- 1Institute of German Studies, University of Duisburg-Essen, Duisburg, Germany
- 2Department of Child and Adolescent Psychiatry, Psychotherapy, and Psychosomatics, Medical Center – University of Freiburg, Faculty of Medicine, University of Freiburg, Freiburg, Germany
- 3Department of Psychiatry and Psychotherapy, Medical Center – University of Freiburg, Faculty of Medicine, University of Freiburg, Freiburg, Germany
- 4Luzerner Psychiatrie, Ambulante Dienste, Luzern, Switzerland
- 5Institute of Acoustics and Speech Communication, Technische Universität Dresden, Dresden, Germany
Introduction: We investigated the prosodic perception of uncertainty cues in adults with Autism Spectrum Disorder (ASD) compared to neurotypical adults (NTC).
Method: We used articulatory synthetic speech to express uncertainty in a human-machine scenario by varying the three acoustic cues pause, intonation, and hesitation. Twenty-eight adults with ASD and 28 NTC adults rated each answer for uncertainty, naturalness, and comprehensibility.
Results: Both groups reliably perceived different levels of uncertainty. Stimuli were rated as less uncertain by the ASD group, but not significantly. Only when we pooled the recipients’ ratings for all three cues, did we find a significant group difference. In terms of reaction time, we observed longer reaction times in the ASD group compared to the neurotypical comparison group for the uncertainty level hesitation & strong intonation, but the differences were not significant after Bonferroni correction. Furthermore, our results showed a significant group difference between the correlation of uncertainty and naturalness, i.e. the correlation in the ASD group is significantly lower than in the NTC group. Obtained effect size estimates can inform sample size calculations in future studies for the reliable identification of group differences.
Discussion: In future work, we would like to further investigate the interaction of all three cues and uncertainty perception. It would be interesting to further vary the duration of the pause and also to use different types of fillers. From a developmental perspective, uncertainty perception should also be investigated in children and adolescents with ASD.
1 Introduction
We present an empirical study investigating the perception of uncertainty cues in adults with ASD compared to the NTC group. To generate our material, we used articulatory speech synthesis with varying prosodic uncertainty features. The utterances were presented to the participants and they were asked to rate them. We consider the ascription of (un)certainty as a part of affective ToM and assume that (u)certainty can be expressed prosodically without interaction with syntactic or semantic features of an utterance. Thus, its effect can be studied in isolation. In the following introductory section, we provide the theoretical background of our research goal and outline the state of research on the role of prosody perception in ASD. This includes studies of emotion perception, speech synthesis perception, and uncertainty perception in both human-human and human-machine interaction.
According to DSM-5 (1) and ICD-11 (2) Autism Spectrum Disorder (ASD) is classified as a neurodevelopmental disorder with severe impairments in the domains of social communication and restrictive repetitive behaviors/interests. The prevalence is approximately 1% (3, 4). The male-female ratio in well-ascertained epidemiological samples is about 3:1. However, there are concerns about under-reporting in girls and women [cf. (1): 64]. The etiology of ASD shows a strong genetic component as well as other causes (4).
In this article we will focus on Autism Spectrum Disorder without accompanying intellectual impairment (ASD without II). A description of ASD without II can be found, for example, in Riedel (5) and in Vogeley (6). In the area of language processing, syntactic and semantic processing are barely affected in ASD without intellectual impairment taking into account the semiotic dimensions according to Morris (7), but problems in pragmatic interpretation are often found [ (8): 4f.]. For example, adults with ASD without II often have difficulty understanding non-lexicalized metaphors as assessed by the Freiburg Questionnaire of Linguistic Pragmatics (FQLP) (9). Although in the literature, often problems in general pragmatic processing in ASD without intellectual impairment are described, it has been shown that the pragmatic abilities for hearers with ASD without II differ between pragmatic domains [cf. (10): 114, see also (11)]. In terms of syntactic processing, Durrleman et al. (12) tested relative clause comprehension in autistic participants with and without reported language delay. They found that the participants with reported language delay had more difficulty with subject relatives than those without language delay. It should be noted here that we assume that syntax is more likely to be impaired in autistic individuals with delayed language development. However, in the case of autism without intellectual impairment pragmatics is the focus of our research interest.
Several empirical studies investigated the prosodic competence of participants with ASD without II. The term prosody is defined as “[ … ] a set of higher-level organizational structures that account for variations in pitch, loudness, duration, spectral tilt, segment reduction and their associated articulatory parameters” [ (13): 327].
At the interface of syntax and pragmatics, the work of Martzoukou et al. (14, 15) suggested evidence of problems with the use of prosody in syntactic processing. Similarly, Terzi et al. (16) reported difficulties at the interface of morpho-syntax with pragmatics and prosody in ASD without intellectual impairment.
However, several studies have focused on the perception and production of prosody and its signaling of pragmatic and emotional features of utterances. In the following, we present selected previous studies that have investigated the role of prosody in both speech production and speech perception in order to place our empirical study in a theoretical context. Since prosody has different linguistic and paralinguistic functions [cf. (17): 326], we refer first to linguistic functions such as the marking/perception of information status, i.e. structural prosodic functions [for an overview see (18)]. For example, prosody can be used to indicate the information status of a sentence (19). Afterwards we will discuss paralinguistic functions of prosody such as emotion expression/perception, i.e. affective prosodic functions [for an overview see (20)]. An overview of linguistic prosody in ASD is given in Grice et al. (21). In this work, the various functions of prosody are described in more detail. Depending on the prosodic function, there are differences between the ASD and NTC (neurotypical control) groups.1
In terms of structural prosody skills in ASD, Shriberg et al. (22) reported for accentuation that speakers with ASD without II aged 10-50 years were less likely to use stress and phrasing appropriately compared to NTC. Similarly, Paul et al. (23) reported difficulties in stress production and also in speech perception more often in the ASD group without II compared to the NTC in speakers aged 14-21 years. In addition, Kiss et al. (24) found significant differences in global pitch distribution comparing children aged 4 to 9 years and a NTC group using the CSLU Autism Speech Corpus. Nadig and Shaw (25) observed a higher pitch range in speakers aged 8-14 years old with ASD without II in contrast to the NTC, but neurotypical students did not rate this as increased pitch variation. Wehrle et al. (26) also found a tendency for adults with ASD to have a higher pitch range compared to the NTC. For both prosody perception and production, Diehl and Paul (27) showed that children and adolescents aged 8-16 years with ASD without II required more time to imitate intonation patterns than the NTC.
For adults with ASD without II, the perception study by Grice et al. (28) suggested evidence that adults with ASD showed a reduced sensitivity to intonation and consequently based their judgments less on the word pronunciation in comparison to neurotypical adult hearers. Instead, word frequency was more important than intonation for decoding of information structure (i.e. the division of sentences into new and known information) in autistic hearers. In contrast, Globerson et al. (29) found no differences between adult hearers with and without ASD using prosody for pragmatic focus interpretation (i.e. the detection of new information in a sentence)2. The groups also did not differ in psychoacoustic tests. In contrast to that, the group with ASD performed less accurately on both the acoustic prosody recognition task and the facial emotion recognition task.
In their systematic review of linguistic prosody in ASD, Grice et al. (21) examined both production and perception of prosodic functions in grammar and pragmatics, as well as emotion. They categorize prosodic functions on a scale of more “formal” (rule-based) functions and more “intuitive” (highly context-dependent functions). Lexical stress, lexical tones and grammatical functions of prosody belong to the most formal functions, marking of intentions and emotions to the most intuitive [cf. (21): 2-5]. The results for perception suggested that the more intuitive aspects of prosody are more difficult in ASD, i.e. perceiving information status, intention, and emotional state. In contrast, the more formal aspects of prosody such as lexical and syntactic functions appear to be relatively unaffected [cf. (21): 6-8]. No clear overarching pattern was found for prosody production [cf. (21): 12]. However, there was a tendency for differences in general prosodic characteristics in speech production [see (21):13].
In conclusion, the results of the presented studies on structural prosody in ASD are not clear with respect to group differences. This can be explained by the different functions of prosody. Prosodic uncertainty marking is not one of the ‘formal aspects’ of prosody and we would therefore expect to see stronger differences between groups.
After reviewing previous studies of prosody production and perception in hearers with ASD without II, we turn to affective prosody skills in ASD and refer to previous work on the expression and recognition of emotion in ASD. The reason for this is that emotions and epistemic states can also be expressed through prosody [cf. (32): 48]. This is relevant to our current experimental study in which we express different degrees of intended uncertainty by means of prosody using articulatory speech synthesis (33)3.
In their Facial Recognition Task, Doi et al. (34) generated varying degrees of anger, happiness, and sadness, as well as a neutral face. In the Emotional Prosody Recognition Task, a naturally spoken Japanese utterance was presented in an angry, happy, and sad way of speaking at different intensities Also a neutral acoustic stimulus was used [cf. (34): 2102 ff.]. The adults in the ASD group performed worse at recognizing angry and sad faces and voices. There was an effect of emotional intensity on emotion recognition. For facial expression recognition, there was a lower recognition in the ASD group compared to the NTC group for the stimuli of intermediate emotional intensities [cf. (34): 2109].
Hsu and Xu (35) used the articulatory speech synthesizer VocalTractLab (36) to produce modal, breathy, and pressed voices in Mandarin. Hearers with ASD without II and a NTC were asked to judge body size, emotion (happiness, anger, and neutral emotion) and attitude [cf. (35): 1925]. The results showed that the adolescents with ASD were less sensitive to auditory manipulation than their neurotypical peers [cf. (35): 1927]. However, to our knowledge, uncertainty perception has not been investigated using articulatory speech synthesis. We will use this type of speech synthesis to model uncertainty and test its influence on uncertainty perception in our empirical study.
In two meta-analyses of facial emotion recognition (37, 38), participants with ASD showed significantly poorer performance in recognizing basic emotions compared to the NTC group for a subset of basic emotions. However, Scheerer et al. (39) found that autistic and typically developing children were accurate in matching emotional voice clips to emotion words, but autistic children had difficulty in matching emotional voice clips to emotional faces. Lartseva et al. (40) likewise document the presence of impairments in emotional language processing in individuals with ASD. These appear to be fairly independent of stimulus complexity, task complexity, and sensory modality as well as the level of language development. Lui et al. (41) investigated the role of psychoacoustic abilities in affective prosody recognition in autistic adults. Their results indicated that psychoacoustic abilities were used as a compensatory mechanism for deficits in higher-order processing of emotional signals in social interactions.
In our recent study (42) we presented a systematic analysis of 12 selected studies on emotion perception for the auditory and/or visual modality. The analysis revealed that in most cases basic emotions according to Ekman (43) were tested exclusively or in combination with complex emotions. The results generally showed a difference in perception between the ASD and NTC groups for the different modalities with only two studies showing no difference in visual emotion perception.
In their systematic review of affective prosody recognition in ASD concerning basic emotions according to Ekman (43), Zhang et al. (44) investigated potential factors for differences in study results comparing ASD and NTC groups. Their results showed that the level of difficulty in affective prosody recognition experienced by hearers with ASD varied across basic emotions.
As the aforementioned studies on emotion perception in ASD have shown divergent results regarding differences in emotion processing between autistic and non-autistic hearers, we believe that further research is needed in this area. The studies mentioned above have in common that mainly basic emotions according to Ekman (43) were investigated. In our work, we focus on uncertainty as a non-prototypical emotion. To our knowledge, there is a research gap regarding the perception of uncertainty in ASD. With our study, we hope to contribute to the understanding of how uncertainty is processed as a non-prototypical emotion by hearers with ASD and thus fill this research gap.
Next, we will further motivate why we consider the perception of uncertainty conveyed by prosodic cues in ASD to be a particular interest. We assume that uncertainty refers to the statement in the utterance of the prosodic information. The speaker’s belief state, including the perceived uncertainty, is part of the hearer’s ToM [see Theory of Mind; (45)]. We regard the attribution of uncertainty to another person, i.e. the speaker, as a case of affective ToM, but with reference to a proposition (a statement or a fact about the speaker is uncertain), i.e. to a conceptual content.
Uncertainty could therefore be understood as an affective propositional attitude. In philosophy, psychology, linguistics, and cognitive science, propositional attitudes are understood as the mental phenomena expressed by sentences such as Galileo believes that the earth moves and Pia hopes that it will rain (i.e. the belief about the movement of earth and the hope of rain). Even if propositional attitudes are discussed critically, it is agreed that they are mental phenomena and play a central role in our everyday practice of describing, explaining, and predicting others and ourselves [cf. (46)]. Even basic emotions according to Ekman (43) such as fear or surprise can be attitudes towards propositions, e.g. fearing that one shall be killed in an avalanche or being surprised that New York is further south than Rome. A discussion of propositional attitude approach to emotions is given in Cudney (47). According to Giannakidou and Mari (48), emotion attitudes appear as gradable psychological attitudes, i.e. be happy, be surprised, be angry, and are assumed to be factive.
In the field of prosody research, Hirschberg [ (49): 532] notes that the variation in prosody influences the interpretation of linguistic phenomena in many languages. Speakers can also use prosody to indicate the propositional attitude they have towards a certain proposition when uttering a sentence expressing that proposition [see also (49): 532].
As already noted above, uncertainty is a complex phenomenon. When we refer to uncertainty we mean uncertainty in answers in question-answer situations as will be explained below. Thus, the aim of our study is to empirically investigate the perception of uncertainty in autistic hearers in order to get a broader picture of emotion processing in ASD.
Next, we will explain the theoretical background of the communication of uncertainty in face-to-face communication in neurotypical hearers. Then we will further explain the motivation for our empirical investigation in hearers with ASD.
1.1 Communication of uncertainty
The expression and perception of uncertainty is essential in communication [cf. (50): 8]. As remarked in Wollermann [ (51): 80f.], uncertainty can generally be regarded as a non-prototypical emotion [see also (52)]. Kuhltau (53) categorizes uncertainty in cognitive terms. Furthermore, uncertainty can be considered from an epistemic point of view in communication (54). A discussion of whether epistemic emotions are metacognitive can be found in Carruthers (55).
Following Wollermann [ (51): 80], we assume that speakers and hearers communicate uncertainty in question-answer situations: communication partner A asks communication partner B a question. B is uncertain about the answer and expresses this uncertainty. A uses these uncertainty cues to decode B’s utterance and concludes that B is uncertain [cf. (51): 80]. It should be noted explicitly here that uncertainty is a complex phenomenon that encompasses different dimensions and definitions [see also (56): 138]. However, as noted above, we focus on uncertainty in responses to questions in communicative situations. We begin by referring to previous studies that have investigated the production and perception of uncertainty. We then discuss ideas of ToM and relate them to ASD in order to provide the theoretical background for our empirical study of uncertainty perception in ASD.
Smith and Clark (57) used the Feeling of Knowing paradigm following Hart (58) in order to test memory processes in adults in question-answering situations. Empirical results showed that uncertainty was marked, among other cues, lexically by the use of phrases such as “I guess” and by fillers such as “uh” and “um”. On the prosodic level pauses and rising intonation were observed as prosodic indicators of uncertainty [cf. (57): 32ff., see also (51): 82f.]. In order to test the perception of another speaker, Brennan and Williams (59) defined the Feeling of Another’s Knowing paradigm. They reproduced the study of Smith and Clark (57). In a further step, they used the audio material for listening evaluation. It was found that lexical hedges, rising intonation and delay contributed to the perception of uncertainty [cf. (59): 383; see also (51): 83]. Swerts and Krahmer (60) investigated the production and perception of uncertainty in the audio, visual, and audiovisual conditions. Uncertainty in answers was recognized in all three conditions, but recognition was easier in the audiovisual condition than in the unimodal conditions.
From a developmental perspective, Krahmer and Swerts (61) tested 7-8 year old neurotypical children and adults for the perception and production of uncertainty in question-answer situations in audiovisual speech. Uncertain utterances produced by adult speakers were recognized more accurately than children’s uncertain utterances by both children and adults as hearers. In addition, adults performed better than children in the recognition of uncertainty.
After referring to studies on uncertainty perception and production, we now provide the relevant background on ToM for our empirical study. Premack and Woodruff [ (45): 515] define ToM as follows: “An individual has a theory of mind if he imputes mental states to himself and others”. The concept of ToM also known as “mind reading” refers to the understanding of one’s own thoughts and feelings and those of others, and is central for human social interaction and communication. There is empirical evidence that it develops very early in human ontogeny [cf. (62): 1357]. An overview of ToM can be found, for example, in Astington and Dack (63) and in Leslie (64).
