES (50). We assessed SES in a metric way.
We used the German parent-report version of the Child Behaviour Checklist 6–18R (CBCL 6–18R (51);) to assess the psychopathology of COPMI and COPWMI. Items are aggregated into three superordinate scales (externalizing problems, internalizing problems, and total problems). Good to very good internal consistencies were reported for the CBCL 6–18R (Cronbach’s alpha =.82 –.93 (51);). We derived T-values for analyses according to the norm tables provided in the questionnaire manual.
We used the German version of the self-reported questionnaire Brief Symptom Inventory (BSI (52);) to assess parents’ psychopathology. The BSI contains 53 items rated on a five-point Likert scale from 0 (not at all) to 4 (very much). We aggregated items to the Global Severity Index (GSI). DerogatisKlicken oder tippen Sie hier, um Text einzugeben (52). reported very good internal consistency of GSI (Cronbach’s alpha = .97). Parents of COPWMI were excluded if GSI was above the cutoff value (TGSI ≥ 62). For further analyses, we derived T-values according to the norm tables provided in the questionnaire manual.
The DIPS (53) is a semistructured diagnostic interview to determine mental disorders according to the DSM-5 (48). Trained clinicians conducted it to assess the diagnostic status of the parents of COPMI. Previous studies report high interrater reliability (.72 < κ <.92) and test–retest reliabilities, mostly in the range of.62 to.94 (53).
This task was developed by Tottenham et al. (54) as a means to assess different components of emotion processing in children. The stimuli for this task consisted of grayscale pictures displaying neutral, sad, and angry faces, derived from the NimStim set (55), including five male and five female faces of White, Black, and Asian individuals, depicting sad, fearful, or neutral expressions. The experiment consisted of six blocks with 48 trials each. Seventy-three percent (35 trials) were Go trials, and 27% (13 trials) were NoGo trials. In each block, one expression (neutral, fearful, sad) was the Go stimulus and another expression was the NoGo stimulus. The order of the blocks was randomized for each participant, while the stimulus order within a block was kept consistent. The stimuli were presented for 1,000 ms with 2,000 ms between stimuli. In between stimuli, a white fixation cross was presented. The children were instructed to press the space bar for each Go stimulus and not to react to NoGo stimuli as fast and with as few mistakes as possible. Reactions were only counted within the stimulus presentation interval of 1,000 ms. In line with the suggestions by Tottenham et al. (54), we calculated D-prime as a measure of accuracy by subtracting z-standardized false alarms (reactions to NoGo stimuli) from z-standardized correct answers (reactions to Go stimuli) for each participant. We also computed mean reaction times for the Go stimuli for each participant across all blocks as a measure for FER speed, excluding reaction times lower than 100 ms.
Morphing tasks are well-established tasks to assess emotion recognition using dynamic facial stimuli. The morphing task included in our study was developed in reference to a morphing task by Schwenck et al. (56). Children watched 48 film clips of 9 s length that started with a neutral facial expression and changed continuously to an emotional expression. The stimuli consisted of 30 different White faces, 15 male and 15 female faces, displaying the emotions anger, sadness, joy, and fear. The faces were sourced from the KDEF database (57). Participants were instructed to press the spacebar on the computer keyboard as soon as they recognized the person’s emotion and to name it out loud to the experimenter afterward. If participants did not press the spacebar during the 9-s-long video, they were encouraged to name or guess the presented emotion. If the children did not name any emotion, the four possible answers were presented on the screen, and children were asked which of those emotions they saw. Experimenters entered the emotion via keystroke. Reaction times of correct trials and accuracy rates were assessed. In a control task, shapes morphed into animals, and children were asked to press the space bar as soon as they recognized the animal and then name the animal they recognized. Reaction times and accuracy rates from this control task were included as covariates in further analyses to ensure possible impairments are specific to identifying emotions.
This task was ad-hoc developed and tested in a pilot study in order to assess emotion recognition as well as compassion, mimicry, and arousal in children while watching emotional video clips. The participants were presented with eight short videos (between 23 and 36 s). Each clip featured a protagonist (a child or teenager) displaying either fear, sadness, happiness, or anger. The video clips were derived from the German children’s TV program. After each clip, participants were asked to a) name the emotion displayed, b) rate their own arousal while watching the video between 1 to 5, and c) rate their compassion for the protagonist on a scale of 1 to 5. After each clip, a marine life clip (approx. 30 s) was presented to avoid carryover effects for the physiological data assessed during the task. When asked to name the presented emotion, participants were aware that the emotion had to be either fear, sadness, happiness, or anger. Participants were given an unlimited amount of time to answer. Accuracy rates were calculated as percentages of correct answers across all trials.
All participants and their parents gave written informed consent before the assessment. Parents received an expense allowance of €50 (COPWMI)/€15 (COPMI). Parents of COPMI were additionally included in the longitudinal intervention study (COMPARE-family) and, therefore, received disorder-specific evidence-based cognitive behavioral treatment after this assessment. Questionnaires were completed by parents online prior to or during the in-person assessment. During the in-person assessment, children were asked to complete three computerized tasks and fill in questionnaires, while the adjoining parent was interviewed by a trained professional regarding their children’s diagnostic status. In a separate appointment, the parents of COPMI completed a clinical interview regarding their own diagnostic status.
Children completed the Go/NoGo task (54), morphing task (56), and a task presenting emotional video clips developed specifically for our study in randomized order. For all computer tasks, children were positioned in a quiet room, in 60 cm distance from a stationary computer screen (24 in., 1,920 × 1,080 pixels). The tasks were presented via the E-Prime software (58).
