- 1First Clinical Medical College, Shandong University of Traditional Chinese Medicine, Jinan, Shandong, China
- 2Key Laboratory of Basic and Application Research of Beiyao, Heilongjiang University of Chinese Medicine, Harbin, Heilongjiang, China
- 3Department of Neurology, Affiliated Hospital of Shandong University of Traditional Chinese Medicine, Jinan, Shandong, China
Background: Extensive observational evidence suggests an association between psychiatric disorders (PDs) and obstructive sleep apnea (OSA), but their causal relationship remains unexplored. The objective of this study was to examine the causal relationship between PDs and OSA.
Methods: Mendelian randomization (MR) analysis was conducted with summary genetic data from the FinnGen and Psychiatric Genomics Consortium (PGC). Inverse-variance weighted (IVW), MR-Egger, weighted median, and weighted mode methods were employed to ascertain causal influence. Sensitivity analysis employing various methodologies assessed the robustness of the findings. Furthermore, multivariable Mendelian randomization (MVMR) was used to clarify if the exposures independently caused OSA.
Results: MR analysis showed that genetically determined major depressive disorder (MDD) increased the risk of OSA (IVW odds ratio [OR]: 1.377, 95% confidence interval [CI]: 1.242–1.526, P = 1.05×10-9). Sensitivity analysis showed no evidence of pleiotropy and heterogeneity. In MVMR, the significant association persisted after adjusting for BMI, smoking, and alcohol consumption. No conclusive evidence indicated the causal impact of other psychological characteristics on OSA. In the reverse MR analyses, there was no causal effect of OSA on PDs.
Conclusion: This study suggests a causal effect of MDD on OSA risk. Further research is needed to confirm these findings and understand how MDD contributes to OSA development, potentially aiding in reducing OSA incidence.
1 Introduction
Obstructive sleep apnea (OSA) is a prevalent chronic sleep disorder characterized by recurring episodes of partial or complete collapse of the upper airway during sleep. This leads to intermittent hypoxia, sympathetic overactivity, sleep fragmentation, and disruption of physiological homeostasis (1). The prevalence of OSA varies, affecting 9% to 38% of the general population, with higher rates observed in men (13%-33%) compared to women (6-19%) (2). Numerous studies have established a link between OSA and an increased risk of several metabolic and cardiovascular conditions, including coronary heart disease, type 2 diabetes mellitus, hypertension, and stroke (3). Therefore, understanding OSA’s pathophysiology and potential risk factors is critical for developing novel prevention and intervention strategies.
Psychiatric disorders (PDs), encompassing a range of complex cognitive psychological syndromes, have emerged as a major public health concern with significant morbidity and mortality rates. These disorders are estimated to affect about 22.1% of the global population (4). The potential link between PDs and OSA has recently garnered increasing research interest (5–7). Numerous studies indicate a high prevalence of OSA in patients with psychiatric conditions (5, 8, 9), with elevated rates of OSA observed explicitly in patients with post-traumatic stress disorder (PTSD) and bipolar disorder (BIP) compared to the general population (10–12). Conversely, increased incidences of PDs are reported in individuals with OSA (13, 14), with depression and anxiety disorder (ANX) being more common in OSA patients than in non-OSA counterparts (15, 16). Moreover, several studies suggest that treatment of OSA with continuous positive airway pressure (CPAP) can lead to improvements in both OSA symptoms and psychiatric comorbidities like depression and PTSD (17–19). However, accurately diagnosing PDs in OSA patients is challenging due to the overlap of symptoms such as excessive daytime sleepiness, cognitive impairment, and mood changes (7). Additionally, factors like alcohol consumption, smoking, and body mass index (BMI) can confound the relationship between OSA and PDs (20, 21), leading to potential biases in observational studies. Therefore, the causality between OSA and PDs remains ambiguous and requires further exploration.
MR is an epidemiological method that employs genetic variants strongly associated with exposure as instrumental variables (IVs) to elucidate causal relationships between risk factors and outcomes (22). Due to the random inheritance of genetic variations and their stability post-pregnancy, MR minimizes the influence of external factors on causality, significantly reducing potential biases and reverse causation. This strengthens the robustness of causal inferences, addressing limitations commonly associated with observational studies (23). Widely applied to validate findings from observational research (24), MR was employed in this study to conduct a two-sample bidirectional analysis. This analysis aimed to investigate the causal associations between five PDs—MDD, PTSD, BIP, ANX, and schizophrenia (SCZ)—and OSA.
2 Materials and methods
2.1 Study design
A two-sample univariable bidirectional MR analysis was initially performed to investigate the causal effects between PDs and OSA. Recognizing that BMI, smoking, and alcohol consumption are significant risk factors for OSA development, multivariable MR (MVMR) analyses were subsequently conducted for outcomes causally linked to OSA. These analyses aimed to ascertain whether the exposures have independent causal effects on OSA. To ensure the reliability of MR results, three critical assumptions must be met: (i) the IVs must be strongly correlated with the exposures, (ii) the IVs should not be significantly associated with confounding factors, and (iii) the IVs must influence the outcomes solely through the exposures (25). The research design is depicted in Figure 1, created using Figdraw (www.figdraw.com).