According to Kamp-Becker and Bölte [ (65): 40], children with ASD often have serious problems executing theory of mind tasks. In their seminal work, Baron-Cohen and et al. (66) discussed whether the autistic child has a ToM. Their study and that of Happé (67) suggested that children with ASD had problems in passing false-belief-tasks.4 However, it has to be discussed critically if a general ToM deficit occurs in individuals with ASD. As Chevallier [ (70): 4825] remarks there is evidence that there are problems related to ToM in ASD on the basis of standard false belief tasks or other more fine-grained tests. However, the characteristics of these impairments are still debated, i.e. if it is a primary or simply consecutive to more basic deficits [cf. (70): 4825]. Furthermore, the study by Tager-Flusberg (71) suggested that autistic participants who had passed a standard test with first-order false belief tasks, were even able to solve more complex second-order belief tasks when processing demands were reduced. In addition, the work of Iao and Leekam (72) showed that difficulties with the false representation tasks in children with ASD could not be explained by executive functions or language impairments. This may provide evidence to support the position that children with ASD may not have a specific theory of mind deficit.
As Gabriel et al. [ (69): 534] pointed out, ToM is a complex phenomenon that can be divided into cognitive and affective ToM [e.g., (73)]. On the one hand affective ToM refers to the representation of implications about emotions. On the other hand cognitive ToM is a term that describes implications about knowledge, intentions, and beliefs [cf. (69): 534]. For early adolescence, there was a correlation between both types of ToM and attention. There was also a correlation between cognitive ToM and language comprehension on the one hand, and a correlation between affective ToM and verbal intelligence, verbal fluency, and verbal flexibility. In middle and late adolescence, both types of ToM were correlated with affective intelligence. On the other hand, there was a correlation between cognitive ToM and working memory, figural intelligence, and language comprehension. Thus, the results for cognitive and affective ToM showed a developmental step in middle adolescence. There were also gender differences in cognitive ToM [cf. (69): 533].
Raimo et al. (74) investigated both types of ToM in neurotypical individuals during adulthood. According to Raimo et al. [ (74): 10], the decline of the affective component of ToM occurs earlier in adulthood (from the age of 60) than the cognitive component (from the age of 70). This decline in the first age group is related to the ability to infer others’ emotions and to decode emotional expressions in the nonverbal modality, rather than to the ability to infer emotional mental states from social stories in the verbal modality. In the older group, the decline is independent of the verbal or nonverbal modality of the task used [cf. (74): 10].
It should be noted that these two subtypes of ToM, i.e. affective vs. cognitive ToM, are not always clearly distinguished. The demarcation is not always consistent and is not always sharp. We talk about needs which have rather an emotional component, e.g. when there is a need for getting comfort, or a cognitive character, e.g. when we are curious about something.
We now turn to previous studies of affective and cognitive ToM in ASD. Begeer et al. (75) investigated affective ToM and tested children’s understanding of emotions based on counterfactual reasoning.5 The autistic children had problems in explaining emotions based on downward counterfactual reasoning (i.e. contentment and relief) compared to the neurotypical children. In contrast, there were no group differences in emotions based on upward counterfactual reasoning (i.e. disappointment and regret). The results also showed a relationship between second-order false-belief reasoning and children’s understanding of second-order counterfactual emotions for the neurotypical comparison group. However, children with ASD were more likely to rely on their general intellectual abilities [cf. (75): 301].
Scheeren et al. (77) tested comprehension of social stories containing second-order false belief display rules, double bluff, faux pas, and sarcasm. They found that children and adolescents with ASD performed as well as the NTC group. The age effect was consistent with adolescents performing better than children. Success on advanced ToM tasks was also determined by age, verbal abilities, and general reasoning abilities.
Similarly, Kimhi’s (78) review showed that language and verbal abilities, as well as general reasoning, facilitated better ToM comprehension in ASD [cf. 78: 340]. They also noted that ToM is a critical factor in children’s socio-cognitive development (cf. (78): 339).
There is currently some debate as to whether or not the feeling of uncertainty (and its supposed opposite, the feeling of certainty) belongs specifically to the category of so-called “epistemic emotions” in particular or can be considered as an emotion at all [see Meylan (79) for a con position, and Silva (80) for a pro position]. Whatever its exact nature, there is broad agreement that the feeling of uncertainty is an affective mental state. For example, Morriss et al. [ (81): 2] emphasize that “current theoretical models posit that uncertainty is aversive in and of itself and is consequently more likely to engage the behavioral inhibition system responsible for stress and associated negative emotional states, particularly anxiety and fear” [for a more detailed discussion see Morriss et al., (81)]. Consistent with this, the glossary of mental state terms in the well-known Reading-the-Mind-in-the-Eyes test (82, 83), which participants are asked to consult when they are unsure of the meaning of a response option, recurs on the concepts of feelings of certainty and uncertainty.
Andres-Roqueta and Katsos (11) investigated pragmatic skills in children with and without ASD. The tasks consisted of a linguistic-pragmatics task requiring competence with structural language and a social-pragmatics task requiring competence with ToM. They reported similar performance on structural pragmatics between the group with ASD and the NTC, but a lower performance on social pragmatics, which the authors explain with difficulties in ToM [cf. (11): 1494].
At this point, we would also like to address the link between ToM and compensation strategies [e.g. (84, 85)]. Livingston et al. [(84): 102] give the following example for compensation strategies: If a difficulty in distinguishing lies from jokes is masked by copying the behavior of others (e.g. laughing), compensation would mean that a conscious rule is developed: if someone makes a nonliteral statement and laughs, it is probably a joke. Otherwise it is probably a lie.
The following observations, which we describe in the next three sections, come from our clinical practice: Socio-cognitive tasks can be solved either intuitively-automatically or cognitively-deliberatively. The following example illustrates this: When a happy face is perceived, the intuitive automatic solution would be “the face shows happiness”. In the case of the cognitively-deliberative solution, different features are combined for interpretation, such as the cheek-raiser and the lip corner puller. This corresponds to the compensatory strategy used by autistic people which can be used to circumvent problems in the socio-cognitive area. However, it requires a great deal of effort on the part of the autistic person. The disadvantage of most of the experiments is that one can concentrate on the tasks and solve them in a cognitive-deliberative way.
Adults with ASD often learn to read the mental states of their fellow human beings via cognitive compensation when they are consciously thinking about them. Most experimental designs can be solved in this way. This could explain the results showing no significant difference in speech interpretation between the ASD and NTC groups.
For neurotypical people, the construction of a ToM often occurs unconsciously, i.e. when they are not thinking about it. An example would be the perception of mental states of hearers during a speaker’s lecture. In our clinical experience, this is not the case for people with ASD, as their focus needs to shift to consciously inferring the mental states of others.
In the research on disfluencies in speech two types of pauses are often discussed: silent pauses and filled pauses [cf. (86): 49; see also (87)]. As Rose [ (86): 49] points out, silent pauses are periods of non-articulation by the speaker, whereas filled pauses are periods of articulation of non-propositional content and also conform to language-specific conventions. Filled pauses are also often referred to as hesitations [for a discussion of the variation in terminology of filled pauses see Belz, (88): 1].
Silent and filled pauses have in common that they are used for speech planning and self-repair [cf. Rose, (86): 49]. Silent pauses are used for breathing and for marking syntactic structures, whereas filled pauses are periods of articulation of non-propositional content [cf. (86): 49] and are relevant for turn holding [see (89)].6
According to Belz [ (91): 41], filled pauses may serve as hesitation markers, repair markers, turn holding markers and others. The work of Wehrle et al. (92) with adults with ASD without intellectual impairment showed that a higher proportion of filled pause tokens were produced with the canonical level pitch contour by the NTC group compared to the autistic speakers.
The pragmatic difference between silent and filled pauses is less relevant for us because the right to speak does not play a role in our scenario. Nevertheless, we test whether filled and silent pauses differ in terms of the attribution of uncertainty. We use a combination of silent and filled pauses to realize particularly long and conspicuous hesitations.
At the phonetic level, the study by Betz et al. (93) suggested that the position of the extension in noun phrases such as ‘the green tree’ influences uncertainty perception. The results showed the following: Firstly, hearers interpreted lengthening in the initial position of a word as uncertainty about the semantic domain represented by the word itself. Secondly, hearers interpreted lengthening in the final position within the word as uncertainty about the semantic domain represented by the following content word [cf. (93): 3993]. As we used only one-word utterances in our study (un)certainty must be ascribed by the hearer to the information conveyed by this word.
Termis like hesitation and (dis)fluency are used differently in the literature [see (94)]. In our study, we use the term hesitation to refer to particles like “uh” which we also refer to as fillers. Hesitation and pause are each defined as independent variables for optimal manipulation of the synthetic signal [see (Table 1)]. However, we are aware that the hesitation particle and pause often form a unit in spoken utterances.
In our study the aim was to investigate whether the hearer attributes uncertainty to the speaker solely on the basis of prosodic information. As already mentioned, we regard the attribution of uncertainty to another person, i.e. the speaker, as a case of affective ToM, but with reference to conceptual content (a statement or a fact which the speaker is uncertain about). It is important to note that in our scenario the speech signal is synthetic, as we expressed different degrees of intended uncertainty through prosody using articulatory speech synthesis (33). The uncertain synthetic utterance served as an answer in the form of a statement to a question in a brief human-machine scenario. We will refer to previous studies in which uncertainty was modeled using a speech synthesizer.
1.2 Modelling and perception of uncertainty in human-machine-communication
In the context of human-machine interaction, the question arises as to whether speech synthesis should be enriched with emotional expressions [for a recent discussion of the role of emotions in synthetic speech see (95)]. According to Murray and Arnott (96), one aspect of the naturalness of the synthetic utterance is that the emotional state of the speaker contributes to the variability of synthetic speech; emotional expressions are regarded as pragmatic variations in speech. Artificial question-answering systems may follow in order to maintain user trust by expressing the degree of uncertainty attached to the provided answers (97). According to Székely et al. [ (98): 804], the expression and communication of a system’s internal uncertainty is a key to successful human-robot interaction.7
In previous studies, disfluent speech for acoustic speech synthesis has been modeled using filled pauses (99) and also of filled pauses and lexical fillers (100) in unit selection speech synthesis.8 In both studies, the activation of hesitations was not perceived differently with respect to naturalness from deactivation. Hönemann and Wagner (102) modeled uncertainty in speech synthesis as one of four emotional states by using features of prosody and voice quality. Furthermore, in the study of Śzekely et al. (98) the perception of uncertainty in synthetic speech was tested by using a synthesis method based on a DNN (deep neural network). Decreased vocal effort, filled pauses and prolongation of function words contributed to an increase in the degree of perceived uncertainty. For an overview of the role of hesitations in spoken dialogue systems, see Betz (103).
In traditional approaches for speech synthesis evaluation [e.g. (104)], the quality of synthetic speech was assessed, among other measures, by hearers’ judgments. Typically, hearers were asked to rate the naturalness and comprehensibility of the synthetic speech [cf. (104): 1012].
In our work, we used the concept of measuring naturalness and comprehensibility to evaluate the synthetic utterances. It should be noted that Wagner et al. (105) discussed the current state of the art in TTS evaluation and presented a new research program for speech synthesis evaluation in a paper published after we had collected the data for this study. The authors suggested that contextual appropriateness plays a crucial role in speech synthesis evaluation. They argued that the specific application and listening situation needs to be taken into account [cf. (105): 105].
For our research goal, however, we were interested in testing whether the articulatory synthetic utterances were perceived as natural. Our aim was not to evaluate the synthetic utterances, but to perceptually test whether the utterances were natural and understandable, in order to rule out that these dimensions function as confounding variables. Furthermore, the purpose of the fictive machine application in our experimental scenario remains too vague to assess contextual appropriateness.
In our previous work on uncertainty perception (106–108) different degrees of intended uncertainty were modeled with articulatory speech synthesis (33) and tested whether neurotypical adult hearers were able to discriminate between the degrees of uncertainty. The synthetic answers were part of a human-machine scenario in which the question was spoken by a human and the answer was the synthetic utterance. The acoustic cues rising intonation, pause and hesitation particle (“uh”) were systematically varied in Lasarcyk et al. (106) and in Wollermann et al. (107). Students from the University of Duisburg-Essen, as neurotypical hearers, were asked to judge the synthetic answers in terms of uncertainty and naturalness.9 In both works an additive principle of the uncertainty cues was described, i.e. the combination of two cues led to a higher level of perceived uncertainty than single cues. The study by Lasarcyk et al. (106) showed no significant difference between judgments when comparing the relative contribution of the single cues intonation vs. filler. Similarly, in Wollermann et al. (107), the single cues pause vs. filler were not rated significantly differently in terms of perceived uncertainty, but intonation was rated significantly more strongly regarding uncertainty than pause. Both Lasarcyk et al. (106) and Wollermann et al. (107) found no correlation between the ratings of uncertainty and the naturalness of the stimuli.
The material used in our pilot study (109) was based on the material of our previous studies (106, 107). In the following, when we refer to our pilot study we mean the study described by Bellinghausen et al. (109). However, we created new articulatory speech utterances with the revised version of Vocal Tract Lab (33) conveying different degrees of uncertainty. The answer to each question was generated by varying pause, intonation, and hesitation as acoustic cues. In the perception task, 28 neurotypical student hearers rated each answer on a rating scale in terms of uncertainty, naturalness and comprehensibility. The results indicated different contributions of acoustic cues to uncertainty perception. The effect of intonation and hesitation was more evident than the effect of pause. We observed an additive principle of the three cues, i.e. the more cues of intended uncertainty were activated, the higher was the perceived degree of uncertainty. The implications can be summarized as follows: In our study, we were able to model different degrees of intended uncertainty using articulatory speech synthesis by different combinations of pause, hesitation and intonation. Neurotypical adult hearers, i.e. students from the University of Duisburg-Essen, were generally able to discriminate the different levels in perception, although the relative contribution of the acoustic cues varied.
2 Method
In the current study, we aim to apply our experimental paradigm for measuring prosodic uncertainty in neurotypical hearers in our pilot study (109) to the investigation of prosody perception in autistic adult hearers. Thus, this study presents a feasibility study. We will present acoustic cues of uncertainty generated by articulatory speech synthesis to autistic adult listeners. To incorporate the developmental perspective, future work could modify the method to test autistic children and adolescents (see the Discussion).
2.1 Goal and research question
Our central research question was the following: Is there a group difference in the perception of uncertainty between hearers without and with ASD? We assumed that the prosodic marking of uncertainty in the speech signal has an effect on the perception on the side of the hearer. As mentioned above, we consider the attribution of uncertainty as part of the affective ToM with respect to a propositional content, here the answer given in a short question-answer scenario. Furthermore, we hypothesized that the marking of uncertainty is less dependent on the structure and semantics of the utterance than other prosodic phenomena such as focus [for an empirical investigation of focus theories see (30); 51]. Therefore, there is less interaction with syntactic and semantic processing and the information conveyed by prosody can hardly be induced by other linguistic information.
With our study we hope to contribute to the understanding of prosodic processing in autistic adult hearers by focusing on uncertainty as an emotional expression.
2.2 Hypotheses
Our primary hypothesis was as follows: There are significant differences in the perception of uncertainty between the ASD group and the NTC group. A low level of expressed intended uncertainty would be perceived as less uncertain by the ASD group than by the NTC group.
The secondary hypothesis was based on the results of our previous studies (106, 107) and was as follows: There would be a monotonic direct relationship between the number of prosodic uncertainty cues and participants’ ratings of uncertainty, regardless of group membership.
We used naturalness and intelligibility as quality measures for speech synthesis to see to what extent differences in naturalness (perception) can act as confounding variables. In our previous studies (106, 107) we only measured naturalness as a standard method for evaluating uncertain synthetic speech. In the current work, we include both naturalness and intelligibility as possible confounding variables.
The quality of speech synthesis may vary under different conditions. We include these two factors in addition to uncertainty in the listeners’ evaluation.
2.2.1 Material
We use the material that we have already tested in our pilot study (109). To express different intended levels of uncertainty, utterances generated by the articulatory speech synthesizer (33) were used. This allowed us to manipulate specific prosodic parameters while minimizing the influence of unintended variation compared to natural speech.10
We chose the articulatory speech synthesizer VocalTractLab 2.2 by Birkholz (33) to generate high quality speech sounds while manipulating the parameters of the time-varying laryngeal and supra-laryngeal actions [cf. (109): 39]. The synthesizer has several components. To simulate the articulation process, 23 parameters control the geometric 3D model of a male vocal tract (110). A self-oscillating model of the vocal folds (36) is controlled by six parameters to specify the following features: subglottal pressure, fundamental frequency, and the rest shape of the glottis. The movements of the models of the vocal tract and the vocal folds are controlled by a gestural score. In this way, it is possible to manually adjust the movements for each word and to use different prosodic features for speech generation [cf. (109): 39].