We performed statistical analyses using SPSS version 28.0 (59) and RStudio version 2023.06.2 (60) along with additional packages brms (61) and bayestestR (62). First, mean reaction times were computed as the mean of all reaction times across a correct trial. Accuracy rates were computed as the number of correct trials divided by the number of total trials. To address the need for confounding variables in further analyses, we examined possible differences in demographic characteristics between COPMI and COPWMI (see Table 1). In all analyses, age, sex, and SES were included as covariates. Two-level Bayesian hierarchical linear models with group (COPMI vs. COPWMI) as fixed and family (to account for siblings) as random effects were fit using the brms package (61) to test our hypotheses. Models with a normal skew distribution were chosen for all outcome variables, except for reaction times in the morphing task, to account for skewness in the data distribution (63). For each model, 95% credibility intervals (CIs) were calculated, and statistical significance for regression coefficients was identified when the CI did not include zero. We fit separate models for mean accuracy and reaction times in the Go/NoGo task and the morphing task, as well as accuracy in the task presenting emotional video sequences. Models yielding significant effects were split up into single models for each emotion to be able to identify the cause of the effect. Bayes factor (BF) was calculated for each model using the Savage–Dickey ratio via the bayestestR package (62) as a means to assess the relative evidence for the alternative over the null model (64). BF smaller than 1.00 suggests that evidence favors the null hypothesis, while BF greater than 1.00 suggests that evidence favors the postulated alternative hypothesis. Priors in each model were adjusted to account for beliefs about general data distributions as well as effect sizes to ensure the best model fit, taking RMSEA, BIC, bulk-ESS, and tail-ESS into account. To ensure that our sample was large enough to detect possible FER differences, we took several parameters, such as R-hat values, effective sample sizes (bulk- and tail-ESS), and BIC into account. Bayesian methods do not rely on power analysis in the classical/frequentist sense to determine the statistical power/required sample size, but rather the model fit determines whether the results are reliable. Generally, Bayesian models are well equipped to model data even in small samples, as they do not rely on asymptotics (65). However, in small samples, the models become more sensitive to the employed priors (66). To address this prior sensitivity, we compared models with varying priors and compared BIC as well as bulk- and tail-ESS. So, while sample size does have an effect on the Bayes models, we assured that our sample was large enough by ensuring the model fit parameters were very good.
Table 1 Demographic characteristics of the participants and means and standard deviations of psychopathological symptoms of children and parents.
In total, 189 children were included in this study, with 77 COPMI and 112 COPWMI. Children ranged in age from 6 to 16 years (M = 10.60, SD = 2.27), and 50.30% (n = 95) were female children. In the COPMI group, 20 children were siblings; in the COPWMI group, 36 children were siblings. For more detailed demographic characteristics, see Table 1. COPMI and COPWMI did not differ significantly in age and gender or parents’ age and gender. There were significant group differences between the groups in children’s psychopathological symptoms and socioeconomic status (SES; see Table 1). In both groups, most of the parents were mothers (81.80% in the COPMI group and 85.10% in the COPWMI group), but there was no significant difference in gender distribution between the groups (see Table 1). The majority of parental primary diagnoses were depressive disorders (48.1%). On average, parents had M = 1.83 diagnoses (SD = 1.17, range from 1 to 5), and the severity of the primary diagnosis ranged from 4 to 8 according to the DIPS (53) (M = 5.89, SD = .75; ranged between 0 and 8, diagnosis being clinically relevant from 4 and above). For further classifications of primary diagnoses, see Table 2.
Means and standard deviations for each outcome measure are reported separately for both groups (COPMI vs. COPWMI) in Table 3. Bayesian correlational measures between the covariates and the outcome measures can be found in the Supplementary Material. Because in Bayesian modeling, decisions regarding the inclusion of covariates in further analyses are not solely based on correlation measures or coefficients but rather on a holistic consideration of the research question, prior knowledge, and the underlying mechanisms, SES, gender, and age were included in the Bayes models. The model fit for each model indicated that Bayes models including all three variables were the best fit for our data.
Table 3 Means and standard deviations for each task separately for COPMI and COPWMI as well as Bayesian analysis statistics for each outcome variable.
We calculated D-prime per participant for the Go/NoGo task across the four blocks where an emotional expression was set as the Go stimulus. The model for accuracy, measured by D-prime, in the Go/NoGo task was fitted to the data using normal skew family and including a random intercept for family and a population-level effect for group (COPMI vs. COPWMI). The model ran 40,000 Markov chain Monte Carlo (MCMC) iterations per chain across five chains. The model showed good convergence, indicated by R-hat values close to 1 for all parameters. Effective sample sizes (bulk-ESS and tail-ESS) were sufficiently high, indicating reliable parameter estimates. The model indicates an effect for being a COPMI on accuracy in the Go/NoGo task [b = −.197, 95% CI (−.293 to −.101)], namely, we observed a statistically significant decrease in Go/NoGo task accuracy of.197 units for COPMI compared to COPWMI. However, BF indicates that the evidence provided mildly favors the null hypothesis over the postulated alternative hypothesis (BF = .839).