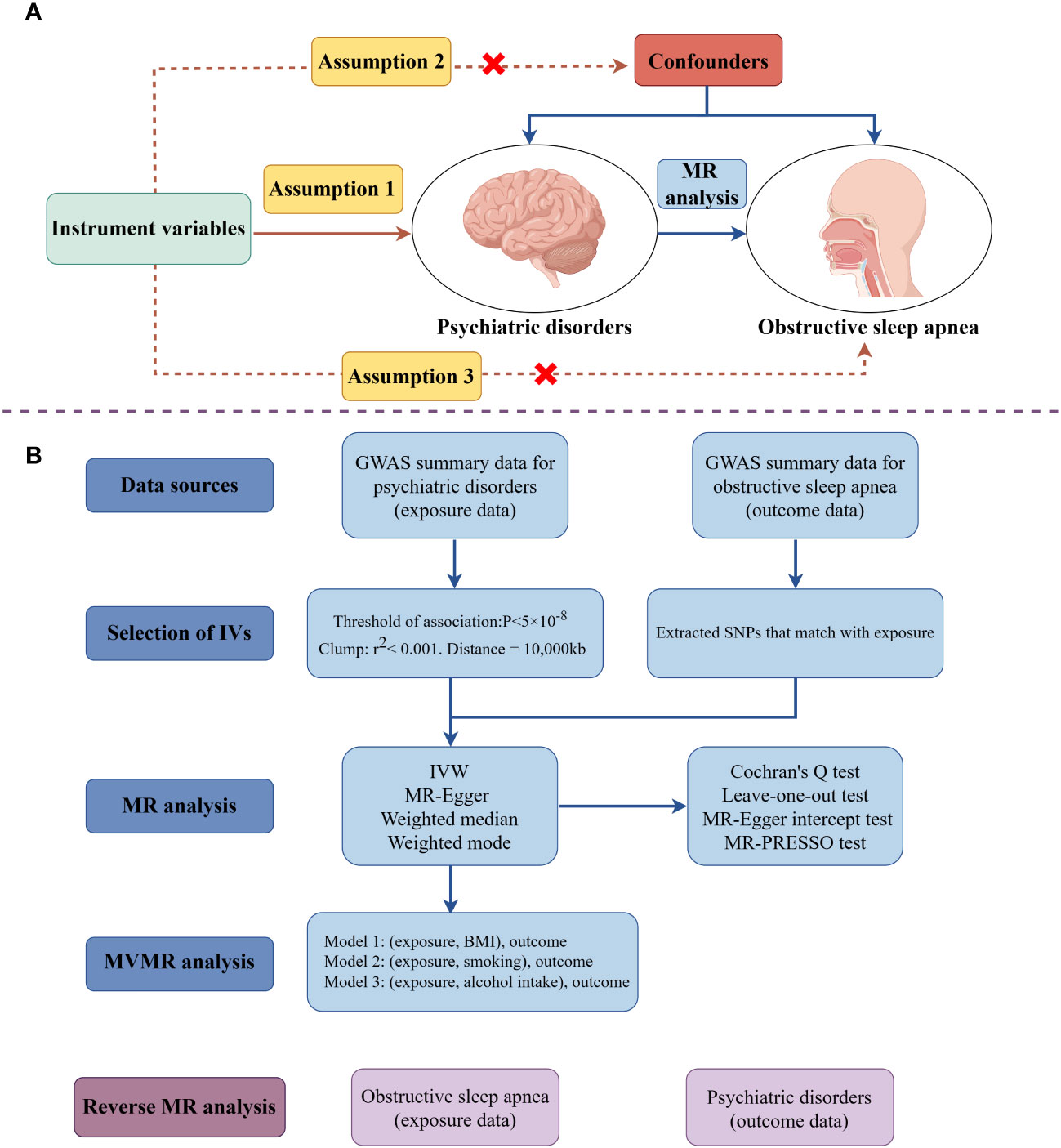
Figure 1 The schematic illustrates the causal relationship between psychiatric disorders and obstructive sleep apnea through MR analyses (drawing by Figdraw). (A) Principles of Mendelian Randomization; (B) The flowchart of the MR analysis. (MR, Mendelian randomization; MVMR, multivariable Mendelian randomization; SNPs, single- nucleotide polymorphisms; IVs, instrument variables; IVW, inverse-variance weighted; BMI, body mass index; GWAS, genome-wide association study).
2.2 Data source
Genome-wide association studies (GWAS) aim to identify relationships between differences in DNA sequences and specific traits by examining individuals with diverse phenotypes and analyzing their genotypes at several single-nucleotide polymorphisms (SNPs) locations (26). As data-sharing has advanced, GWAS has discovered several significant variants related to PDs and OSA. These variations provide valuable IVs for conducting a robust MR analysis to investigate the causal relationship between PDs and OSA. To mitigate the risk of pleiotropic bias in cross-lineage cases, this study incorporated publicly available GWAS results of five PDs, and OSA conducted within a European population.
The most recent GWAS data for OSA was obtained from the FinnGen (27), which included a total of 38,998 patients and 336,659 controls. The patients are identified using the Finnish National Hospital Discharge Registry and the Causes of Death Registry. OSA was diagnosed depending on the International Statistical Classification of Diseases and Related Health Problems, 9th edition (ICD-9: 3472A) and 10th edition (ICD-10: G47.3), which are based on subjective symptoms, clinical examination, and sleep registration, utilizing the apnea-hypopnea index of five per hour or the respiratory event index of five per hour. All association tests for all sources were adjusted for principal variables, including age and sex. The Psychiatric Genomics Consortium (PGC) provided summarized GWAS data on the five PDs:
The GWAS data for MDD was obtained from Howard DM et al.’s GWAS meta-analysis (28). It consists of PGC, UK Biobank, and 23andMe, three large-scale GWAS. We included the complete summary statistics from 2 cohorts to conduct bi-directional MR analysis because, among these three GWAS, the summary statistics for all examined genetic variants were only publically available for UK Biobank and PGC (which contains 170,756 cases and 344,901 controls). Howard DM et al. employed the inclusive criteria for MDD within UK Biobank, which was determined based on participants’ answers to the questions ‘Have you ever sought medical help from a general practitioner or psychiatrist for nerves, anxiety, tension, or depression?’ MDD was diagnosed in PGC cohorts using internationally recognized criteria, such as DSM-IV, ICD-9, or ICD-10.
The GWAS data for ANX were obtained from the GWAS meta-analysis conducted by Otowa T et al. (29), which included 7 distinct studies with a combined sample size of 5580 cases and 11730 controls. The identification of ANX primarily relied on the Diagnostic and Statistical Manual of Mental Disorders (DSM) and utilized standardized evaluation tools (30).
The GWAS data for BIP were obtained from a meta-analysis of 57 cohorts from Europe, North America, and Australia undertaken by Mullins N et al. (31). These cohorts included a total of 41,917 cases and 371,549 controls in European heritage. Cases that satisfied the global consensus criteria (DSM-IV, ICD-9, or ICD-10) for BIP were determined through formal diagnostic interviews, clinician checklists, or medical records reviews.