In contrast to the articulatory speech synthesizer used here, state-of-the-art unit selection or neural synthesizers usually do not allow the individual manipulation of prosodic parameters such as f0 without causing involuntary changes in other prosodic parameters (e.g. voice quality) or articulation at the same time. This would make the specific assessment of the perceptual effect of individual prosodic parameters unreliable. Another way to manipulate prosodic parameters would have been to use a voice morphing method such as the change gender function in Praat (111), but this may introduce small acoustic artefacts in the manipulated signal, depending on the properties of the original signal (e.g. the irregularity of the voice).
The synthetic utterances were part of short question-answer pairs embedded in a human-machine interaction scenario designed to motivate the use of synthetic speech. The scenario was presented to the participants as follows: The question in German language was spoken by a natural voice (Was siehst Du?/What do you see)? and asked by a research assistant who showed pictures of fruit and vegetable objects to an image recognition robot. The synthetic answer, such as Bananen/Bananas, was given by the robot. The robot recognized the items with a certain level of confidence and was able to express uncertainty about the recognition in its answer. The critical stimuli were the following trisyllabic one word sentences in German: Bananen/bananas, Limetten/limes, Melonen/melons, Tomaten/tomatoes [cf. (109) 40]. We have opted for one-word sentences because they represent the smallest meaningful unit for an answer. In total, there were nine different levels of intended uncertainty, i.e. all possible combinations of the three cues pause, hesitation and intonation [see (Table 1)]. In addition, the following one-word phrases were used as distractors (without uncertainty cues) to the synthetic speech signal in order to minimize learning effects when the recipients judged the critical stimuli: Birnen/pears, Blaubeeren/blueberries, Bohnen/beans, Erdbeeren/strawberries, Gurken/cucumbers, Knoblauch/garlic, Mandarinen/mandarins, Orangen/oranges and Paprika/paprica [cf. (109) 40].
Following Bellinghausen et al. [ (109): 40], we describe below the three cues pause, hesitation and intonation used to generate the experimental stimuli.
Pause: This cue refers to the time between the question and the answer. For each level of intended uncertainty, a default silent pause of 1 s was used between the question and the answer. When the pause was activated (pause[+]), we used either a silent pause of 4 s as strongly marked pause or a filled pause,11 i.e. the hesitation äh/uh with a duration of 0.37 followed by a silent pause of 3.632 s giving a total duration of 4 s [cf. (109): 40].
It has to be noted that the pause can have other functions than expressing uncertainty. In this scenario, it could also be interpreted as the robot’s processing time while producing the synthetic utterance. In our previous study (109) it emerged from the text comments that the robot was obviously considered to be uncertain. However, due to the close relationship between uncertainty and processing time, these two aspects cannot be separated.
Hesitation: The hesitation particle äh/uh was either present (hes[+]) or absent (hes[-]) [cf. (109) 40].
Intonation: The intended level of certainty was expressed by a falling contour with a difference of 8 ST (semitones) between the highest pitch on the stressed syllable of the word and the lowest pitch at the end of the utterance. In addition, two intonation contours were used to express intended uncertainty. In the level Into1, the pitch of the last syllable rises by 8 ST (semitones) above the lowest pitch in the first syllable for moderate uncertainty, and in Into2 it rises by 13 ST for intended strong uncertainty [see also (109) 40]. Different intonation contours for the critical stimulus Bananen are shown in Figures 1–3. The pitch contour on the left side is the question uttered by a human speaker. On the right the pitch contour of the synthetic answer is shown.
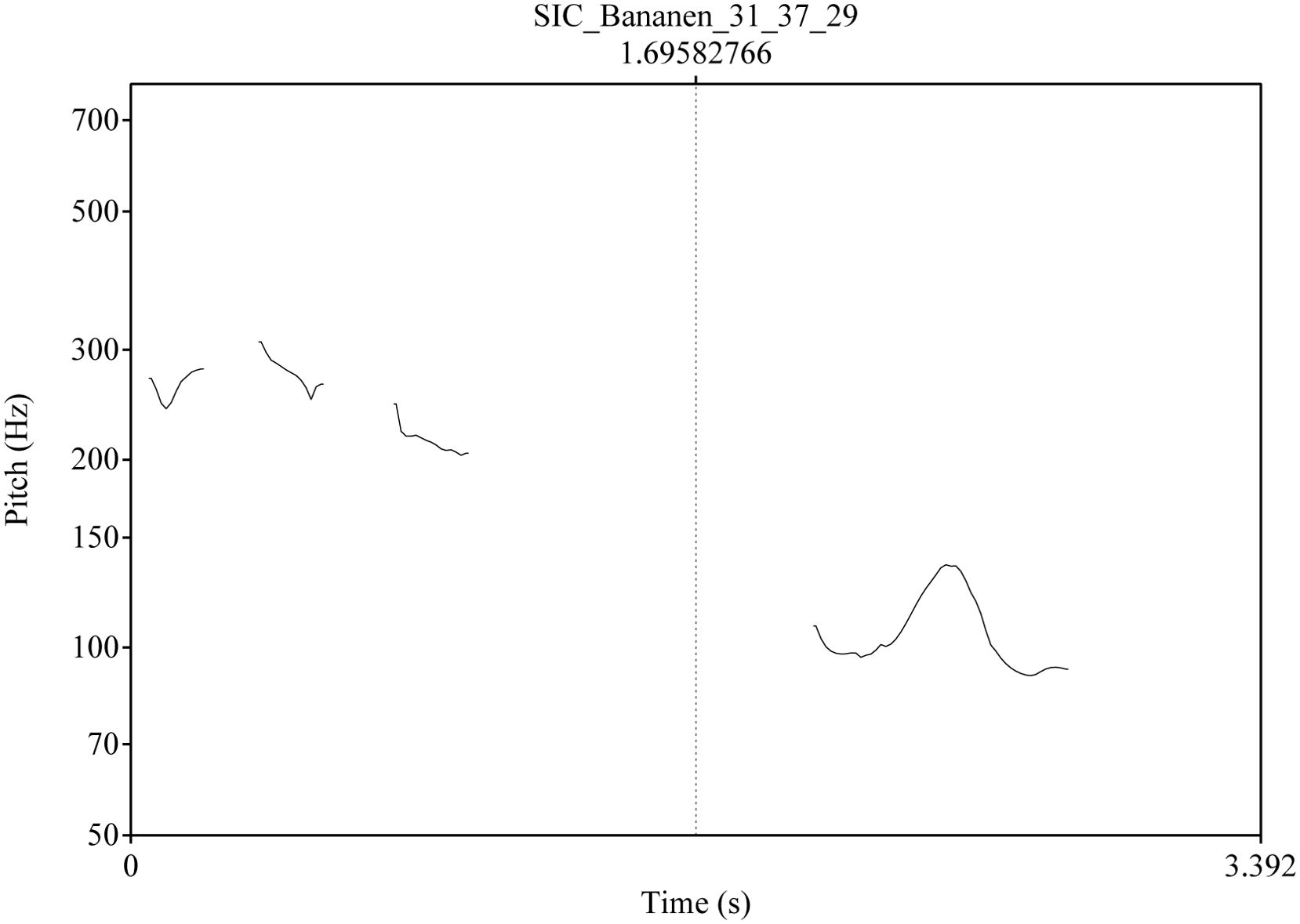
Figure 1. Intonation contour for the question “Was siehst Du/What do you see?” (left side) and for the answer Bananen/Bananas; level: Certainty (Cer) [see also (109): 41].
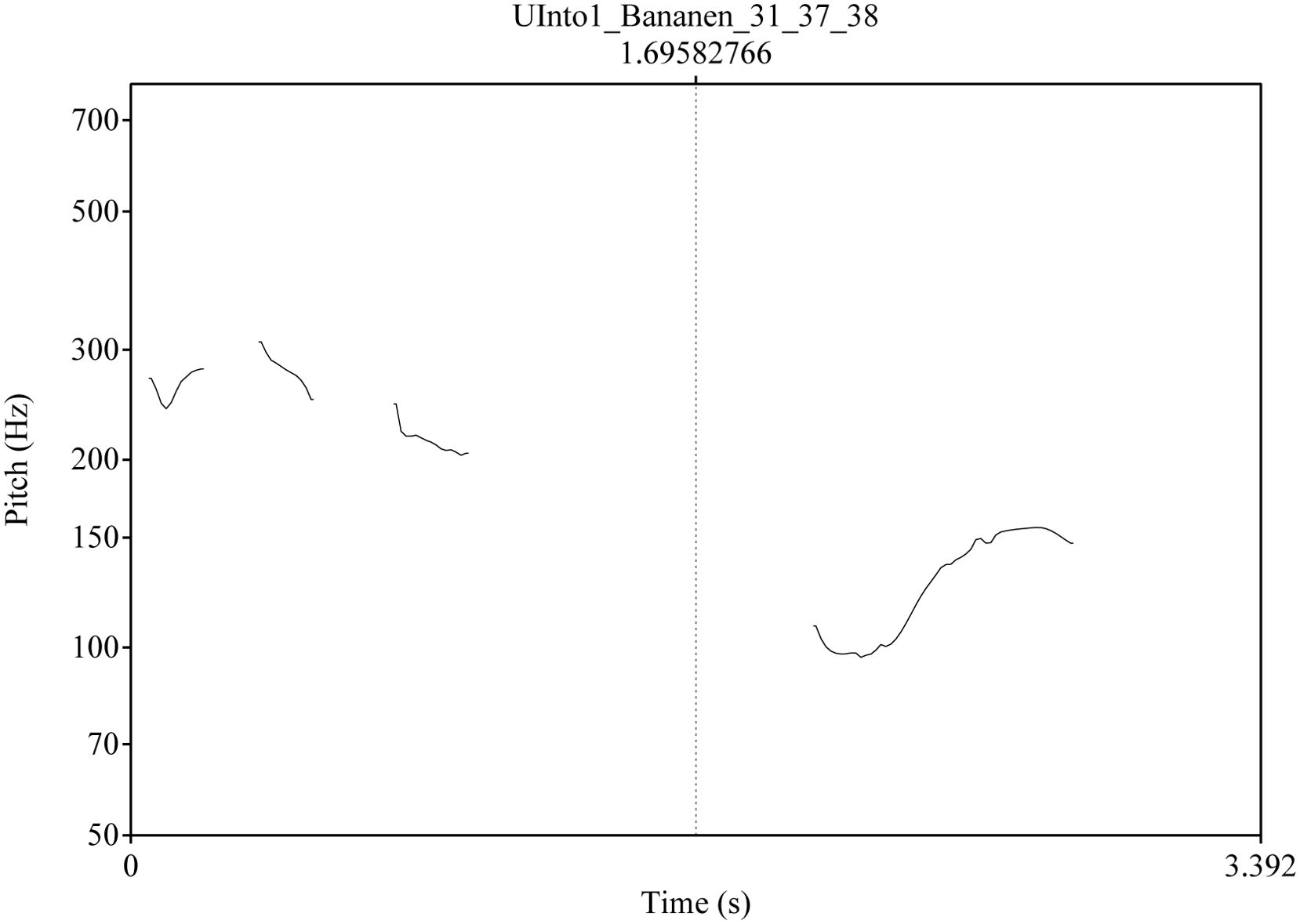
Figure 2. Intonation contour for the question “Was siehst Du/What do you see?” (left side) and for the answer Bananen/Bananas; level: Intonation 1 (int) [see also (109): 41].
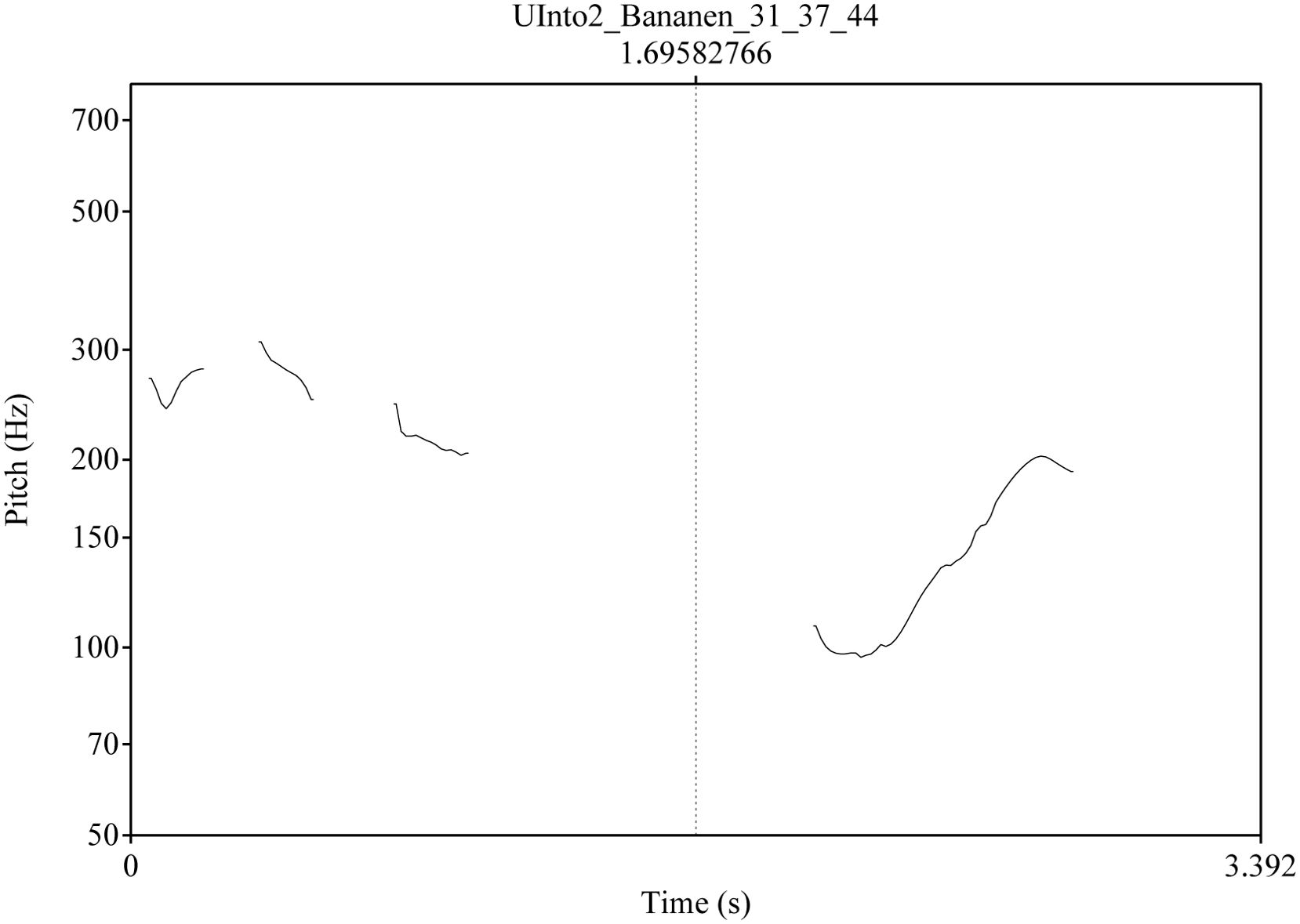
Figure 3. Intonation contour for the question “Was siehst Du/What do you see?” (left side) and for the answer Bananen/Bananas; three intended levels of uncertainty:; level: Intonation 2 (Into2) [see also (109): 41].
The number of critical stimuli was 36 (4 one-word utterances x 9 conditions). There were also 9 distractors and one practice trial Was siehst Du?/What do you see? The stimulus Rosinen/Raisins was presented at the beginning of the experiment. In order to minimize the influence of participants’ learning effects on their perceptual judgments, we constructed four task sets. Each critical item occurred only once within the four sets. Thus, each task set consisted of the practice trial, nine critical items complemented by nine distractors; the order of presentation of critical trials and distractors was randomized in advance. In this way, each participant had to work on one task set of 19 trials with question-answer pairs. Within each group, the four task sets were counterbalanced across participants (see Appendix for the experimental design). As we wanted to use as many potentially relevant questionnaires and control tests as possible in the feasibility study, we had to limit the number of trials in the prosody test to n=1 per condition, so that the experimental session would not be too long and become too strenuous, especially with regard to our patients.
2.2.2 Participants
56 participants (age range: 18-65, IQ > 80) with German as their first language took part in the study. The ASD group consisted of 28 adults (12 female, 16 male) diagnosed according to ICD-10 criteria (F84.0 Childhood autism, F84.1 Atypical autism, F84.5 Asperger syndrome). Only for the ASD group the ADOS-2, Module 4 was used (scale Communication + Social Interaction Total: M=8.04, SD=4.46). There were also 28 neurotypical adults (14 female) in the NTC. As shown in Table 2, there were no significant group differences in terms of age, gender, and IQ. In terms of autistic symptomatology, the ASD group had significantly higher values on the two self-report measures SRS-2 Adult Self-Report (ASD: M=112.50, SD=28.50; NTC: M=33.89, SD=19.07; t(54)= 12.13, p <.001) and the AQ [ASD: M=38.61, SD=7.16; NTC: M=13.29, SD=6.42; t(54)= 13.93, p <.001)].