We calculated mean reaction times per participant for the Go/NoGo task across the four blocks where an emotional expression was set as the Go stimulus. The model for reaction times in the Go/NoGo task was fitted to the data using normal skew family and including a random intercept for family and a population-level effect for group. The model ran 40,000 MCMC iterations per chain across five chains. The model showed good convergence, indicated by R-hat values close to 1 for all parameters. Effective sample sizes (bulk-ESS and tail-ESS) were sufficiently high, indicating reliable parameter estimates. The model indicates no effect of being a COPMI on reaction times in the Go/NoGo task [b = −3.876, 95% CI (−21.293 to 14.064)]. Furthermore, BF indicates that the evidence provided strongly favors the null hypothesis over the postulated alternative hypothesis (BF = .027).
Mean accuracy rates were computed for each participant across all emotions. The model for accuracy in the morphing task was fitted to the data using normal skew family and including a random intercept for family and a population-level effect for group. The model ran 40,000 MCMC iterations per chain across five chains. The model showed good convergence, indicated by R-hat values close to 1 for all parameters. Effective sample sizes (bulk-ESS and tail-ESS) were sufficiently high, indicating reliable parameter estimates. The model indicates a small effect of being COPMI on the accuracy in the morphing task [b = −.024, 95% CI (−.045 to −.003)], namely, we observed a statistically significant decrease in morphing task accuracy of.024 units for COPMI compared to COPWMI. However, BF indicates that the evidence provided strongly favors the null hypothesis over the alternative hypothesis (BF = .187). Subsequent models fit for accuracy for each emotion separately did not yield significant results (see Table 4).
Mean reaction times were calculated for each participant across all trials and all emotions. The model for reaction times in the morphing task was fitted to the data using the Gaussian family and including a random intercept for family and a population-level effect for group. The model ran 40,000 MCMC iterations per chain across five chains. The model showed good convergence, indicated by R-hat values close to 1 for all parameters. Effective sample sizes (bulk-ESS and tail-ESS) were sufficiently high, indicating reliable parameter estimates. The model indicates no effect of being COPMI on reaction times in the morphing task [b = .095, 95% CI (−.228 to.417)]. BF indicates that the evidence provided strongly favors the null hypothesis over the alternative hypothesis (BF <.001).
Mean accuracy was calculated for each participant across all video clips. The model for accuracy in the task presenting emotional video sequences was fitted to the data using normal skew family and including a random intercept for family and a population-level effect for group. The model ran 40,000 MCMC iterations per chain across five chains. The model showed good convergence, indicated by R-hat values close to 1 for all parameters. Effective sample sizes (bulk-ESS and tail-ESS) were sufficiently high, indicating reliable parameter estimates. The model indicates no effect of being COPMI on accuracy in a task presenting emotional video sequences [b = .001, 95% CI (−.011 to.014)]. BF indicates that the evidence provided strongly favors the null hypothesis over the alternative hypothesis (BF = .008). For a summary of the parameters of each Bayes model, see Table 3.
Our study aimed to compare FER abilities between COPMI and those without (COPWMI), expanding beyond depression to various parental disorder types. We utilized diverse tasks involving static and dynamic stimuli, considering task influence on outcomes (27). Based on previous findings, we hypothesized that COPMI show FER impairments across all three tasks in accuracy as well as recognition speed in the two tasks, in which RT was measured. Contrasting our expectations, our results did not indicate a general FER deficit in COPMI compared to COPWMI. There was no effect of having a mentally ill parent on recognition speed (measured via reaction times) in either the Go/NoGo task or the morphing task. For accuracy, the models fit for the morphing task and the Go/NoGo task yielded a significant effect: COPMI were significantly less accurate in identifying emotional expressions in both tasks (see Table 3). However, the effect size was small and the Bayes factor calculated for the models was below 1, pointing toward the null hypothesis and suggesting that the effect could be due to noise or random variations. Furthermore, deconstructing the accuracy in the morphing task by fitting models for each emotion separately yielded no significant results (see Table 4).
In summary, our results suggest no effects of having a mentally ill parent on recognition speed and only little effects on accuracy for FER. This does not align with the majority of previous findings. Our divergent result could be based on numerous reasons, of which we consider three to be specifically interesting for future investigation: Firstly, our study exclusively includes parents with a mental disorder acute at the time of assessment, which differed from prior research, often including parents with a history of mental disorders rather than a current diagnosis (6, 21, 32, 42, 43). Research on the development of FER abilities and the influence of a parental mental illness on children suggests that this might make a relevant difference. Research on the influence of parental mental health found children to be especially sensitive to their parent’s mental health during the first years of life (39, 67–69).
In addition, FER abilities start to develop in early infancy (2, 4) and Pascalis et al. (70) argue for a sensitive period for face processing throughout the early years of life. Parental influence on children’s FER abilities is also strongest during infancy because parents are the main interaction partners for their children (12). Because mental illness is also associated with changes in facial expressivity (71–73), this means that COPMI are confronted with deviations in their main interaction partner’s facial expression during a sensitive period.
Taking into account the early development of FER and children being highly sensitive to parental mental health issues in infancy, it is possible that not the parental mental illness itself is associated with FER impairments in children, but rather the timing of the first parental disorder onset might be relevant. Therefore, it is possible that in our sample, we might not see an effect of having a mentally ill parent on FER abilities in COPMI, because the mental illness did not occur at a specific time point in the child’s life. Future research should take this into consideration by assessing FER in COPMI in the context of the timing of the parental mental disorder, optimally through prospective longitudinal studies.