The largest GWAS meta-analysis, carried out by Trubetskoy V et al. (32), yielded the GWAS data for SCZ. The SCZ samples were from several SCZ studies. The DSM-IV or ICD-10 diagnostic criteria were used to make the diagnoses. For the purpose of performing a bi-directional MR study, we only included the SCZ meta-analysis in participants of European ancestry. This analysis included 53,386 cases and 77,258 controls.
The GWAS data for PTSD were obtained from the GWAS meta-analysis of an ancestrally diverse group from 60 different PTSD studies carried out by Nievergelt CM et al. (33), ranging from large cohorts with self-reported PTSD symptoms to clinically deeply characterized small patient groups. The diagnosis of PTSD was determined using a variety of instruments and DSM versions (DSM-III-R, DSM-IV, DSM-5). To conduct a bi-directional MR analysis, we exclusively included the PTSD meta-analysis in participants of European ancestry, which included 23,212 cases and 151,447 controls.
Additionally, summary-level data for BMI, smoking, and alcohol intake were acquired from corresponding publicly available GWAS. The Genetic Investigation of Anthropometric Traits (GIANT) Consortium, which includes 681,275 samples with European ancestry, provided the GWAS data for BMI (34). The GWAS data on alcohol consumption and smoking initiation were taken from the GWAS meta-analysis conducted by the Sequencing Consortium of Alcohol and Nicotine Use (GSCAN) and the GWAS, which included up to 1.2 million people with European ancestry (35). The binary phenotype of smoking initiation indicates whether a person has ever smoked regularly throughout their life. The average number of drinks a person reported having each week, regardless of the type of alcohol, was used to characterize alcohol consumption. As all original studies were public, anonymized, and de-identified, ethical approval did not apply to this study. Table 1 provides further details about the GWAS dataset included in this analysis.
2.3 Instrument variables selection
A specific methodology was employed to select IVs to adhere to the three core assumptions of MR analysis. Genome-wide significant SNPs (P<5×10−8) were initially extracted from exposure GWAS. For the GWAS of ANX and PTSD, suggestively significant SNPs (P <5×10−6) were also used as IVs to ensure comprehensive results. Subsequently, linkage disequilibrium (LD) clumping (R2 < 0.001, clumping distance = 10,000 kb), based on the European 1,000 Genomes Project reference panel, was performed to select independent significant SNPs. The harmonization process involved removing palindrome SNPs with intermediate or incompatible allele frequencies. The statistical strength of each SNP was then evaluated by calculating the F-statistic (beta2/se2) (36, 37), with an F-statistic > 10 indicating the absence of bias due to weak IVs. Additionally, MR pleiotropy residual sum and outlier (MR-PRESSO) test analysis were conducted to eliminate outlier IVs. A stringent filtering step was adopted, discarding SNPs with a P-value < 1 in the outlier test and repeating this process until no outliers remained (38). Finally, the Steiger test was applied to each SNP to ascertain if the R2 of the exposure exceeded that of the outcome, excluding SNPs where the test indicated a ‘FALSE’ direction.
2.4 Univariable MR analyses
This study used the inverse-variance weighted (IVW) as the primary analytical approach to assess the causal relationship between PDs and OSA. This method aggregates Wald ratio estimates for each instrumental SNP using a meta-analysis-like model to derive overall effect estimates (39). Given that IVW yields unbiased causal estimates only when all IVs are valid, additional methods were employed to ensure accuracy and stability in cases of assumption violations. These methods included weighted mode, weighted median, and MR-Egger. The weighted median method can offer a consistent estimate even if up to 50% of the IVs are invalid, albeit with reduced statistical power (40). Under the Instrument Strength Independent of Direct Effect (InSIDE) assumption, MR-Egger regression allows all genetic variants to be potentially invalid IVs. It provides an intercept term for estimating average pleiotropic effects across genetic variants (41). Despite stringent control measures, the MR-PRESSO method might still detect horizontal pleiotropy in the global test, potentially due to InSIDE assumption violations (42). Therefore, if the MR-PRESSO international test detected pleiotropy or if the MR-Egger intercept’s P-value was below 0.05, results from the weighted median method were prioritized. The weighted mode approach is applicable even when most IVs do not fulfill the causal inference prerequisites in MR as long as most IVs yield consistent causal estimations (41).
2.5 Sensitivity analysis
A series of essential sensitivity assessments were conducted to evaluate the results’ reliability and stability. The Cochran Q statistic (MR-IVW) was employed to assess heterogeneity among the effect sizes derived from the selected genetic IVs (43). A P-value greater than 0.05 was indicative of negligible heterogeneity. The MR-PRESSO method and MR-Egger intercept were then applied to detect horizontal pleiotropy (42). Furthermore, a leave-one-out analysis addressed potential biases due to horizontal pleiotropy arising from any SNP. For multiple testing adjustments, a Bonferroni-corrected P-value threshold was set at 0.05/5, while a P < 0.05 was considered nominally significant. Additionally, reverse MR analysis was conducted to explore the possibility of reverse causality, precisely the effect of OSA on PDs.
2.6 Multivariable MR analyses
Two distinct methodologies were implemented to mitigate the influence of potential confounders. The first step involved the identification and exclusion of pleiotropic SNPs associated with known confounders like BMI, smoking, and alcohol consumption. This identification was conducted using the PhenoScanner V2 database (44). After removing SNPs linked to these confounding variables, a reanalysis was carried out using the remaining IVs. Additionally, MVMR analysis was used. An improved version of MR named MVMR enables the simultaneous analysis of several genetic variations linked to various exposures (45). This method is advantageous in discerning whether the exposures under study have independent effects on the outcome, in this case, OSA. In the second approach, MVMR analysis was explicitly used to determine if these exposures had independent causal effects on OSA. These analyses were conducted using various packages in R Software 4.3.0, including ‘TwoSampleMR’ (version 0.5.7), ‘MendelianRandomization’ (version 0.8.0), and ‘MRPRESSO’ (version 1.0).
3 Results
3.1 Selection of IVs
A thorough quality control process led to the selection of 41, 6, 38, 137, and 20 SNPs as IVs for MDD, ANX, BIP, SCZ, and PTSD, respectively, to evaluate their causal effect on OSA. Conversely, in the reverse MR analysis, 11, 14, 14, 16, and 18 SNPs were chosen as IVs for OSA to assess its causal impact on the five PDs. The F-statistic for each selected SNP was greater than 10, indicating the absence of weak instrument bias. Detailed information about the IVs ultimately included in our MR analysis can be found in Supplementary Tables S1–S10.