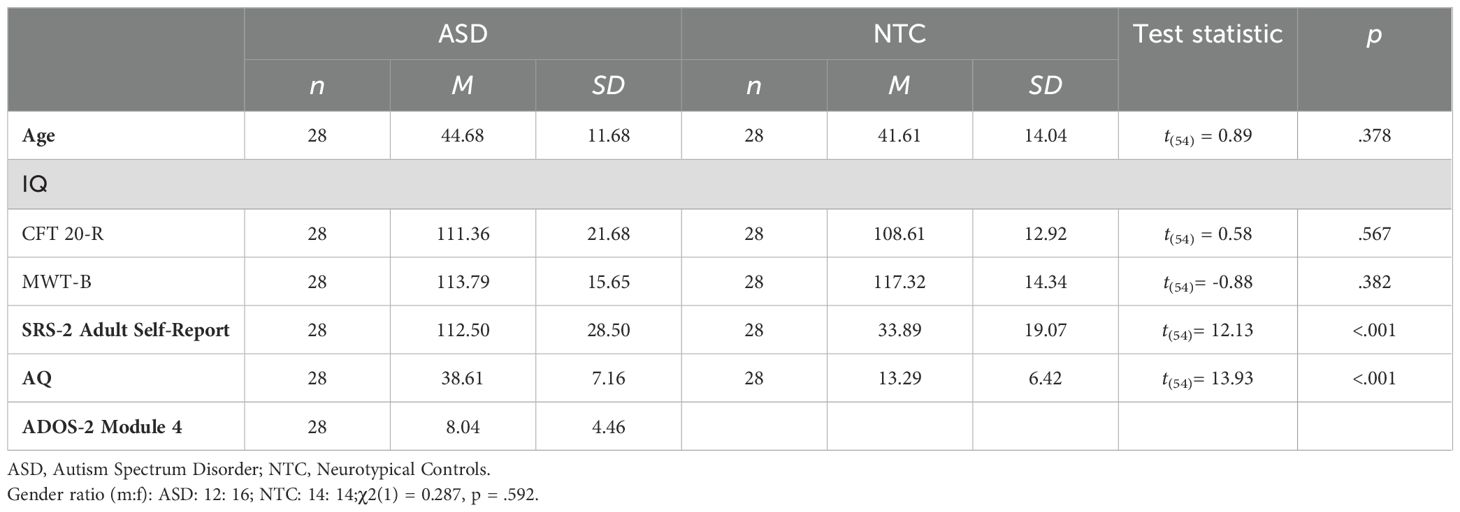
Table 2. Sample characteristics: Age, gender, IQ, and autistic symptomatology for the ASD and the NTC group.
Participants were screened for eligibility with regard to inclusion and exclusion criteria prior to the study. Exclusion criteria for the study participants were an IQ < 80, non-native speaker of German, as well as an acute depressive episode, psychotic symptoms or suicidal tendencies.
Regarding the language abilities of the autistic participants, it is noted that they completed the CFT 20-R and MWT-B test [see (Table 2)].The Basic Intelligence Test (CFT) is considered culturally fair because it is based on non-verbal and illustrative test tasks. It measures basic mental ability (g-factor) independent of socio-cultural and educational influences. The CFT 20-R consists of two similarly structured test parts with the four subtests: Series Continuation, Classification, Matrices, and four Topological Conclusions. The Multiple Choice Vocabulary Intelligence Test (MWT-B) measures general vocabulary. Intelligence levels. For each item, the candidate has to find the correct German word from five given words and four nonsense words.
The study took place at the Department of Psychiatry and Psychotherapy of the Medical Center - University of Freiburg, Germany. Participants with ASD were recruited through the outpatient clinic or from inpatient wards or after their discharge, and through the website and notices of the autism outpatient clinic.
2.2.3 Procedure
The following instruments [see (Table 3)] were performed as part of the study: both the ASD and the NTC group completed self-report questionnaires on the AQ, EQ, SRS-2 Self-Report, BDI-II, BVAQ and FQLP prior to the examination. Furthermore, the SCL-90-S was administered to the NTC group only. Interviews about the psychotic symptoms and two IQ tests, the CFT 20-R and the MWT-B, were also administered to both groups before the examination. In addition, the diagnosis of the ASD group was confirmed by the ADOS-2.
The AQ, EQ, SRS-2 self-report and FQLP questionnaires were used to characterize autistic symptoms. The BVAQ was collected because of possible alexithymic symptoms, which are more common in ASS. The ADOS was only collected from the ASS group in order to describe the communicative and social-interactive behavior of this group. The SCL-90 was only used in the NTC group to detect signs of psychiatric disorders.
Participants were informed of the aim and the procedure of the study, and a short interview was conducted to exclude possible psychotic symptoms for the participants with ASD. All participants signed an informed consent. The study was approved by the ethics committee (EK-Freiburg: 558/17). It was conducted in accordance with the Declaration of Helsinki. The experimental session included a prosody test, a complementary audiometry test, a pitch discrimination task and a pitch change task assessing sensory pitch perception. Data were collected during a two-hour individual session with the participants.
2.2.3.1 Prosody test
In the prosody test, participants were presented with short question-answer pairs consisting of the natural language question Was siehst Du?/What do you see? and the articulatory synthetic utterance serving as an answer, e.g. Bananen/bananas. The synthetic response instantiated one of the nine experimental conditions in which the three cues intonation, pause, and hesitation were either present or absent [see (Table 1)].
The prosody test was presented to the participants via a computer program (see Appendix for the experimental design). Each participant completed 19 trials (nine levels of intended uncertainty plus nine distractors following an example stimulus). Each question-answer pair was played only once. Participants were asked to rate a) uncertainty b) naturalness, and c) comprehensibility of the synthetic response on a 5-point rating scale (1 = uncertain/little natural/little comprehensible and 5 = certain/very natural/very comprehensible). In contrast to Bellinghausen et al. (109), the reaction time was also measured when rating the response.
As discussed in the introduction, we measure not only the perception of certain prosodic features in terms of perceived uncertainty, but also their effect on naturalness and comprehensibility.
2.2.3.2 Audiometry
An audiometry test from Electronica-Technologies was used to ensure that the prosodic stimuli used were reliably recognized by the participants, and that they had no significant hearing loss. Each ear was tested separately. Sine tones (250 Hz, 500 Hz, 1000 Hz, 2000 Hz, 3000 Hz, 4000 Hz, 8000 Hz) were presented at increasing loudness via headphones.
2.2.3.3 Minimal pitch discrimination and change
Following Globerson et al. (29), minimal pitch discrimination was used to investigate whether two sine tones of only slightly different frequency could be perceived as different. Thus, the level of the minimal perceived tone difference could have a significant influence on the perception of prosodic intonation [see also (29)]. The difference between the two tones amounted to 200 Hz at the beginning and was reduced to the minimum pitch difference perceived by the participant. Thus, if hearers can only perceive large differences between the reference and the comparison tone, this could have a significant impact on the perception of prosodic intonation [see also (29)].
The minimum pitch change detection for each participant was determined by assessing the course of a tone rising or falling in frequency. The test started with tone movements of 12 Hz up or down from the starting tone of 200 Hz. For reduction of the pitch change the same staircase function as for the pitch discrimination task was used according to Globerson et al. (29).
By testing pitch discrimination and pitch change detection, we wanted to ensure that basic auditory perception is not impaired in hearers with ASD without II and thus could be excluded from influencing prosody perception. Therefore, both pitch tests served as a kind of control condition in order to rule out the possibility that putative group differences could be explained by differences in mere low-level auditory processing.
2.2.4 Statistical analysis
For the sample characteristics, group differences in age and IQ were tested using t-tests, and group differences in gender were tested applying the chi-square test. Since significant deviations from normality could be expected for all other variables (minimum pitch discrimination and change, ratings of uncertainty, naturalness and comprehensibility, and their corresponding response time variables), we conducted robust tests as described and recommended by Field and Wilcox (112), Mair and Wilcox (113) and Wilcox (114).
Robust methods address two key properties of a statistical test: the probability of a false positive, also known as a Type I error, and power, the probability of detecting true differences between groups (or a true association between two or more variables). They attempt to overcome serious drawbacks when assumptions of conventional methods such as ANOVA are violated, in order to avoid misleading results and interpretations [see (115) for more details]. To our knowledge, robust methods do not differ from classical non-parametric techniques (such as the Wilcoxon-Mann-Whitney test) in terms of controlling for item and individual variability.
For the analyses of minimum pitch discrimination and change, we had a one-factorial design with “diagnostic group” (ASD, NTC) as an independent factor. For the ratings of uncertainty, naturalness and comprehensibility, and their corresponding response time variables, we used a 2 x 8 design with the independent factor “diagnostic group” (ASD, NTC) and “prosodic condition” (Cer, Hes, Pau, Into2, HesPau, HesInto2, PauInto2, PauInto2Hes; see Table 1 for a description of these conditions) as the dependent factor. For some analyses, we also considered the distractor trials as an additional prosodic condition and Into1 as a “milder” condition for an intonation (see above) that was not combined with the other two cues pause and hesitation. Therefore, only Into1 was statistically tested against Into2.
The 2 x 8 design was analyzed with a two-way mixed design robust test statistic [bwtrim, F-like test values, see (112): 29-30; (113): 479]. t1waybt is a robust one-way alternative with an outcome of F-like values for between-subjects effects and effect sizes [see (112): 28-29]. yuend is used as a robust alternative for a dependent t-test that also outputs the explanatory measure of effect size ξ. Similar to Pearson correlations, ξ = .10,.30, and.50 correspond to small, medium, and large effect sizes, respectively [ (112: 25-26; (113): 458] [see also (114): 506-511 for three factor design, 2 x 2 x 8].
All robust tests were performed with the same following parameters (except bwtrim without bootstrapping): trimmed mean with 20% trimmed scores (tr = 0.2), the modified one-step estimator (est = “mom”), and the number of bootstrapping samples of 5000 (nboot = 5000). In order to control the overall probability of a Type I error (false positive) for multiple hypothesis tests, post-hoc tests are reported after Bonferroni adjustment.
All statistical analyses were performed with R version 4.1.2 using the R package WRS2 version 1.1-3 with its collection of robust statistical methods. A significance level of α = .05 was used for hypothesis testing.
3 Results
3.1 Audiometry test
All participants in the study had unaffected hearing abilities at the frequencies measured.
3.2 Minimal pitch discrimination and change
There were no significant differences between the ASD and NTC groups in either pitch discrimination or pitch change perception or reaction time [see (Table 4)]. However, the ASD group descriptively achieved lower values for pitch discrimination and change (in Hertz) than the NTC. There was only a minimally longer response time for pitch change detection in the ASD group than in the NTC. No significant differences were observed.
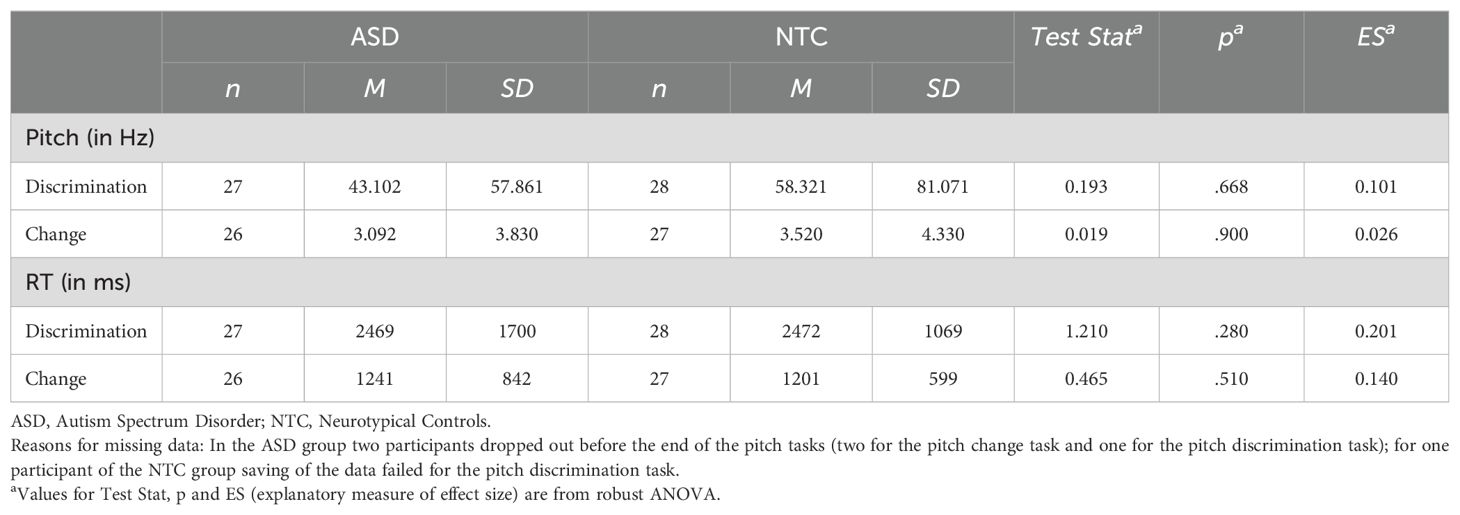
Table 4. Test results for pitch variation and for pitch change. Minimum pitch discrimination in Hertz and reaction times in milliseconds.
3.3 Prosody test
3.3.1 Perception of uncertainty
3.3.1.1 Distractor analysis
Before describing the results for the ratings of the critical stimuli in terms of perceived uncertainty, naturalness, and comprehensibility we report on the ratings of the distractor items. As mentioned above, we used 10 distractor items, all of which were exclusively generated in an intended certain way of speaking. As shown in Table 5, there was no significant difference between the ratings of uncertainty for the distractor trial condition Dist and the prosodic uncertainty condition Cer (M = 4.20, SD = 0.65 vs. M = 4.20, SD = 0.97; robust test statistic = -0.71, p = .482) for the whole sample, and also the pattern of the results of these two with all other conditions is remarkably similar [see (Table 5)]. This was also true for the ratings of uncertainty within the ASD group (Dist: M = 4.31, SD = 0.63, Cer: M = 4.21, SD = 0.92; robust test statistic = -0.18, p = .861) as well as in the NTC group (Dist: M = 4.15, SD = 0.67, Cer: M = 4.14, SD = 1.04; robust test statistic = -0.91, p = .375).
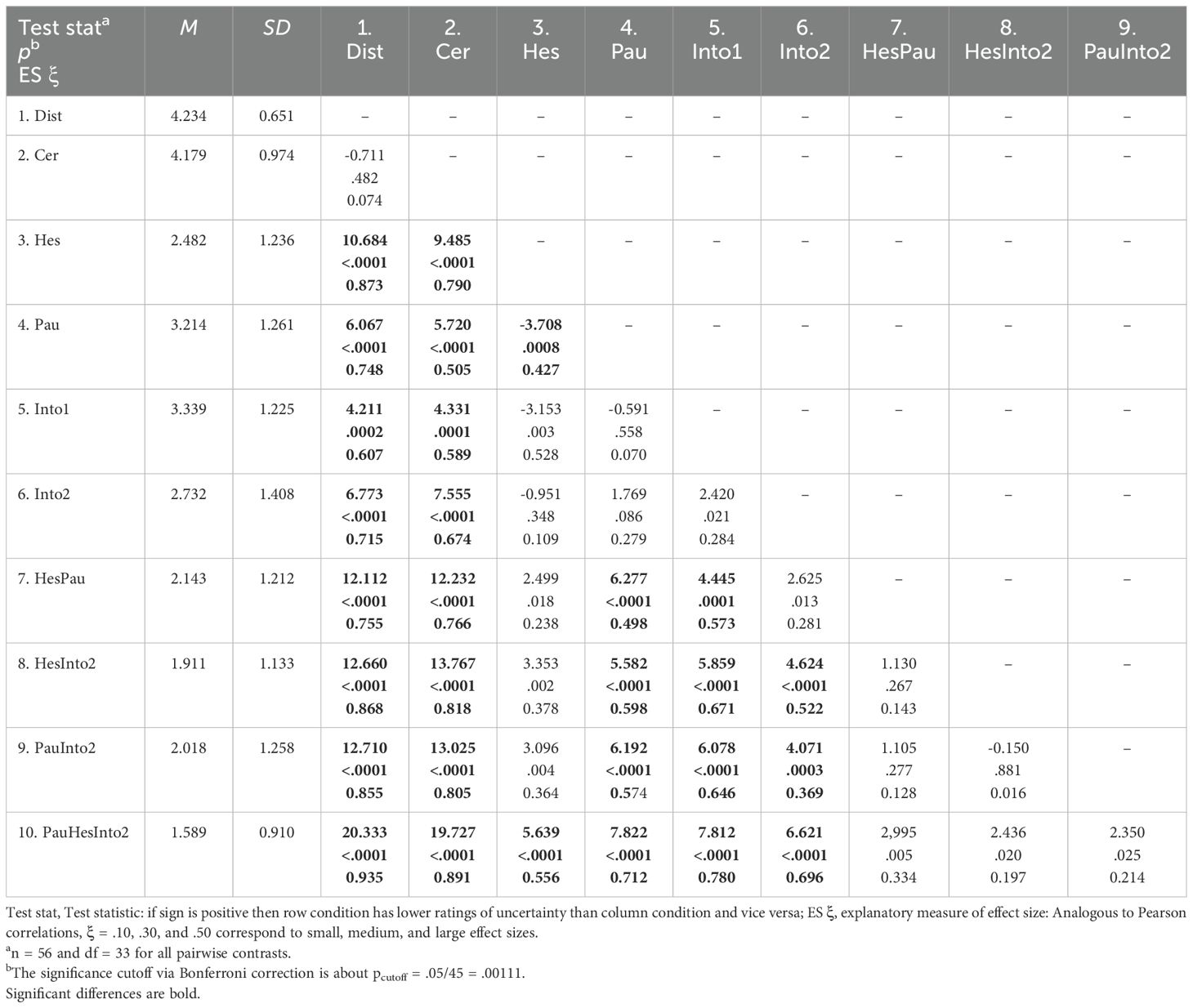
Table 5. Pairwise comparisons of ratings of uncertainty between prosodic uncertainty conditions (independent of diagnostic group).