Secondly, the COMPARE project was an at-risk evaluation, aiming to assess COPMI that do not show clinically relevant signs of a mental disorder themselves yet. It could therefore be due to selection bias that we included specifically those children that built up resilience from the parental mental disorder. In this case, those children would not show any FER impairments but would also not be representative of COPMI in general. We assessed internalizing and externalizing behaviors in COPMI, and while levels were significantly heightened in COPMI compared to COPWMI, mean T-scores were still below the clinical cutoff for COPMI, suggesting that they did not display clinically relevant levels of internalizing and/or externalizing behaviors. However, the cross-sectional study design did not allow any further insights, because we are unable to assess possible mental illness in the children’s future.
Lastly, past research mainly focused on children of parents with depression and mood disorders (6, 21, 32, 33, 37, 38, 43). To our knowledge, there is no study to date examining FER in a heterogeneous COPMI sample regarding parental disorder type. However, results found in children of parents with depression cannot necessarily be generalized to other disorders, because disorders have varying etiologies, symptom profiles, and neurobiological underpinnings. It is possible that FER is, in fact, not a transdiagnostic feature but rather only relevant for specific disorders and that the diversity in parental disorders in our sample might have diluted the potential FER effects for specific disorders. This could explain the trend for worse accuracy in COPMI compared to COPWMI in our sample. Future research should take a closer look at this when assessing FER impairments in COPMI by conducting subgroup analyses.
While our study offers valuable insights, it is essential to acknowledge several limitations when interpreting our findings. The most significant limitation lies in the cross-sectional study design. While it allowed for comparing FER abilities between COPMI and COPWMI, it did not allow for prognostic conclusions regarding FER in COPMI. To be able to understand possible risk factors for transgenerational transmission of mental disorders and monitor their role in the onset and course of mental illness, longitudinal research is needed. Furthermore, our assessment paradigms also came with a set of limitations. The accuracy rates, particularly in the task presenting emotional video sequences, displayed strong ceiling effects, suggesting that the tasks may have been too easy for the children to depict a variability in FER abilities. Therefore, the task’s suitability for assessing FER impairments should be reconsidered for future studies, especially since we found a trend of COPMI being less accurate in FER in the other tasks. Future research should adapt this task further to mitigate ceiling effects. To address the issue of skewed data in our study, we employed Bayesian models with normal skew family (63), because they offer greater predictive utility compared to frequentist models using data transformations and, therefore, were the favorable solution in this context. Lastly, our study sample presents some limitations as well. While our sample is representative of the population assessing outpatient mental health services, sample sizes for parental disorder types are not homogeneous and, for some disorders, very small (e.g., n = 2 for feeding and eating disorders), preventing us from performing subgroup analyses to assess FER separately for different parental disorder types. Keeping these limitations in mind, the study still provides essential information for the field of FER in COPMI. Our study was the first to assess FER in a COPMI sample representative for outpatient mental health services. The diagnostic status of parents and children was assessed using state-of-the-art clinical interviews. The results were rated by trained clinicians, which assured proper diagnosis rather than self-report symptomatology. In addition, to our knowledge, this study was the first to assess FER in COPMI using three different tasks varying in their task demands for assessment.
Our results suggest that FER impairments are not a transdiagnostic feature in COPMI. However, these results need to be confirmed by future research, especially including information on the time point of the onset of the parental disorder and taking a closer look at different disorders separately, for example by conducting subgroup analyses. Drawing practical implications from our study, our results suggest that COPMI are a heterogeneous group with many factors interplaying. Individual factors, such as parental disorder type and time point of disorder onset, need to be assessed and considered when developing COPMI interventions.
Data are not available due to ethical/legal/commercial restrictions. Requests to access the datasets should be directed to NW,bmFvbWkud2Vya21hbm5AcHN5Y2hvbC51bmktZ2llc3Nlbi5kZQ==.
The studies involving humans were approved by Lokale Ethik-Kommission des Fachbereichs 06 (LEK-FB06), Justus-Liebig-Universität, Gießen, Germany. The studies were conducted in accordance with the local legislation and institutional requirements. Written informed consent for participation in this study was provided by the participants’ legal guardians/next of kin.
NW: Methodology, Visualization, Writing – original draft, Writing – review & editing, Validation, Data curation, Formal analysis, Investigation. AL: Conceptualization, Investigation, Writing – review & editing. KH: Conceptualization, Data curation, Formal analysis, Investigation, Software, Writing – review & editing, Validation. RuS: Conceptualization, Funding acquisition, Project administration, Resources, Writing – review & editing. SW: Conceptualization, Funding acquisition, Project administration, Resources, Writing – review & editing. HC: Conceptualization, Funding acquisition, Project administration, Resources, Writing – review & editing. MK: Conceptualization, Formal analysis, Funding acquisition, Project administration, Resources, Software, Writing – review & editing. KO: Conceptualization, Funding acquisition, Project administration, Resources, Writing – review & editing. CR: Conceptualization, Funding acquisition, Project administration, Resources, Writing – review & editing. RiS: Conceptualization, Funding acquisition, Project administration, Resources, Writing – review & editing. LW: Conceptualization, Funding acquisition, Project administration, Resources, Writing – review & editing. A-LZ: Conceptualization, Funding acquisition, Project administration, Resources, Writing – review & editing. CS: Conceptualization, Funding acquisition, Project administration, Resources, Supervision, Writing – review & editing.