3.2 Causal effect of PDs on OSA
The univariable MR analysis revealed that genetically predicted MDD was associated with an increased risk of OSA (IVW OR: 1.377, 95% CI: 1.242–1.526, P = 1.05×10-9). This association was corroborated by the weighted median method (OR: 1.301, 95% CI: 1.135–1.491, P = 1.576×10-4). Although MR-Egger and weighted mode methods provided consistent estimates, they were not statistically significant. No heterogeneity was observed in Cochran’s Q test (P = 0.084), and both the MR-Egger intercept (P = 0.480) and MR-PRESSO test (P = 0.114) indicated an absence of significant directional pleiotropy for the IVs. Leave-one-out analyses further confirmed that individual SNPs did not influence the causal relationship. For SCZ, despite stringent controls and no outliers detected pre-MR analysis, the MR-PRESSO global test indicated pleiotropy among IVs (P = 0.037), suggesting unreliability of causation estimates from IVW and MR-Egger due to violation of the InSIDE assumption (38). However, estimates from the weighted median method were also non-significant (OR: 0.997, 95% CI: 0.964–1.032, P = 0.884). MR analysis indicated no causal effect of genetically predicted ANX, BIP, and PTSD on OSA. Cochran’s Q test detected no heterogeneities except for PTSD (P = 0.042), and no notable directional pleiotropies were observed in the MR-Egger intercept and MR-PRESSO test. Detailed results of the causal effects of PDs on OSA, including outcomes of the four causal estimation methods, pleiotropy tests, and heterogeneity tests, are presented in Table 2. The scatter, funnel, and forest plots are shown in Supplementary Figure S1.
3.3 Causal effect of OSA on PDs
To elucidate the precise causal relationship between PDs and OSA, a reverse MR analysis was conducted, positioning OSA as the exposure and PDs as the outcomes. However, no significant causal effects of OSA were found on five PDs across all MR methods. Additionally, no heterogeneities or directional pleiotropies were observed in the reverse MR analysis. Detailed results of the causal effects of OSA on PDs, encompassing outcomes from the four causal estimation methods, pleiotropy tests, and heterogeneity tests, are provided in Supplementary Table S11. The associated scatter and forest plots are illustrated in Supplementary Figure S2.
3.4 Results of multivariable MR analysis
Employing the online tool PhenoScanner V2, the study identified that rs10235664, rs17641524, rs2568958, rs30266, and rs9831648 correlated with BMI, while rs2232423 and rs4799949 linked to smoking, and rs4936276 and rs66511648 were associated with alcohol consumption. Upon excluding these SNPs from the IVs, the significant causal relationship between MDD and OSA remained intact (OR: 1.373, 95%CI: 1.230–1.532, P = 1.60×10-8). No considerable heterogeneity or pleiotropy was observed (Supplementary Table S12). Subsequently, an MVMR analysis was performed to investigate MDD’s direct effect on OSA, adjusting for BMI, smoking, and alcohol consumption. The MVMR analysis demonstrated that MDD still exerted an immediate impact on OSA. Detailed results of the MVMR analysis are presented in Table 3.
4 Discussion
Although there is a strong correlation between PDs and OSA based on observation, the exact cause relationship is yet unknown. In this study, a bidirectional two-sample MR analysis was conducted to investigate the causal effects of five PDs (MDD, ANX, SCZ, BIP, and PTSD) on OSA. The analysis revealed that genetically determined MDD was associated with an increased risk of OSA, a finding that remained consistent and robust across various sensitivity analyses. Further MVMR analysis confirmed that the impact of MDD on OSA persisted even after adjusting for BMI, smoking, and alcohol consumption. Conversely, the inverse MR analysis indicated that genetically predicted OSA did not have a causal effect on any of the five PDs.
The established association of both MDD and OSA with increased cardiovascular morbidity and mortality (46, 47), combined with their frequent comorbidity, has garnered significant attention. Studies indicate that MDD patients often have elevated pro-inflammatory cytokines, potentially causing neural injury and disrupting homeostatic and circadian processes of the sleep-wake cycle. This may increase the risk of OSA and exacerbate its symptoms (48, 49). Patients undergoing evaluation of OSA in a sleep laboratory also frequently use antidepressants; up to 25% of patients use drugs for depression (50). Patients who had been administered antidepressants had a higher chance of having OSA patients (51). There may be a relationship between MDD, OSA and obesity. Research has demonstrated that being fat increases the likelihood of having moderate-to-severe OSA (more than 50% of those with this condition are obese), and it also increases the likelihood of developing MDD and its onset (52). Observational studies reveal a greater incidence of OSA in individuals with MDD. For example, a large cohort study found that patients with depression had a higher incidence of OSA diagnoses (7.4% vs. 2.9%) compared to non-depressed controls (53). A population-based longitudinal study in Taiwan also reported an increased likelihood of OSA diagnosis in patients with depression compared to controls (54). Our findings corroborate these observations, suggesting that genetically determined MDD is associated with an elevated risk of OSA. Because of the potential confounding effects of alcohol consumption, smoking, and BMI on the association between OSA and PDs, MVMR analysis was carried out. The MVMR analysis has verified that MDD continues to have a direct impact on OSA, even after accounting for factors such as BMI, alcohol consumption, and smoking. However, research on the prevalence of OSA in MDD patients is limited, potentially due to patient non-compliance, resulting in underdiagnosis (55). Therefore, while these MR findings are promising, they should be interpreted cautiously. Further research is required to validate these results and understand the mechanisms through which MDD influences OSA development, which could significantly reduce OSA incidence.
In this study, no causal link was found between psychiatric conditions like SCZ, BIP, PTSD, and OSA, diverging from previous research outcomes. Earlier observational studies suggested an increased OSA risk among SCZ patients compared to those without SCZ (56, 57) and a higher OSA prevalence in individuals with PTSD and BIP than in the general population (10–12). However, these contrasts with our MR analysis might stem from confounding elements in observational studies. For instance, the increased OSA prevalence and severity in patients on antipsychotic medications could be a consequence of side effects like extrapyramidal symptoms or metabolic syndrome. These effects can increase upper respiratory tract resistance and disrupt sleep breathing, escalating OSA risks (58, 59). Unraveling the complex relationship between these PDs and OSA demands further population-based and experimental research. A deeper understanding of these links could be pivotal in the early prevention and diagnosis of OSA.