With respect to response time for the ratings of uncertainty, participants needed more time for the distractor trials than for the utterances in the condition Cer (M = 4511, SD = 2365 vs. M = 4230, SD = 4508; robust test statistic = 2.80, p = .008, ES = 0.27). This difference was also significant within the ASD group (Dist: M = 4966, SD = 2689; Cer: M = 4134, SD = 4866; robust test statistic = 2.95, p = .009, ES = 0.45), but not within the NTC group (Dist: M = 4056, SD = 1933; Cer: M = 4325, SD = 4208; robust test statistic = 0.95, p = .360, ES = 0.14). The distractors differ from the stimuli words in their syllable structure. These phonological discrepancies could explain the differences in reaction times.
3.3.1.2 Ratings of uncertainty of the 2 x 8 design
In the statistical analysis of the ratings of uncertainty with robust ANOVA, the main effect of diagnostic group was not significant (robust test statistic F(1, 32) = 2.10, p = .160), whereas the main effect of prosodic uncertainty conditions was significant (robust test statistic F(7, 25) = 43.20, p <.0001). However, the interaction between these two factors was far from being significant (robust test statistic F(7, 25) = 1.30, p = .27). In Figure 4, means of the ratings of uncertainty for all factorial combinations are shown. Due to the non-significant interaction, post-hoc comparisons are only reported for the different levels of the significant condition main effect and for the hypothesized group main effect, but not for the non-significant interaction.
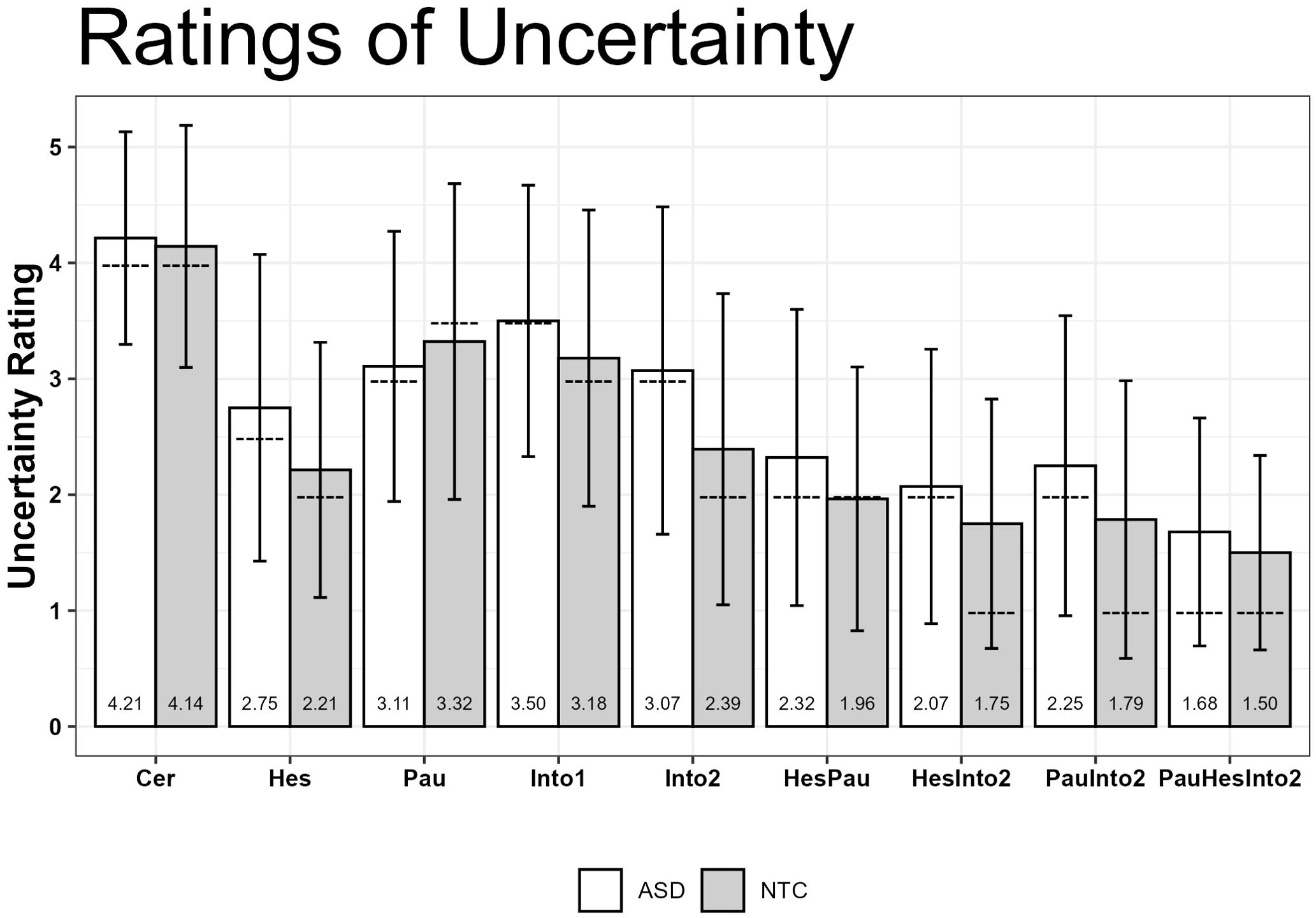
Figure 4. Means of uncertainty ratings (1=uncertain, 5=certain), dashed lines denote the median. Abbreviations for the prosodic uncertainty conditions are explained in Table 1.
In Table 5, all pairwise comparisons between the prosodic uncertainty conditions (including the distractor trials) are reported. There are noteworthy differences between the condition CER (and, as already mentioned above, the distractors DIST) and all the other prosody conditions. Also, most of the 2-cue prosody conditions (HesPau, HesInto2, PauInto2) were judged to be more uncertain than the 1-cue prosody conditions (Hes, Pau, Into1, Into2). The 3-cue prosody condition PauInto2Hes which had descriptively the lowest mean, elicited significantly lower ratings of uncertainty than all the 1-cue prosody conditions, whereas differences to the 2-cue prosody conditions were not significant after Bonferroni correction. All significant differences between conditions had medium up to very large effect sizes (all ξs >.37).
The top part of Table 6 shows all the contrasts in ratings of uncertainty between the two groups ASD and NTC for each prosodic uncertainty condition. The corresponding means are shown in Figure 4. As we had nine different conditions and three combined comparisons the number of comparisons was twelve, and therefore our p-values should be below.05/12=.004166 in order to be considered as significant after Bonferroni correction. Descriptively, the ASD group had higher ratings of uncertainty in all conditions except for the condition Pau. However, the most pronounced between group difference for the combined condition “All cues”, was not significant after Bonferroni correction.
3.3.1.3 Response times of ratings of uncertainty for the 2 x 8 design
In the statistical analysis of the response times of the ratings of uncertainty with robust ANOVA, the two main effects and the interaction were not significant (main effect “diagnostic group”: robust test statistic F(1, 30) = 3.40, p = .074; main effect “prosodic uncertainty condition”: F(7, 22) = 2.30, p = .061; interaction: F(7, 22) = 1.70, p = .163).
Descriptive statistics for all contrasts between the two groups ASD and NTC for each prosodic uncertainty condition are presented in the lower part of Table 6. As can be seen, the ASD group needed descriptively more time to reach ratings of uncertainty in almost all prosodic uncertainty conditions (except for the condition “Cer”). The largest difference can be seen in the condition HesInto2: The ASD group took almost twice as long (4683 ms) as the NTC group (2629 ms). Note that this difference is no longer significant after Bonferroni correction (robust test statistic = 9.90, p = .001, ES = 0.66).
When integrating the data on perceptual judgments for single cues, two combined cues, and all the cues, the following was observed: the mean for the ASD group was always higher compared to the NTC, i.e. the ASD group needed more time to rate the different levels of uncertainty than the NTC, but the differences were no longer significant after Bonferroni correction.
3.3.1.4 Exploratory analyses: effect of gender, IQ, and severity of autistic symptoms on the processing of uncertainty cues
In order to assess whether or not other variables might influence the processing of uncertainty cues, we also conducted exploratory statistical analyses with the possible impact factors of gender, IQ, and degree of autistic symptom severity.
IQ. Concerning the IQ, we computed Spearman rank-order correlations (rs) for both IQ measures (CFT 20-R and MWT-B) with the ratings of uncertainty and also for the response times within each experimental uncertainty cue condition for the total sample and additionally also for the NTC and ASD groups separately. For the IQ measures, we found no significant Spearman rank-order correlations between IQ and ratings of uncertainty for the total sample (CFT 20-R: all rs in [-.167; +.160], all ps >.219; MWT-B: all rs in [-.139; +.092], all ps >.309) as well as for both groups (ASD: CFT 20-R: all rs in [-.288; +.366], all ps >.055; MWT-B: all rs in [-.202; +.067], all ps >.302; NTC: CFT 20-R: all rs in [-.157; +.369], all ps >.053; MWT-B: all rs in [-.125; +.259], all ps >.183).
There were no significant correlations for the response times of the ratings of uncertainty with the IQ measure CFT 20-R (Total: all rs in [-.199; +.145], all ps >.141; ASD: all rs in [-.364; +.100], all ps >.057; NTC: all rs in [-.325; +.350], all ps >.068). Similarly, for the MWT-B, almost all correlations were not significant except for two coefficients in the NTC group (Total: all rs in [-.083; +.156], all ps >.251; ASD: all rs in [-.326; +.166], all ps >.091; NTC: all rs in [+.060; +.459], rs = +.459, p = .014 in condition Hes and rs = +.459, p = .014 in condition PauInto2Hes, all other ps >.120). It should be noted that all mentioned p-values are uncorrected with respect to multiple testing.
Degree of autistic symptom severity. As the degree of autistic symptom severity is strongly associated with the diagnostic group membership (see Table 2), it is useful to check for correlations within diagnostic groups only in order to assess whether or not this variable has an additional influence on the processing characteristics of uncertainty cues. For the ratings of uncertainty with the autistic symptom severity measure SRS-2 Adult Self-Report there were no significant correlations except for the conditions Hes, Pau, and HesPau within the ASD group, and for the condition Cer within the NTC group (ASD: all rs in [-.314; +.627], rs = +.487, p = .009 in condition Hes, rs = +.627, p <.001 in condition Pau, rs = +.498, p = .007 in condition HesPau, all other ps >.100; NTC: all rs in [-.409; +.253], rs = -.409, p = .031 in condition Cer, all other ps >.063). For the autistic symptom severity measure AQ, there were no significant correlations except for the condition HesPau for the ASD group (ASD: all rs in [-.237; +.404], rs = +.404, p = .033 in condition HesPau, all other ps >.062; NTC: all rs in [-.258; +.261], all ps >.180). No significant correlations were found for the ADOS-2 (ASD: all rs in [-.280; +.276], all ps >.149).
There were no significant correlations for the response times of the ratings of uncertainty with the autistic symptom severity measure SRS-2 Adult Self-Report, except for one condition within the NTC group (ASD: all rs in [-.233; +.126], all ps >.233; NTC: all rs in [-.023; +.417], rs = +.417, p = .027 in condition Into1, all other ps >.167). No significant correlations were found for the autistic symptom severity measure AQ (ASD: all rs in [-.035; +.327], all ps >.090; NTC: all rs in [-.015; +.356], all ps >.063): For the ADOS-2, only one significant correlation with response times was found in the condition Cer (ASD: all rs in [+.080; +.438], rs = +.438, p = .021 in condition Cer, all other ps >.094).
Gender. In order to assess a potential influence of gender on the processing of uncertainty cues, we added gender as an additional independent factor in the robust ANOVA. There was no significant main effect of gender on ratings of uncertainty (F(1, 999) = 1.46, p = .228), nor were there any significant interactions of the other factors with gender (gender x diagnostic group: F(1, 999) < 1; gender x prosodic uncertainty condition: F(9, 999) < 1; gender x diagnostic group x prosodic uncertainty condition: F(9, 999) < 1). A similar pattern was found for response times: No significant main effect for gender (F(1, 999) = 2.30, p = .130) and no significant interactions of the other factors with gender (gender x diagnostic group: F(1, 999) < 1; gender x prosodic uncertainty condition: F(9, 999) < 1; gender x diagnostic group x prosodic uncertainty condition: F(9, 999) < 1).
In summary, the exploratory analyses revealed no strong evidence that gender or IQ are reliably related to the processing of prosodic uncertainty cues. There was weak evidence that severity of autistic symptoms may play an additional role beyond mere diagnostic group membership.
3.3.2 Perception of naturalness and comprehensibility
In section 2.2, the (perceived) quality of the synthetic stimuli was mentioned as a possible confounding variable. In the following two subsections we look at the two quality measures naturalness and comprehensibility, and analyze whether there were differences between the two groups that could have influenced the differences in ratings of uncertainty.
3.3.2.1 Naturalness
The statistical analysis of the naturalness ratings using the robust ANOVA revealed neither significant main effects nor a significant interaction (main effect “diagnostic group”: robust test statistic F(1, 32.872) < 1; main effect “prosodic uncertainty condition”: F(7, 24.970) = 2.24, p = .065; interaction: F(7, 24.970) < 1). In Figure 5, means of naturalness ratings are depicted for all factorial combinations. Further exploratory analyses for the prosodic uncertainty conditions revealed that the largest difference between conditions was found for the contrast Cer-PauInto2, which had the highest/lowest mean naturalness ratings (Cer: M = 3.41, SD = 1.30, PauInto2: M = 2.95, SD = 1.26; robust test statistic = 3.19, p = .003, ES = 0.28).