Study management: Stracke, Gilbert, Eitenmüller; biometry and data management: Awounvo, Kirchner, Klose; clinical study monitoring: Buntrock, Ebert; recruiting center Bielefeld: Schlarb; recruiting center Bochum: Margraf, Schneider, Friedrich, Teismann; recruiting center Gießen: Stark, Metzger; recruiting center Greifswald: Brakemeier, Wardenga, Hauck; recruiting center Landau: Glombiewski, Schröder, Heider; recruiting center Mainz: Jungmann, Witthöft; recruiting center Marburg: Rief, Eitenmüller.
The author(s) declare financial support was received for the research, authorship, and/or publication of this article. This work was supported by the Federal Ministry of Education and Research (BMBF) under Grant # 01GL1748C.
The authors declare that the research was conducted in the absence of any commercial or financial relationships that could be construed as a potential conflict of interest.
The author(s) declared that they were an editorial board member of Frontiers, at the time of submission. This had no impact on the peer review process and the final decision.
All claims expressed in this article are solely those of the authors and do not necessarily represent those of their affiliated organizations, or those of the publisher, the editors and the reviewers. Any product that may be evaluated in this article, or claim that may be made by its manufacturer, is not guaranteed or endorsed by the publisher.
The Supplementary Material for this article can be found online at: https://www.frontiersin.org/articles/10.3389/fpsyt.2024.1366005/full#supplementary-material
Supplementary Table | Bayesian Correlations between outcome measures and covariates including Person Correlation Coefficients and Credibility intervals.
1. Ekman P, Friesen WV. Measuring facial movement. J Nonverbal Behav. (1976) 1:56–75. doi: 10.1007/BF01115465
2. Ford RM, Lobao SN, Macaulay C, Herdman LM. Empathy, theory of mind, and individual differences in the appropriation bias among 4- and 5-year-olds. J Exp Child Psychol. (2011) 110:626–46. doi: 10.1016/j.jecp.2011.06.004
3. Durand K, Gallay M, Seigneuric A, Robichon F, Baudouin J-Y. The development of facial emotion recognition: The role of configural information. J Exp Child Psychol. (2007) 97:14–27. doi: 10.1016/j.jecp.2006.12.001
4. Roth-Hanania R, Davidov M, Zahn-Waxler C. Empathy development from 8 to 16 months: early signs of concern for others. Infant Behav Dev. (2011) 34:447–58. doi: 10.1016/j.infbeh.2011.04.007
5. Schwenck C, Göhle B, Hauf J, Warnke A, Freitag CM, Schneider W. Cognitive and emotional empathy in typically developing children: The influence of age, gender, and intelligence. Eur J Dev Psychol. (2014) 11:63–76. doi: 10.1080/17405629.2013.808994
6. Bilodeau C, Bradwejn J, Koszycki D. Impaired facial affect perception in unaffected children at familial risk for panic disorder. Child Psychiatry Hum Dev. (2015) 46:715–24. doi: 10.1007/s10578–014-0513-z
7. Williams LM, Mathersul D, Palmer DM, Gur RC, Gur RE, Gordon E. Explicit identification and implicit recognition of facial emotions: I. Age effects in males and females across 10 decades. J Clin Exp Neuropsychol. (2009) 31:257–77. doi: 10.1080/13803390802255635
8. Wilmer JB, Germine L, Chabris CF, Chatterjee G, Williams M, Loken E, et al. Human face recognition ability is specific and highly heritable. Proc Natl Acad Sci USA. (2010) 107:5238–41. doi: 10.1073/pnas.0913053107
9. Burley DT, Hobson CW, Adegboye D, Shelton KH, van Goozen SHM. Negative parental emotional environment increases the association between childhood behavioral problems and impaired recognition of negative facial expressions. Dev Psychopathol. (2022) 34:936–45. doi: 10.1017/S0954579420002072
10. Lau JYF, Burt M, Leibenluft E, Pine DS, Rijsdijk F, Shiffrin N, et al. Individual differences in children’s facial expression recognition ability: The role of nature and nurture. Dev Neuropsychol. (2009) 34:37–51. doi: 10.1080/87565640802564424
11. Anokhin AP, Golosheykin S, Heath AC. Heritability of individual differences in cortical processing of facial affect. Behav Genet. (2010) 40:178–85. doi: 10.1007/s10519–010-9337–1
12. Castro VL, Halberstadt AG, Lozada FT, Craig AB. Parents’ Emotion-related beliefs, behaviors, and skills predict children’s recognition of emotion. Infant Child Dev. (2015) 24:1–22. doi: 10.1002/icd.1868
13. Kret ME, Ploeger A. Emotion processing deficits: a liability spectrum providing insight into comorbidity of mental disorders. Neurosci Biobehav Rev. (2015) 52:153–71. doi: 10.1016/j.neubiorev.2015.02.011