A reverse MR analysis was conducted to determine the causal relationship between PDs and OSA. However, no significant causal effects of OSA were found on PDs in reverse MR. This contrasts with previous studies suggesting that OSA patients exhibit a dysfunctional immune response, characterized by an increase in pro-inflammatory cytokines like C-reactive protein, IL-6, and TNF-α, which could influence the onset and maintenance of MDD (60, 61). A meta-analysis of five longitudinal studies indicated a higher risk of developing depression in individuals with OSA compared to those without (52). Furthermore, a cross-sectional study reported a higher frequency of anxiety in OSA patients than in the general population (62). The discrepancy between these observational studies and our MR findings could be due to several factors. There is a similarity between the symptoms of OSA and PDs (6, 7). The diagnostic criteria for OSA encompass symptoms such as drowsiness, exhaustion, or sleeplessness, which are also shared diagnostic criteria for some PDs (63). For instance, the Diagnostic and Statistical Manual of Mental Disorders, Fourth Edition, Text Revision (DSM-IV-TR) and the International Classification of Sleep Disorders, Second Edition (ICSD-2) diagnostic criteria for MDD and OSA, respectively, show that these two disorders share symptoms such as daytime tiredness, irritability, and poor concentration (64). Sleep laboratories frequently assess the depressed symptoms of their patients through the use of screening questionnaires (65). The scales, such as the Hamilton Depression Scale (HAMD), the Montgomery-Asberg Depression Rating Scale, and the Beck Depression Inventory (BDI), assess several aspects of sleep, including drowsiness, sleep disturbance, sleep quality, insomnia, and exhaustion. Hence, symptoms of OSA can impact the results of widely employed psychological evaluation instruments. When the HAMD questionnaire asks patients to indicate if they have symptoms such as feeling weak or weary and being easily awakened from sleep, the scores on the psychiatric assessment may be inflated if the patient has sleep-related symptoms caused by OSA. In observational studies, the presence of confounding scores on psychiatric exams may result in an exaggerated diagnosis of PDs. Secondly, the frequent comorbidity of OSA with conditions like insomnia, stroke, diabetes, and cardiovascular disease (66, 67) could confound the association between OSA and PDs. For example, one study found that patients with both OSA and insomnia had significantly higher rates of PDs than those with OSA alone (68).
This study presents several key strengths. Firstly, while previous epidemiological studies have indicated a contentious relationship between PDs and OSA, potentially confounded by extraneous factors and reverse causality, our MR analysis, particularly the MVMR approach, mitigates such biases inherent in observational studies. Secondly, the MR analysis leveraged data from large-scale, published GWAS pooled studies, enhancing the robustness and power of our findings. Finally, this MR study explored the potential causal relationships across a broad spectrum of PDs and OSA, addressing research gaps in existing observational studies and significantly expanding the scope of current research in this domain.
However, recognizing the limitations of this investigation is essential. First and foremost, the GWAS primarily consisted of individuals of European ancestry, necessitating a cautious approach to generalizing our findings to other ethnic groups. Furthermore, the severity of OSA can influence the cause-and-effect connection between OSA and PDs. A comprehensive study including a large group of participants has demonstrated that transitioning from one degree of OSA severity to the next is linked to a 1.8-fold rise in the adjusted probability of developing depression (69). However, no subgroup analysis of OSA severity was performed in our study due to a lack of essential data, which limited our investigation into the causal relationship between PDs and various degrees of OSA. When GWAS with severity distribution becomes publicly available, further in-depth MR analysis will be required. Finally, while specific known confounders were identified and adjusted for, it is essential to acknowledge that residual confounding factors might still influence the study results.
5 Conclusion
This study provides proof that genetically determined MDD is associated with an increased risk of OSA. However, it is crucial to recognize that these findings are based on statistical analyses and necessitate additional empirical research. Further investigation is necessary to confirm these results and to explore the mechanisms by which MDD contributes to the development of OSA. Such understanding could help develop strategies to reduce the incidence of OSA.
Data availability statement
The original contributions presented in the study are included in the article/Supplementary Material. Further inquiries can be directed to the corresponding author.
Ethics statement
Our analyses used published studies or publicly available GWAS abstract data. All studies were conducted with the approval of the appropriate institutional ethics committees, and therefore did not require additional ethical approval.
Author contributions
CM: Conceptualization, Software, Writing – original draft, Writing – review & editing. AH: Writing – original draft. YL: Writing – original draft. XQ: Writing – review & editing. JT: Writing – review & editing.
Funding
The author(s) declare financial support was received for the research, authorship, and/or publication of this article. This study was supported by the Shandong Traditional Chinese Medicine Science and Technology Project (Z-2022087T).
Acknowledgments
We want to acknowledge the participants and investigators of the FinnGen, Genetic Investigation of Anthropometric Traits (GIANT) Consortium, Sequencing Consortium of Alcohol and Nicotine Use (GSCAN) and Psychiatric Genomics Consortium (PGC) studies. Figure 1 was drawn by Figdraw (www.figdraw.com), and we are grateful for the website.
Conflict of interest
The authors declare that the research was conducted in the absence of any commercial or financial relationships that could be construed as a potential conflict of interest.
Publisher’s note
All claims expressed in this article are solely those of the authors and do not necessarily represent those of their affiliated organizations, or those of the publisher, the editors and the reviewers. Any product that may be evaluated in this article, or claim that may be made by its manufacturer, is not guaranteed or endorsed by the publisher.