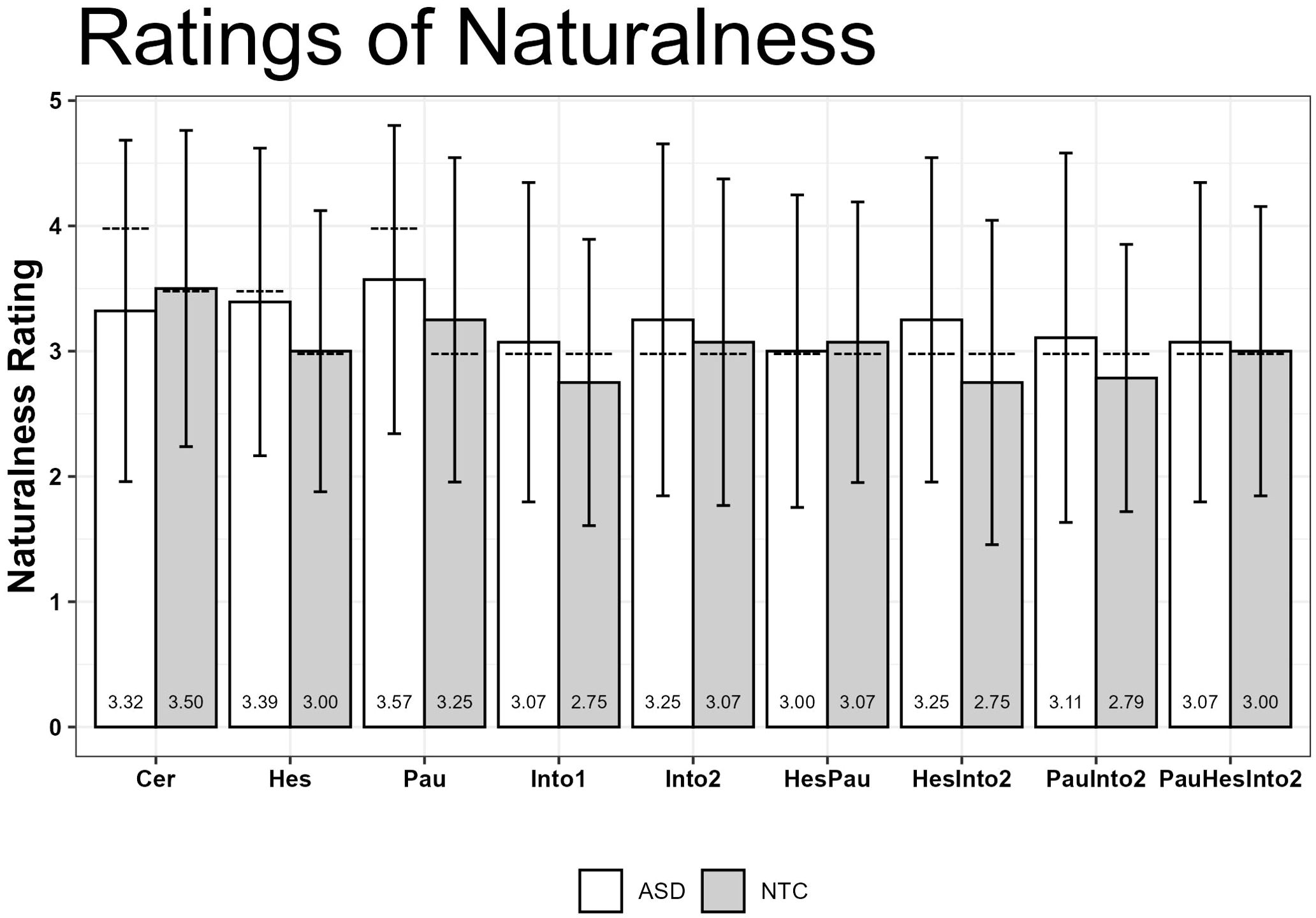
Figure 5. Means of naturalness ratings (1=little natural, 5=very natural), dashed lines denote the median.
The robust analysis of response times for naturalness ratings showed a significant main effect of “prosodic uncertainty condition”: F(7, 24.643) = 3.33, p = .012), whereas the main effect “diagnostic group” and the interaction were far from being significant (robust test statistic F(1, 32) < 1 and F(7, 25) < 1, respectively). Further exploratory analyses for the prosodic uncertainty conditions revealed that the largest response time difference between conditions was noted for the contrast Cer-PauHesInto2 that had the highest/lowest mean response times (Cer: M = 3155, SD = 2300, PauHesInto2: M = 4383, SD = 2922; robust test statistic = -3.70, p <.001, ES = 0.44).
3.3.2.2 Comprehensibility
Statistical analysis for the ratings of comprehensibility using the robust ANOVA revealed neither significant main effects nor a significant interaction (main effect “diagnostic group”: robust test statistic F(1, 32.647) = 3.21, p = .082; main effect “prosodic uncertainty condition”: F(7, 24.887) = 1.22, p = .331; interaction: F(7, 24.887) < 1). In Figure 6, means of comprehensibility ratings are shown for all factorial combinations. Further explorative analyses for the prosodic uncertainty conditions revealed that the biggest difference between conditions was noted for the contrast Cer-PauHesInto2, which had the highest/second lowest mean naturalness ratings (Cer: M = 3.89, SD = 1.11, PauInto2: M = 3.52, SD = 1.11; robust test statistic = 2.54, p = .016, ES = 0.25).
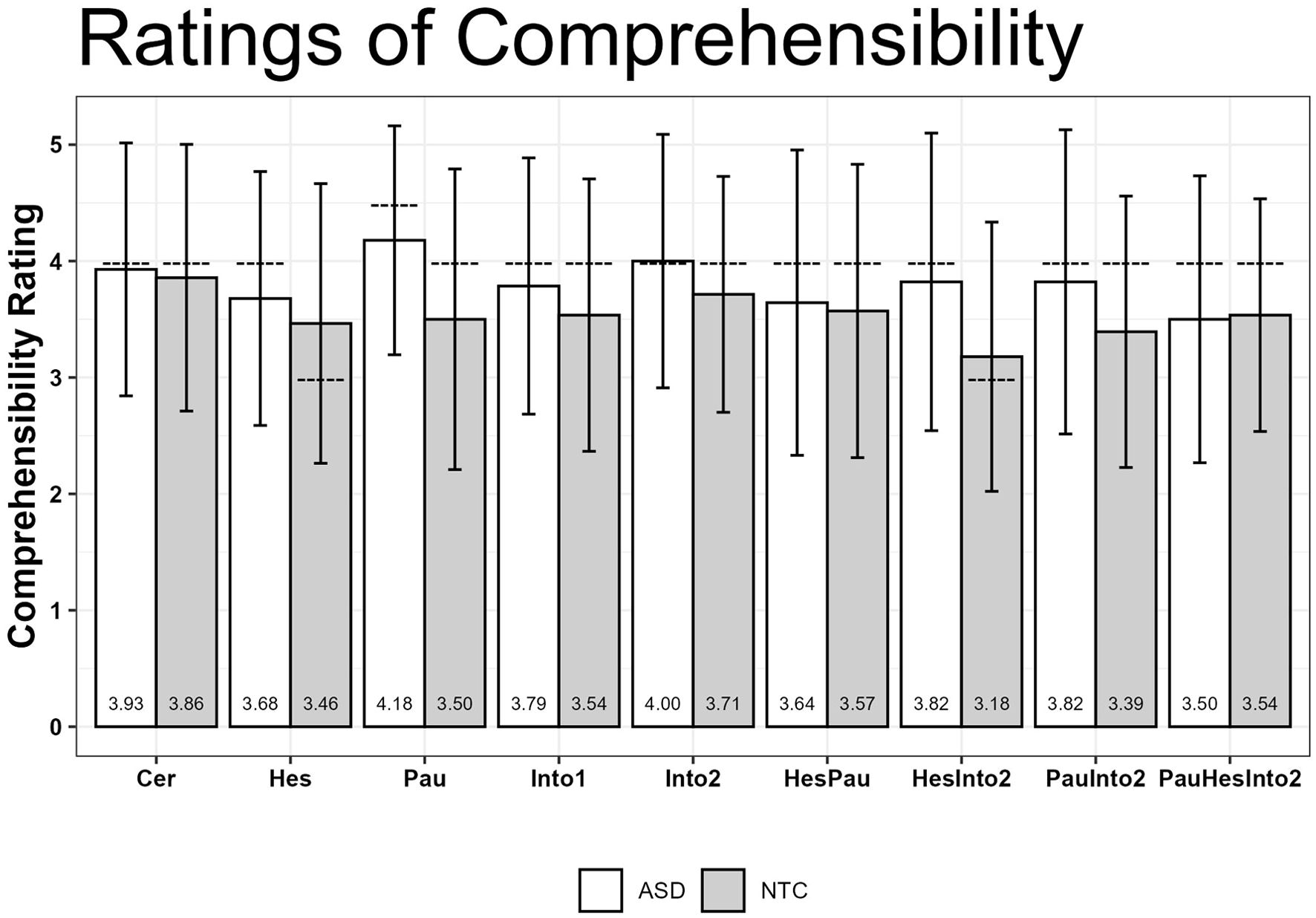
Figure 6. Means of comprehensibility ratings (1=little comprehensible, 5=very comprehensible), dashed lines denote the median.
The robust analysis of response times for comprehensibility ratings showed a significant main effect of “prosodic uncertainty condition”: F(7, 24.834) = 6.59, p = .0002), whereas the main effect “diagnostic group” and the interaction were not significant (robust test statistic F(1, 29.434) = 1.10, p = .303 and F(7, 24.834) = 1.858, p = .120, respectively). Further exploratory analyses for the prosodic uncertainty conditions revealed that the largest response time difference between conditions was noted for the contrast Cer-PauHesInto2, which had the second highest/lowest mean response times (Cer: M = 3013, SD = 1975, PauHesInto2: M = 4608, SD = 3341; robust test statistic = -4.84, p <.0001, ES = 0.44).
We summarize the results for the assessment of the perceived naturalness and comprehensibility of the stimuli as follows: Our data show no significant group difference with respect to either dimension.
3.3.2.3 Correlation between perceived uncertainty and naturalness or comprehensibility
Fisher’s z-transformed correlations were calculated to test the relationship between perceived uncertainty and naturalness as well as perceived uncertainty and comprehensibility [see (Table 7)]. Significant group differences were found between the correlation of uncertainty and naturalness, i.e. in the ASD group the correlation is significantly lower than in the NTC group. This means that the processing of naturalness and uncertainty are more closely linked in the NTC group than in the ASD group which may indicate a different type of processing in ASD.
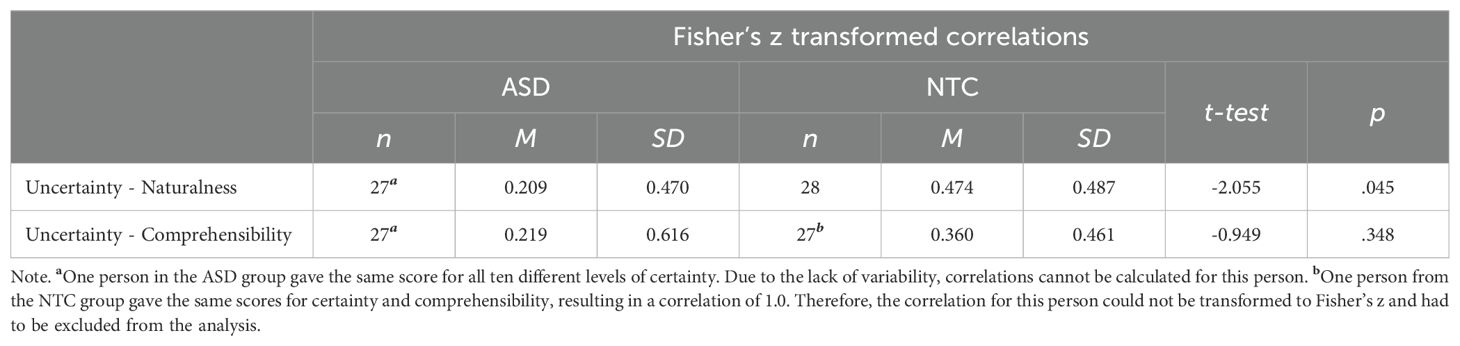
Table 7. Fisher’s z transformed correlations for uncertainty and naturalness and also for uncertainty and comprehensibility.
4 Discussion
4.1 Summary
In this study, we experimentally investigated how prosodic cues of uncertainty were perceived by hearers with ASD without II in comparison to a NTC group. The synthetic utterances were generated by an articulatory speech synthesizer (33). They were embedded in short question-answer pairs using a scenario in which the question was asked by a human in a natural voice. The robot gave a synthetic response in which the cues pause, intonation and hesitation were varied to generate different levels of intended uncertainty. The synthetic responses were rated by participants on rating scales for (i) uncertainty, (ii) naturalness, and (iii) comprehensibility. Reaction time of the rating was also measured. In addition, a complementary audiometric test, a pitch discrimination test and a pitch change test were performed.
The results for the level Hesitation combined with Intonation 2 showed a group difference in reaction times to judge uncertainty perception: the ASD group took longer for the judgment than the NTC group. Note that this difference is no longer significant after Bonferroni correction. All other levels of uncertainty were not reliably different between the two groups (all ps >.10). With the exception of pause, all judgments were reported as more certain in average in the ASD group. In addition, the intended levels of uncertainty showed a tendency for longer reaction times in the ASD group.
No significant difference was found between the ASD and NTC groups in the pitch discrimination and pitch change task for baseline discrimination. Although pitch differences were perceived equally well, the prosodic cues tended to be interpreted differently in terms of uncertainty perception: the intended prosodic cues of uncertainty influenced the perception of hearers less in the ASD group than in the NTC group. However, the ASD group showed longer reaction times than the NTC group. A possible explanation could be that a higher cognitive load was required for the hearers with ASD without II. It is assumed that hearers in the NTC group processed the prosodic cues automatically and with less cognitive effort, allowing them to make their ratings of uncertainty more quickly.
The differential correlation effect, i.e. that ASD individuals show a lower correlation between their ratings of naturalness on the one hand and uncertainty on the other, can be taken as evidence that the co-processing of naturalness and uncertainty is not as tightly linked in the ASD group compared to the typical co-processing of uncertainty cues with the naturalness of the utterance. This weaker relationship would be consistent with weak central coherence accounts of autism [e.g. (10)].
4.2 Limitations and future directions
Due to the design of the study, there were only few observations per participant, which means that in the current study there were significantly fewer trials per condition in the responses to uncertainty perception than in the pilot study (109). It is possible that the few observations from participants and the resulting study design had an impact on the results and could explain the non-significant differences in uncertainty judgments between the ASD group and the NTC group in our data. The reason for presenting only a subset of the stimuli to the participants was to minimize learning effects of participants. Previous experimental research on the role of prosody in pragmatic focus interpretation and possible learning effects is described in Fisseni (30), and Wollermann (51).
As a consequence of our feasibility study, the number of trials per condition could be increased and the test conditions could be adjusted in order to collect more data and verify the results. We could also reduce the number of psychological and psychiatric tests in order to save time and cognitive capacity. In particular, the tests for minimal pitch discrimination and pitch change could be omitted, as we have not found correlations between baseline auditory abilities and prosody perception in ASD. Instead, we would like to focus on the presentation of the critical stimuli for uncertainty perception by further minimizing learning effects.
It is possible that the order of presentation of the stimuli has an effect on recipients’ judgments, i.e. the stimulus presented first may be judged differently from stimuli presented later due to possible learning effects. Furthermore, in future research, we could focus on testing hearing abilities by including a group of participants with hearing impairments for comparison with the ASD and NTC groups.
In our approach, we used synthetic speech to generate the different utterances with intended uncertainty. In this way, specific prosodic parameters could be manipulated while the influence of unintended variation was minimized compared to natural speech, so we gave high priority to controllability and selective manipulation. A possible explanation for the non-significant effects of prosody on uncertainty perception could be the use of synthetic speech. It is conceivable that the effects of prosody on uncertainty perception might be more evident when natural speech is used. However, natural speech is less controllable than synthetic speech. In future work, it may be an option to consider neural synthesizers in comparison to our articulatory speech synthesizer for similar experiments. However, our primary goal was to achieve manipulability and controllability. It is an open question to what extent this can be guaranteed by neural synthesis.12
In future experiments we would like to further exploit the advantages of speech synthesis. In particular, we would like to explore the interplay between the three acoustic cues pause, intonation and hesitation to model different degrees of uncertainty in more detail. For example, it would be interesting to test a duration <4 seconds for the pause and also to use other hesitation particles besides uh, such as um. We also think it is important to experimentally investigate the role of lengthening in uncertainty perception, as pointed out by Betz et al. (93).
Another limitation is the material used in this study. We designed short question-answer situations in order to not only present the synthetic stimuli without embedded context. The answers were one word sentences. In future work, it would be important to test more complex sentences for ecological validity. However, it should be noted that the dialogues were simulated and did not approximate real-life dialogues, which induce uncertainty. This may have influenced the pattern of the results, i.e., the lack of significant interactions of the different variables with the group.
Next, we tested only adult participants. A wider range of ages, especially children and adolescents, might provide more information about the developmental trajectories of the ability to adequately process prosodic cues of uncertainty. Krahmer and Swerts (61) tested 7-8 year old neurotypical children and adults on the perception and production of uncertainty in question-answer situations. Uncertain utterances produced by adult speakers were recognized more accurately by both children and adult hearers than uncertain utterances produced by children. In addition, adults performed better than children in the recognition of uncertainty. We therefore plan to conduct further studies with children and adolescents. It should be noted that it would be necessary to modify the methodological approach with regard to cognitive abilities, especially in the case of children.