14. Ehring T, Fischer S, Schnülle J, Bösterling A, Tuschen-Caffier B. Characteristics of emotion regulation in recovered depressed versus never depressed individuals. Pers Individ Differ. (2008) 44:1574–84. doi: 10.1016/j.paid.2008.01.013
15. Bell C, Bourke C, Colhoun H, Carter F, Frampton C, Porter R. The misclassification of facial expressions in generalised social phobia. J Anxiety Disord. (2011) 25:278–83. doi: 10.1016/j.janxdis.2010.10.001
16. Yoon KL, Joormann J, Gotlib IH. Judging the intensity of facial expressions of emotion: depression-related biases in the processing of positive affect. J Abnorm Psychol. (2009) 118:223–8. doi: 10.1037/a0014658
17. van ‘t Wout M, Aleman A, Bermond B, Kahn RS. No words for feelings: alexithymia in schizophrenia patients and first-degree relatives. Compr Psychiatry. (2007) 48:27–33. doi: 10.1016/j.comppsych.2006.07.003
18. Mitchell AE, Dickens GL, Picchioni MM. Facial emotion processing in borderline personality disorder: a systematic review and meta-analysis. Neuropsychol Rev. (2014) 24:166–84. doi: 10.1007/s11065–014-9254–9
19. Cooper S, Hobson CW, van Goozen SH. Facial emotion recognition in children with externalising behaviours: A systematic review. Clin Child Psychol Psychiatry. (2020) 25:1068–85. doi: 10.1177/1359104520945390
20. Hosman CMH, van Doesum KTM, van Santvoort F. Prevention of emotional problems and psychiatric risks in children of parents with a mental illness in the Netherlands: I. The scientific basis to a comprehensive approach. Aust e-Journal Advancement Ment Health. (2009) 8:250–63. doi: 10.5172/jamh.8.3.250
21. Mannie ZN, Bristow GC, Harmer CJ, Cowen PJ. Impaired emotional categorisation in young people at increased familial risk of depression. Neuropsychologia. (2007) 45:2975–80. doi: 10.1016/j.neuropsychologia.2007.05.016
22. van Zonneveld L, de Sonneville L, van Goozen S, Swaab H. Recognition of facial emotion and affective prosody in children at high risk of criminal behavior. J Int Neuropsychol Soc. (2019) 25:57–64. doi: 10.1017/S1355617718000796
23. Wells AE, Hunnikin LM, Ash DP, van Goozen SHM. Children with behavioural problems misinterpret the emotions and intentions of others. J Abnorm Child Psychol. (2020) 48:213–21. doi: 10.1007/s10802–019-00594–7
24. Ambadar Z, Schooler JW, Cohn JF. Deciphering the enigmatic face: the importance of facial dynamics in interpreting subtle facial expressions. Psychol Sci. (2005) 16:403–10. doi: 10.1111/j.0956–7976.2005.01548.x
25. Darke H, Cropper SJ, Carter O. A novel dynamic morphed stimuli set to assess sensitivity to identity and emotion attributes in faces. Front Psychol. (2019) 10:757. doi: 10.3389/fpsyg.2019.00757
26. Herba C, Phillips M. Annotation: Development of facial expression recognition from childhood to adolescence: behavioural and neurological perspectives. J Child Psychol Psychiatry. (2004) 45:1185–98. doi: 10.1111/j.1469–7610.2004.00316.x
27. Hayes GS, McLennan SN, Henry JD, Phillips LH, Terrett G, Rendell PG, et al. Task characteristics influence facial emotion recognition age-effects: A meta-analytic review. Psychol Aging. (2020) 35:295–315. doi: 10.1037/pag0000441
28. Phan KL, Wager T, Taylor SF, Liberzon I. Functional neuroanatomy of emotion: a meta-analysis of emotion activation studies in PET and fMRI. Neuroimage. (2002) 16:331–48. doi: 10.1006/nimg.2002.1087
29. Wiegand-Grefe S, Geers P, Plaß A, Petermann F, Riedesser P. Kinder psychisch kranker Eltern: Zusammenhänge zwischen subjektiver elterlicher Beeinträchtigung und psychischer Auffälligkeit der Kinder aus Elternsicht. Kindheit und Entwicklung. (2009) 18:111–21. doi: 10.1026/0942–5403.18.2.111
30. Mattejat F, Remschmidt H. The children of mentally ill parents. Dtsch Arztebl Int. (2008) 105:413–8. doi: 10.3238/arztebl.2008.0413
31. Lenz A. Kinder psychisch kranker Eltern stärken: Informationen zur Förderung von Resilienz in Familie, Kindergarten und Schule. Göttingen, Germany: Hogrefe Verlag GmbH & Co. KG (2022).
32. Joormann J, Gilbert K, Gotlib IH. Emotion identification in girls at high risk for depression. J Child Psychol Psychiatry. (2010) 51:575–82. doi: 10.1111/j.1469–7610.2009.02175.x
33. Székely E, Lucassen N, Tiemeier H, Bakermans-Kranenburg MJ, van Ijzendoorn MH, Kok R, et al. Maternal depressive symptoms and sensitivity are related to young children’s facial expression recognition: the Generation R Study. Dev Psychopathol. (2014) 26:333–45. doi: 10.1017/S0954579413001028
34. Meiser S, Zietlow A-L, Reck C, Träuble B. The impact of postpartum depression and anxiety disorders on children’s processing of facial emotional expressions at pre-school age. Arch Womens Ment Health. (2015) 18:707–16. doi: 10.1007/s00737–015-0519-y