Supplementary material
The Supplementary Material for this article can be found online at: https://www.frontiersin.org/articles/10.3389/fpsyt.2024.1351216/full#supplementary-material
References
1. Gottlieb DJ, Punjabi NM. Diagnosis and management of obstructive sleep apnea: A review. Jama. (2020) 323:1389–400. doi: 10.1001/jama.2020.3514
2. Senaratna CV, Perret JL, Lodge CJ, Lowe AJ, Campbell BE, Matheson MC, et al. Prevalence of obstructive sleep apnea in the general population: A systematic review. Sleep Med Rev. (2017) 34:70–81. doi: 10.1016/j.smrv.2016.07.002
3. Drager LF, McEvoy RD, Barbe F, Lorenzi-Filho G, Redline S. Sleep apnea and cardiovascular disease: lessons from recent trials and need for team science. Circulation. (2017) 136:1840–50. doi: 10.1161/circulationaha.117.029400
4. Charlson F, van Ommeren M, Flaxman A, Cornett J, Whiteford H, Saxena S. New who prevalence estimates of mental disorders in conflict settings: A systematic review and meta-analysis. Lancet. (2019) 394:240–8. doi: 10.1016/s0140-6736(19)30934-1
5. Gupta MA, Simpson FC. Obstructive sleep apnea and psychiatric disorders: A systematic review. J Clin Sleep Med. (2015) 11:165–75. doi: 10.5664/jcsm.4466
6. Vanek J, Prasko J, Genzor S, Ociskova M, Kantor K, Holubova M, et al. Obstructive sleep apnea, depression and cognitive impairment. Sleep Med. (2020) 72:50–8. doi: 10.1016/j.sleep.2020.03.017
7. Benca RM, Krystal A, Chepke C, Doghramji K. Recognition and management of obstructive sleep apnea in psychiatric practice. J Clin Psychiatry. (2023) 84(2):22r14521. doi: 10.4088/JCP.22r14521
8. Stubbs B, Vancampfort D, Veronese N, Solmi M, Gaughran F, Manu P, et al. The prevalence and predictors of obstructive sleep apnea in major depressive disorder, bipolar disorder and schizophrenia: A systematic review and meta-analysis. J Affect Disord. (2016) 197:259–67. doi: 10.1016/j.jad.2016.02.060
9. Vanek J, Prasko J, Ociskova M, Genzor S, Sovova E, Sova M, et al. Screening for obstructive sleep apnoea in high-risk patients with mood disorders. Neuro Endocrinol Lett. (2022) 43:218–26.
10. Chang ET, Chen SF, Chiang JH, Wang LY, Hsu CY, Shen YC. Risk of obstructive sleep apnea in patients with bipolar disorder: A nationwide population-based cohort study in Taiwan. Psychiatry Clin Neurosci. (2019) 73:163–8. doi: 10.1111/pcn.12802
11. Varadharajan N, Grover S. Prevalence of risk for obstructive sleep apnea in patients with bipolar disorder. Ind Psychiatry J. (2021) 30:285–90. doi: 10.4103/ipj.ipj_91_19
12. McCall CA, Watson NF. A narrative review of the association between post-traumatic stress disorder and obstructive sleep apnea. J Clin Med. (2022) 11(2):415. doi: 10.3390/jcm11020415
13. Tanielian M, Doghramji K, Certa K. Obstructive sleep apnea in psychiatric inpatients. J Nerv Ment Dis. (2020) 208:190–3. doi: 10.1097/nmd.0000000000001129
14. Lu MK, Tan HP, Tsai IN, Huang LC, Liao XM, Lin SH. Sleep apnea is associated with an increased risk of mood disorders: A population-based cohort study. Sleep Breath. (2017) 21:243–53. doi: 10.1007/s11325-016-1389-x
15. Lee SA, Im K, Seo JY, Jung M. Association between sleep apnea severity and symptoms of depression and anxiety among individuals with obstructive sleep apnea. Sleep Med. (2023) 101:11–8. doi: 10.1016/j.sleep.2022.09.023
16. Garbarino S, Bardwell WA, Guglielmi O, Chiorri C, Bonanni E, Magnavita N. Association of anxiety and depression in obstructive sleep apnea patients: A systematic review and meta-analysis. Behav Sleep Med. (2020) 18:35–57. doi: 10.1080/15402002.2018.1545649
17. Ullah MI, Campbell DG, Bhagat R, Lyons JA, Tamanna S. Improving ptsd symptoms and preventing progression of subclinical Ptsd to an overt disorder by treating comorbid osa with Cpap. J Clin Sleep Med. (2017) 13:1191–8. doi: 10.5664/jcsm.6770
18. El-Sherbini AM, Bediwy AS, El-Mitwalli A. Association between obstructive sleep apnea (Osa) and depression and the effect of continuous positive airway pressure (Cpap) treatment. Neuropsychiatr Dis Treat. (2011) 7:715–21. doi: 10.2147/ndt.S26341
19. Giles JJ, Ling I, McArdle N, Bucks RS, Cadby G, Singh B, et al. Obstructive sleep apnea is treatable with continuous positive airway pressure in people with schizophrenia and other psychotic disorders. Schizophr Bull. (2022) 48:437–46. doi: 10.1093/schbul/sbab100
20. Jordan AS, McSharry DG, Malhotra A. Adult obstructive sleep apnoea. Lancet. (2014) 383:736–47. doi: 10.1016/s0140-6736(13)60734-5
21. Melin EO, Wanby P, Neumark T, Holmberg S, Neumark AN, Johansson K, et al. Depression was associated with younger age, female sex, obesity, smoking, and physical inactivity, in 1027 patients with newly diagnosed type 2 diabetes: A swedish multicentre cross-sectional study. BMC Endocr Disord. (2022) 22:273. doi: 10.1186/s12902-022-01184-3
22. Swanson SA, Tiemeier H, Ikram MA, Hernán MA. Nature as a trialist?: deconstructing the analogy between mendelian randomization and randomized trials. Epidemiology. (2017) 28:653–9. doi: 10.1097/ede.0000000000000699
23. Emdin CA, Khera AV, Kathiresan S. Mendelian randomization. Jama. (2017) 318:1925–6. doi: 10.1001/jama.2017.17219
24. Plotnikov D, Guggenheim JA. Mendelian randomisation and the goal of inferring causation from observational studies in the vision sciences. Ophthalmic Physiol Opt. (2019) 39:11–25. doi: 10.1111/opo.12596