At this point, we would like to take a critical look at the role of ToM for prosody perception. For prosody processing, it may be important whether the ToM is built up automatically in an incidental or cognitive-compensatory manner, as we have explained above. If we assume that prosody perception supports the construction of ToM, there may also be incidental and compensatory prosody processing. This could explain differences in reaction times.
In addition, we only looked at individuals with ASD without II, so it is not possible to generalize the results to all individuals with a diagnosis of ASD.
In future work, we would like to conduct further perceptual experiments of affective prosody recognition, as the investigation of speech characteristics may display a promising novel biomarker and may contribute to the better understanding of mental disorders [cf. (117): 337; see also (118): 99].
Data availability statement
The original contributions presented in the study are included in the article/Supplementary Material. Further inquiries can be directed to the corresponding author.
Author contributions
CB: Conceptualization, Funding acquisition, Methodology, Visualization, Writing – original draft, Writing – review & editing. BS: Conceptualization, Data curation, Formal analysis, Methodology, Writing – original draft, Writing – review & editing. RR: Formal analysis, Methodology, Software, Visualization, Writing – original draft, Writing – review & editing. AR: Conceptualization, Methodology, Writing – original draft, Writing – review & editing. PD: Writing – original draft, Writing – review & editing. PB: Conceptualization, Methodology, Writing – review & editing. LT: Supervision, Writing – review & editing. TF: Conceptualization, Formal analysis, Methodology, Software, Writing – original draft.
Funding
The author(s) declare that financial support was received for the research, authorship, and/or publication of this article. The study was funded by the “Programm zur Förderung des exzellenten wissenschaftlichen Nachwuchses” of the University of Duisburg-Essen. We acknowledge support by the Open Access Publication Fund of the University of Duisburg-Essen.
Acknowledgments
We would like to thank Johanna Keller and Alisa Aschrich for testing and data collection. We used DeepL Translator and Writer to improve the quality of the article.
Conflict of interest
The authors declare that the research was conducted in the absence of any commercial or financial relationships that could be construed as a potential conflict of interest.
Publisher’s note
All claims expressed in this article are solely those of the authors and do not necessarily represent those of their affiliated organizations, or those of the publisher, the editors and the reviewers. Any product that may be evaluated in this article, or claim that may be made by its manufacturer, is not guaranteed or endorsed by the publisher.
Supplementary material
The Supplementary Material for this article can be found online at: https://www.frontiersin.org/articles/10.3389/fpsyt.2024.1347913/full#supplementary-material
Footnotes
- ^ Our own empirical studies refer only to German. However, we assume that other West Germanic languages work in a very similar way. Studies on other languages are cited for methodological reasons.
- ^ Fisseni (30) provides a systematic overview of the term focus; for a model of pragmatic focus interpretation see also Wollermann et al. (31).
- ^ It should be noted that we are referring to the current version of the VocalTractlab website (33).
- ^ A description of the false-belief task can be found in Wimmer and Perner (68). A brief overview of the different levels of ToM, i.e. first-order ToM (e.g., “X thinks or feels…”), second-order ToM (e.g., “X thinks that Y feels…”), and third-order ToM (e.g., “X believes that Y assumes that Z intends …”) can be found in Gabriel et al. [ (69): 534-35].The ToM of a subject S is generally understood as S's beliefs about mental states, such as beliefs, intentions, or emotions, of another subject O. If S's beliefs concern O's beliefs about mental states of subjects O_2 other than O, we speak of second-order ToM. O_2's mental states can also be beliefs about mental states of other persons. In this case we speak of third-order beliefs.
- ^ According to Begeer et al. (75) counterfactual reasoning describes a phenomenon in which people imagine alternatives to one or more features of a perceived event [see also (76)]. It can be characterized by switching back and forth between a real situation and an imagined situation, i.e. a so-called counterfactual situation.
- ^ For a more detailed discussion see Gyarmathy and Horváth [ (90): 27].
- ^ For a detailed discussion of the role of uncertainty in human-machine-interaction, see Wollermann [ (51): 91].
- ^ Unit selection is a method for acoustic speech synthesis based on large corpora of naturally spoken utterances. Units are selected with respect to a target utterance by means of concatenation. The generated speech is characterized by high comprehensibility [cf. (101): 279].
- ^ It should be noted that in our current study we also measure comprehensibility in order to better understand the processing of synthetic utterances.
- ^ It should be noted that the articulatory speech synthesizer is regularly updated. In the following we refer to the Vocaltract Lab website (33), but in our previous studies we have used older versions of the system.
- ^ A strong pause of 4 s was used because we could not find exact values for pauses in the literature for modeling uncertainty in question-answer situations in human-machine communication [cf. (109): 40]. It was important for us to use very clear characteristics of uncertainty to test whether there are effects. We have already successfully used this value of 4 s in our pilot study (109).
- ^ An overview of neural text-to-speech synthesis is given by Tan (116).
References
1. American Psychiatric Association. Diagnostic and statistical manual of mental disorders. In: Text Revision: DSM-5-TR, Fifth Edition. American Psychiatric Association Publishing, Washington, DC (2022).
2. World Health Organization. International classification of diseases for mortality and morbidity statistics (11th Revision) (2018). Available online at: https://icd.who.int/browse11/l-m/en#/http://id.who.int/icd/entity/437815624 (Accessed August 28, 2024).
3. NICE. Autism diagnosis in children and young people. Recognition, referral and diagnosis of children and young people on the autism spectrum. In: Clinical Guideline no. 128. National Institute for Health and Clinical Excellence, London (2011).
4. Lord C, Elsabbagh M, Baird G, Veenstra-Vanderweele J. Autism spectrum disorder. Lancet. (2018) 392:508–20. doi: 10.1016/S0140-6736(18)31129-2
5. Riedel A. Hochfunktionaler Autismus im Erwachsenenalter – klinische, neuropsychologische und morphometrische Befunde [habilitation thesis]. Freiburg: University of Freiburg (2015).
6. Vogeley K. Anders sein: Autismus-Spektrum-Störungen im Erwachsenenalter – ein Ratgeber. 2nd ed. Weinheim: Beltz (2016).
8. Riedel A. Sprachpragmatik bei Autismus-Spektrum-Störungen, in: 8. Newsletter, Wissenschaftliche Gesellschaft Autismus-Spektrum-Störung (2015). Available online at: https://silo.tips/download/newsletter-sprachpragmatik-bei-autismus-spektrum-strungen-mai-nr-8-dr-med-dr-phi (Accessed August 28, 2024).
9. Riedel A, Suh H, Haser V, Hermann I, Ebert D, Riemann D, et al. Freiburg Questionnaire of linguistic pragmatics (FQLP): psychometric properties on a psychiatric sample. BMC Psychiatry. (2014) 14:374. doi: 10.1186/s12888-014-0374-9
10. Geurts B, Kissine M, van Tiel B. “Pragmatic reasoning in autism”. In: Morsanyi K, Byrne RMJ, editors. Thinking, reasoning and decision making in autism. Routledge, London (2019). p. 113–34.
11. Andrés-Roqueta C, Katsos NA. A distinction between linguistic- and social-pragmatics helps the precise characterization of pragmatic challenges in children with Autism Spectrum Disorders and Developmental Language Disorder. J Speech Lang Hear Res. (2020) 63:1494–508. doi: 10.1044/2020_JSLHR-19-00263
12. Durrleman S, Hippolyte L, Zufferey S, Iglesias K, Hadjikhani N. Complex syntax in autism spectrum disorders: a study of relative clauses. Int J Lang Commun Disord. (2015) 50:260–7. doi: 10.1111/1460-6984.12130
13. Clark JE, Yallop C, Fletcher J. An Introduction to Phonetics and Phonology. 3rd ed. Oxford: Blackwell Publishing (2007).
14. Martzoukou M, Papadopoulou D, Kosmidis MH. The comprehension of syntactic and affective prosody by adults with autism spectrum disorder without accompanying cognitive deficits. J Psycholinguist Res. (2017) 46:1573–95. doi: 10.1007/s10936-017-9500-4
15. Martzoukou M, Papadopoulos D, Kosmidis MH. Syntactic and affective prosody recognition: Schizophrenia vs. Autism spectrum disorders. PloS One. (2023) 18:e0292325. doi: 10.1371/journal.pone.0292325
16. Terzi A, Marinis T, Francis K. The interface of syntax with pragmatics and prosody in children with autism spectrum disorders. J Autism Dev Disord. (2016) 46:2692–706. doi: 10.1007/s10803-016-2811-8
17. McCann J, Peppé S. Prosody in autism spectrum disorders; a critical review. Int J Lang Commun Disord. (2003) 38:325–50. doi: 10.1080/1368282031000154204
18. Gussenhoven C, Chen A eds. The Oxford handbook of language prosody. In: Oxford Handbooks in Linguistics. Oxford University Press, Oxford.
19. Tonhauser J. "Prosody and meaning", in: The Oxford Handbook of Experimental Semantics and Pragmatics (2019). online edn: Oxford Academic. doi: 10.1093/oxfordhb/9780198791768.013.30 (Accessed August 28, 2024).
20. Larrouy-Maestri P, Opeppel D, Pell MD. The sound of emotional prosody: nearly 3 years of research and future directions. Perspect psychol Sci. (2024) 0. doi: 10.1177/17456916231217722
21. Grice M, Wehrle S, Krüger M, Spaniol M, Cangemi F, Vogeley K. Linguistic prosody in autism spectrum disorder - An overview. Lang Linguistics Compass. (2023) 17(5):e12498. doi: 10.1111/lnc3.12498
22. Shriberg LD, Paul R, McSweeney JL, Klin AM, Cohen DJ, Volkmar FR. Speech and prosody characteristics of adolescents and adults with high functioning autism and Asperger syndrome. J Speech Lang Hear Res. (2001) 44:1097–115. doi: 10.1044/1092-4388(2001/087
23. Paul R, Augustyn A, Klin A, Volkmar FR. Perception and production of prosody by speakers with autism spectrum disorders. J Autism Dev Disord. (2005) 35:205–20. doi: 10.1007/s10803-004-1999-1
24. Kiss G, van Santen JPH, Prud'hommeaux E, Black LM. (2012). Quantitative analysis of pitch in speech of children with neurodevelopmental disorders, in: Proceedings of Interspeech, Portland, OR, USA, 2012 Sep 9-13. (Speech Communication Association), pp. 1343–46.
25. Nadig A, Shaw H. Acoustic and perceptual measurement of expressive prosody in high-functioning autism: increased pitch range and what it means to listeners. J Autism Dev Disord. (2012) 42:499–511. doi: 10.1007/s10803-011-1264-3
26. Wehrle S, Cangemi F, Hanekamp H, Vogeley K, Grice M. (2020). Assessing the intonation style of speakers with autism spectrum disorder, in: Proceedings of the 10th International Conference on Speech Prosody, Tokyo, Japan. Nobuaki Minematsu, Mariko Kondo, Takayuki Arai & Ryoko Hayashi. pp. 809–13.
27. Diehl JJ, Paul R. Acoustic differences in the imitation of prosodic patterns in children with autism spectrum disorders. Res Autism Spectr Disord. (2012) 6:123–34. doi: 10.1016/j.rasd.2011.03.012
28. Grice M, Krüger M, Vogeley K. Adults with Asperger syndrome are less sensitive to intonation than control persons when listening to speech. Cult Brain. (2016) 4:38–50. doi: 10.1007/s40167-016-0035-6
29. Globerson E, Amir N, Kishon-Rabin L, Golan O. Prosody recognition in adults with high-functioning autism spectrum disorders: from psychoacoustics to cognition. Autism Res. (2015) 8:153–63. doi: 10.1002/aur.1432
30. Fisseni B. Focus: interpretation? In: Empirical Investigations on Focus Interpretation [dissertation]. Universitätsverlag Rhein-Ruhr, Duisburg (2011).
31. Wollermann C, SChade U, Fisseni B, Schröder B. (2010). Accentuation, Uncertainty and Exhaustivity: towards a model of pragmatic focus interpretation, in: Proceedings of the 5th International Conference on Speech prosody, Chicago, IL, 2010 May 11-14, Vol. 100063, pp. 1–4.
32. Henrichsen PJ, Allwood J. (2013). Predicting the attitude flow in dialogue based on multi-modal speech cues, in: Proceedings of the Fourth Nordic Symposium on Multimodal Communication, NEALT Proceedings Series No. 21, Gothenburg, Sweden. Gothenburg, 2013 Nov 15-16, pp. 47–53.
33. Vocal Tract Lab. VocalTractLab (2022). Available online at: https://www.vocaltractlab.de (Accessed August 28, 2024).
34. Doi H, Fujisawa TX, Kanai C, Ohta H, Yokoi H, Iwana*-mi A, et al. Recognition of facial expressions and prosodic cues with graded emotional intensities in adults with Asperger syndrome. J Autism Dev Disord. (2013) 43:2099–113. doi: 10.1007/s10803-013-1760-8
35. Hsu C, Xu Y, S. (2014). Can adolescents with autism perceive emotional prosody? in: Proceedings of Interspeech 2014, Singapore: Speech Communication Association., pp. 1924–28.
36. Birkholz P, Kröger BJ, Neuschaefer-Rube C. (2011). Synthesis of breathy, normal, and pressed phonation using a two-mass model with a triangular glottis, in: Proceedings of Interspeech 2011, Italy Florence: Speech Communication Association., pp. 2681–84.
37. Lozier L, Vanmeter J, Marsh A. Impairments in facial affect recognition associated with autism spectrum disorders: A meta-analysis. Dev Psychopathol. (2014) 26:933–45. doi: 10.1017/S0954579414000479
38. Uljarevic M, Hamilton A. Recognition of emotions in autism: A formal meta-analysis. J Autism Dev Disord. (2012) 43:1517–26. doi: 10.1007/s10803-012-1695-5
39. Scheerer NE, Shafai F, Stevenson RA, Iarocci G. Affective prosody perception and the relation to social competence in autistic and typically developing children. J Abnorm Child Psychol. (2020) 48:965–75. doi: 10.1007/s10802-020-00644-5
40. Lartseva A, Dijkstra T, Buitelaar J. Emotional language processing in Autism Spectrum Disorders: A systematic review. Front Hum Neurosci. (2014) 8:991. doi: 10.3389/fnhum.2014.00991
41. Lui M, Lau G, Han Y, Yuen K, Sommer W. Strong relationship between rapid auditory processing and affective prosody recognition among adults with high autistic traits. J Autism Dev Disord. (2023) 53:3180–93. doi: 10.1007/s10803-022-05600-4
42. Bellinghausen C, Schröder B, Rau R, Fangmeier T, Dahmen P, Riedel A. Tebartz van Elst L Systematische Analyse von Studien zur Emotionswahrnehmung bei ASS mit Fokus auf der Analyse des sprachlichen Materials. In: Abstract book of the Wissenschaftliche Tagung Autismus Spektrum. Freiburg University, Freiburg (2022).
43. Ekman P. Universals and cultural differences in facial expressions of emotion. In: Cole J, editor. Nebraska Symposium on Motivation 1971. University of Nebraska Press, Lincoln (1972). p. 207–82.
44. Zhang M, Xu S, Chen Y, Lin Y, Ding H, Zhang Y. Recognition of affective prosody in autism spectrum conditions: A systematic review and meta-analysis. Autism. (2022) 26:798–813. doi: 10.1177/1362361321995725
45. Premack D, Woodruff G. Does the chimpanzee have a theory of mind? Behav Brain Sci. (1978) 4:515–26. doi: 10.1017/S0140525X00076512
46. Lindeman L. “Propositional attitudes”, in: The Internet Encyclopedia of Philosophy (2023). Available online at: https://iep.utm.edu/ (Accessed August 28, 2024).
47. Cudney PB. A propositional attitude approach to emotions. [dissertation]. Washington, DC: Georgetown University (2018).
48. Giannakidou A, Mari A. "Chapter 7. Propositional attitudes of emotion: gradability and nonveridicality". In: Giannakidou A, Mari A, editors. Truth and Veridicality in Grammar and Thought: Mood, Modality, and Propositional Attitudes. University of Chicago Press, Chicago (2021). p. 273–308.
49. Hirschberg J. “Pragmatics and prosody”. In: Huang Y, editor. The Oxford Handbook of Pragmatics, Oxford Handbooks. Oxford Academic, online edn (2017). p. 532–49. Available at: https://academic.oup.com/edited-volume/28055.
50. Oh I. Modeling Believable Human-Computer Interaction with an Embodied Conversational Agent: Face-to-Face Communication of Uncertainty. USA: Rutgers University (2006).
51. Wollermann C. Prosodie, nonverbale Signale, Unsicherheit und Kontext – Studien zur pragmatischen Fokusinterpretation. [dissertation]. Essen: University of Duisburg-Essen (2012).