35. Creswell C, Woolgar M, Cooper P, Giannakakis A, Schofield E, Young AW, et al. Processing of faces and emotional expressions in infants at risk of social phobia. Cogn Emotion. (2008) 22:437–58. doi: 10.1080/02699930701872392
36. Pine DS, Klein RG, Mannuzza S, Moulton JL, Lissek S, Guardino M, et al. Face-emotion processing in offspring at risk for panic disorder. J Am Acad Child Adolesc Psychiatry. (2005) 44:664–72. doi: 10.1097/01.chi.0000162580.92029.f4
37. Hanford LC, Sassi RB, Hall GB. Accuracy of emotion labeling in children of parents diagnosed with bipolar disorder. J Affect Disord. (2016) 194:226–33. doi: 10.1016/j.jad.2016.01.031
38. Sharma AN, Barron E, Le Couteur J, Close A, Rushton S, Grunze H, et al. Facial emotion labeling in unaffected offspring of adults with bipolar I disorder. J Affect Disord. (2017) 208:198–204. doi: 10.1016/j.jad.2016.10.006
39. Lussier AA, Zhu Y, Smith BJ, Cerutti J, Fisher J, Melton PE, et al. Association between the timing of childhood adversity and epigenetic patterns across childhood and adolescence: findings from the Avon Longitudinal Study of Parents and Children (ALSPAC) prospective cohort. Lancet Child Adolesc Health. (2023) 7:532–43. doi: 10.1016/S2352–4642(23)00127-X
40. Horton LE, Bridgwater MA, Haas GL. Emotion recognition and social skills in child and adolescent offspring of parents with schizophrenia. Cogn Neuropsychiatry. (2017) 22:175–85. doi: 10.1080/13546805.2017.1297223
41. Castro-Vale I, Severo M, Carvalho D. Lifetime PTSD is associated with impaired emotion recognition in veterans and their offspring. Psychiatry Res. (2020) 284:112666. doi: 10.1016/j.psychres.2019.112666
42. Lopez-Duran NL, Kuhlman KR, George C, Kovacs M. Facial emotion expression recognition by children at familial risk for depression: high-risk boys are oversensitive to sadness. J Child Psychol Psychiatry. (2013) 54:565–74. doi: 10.1111/jcpp.12005
43. Burkhouse KL, Woody ML, Owens M, McGeary JE, Knopik VS, Gibb BE. Sensitivity in detecting facial displays of emotion: Impact of maternal depression and oxytocin receptor genotype. Cogn Emotion. (2016) 30:275–87. doi: 10.1080/02699931.2014.996531
44. Joormann J, Talbot L, Gotlib IH. Biased processing of emotional information in girls at risk for depression. J Abnorm Psychol. (2007) 116:135–43. doi: 10.1037/0021–843X.116.1.135
45. Callaghan BL, Tottenham N. The Stress Acceleration Hypothesis: Effects of early-life adversity on emotion circuits and behavior. Curr Opin Behav Sci. (2016) 7:76–81. doi: 10.1016/j.cobeha.2015.11.018
46. Stracke M, Gilbert K, Kieser M, Klose C, Krisam J, Ebert DD, et al. COMPARE family (Children of mentally ill parents at risk evaluation): A study protocol for a preventive intervention for children of mentally ill parents (Triple P, evidence-based program that enhances parentings skills, in addition to gold-standard CBT with the mentally ill parent) in a multicenter RCT-part II. Front Psychiatry. (2019) 10:54. doi: 10.3389/fpsyt.2019.00054
47. Christiansen H, Reck C, Zietlow A-L, Otto K, Steinmayr R, Wirthwein L, et al. Children of mentally III parents at risk evaluation (COMPARE): design and methods of a randomized controlled multicenter study-part I. Front Psychiatry. (2019) 10:128. doi: 10.3389/fpsyt.2019.00128
48. American Psychiatric Association. Diagnostic and statistical manual of mental disorders: DSM-5. 5th ed. Washington (D. C.), London (England: American Psychiatric Association, American Psychiatric Publishing (2013).
49. Lange M, Kamtsiuris P, Lange C, Schaffrath Rosario A, Stolzenberg H, Lampert T. Messung soziodemographischer Merkmale im Kinder- und Jugendgesundheitssurvey (KiGGS) und ihre Bedeutung am Beispiel der Einschätzung des allgemeinen Gesundheitszustands. [Sociodemographic characteristics in the German Health Interview and Examination Survey for Children and Adolescents (KiGGS) - operationalisation and public health significance, taking as an example the assessment of general state of health]. Bundesgesundheitsblatt Gesundheitsforschung Gesundheitsschutz. (2007) 50:578–89. doi: 10.1007/s00103–007-0219–5
50. Lampert T, Hoebel J, Kuntz B, Muters S, Kroll LE. Messung des soziookonomischen Status und des subjektiven sozialen Status in KiGGS Welle 2. J Health Monitoring. (2018) 3:114–33.
51. Döpfner M, Plück J, Kinnen C. für die arbeitsgruppe Deutsche Child Behavior Checklist. In: Manual deutsche Schulalter-Formen der Child Behavior Checklist von Thomas M. Achenbach. Elternfragebogen über das Verhalten von Kindern und Jugendlichen. CBCL/6–18R), Lehrerfragebogen über das Verhalten von Kindern und Jugendlichen (TRF/6–18R), Fragebogen für Jugendliche (YSR/11–18R). Hogrefe, Göttingen (2014).