25. Davies NM, Holmes MV, Davey Smith G. Reading mendelian randomisation studies: A guide, glossary, and checklist for clinicians. BMJ. (2018) 362:k601. doi: 10.1136/bmj.k601
26. Song L, Li H, Wang J, Xie J, Chen G, Liang T, et al. Educational attainment could be a protective factor against obstructive sleep apnea: A study based on mendelian randomization. J Thorac Dis. (2022) 14:210–5. doi: 10.21037/jtd-21-945
27. Kurki MI, Karjalainen J, Palta P, Sipilä TP, Kristiansson K, Donner KM, et al. Finngen provides genetic insights from a well-phenotyped isolated population. Nature. (2023) 613:508–18. doi: 10.1038/s41586-022-05473-8
28. Howard DM, Adams MJ, Clarke TK, Hafferty JD, Gibson J, Shirali M, et al. Genome-wide meta-analysis of depression identifies 102 independent variants and highlights the importance of the prefrontal brain regions. Nat Neurosci. (2019) 22:343–52. doi: 10.1038/s41593-018-0326-7
29. Otowa T, Hek K, Lee M, Byrne EM, Mirza SS, Nivard MG, et al. Meta-analysis of genome-wide association studies of anxiety disorders. Mol Psychiatry. (2016) 21:1391–9. doi: 10.1038/mp.2015.197
30. Liu L, Xue Y, Chen Y, Chen T, Zhong J, Shao X, et al. Acne and risk of mental disorders: A two-sample mendelian randomization study based on large genome-wide association data. Front Public Health. (2023) 11:1156522. doi: 10.3389/fpubh.2023.1156522
31. Mullins N, Forstner AJ, O'Connell KS, Coombes B, Coleman JRI, Qiao Z, et al. Genome-wide association study of more than 40,000 bipolar disorder cases provides new insights into the underlying biology. Nat Genet. (2021) 53:817–29. doi: 10.1038/s41588-021-00857-4
32. Trubetskoy V, Pardiñas AF, Qi T, Panagiotaropoulou G, Awasthi S, Bigdeli TB, et al. Mapping genomic loci implicates genes and synaptic biology in schizophrenia. Nature. (2022) 604:502–8. doi: 10.1038/s41586-022-04434-5
33. Nievergelt CM, Maihofer AX, Klengel T, Atkinson EG, Chen CY, Choi KW, et al. International meta-analysis of Ptsd genome-wide association studies identifies sex- and ancestry-specific genetic risk loci. Nat Commun. (2019) 10:4558. doi: 10.1038/s41467-019-12576-w
34. Yengo L, Sidorenko J, Kemper KE, Zheng Z, Wood AR, Weedon MN, et al. Meta-analysis of genome-wide association studies for height and body mass index in ∼700000 individuals of european ancestry. Hum Mol Genet. (2018) 27:3641–9. doi: 10.1093/hmg/ddy271
35. Liu M, Jiang Y, Wedow R, Li Y, Brazel DM, Chen F, et al. Association Studies of up to 1.2 Million Individuals Yield New Insights into the Genetic Etiology of Tobacco and Alcohol Use. Nat Genet. (2019) 51:237–44. doi: 10.1038/s41588-018-0307-5
36. Huang W, Xiao J, Ji J, Chen L. Association of lipid-lowering drugs with covid-19 outcomes from a mendelian randomization study. Elife. (2021) 10:e73873. doi: 10.7554/eLife.73873
37. Liu M, Zhang Y, Wu J, Gao M, Zhu Z, Chen H. Causal relationship between kidney stones and gut microbiota contributes to the gut-kidney axis: A two-sample mendelian randomization study. Front Microbiol. (2023) 14:1204311. doi: 10.3389/fmicb.2023.1204311
38. Wu Q, Liu S, Huang X, Liu J, Wang Y, Xiang Y, et al. Bidirectional mendelian randomization study of psychiatric disorders and parkinson's disease. Front Aging Neurosci. (2023) 15:1120615. doi: 10.3389/fnagi.2023.1120615
39. Pagoni P, Dimou NL, Murphy N, Stergiakouli E. Using mendelian randomisation to assess causality in observational studies. Evid Based Ment Health. (2019) 22:67–71. doi: 10.1136/ebmental-2019-300085
40. Bowden J, Davey Smith G, Haycock PC, Burgess S. Consistent estimation in mendelian randomization with some invalid instruments using a weighted median estimator. Genet Epidemiol. (2016) 40:304–14. doi: 10.1002/gepi.21965
41. Bowden J, Davey Smith G, Burgess S. Mendelian randomization with invalid instruments: effect estimation and bias detection through egger regression. Int J Epidemiol. (2015) 44:512–25. doi: 10.1093/ije/dyv080
42. Verbanck M, Chen CY, Neale B, Do R. Detection of widespread horizontal pleiotropy in causal relationships inferred from mendelian randomization between complex traits and diseases. Nat Genet. (2018) 50:693–8. doi: 10.1038/s41588-018-0099-7
43. Greco MF, Minelli C, Sheehan NA, Thompson JR. Detecting pleiotropy in mendelian randomisation studies with summary data and a continuous outcome. Stat Med. (2015) 34:2926–40. doi: 10.1002/sim.6522
44. Kamat MA, Blackshaw JA, Young R, Surendran P, Burgess S, Danesh J, et al. Phenoscanner V2: an expanded tool for searching human genotype-phenotype associations. Bioinformatics. (2019) 35:4851–3. doi: 10.1093/bioinformatics/btz469
45. Burgess S, Thompson SG. Multivariable mendelian randomization: the use of pleiotropic genetic variants to estimate causal effects. Am J Epidemiol. (2015) 181:251–60. doi: 10.1093/aje/kwu283
46. Van der Kooy K, van Hout H, Marwijk H, Marten H, Stehouwer C, Beekman A. Depression and the risk for cardiovascular diseases: systematic review and meta analysis. Int J Geriatr Psychiatry. (2007) 22:613–26. doi: 10.1002/gps.1723
47. Kendzerska T, Gershon AS, Hawker G, Leung RS, Tomlinson G. Obstructive sleep apnea and risk of cardiovascular events and all-cause mortality: A decade-long historical cohort study. PloS Med. (2014) 11:e1001599. doi: 10.1371/journal.pmed.1001599
48. Irwin MR, Miller AH. Depressive disorders and immunity: 20 years of progress and discovery. Brain Behav Immun. (2007) 21:374–83. doi: 10.1016/j.bbi.2007.01.010