52. Derogatis LR. BSI: Brief Symtom Inventory: Manual. Kliem S, Brahler E, editors. Deutsche Fassung: Pearson (2017).
53. Margraf J, Cwik JC, Suppiger A, Schneider S. Diagnostic Interview for Mental Disorders. [DIPS Open Access: Diagnostisches Interview bei psychischen Störungen]. Bochum. (2017). doi: 10.13154/rub.100.89
54. Tottenham N, Hare TA, Casey BJ. Behavioral assessment of emotion discrimination, emotion regulation, and cognitive control in childhood, adolescence, and adulthood. Front Psychol. (2011) 2:39. doi: 10.3389/fpsyg.2011.00039
55. Tottenham N, Tanaka JW, Leon AC, McCarry T, Nurse M, Hare TA, et al. The NimStim set of facial expressions: judgments from untrained research participants. Psychiatry Res. (2009) 168:242–9. doi: 10.1016/j.psychres.2008.05.006
56. Schwenck C, Mergenthaler J, Keller K, Zech J, Salehi S, Taurines R, et al. Empathy in children with autism and conduct disorder: group-specific profiles and developmental aspects. J Child Psychol Psychiatry. (2012) 53:651–9. doi: 10.1111/j.1469–7610.2011.02499.x
57. Lundqvist D, Flykt A, Öhman A. Karolinska directed emotional faces. PsycTESTS Dataset. (1998) 91:630. doi: 10.1037/t27732–000
58. Psychology Software Tools, Inc. [E-Prime 3.0]. (2016). Available at: https://support.pstnet.com/.
61. Bürkner P-C. Bayesian item response modeling in R with brms and stan. J Stat Soft. (2021). doi: 10.18637/jss.v100.i05
62. Makowski D, Ben-Shachar M, Lüdecke D. bayestestR: Describing Effects and their Uncertainty, Existence and Significance within the Bayesian Framework. JOSS. (2019) 4:1541. doi: 10.21105/joss.01541
63. Martin SR, Williams DR. Outgrowing the procrustean bed of normality: the utility of bayesian modeling for asymmetrical data analysis: center for open science. (2017). doi: 10.31234/osf.io/26m49
64. Makowski D, Ben-Shachar MS, Chen SHA, Lüdecke D. Indices of effect existence and significance in the bayesian framework. Front Psychol. (2019) 10:2767. doi: 10.3389/fpsyg.2019.02767
65. McNeish D. On using bayesian methods to address small sample problems. Struct Equation Modeling: A Multidiscip J. (2016) 23:750–73. doi: 10.1080/10705511.2016.1186549
66. Smid SC, McNeish D, Miočević M, van de Schoot R. Bayesian versus frequentist estimation for structural equation models in small sample contexts: A systematic review. Struct Equation Modeling: A Multidiscip J. (2020) 27:131–61. doi: 10.1080/10705511.2019.1577140
67. Kuramoto SJ, Runeson B, Stuart EA, Lichtenstein P, Wilcox HC. Time to hospitalization for suicide attempt by the timing of parental suicide during offspring early development. JAMA Psychiatry. (2013) 70:149–57. doi: 10.1001/jamapsychiatry.2013.274
68. Ranning A, Uddin MJ, Sørensen HJ, Laursen TM, Thorup AAE, Madsen T, et al. Intergenerational transmission of suicide attempt in a cohort of 4.4 million children. Psychol Med. (2022) 52:3202–9. doi: 10.1017/S0033291720005310
69. Schalinski I, Teicher MH, Nischk D, Hinderer E, Müller O, Rockstroh B. Type and timing of adverse childhood experiences differentially affect severity of PTSD, dissociative and depressive symptoms in adult inpatients. BMC Psychiatry. (2016) 16:295. doi: 10.1186/s12888–016-1004–5
70. Pascalis O, Fort M, Quinn PC. Development of face processing: are there critical or sensitive periods? Curr Opin Behav Sci. (2020) 36:7–12. doi: 10.1016/j.cobeha.2020.05.005
71. Harrigan JA, Wilson K, Rosenthal R. Detecting state and trait anxiety from auditory and visual cues: a meta-analysis. Pers Soc Psychol Bull. (2004) 30:56–66. doi: 10.1177/0146167203258844
72. Peham D, Bock A, Schiestl C, Huber E, Zimmermann J, Kratzer D, et al. Facial affective behavior in mental disorder. J Nonverbal Behav. (2015) 39:371–96. doi: 10.1007/s10919–015-0216–6
Keywords: transgenerational transmission of mental disorders, facial emotion recognition, parents with mental illness, children of parents with mental illness (COPMI), multimodal assessment
Citation: Werkmann NL, Luczejko AA, Hagelweide K, Stark R, Weigelt S, Christiansen H, Kieser M, Otto K, Reck C, Steinmayr R, Wirthwein L, Zietlow A-L, Schwenck C and the COMPARE-family research group (2024) Facial emotion recognition in children of parents with a mental illness. Front. Psychiatry 15:1366005. doi: 10.3389/fpsyt.2024.1366005
Received: 05 January 2024; Accepted: 23 May 2024;
Published: 13 June 2024.
Edited by:
Anja Wittkowski, The University of Manchester, United KingdomCopyright © 2024 Werkmann, Luczejko, Hagelweide, Stark, Weigelt, Christiansen, Kieser, Otto, Reck, Steinmayr, Wirthwein, Zietlow, Schwenck and the COMPARE-family research group. This is an open-access article distributed under the terms of the Creative Commons Attribution License (CC BY). The use, distribution or reproduction in other forums is permitted, provided the original author(s) and the copyright owner(s) are credited and that the original publication in this journal is cited, in accordance with accepted academic practice. No use, distribution or reproduction is permitted which does not comply with these terms.
*Correspondence: Naomi Leona Werkmann, bmFvbWkud2Vya21hbm5AcHN5Y2hvbC51bmktZ2llc3Nlbi5kZQ==
Disclaimer: All claims expressed in this article are solely those of the authors and do not necessarily represent those of their affiliated organizations, or those of the publisher, the editors and the reviewers. Any product that may be evaluated in this article or claim that may be made by its manufacturer is not guaranteed or endorsed by the publisher.
Research integrity at Frontiers
Learn more about the work of our research integrity team to safeguard the quality of each article we publish.