49. Vgontzas AN, Zoumakis E, Lin HM, Bixler EO, Trakada G, Chrousos GP. Marked decrease in sleepiness in patients with sleep apnea by etanercept, a tumor necrosis factor-alpha antagonist. J Clin Endocrinol Metab. (2004) 89:4409–13. doi: 10.1210/jc.2003-031929
50. Law M, Naughton MT, Dhar A, Barton D, Dabscheck E. Validation of two depression screening instruments in a sleep disorders clinic. J Clin Sleep Med. (2014) 10:683–8. doi: 10.5664/jcsm.3802
51. Farney RJ, Lugo A, Jensen RL, Walker JM, Cloward TV. Simultaneous use of antidepressant and antihypertensive medications increases likelihood of diagnosis of obstructive sleep apnea syndrome. Chest. (2004) 125:1279–85. doi: 10.1378/chest.125.4.1279
52. Edwards C, Almeida OP, Ford AH. Obstructive sleep apnea and depression: A systematic review and meta-analysis. Maturitas. (2020) 142:45–54. doi: 10.1016/j.maturitas.2020.06.002
53. Sharafkhaneh A, Giray N, Richardson P, Young T, Hirshkowitz M. Association of psychiatric disorders and sleep apnea in a large cohort. Sleep. (2005) 28:1405–11. doi: 10.1093/sleep/28.11.1405
54. Pan ML, Tsao HM, Hsu CC, Wu KM, Hsu TS, Wu YT, et al. Bidirectional association between obstructive sleep apnea and depression: A population-based longitudinal study. Med (Baltimore). (2016) 95:e4833. doi: 10.1097/md.0000000000004833
55. Hein M, Lanquart JP, Loas G, Hubain P, Linkowski P. Prevalence and risk factors of moderate to severe obstructive sleep apnea syndrome in major depression: A observational and retrospective study on 703 subjects. BMC Pulm Med. (2017) 17:165. doi: 10.1186/s12890-017-0522-3
56. Wu YY, Chang ET, Yang YC, Chen SF, Hsu CY, Shen YC. Risk of obstructive sleep apnea in patients with schizophrenia: A nationwide population-based cohort study. Soc Psychiatry Psychiatr Epidemiol. (2020) 55:1671–7. doi: 10.1007/s00127-020-01870-4
57. Myles H, Vincent A, Myles N, Adams R, Chandratilleke M, Liu D, et al. Obstructive sleep apnoea is more prevalent in men with schizophrenia compared to general population controls: results of a matched cohort study. Australas Psychiatry. (2018) 26:600–3. doi: 10.1177/1039856218772241
58. Shirani A, Paradiso S, Dyken ME. The impact of atypical antipsychotic use on obstructive sleep apnea: A pilot study and literature review. Sleep Med. (2011) 12:591–7. doi: 10.1016/j.sleep.2010.12.013
59. Rishi MA, Shetty M, Wolff A, Amoateng-Adjepong Y, Manthous CA. Atypical antipsychotic medications are independently associated with severe obstructive sleep apnea. Clin Neuropharmacol. (2010) 33:109–13. doi: 10.1097/WNF.0b013e3181db8040
60. Teramoto S, Yamamoto H, Ouchi Y. Increased C-reactive protein and increased plasma interleukin-6 may synergistically affect the progression of coronary atherosclerosis in obstructive sleep apnea syndrome. Circulation. (2003) 107:E40–0. doi: 10.1161/01.cir.0000053956.46188.5f
61. Dowlati Y, Herrmann N, Swardfager W, Liu H, Sham L, Reim EK, et al. A meta-analysis of cytokines in major depression. Biol Psychiatry. (2010) 67:446–57. doi: 10.1016/j.biopsych.2009.09.033
62. Rezaeitalab F, Moharrari F, Saberi S, Asadpour H, Rezaeetalab F. The correlation of anxiety and depression with obstructive sleep apnea syndrome. J Res Med Sci. (2014) 19:205–10.
63. Sateia MJ. International classification of sleep disorders-third edition: highlights and modifications. Chest. (2014) 146:1387–94. doi: 10.1378/chest.14-0970
64. Ejaz SM, Khawaja IS, Bhatia S, Hurwitz TD. Obstructive sleep apnea and depression: A review. Innov Clin Neurosci. (2011) 8:17–25.
65. Schröder CM, O'Hara R. Depression and obstructive sleep apnea (Osa). Ann Gen Psychiatry. (2005) 4:13. doi: 10.1186/1744-859x-4-13
66. Sweetman A, Frank O, Stocks N, Mukherjee S, Lack L. General practitioner management of comorbid insomnia and sleep apnoea. Aust J Gen Pract. (2023) 52:607–12. doi: 10.31128/ajgp-12-22-6648
67. Gleeson M, McNicholas WT. Bidirectional relationships of comorbidity with obstructive sleep apnoea. Eur Respir Rev. (2022) 31(164):210256. doi: 10.1183/16000617.0256-2021
68. Krell SB, Kapur VK. Insomnia complaints in patients evaluated for obstructive sleep apnea. Sleep Breath. (2005) 9:104–10. doi: 10.1007/s11325-005-0026-x
Keywords: genome-wide association study, obstructive sleep apnea, causal relationship, psychiatric disorders, mendelian randomization
Citation: Mi C, Hou A, Liu Y, Qi X and Teng J (2024) Assessing the causal relationship between psychiatric disorders and obstructive sleep apnea: a bidirectional Mendelian randomization. Front. Psychiatry 15:1351216. doi: 10.3389/fpsyt.2024.1351216
Received: 06 December 2023; Accepted: 29 January 2024;
Published: 14 February 2024.
Edited by:
Mieszko Wieckiewicz, Wroclaw Medical University, PolandReviewed by:
Tomasz Wieczorek, Wroclaw Medical University, PolandMonika Michałek-Zrąbkowska, Wroclaw Medical University, Poland
Copyright © 2024 Mi, Hou, Liu, Qi and Teng. This is an open-access article distributed under the terms of the Creative Commons Attribution License (CC BY). The use, distribution or reproduction in other forums is permitted, provided the original author(s) and the copyright owner(s) are credited and that the original publication in this journal is cited, in accordance with accepted academic practice. No use, distribution or reproduction is permitted which does not comply with these terms.
*Correspondence: Jing Teng, 60170099@sdutcm.edu.cn