52. Rozin P, Cohen AB. High frequency of facial expressions corresponding to confusion, concentration, and worry in an analysis of naturally occurring facial expressions of Americans. Emotion. (2003) 3:68–75. doi: 10.1037/1528-3542.3.1.68
53. Kuhlthau CC. Seeking Meaning: A Process Approach to Library and Information Services. Norwood: Ablex Publishing (1993).
54. van der Bles M, van der Linden S, Freeman ALJ, Mitchell J, Galvao AB, Zaval L, et al. Communicating uncertainty about facts, numbers and science. R Soc Open Sci. (2019) 6:181870. doi: 10.1098/rsos.181870
55. Carruthers P. Are epistemic emotions metacognitive? Philos Psychol. (2017) 30:58–78. doi: 10.1080/09515089.2016.1262536
56. Wollermann C, Schröder B, SChade U. Audiovisual prosody of uncertainty: an overview. J Theory Res Educ. (2014) 9:137–57. doi: 10.6092/issn.1970-2221/4295
57. Smith VL, Clark HH. On the course of answering questions. J Mem Lang. (1993) 32:25–38. doi: 10.1006/jmla.1993.1002
58. Hart JT. Memory and the feeling-of-knowing experience. J Educ Psychol. (1967) 56:208–16. doi: 10.1037/h0022263
59. Brennan SE, Williams M. The feeling of another’s knowing: Prosody and filled pauses as cues to listeners about the metacognitive states of speakers. J Mem Lang. (1995) 34:383–98. doi: 10.1006/jmla.1995.1017
60. Swerts M, Krahmer E. Audiovisual prosody and feeling of knowing. J Mem Lang. (2005) 53:81–94. doi: 10.1016/j.jml.2005.02.003
61. Krahmer E, Swerts M. How children and adults produce and perceive uncertainty in audiovisual speech. Lang Speech. (2005) 48:29–53. doi: 10.1177/00238309050480010201
62. Heyes CM, Frith CD. The cultural evolution of mind reading. Science. (2014) 344:1243091. doi: 10.1126/science.1243091
63. Astington JW, Dack LA. Theory of mind. In: Benson JB, editor. Encyclopedia of Infant and Early Childhood Development. Amsterdam: Elsevier (2008). p. 365–79. Available at: https://www.sciencedirect.com/referencework/9780128165119/encyclopedia-of-infant-and-early-childhood-development#book-info.
64. Leslie AM. Theory of mind. In: Smelser NJ, Baltes PB, editors. International Encyclopedia of the Social & Behavioral Sciences. Amsterdam: Elsevier (2001). p. 15652–6. Available at: https://www.sciencedirect.com/referencework/9780080430768/international-encyclopedia-of-the-social-and-behavioral-sciences#book-info.
65. Kamp-Becker I, Bölte S. Autismus. München: Ernst Reinhardt Verlag 3. vollst. überarb. Aufl. (2021). doi: 10.1026/1616-3443/a000477
66. Baron-Cohen S, Leslie AM, Frith U. Does the autistic child have a ‘theory of mind’? Cognition. (1985) 21:37–46. doi: 10.1016/0010-0277(85)90022-8
67. Happé FGE. The role of age and verbal ability in the theory of mind task performance of subjects with autism (1995) child development. Child Dev. (1995) 66(3):843–55. doi: 10.2307/1131954
68. Wimmer H, Perner J. Beliefs about beliefs: Representation and constraining function of wrong beliefs in young children’s understanding of deception. Cognition. (1983) 13:103–28. doi: 10.1016/0010-0277(83)90004-5
69. Gabriel TE, Oberger R, Schmoeger M, Deckert M, Vockh S. Cognitive and affective Theory of Mind in adolescence: developmental aspects and associated neuropsychological variables. psychol Res. (2021) 85:533–53. doi: 10.1007/s00426-019-01263-6
70. Chevallier C. "Theory of mind". In: Volkmar FR, editor. Encyclopedia of autism spectrum disorders. Springer International Publishing, Cham (2021).
71. Tager-Flusberg H, Sullivan K. A second look at second-order belief attribution in autism. J Autism Dev Disord. (1994) 24:577–86. doi: 10.1007/BF02172139
72. Iao LS, Leekam SR. Nonspecificity and theory of mind: new evidence from a nonverbal false-sign task and children with autism spectrum disorders. J Exp Child Psychol. (2014) 122:1–20. doi: 10.1016/j.jecp.2013.11.017
73. Shamay-Tsoory SG, Aharon-Peretz J. Dissociable prefrontal networks for cognitive and affective theory of mind: A lesion study. Neuropsychologia. (2007) 45:3054–67. doi: 10.1016/j.cortex.2009.04.008
74. Raimo S, Cropano M, Roldán-Tapia MD, Ammendola L, Malangone D, Santangelo G. Cognitive and affective theory of mind across adulthood. Brain Sci. (2022) 12:899. doi: 10.3390/brainsci12070899
75. Begeer S, De Rosnay M, Lunenburg P, Stegge H, Terwogt MM. Understanding of emotions based on counterfactual reasoning in children with autism spectrum disorders. Autism. (2014) 18:301–10. doi: 10.1177/1362361312468798
76. Epstude K, Roese NJ. The functional theory of counterfactual thinking. Pers Soc Psychol Rev. (2008) 12:168–92. doi: 10.1177/1088868308316091
77. Scheeren AM, de Rosnay M, Koot HM, Begeer S. Rethinking theory of mind in high-functioning autism spectrum disorder. J Child Psychol Psychiatry Allied Discip. (2013) 54:628–35. doi: 10.1111/jcpp.12007
78. Kimhi Y. Theory of mind abilities and deficits in autism spectrum disorders. Top Lang Disord. (2014) 34:329–43. doi: 10.1097/TLD.0000000000000033
79. Meylan A. Epistemic emotions: a natural kind? Philos Inquiries. (2014) 2:173–90. doi: 10.4454/philinq.v2i1.83
80. Silva L. Epistemic emotions justified. Philosophies. (2022) 7:104. doi: 10.3390/philosophies7050104
81. Morriss J, Tupitsa E, Dodd HF, Hirsch CR. Uncertainty makes me emotional: uncertainty as an elicitor and modulator of emotional states. Front Psychol. (2022) 13:777025. doi: 10.3389/fpsyg.2022.777025
82. Baron-Cohen S, Wheelwright S, Hill J, Raste Y, Plumb I. The "Reading the mind in the eyes" Test revised version: A study with normal adults, and adults with asperger syndrome or high-functioning autism. J Child Psychol Psychiatry. (2001) 42:241–51. doi: 10.1017/S0021963001006643
83. Peñuelas-Calvo I, Sareen A, Sevilla-Llewellyn-Jones J, Fernández-Berrocal P. The “Reading the mind in the eyes” Test in autism-spectrum disorders comparison with healthy controls: A systematic review and meta-analysis. J Autism Dev Disord. (2018) 49:1048–61. doi: 10.1007/s10803-018-3814-4
84. Livingston LA, Colvert E, the Social Relationships Study Team, Bolton P, Happe F. Good social skills despite poor theory of mind: exploring compensation in autism spectrum disorder. J Child Psychol Psychiatry. (2019) 60:102–10. doi: 10.1111/jcpp.12886
85. Corbett BA, Schwartzman JM, Libsack EJ, Muscatello RA, Lerner MD, Simmons GL, et al. Camouflaging in autism: examining sex-based and compensatory models in social cognition and communication. Autism Res. (2021) 14:127–42. doi: 10.1002/aur.2440
86. Rose RL. (2017). Silent and filled pauses and speech planning in first and second language production, in: Proceedings of the Workshop on Disfluency in Spontaneous Speech, Royal Institute of Technology: Stockholm Sweden, 2017 18–19 August.
87. Fox Tree JE. Disfluencies in spoken language. In: Nadel L, editor. Encyclopedia of Cognitive Science, vol. 1 . Nature Publishing Group, London (2003). p. 983–6.
88. Belz M. Defining filler particles: A phonetic account of the terminology, form, and grammatical classification of “Filled pauses. Languages. (2023) 8:57. doi: 10.3390/languages8010057
89. Beňuš Š. Variability and stability in collaborative dialogues: turn-taking and filled pauses. In: Proceedings of Interspeech 2009. Brighton, UK (2009). p. 796–9. doi: 10.21437/Interspeech.2009-180
90. Gyarmathy D, Horváth V. (2019). Pausing strategies with regard to speech style, in: Proceedings of the Workshop on Disfluency in Spontaneous Speech 2019, Budapest: Eötvös Loránd University, pp. 27–30. doi: 10.21862/diss-09-008-gyar-horv
91. Belz M. Die Phonetik von äh und ähm. In: Akustische Variation von Füllpartikeln im Deutschen. J.B. Metzler, Berlin (2021). doi: 10.1007/978-3-662-62812-6
92. Wehrle S, Grice M, Vogeley K. Filled Pauses Produced by Autistic Adults Differ in Prosodic Realization, but not Rate or Lexical Type. J Autism Dev Disord. (2023) 54:2513–25. doi: 10.1007/s10803-023-06000-y
93. Betz S, Zarrieß S, Székely É, Wagner P. The greennn tree — Lengthening position influences uncertainty perception. Proc Interspeech. (2019) 2019):3990–4. doi: 10.21437/Interspeech.2019-2572
94. Lickley RJ. Fluency and disfluency. In: Redford MA, editor. The Handbook of Speech Production. Wiley Online Library, Chichester (2015). p. 445–74.
95. Tits N. Controlling the emotional expressiveness of synthetic speech: a deep learning approach. 4OR. (2022) 20:165–66. doi: 10.1007/s10288-021-00473-2
96. Murray IR, Arnott JL. (1996). Synthesizing emotions in speech: is it time to get excited?, in: Proceedings of the International Conference on Spoken Language Processing, Philadelphia, PA. New Castle, Delaware: University of Delaware, 1996 Oct 3-6, pp. 1816–9. doi: 10.21437/ICSLP.1996-461
97. Marsi E, Rooden Fv. (2007). Expressing uncertainty with a talking head in a multimodal question-answering system, in: Proceedings of the Workshop on Multimodal Output Generation, University of Aberdeen, United Kingdom. Enschede, Netherlands: Centre for Telematics and Information Technology, 2007 Jan 25-26, pp. 105–16.
98. Śzekely E, Mendelson J, Gustafson J. (2017). Synthesizing uncertainty: The interplay of vocal effort and hesitation disfluencies, in: Proceedings of Interspeech, Stockholm, Sweden, 2017 August 20-24, pp. 804–8. doi: 10.21437/Interspeech.2017-1507
99. Adell J, Bonafonte A, Escudero-Mancebo D. (2010). Modelling. Filled pauses prosody to synthesize disfluent speech, in: Proceedings of Speech Prosody, Chicago, IL, 2010, May 11-14, Vol. . 100624, pp. 1–4. doi: 10.21437/SpeechProsody.2010-75
100. Andersson S, Georgila K, Traum D, Aylett M, Clark RAJ. (2010). Prediction and realization of conversational characteristics by utilizing spontaneous speech for unit selection, in: Proceedings of Speech Prosody, Chicago, IL, 2010; May 11-14, Vol. . 100116, pp. 1–4. doi: 10.21437/SpeechProsody.2010-89
101. Campbell N, Black AW. Prosody and the selection of source units for concatenative synthesis. In: van Santen JPH, Sproat RW, Olive JP, Hirschberg J, editors. Progress in speech synthesis. Springer Verlag, New York (1996). p. 279–92. doi: 10.1007/978-1-4612-1894-4_22
102. Hönemann A, Wagner P. (2016). Synthesizing attitudes in german, in: Proceedings of the 16th Speech Science and Technology Conference 2016, Parramatta; Australia. Australia: Australasian Speech Science and Technology Association, 2016 December 6-9, pp. 209–13.
103. Betz S. Hesitations in Spoken Dialogue Systems. [dissertation]. Bielefeld: Universität Bielefeld (2020).
104. Dall R, Yamagishi J, King S. (2014). Rating naturalness in speech synthesis: the effect of style and expectation, in: Proceedings of Speech Prosody 2014, Dublin Ireland, May 20-23, pp. 1012–16. doi: 10.21437/SpeechProsody.2014-192
105. Wagner P, Beskow J, Betz S, Edlund J, Gustafson J, Eje Henter G, et al. (2019). Speech synthesis evaluation — State-of-the-art assessment and suggestion for a novel research program, in: Proc. 10th ISCA Workshop on Speech Synthesis (SSW 10), Vienna, Austria, September 20-22. pp. 105–10. doi: 10.21437/SSW.2019-19
106. Lasarcyk E, Wollermann C, Schröder B, SChade U. (2013). On the modelling of prosodic cues in synthetic speech: what are the effects on perceived uncertainty and naturalness?, in: Proceedings of the 10th International Workshop on Natural Language Processing and Cognitive Science, Marseille, France. International Speech Communication Association, 2013 October 15-16, pp. 117–28.
107. Wollermann C, Lasarcyk E, SChade U, Schröder B. (2013). Disfluencies and uncertainty perception - evidence from a human-machine scenario, in: Proceedings of Diss 2013 the 6th Workshop on Disfluency in Spontaneous Speech, Stockholm, Sweden. Sweden: Department of Speech, Music and Hearing, KTH, 2013 August 21-23, pp. 73–6.
108. Wollermann C, Lasarcyk E. (2007). Modeling and perceiving of (Un)Certainty in articulatory speech synthesis, in: Proceedings of the 6th ISCA Workshop on Speech Synthesis, Bonn, Germany. Pittsburgh: International Speech Communication Association, 2007 August 22-24. pp. 40–5.
109. Bellinghausen C, Fangmeier T, Schröder B, Keller J, Drechsel S, Birkholz P, et al. (2019). On the role of disfluent speech for uncertainty in articulatory speech synthesis, in: Proceedings of DiSS 2019: The 9th Workshop on Disfluency in Spontaneous Speech, Budapest, Hungary. Hungary: Eötvös Loránd University (ELTE, 2019 September 12-13, pp. 39–42. doi: 10.21862/diss-09-011-bell-etal
110. Birkholz P. Modeling consonant-vowel coarticulation for articulatory speech synthesis. PloS One. (2013) 8:e60603. doi: 10.1371/journal.pone.0060603
111. Praat. Praat doing phonetics by computer (2023). Available online at: https://www.fon.hum.uva.nl/praat/ (Accessed August 28, 2024).
112. Field AP, Wilcox RR. Robust statistical methods: A primer for clinical psychology and experimental psychopathology researchers. Behav Res Ther. (2017) 98:19–38. doi: 10.1016/j.brat.2017.05.013
113. Mair P, Wilcox R. Robust statistical methods in R using the WRS2 package. Behav Res Methods. (2020) 52:464–88. doi: 10.3758/s13428-019-01246-w
114. Wilcox RR. Introduction to robust Estimation and Hypothesis Testing. 5th ed. Elsevier: Academic Press (2022).
115. Wilcox RR, Rousselet GA. An updated guide to robust statistical methods in neuroscience. Curr Protoc. (2023) 3:1–31. doi: 10.1002/cpz1.719
116. Tan X. Neural text-to-speech synthesis. In: Artificial Intelligence: Foundations, Theory, and Algorithms. Springer Nature, Singapore (2023).
117. Ding H, Zhang Y. Speech prosody in mental disorders. Annu Rev Linguistics. (2023) 9:335–55. doi: 10.1146/annurev-linguistics-030421-065139
Keywords: speech perception, autism spectrum disorder, prosody, uncertainty, emotion perception, theory of mind
Citation: Bellinghausen C, Schröder B, Rauh R, Riedel A, Dahmen P, Birkholz P, Tebartz van Elst L and Fangmeier T (2024) Processing of prosodic cues of uncertainty in autistic and non-autistic adults: a study based on articulatory speech synthesis. Front. Psychiatry 15:1347913. doi: 10.3389/fpsyt.2024.1347913
Received: 01 December 2023; Accepted: 09 September 2024;
Published: 14 October 2024.
Edited by:
Antonio M. Persico, University of Modena and Reggio Emilia, ItalyReviewed by:
Elisabetta Genovese, University of Modena and Reggio Emilia, ItalyLoredana Schettino, Free University of Bozen-Bolzano, Italy
Simon Betz, Bielefeld University, Germany
Copyright © 2024 Bellinghausen, Schröder, Rauh, Riedel, Dahmen, Birkholz, Tebartz van Elst and Fangmeier. This is an open-access article distributed under the terms of the Creative Commons Attribution License (CC BY). The use, distribution or reproduction in other forums is permitted, provided the original author(s) and the copyright owner(s) are credited and that the original publication in this journal is cited, in accordance with accepted academic practice. No use, distribution or reproduction is permitted which does not comply with these terms.
*Correspondence: Charlotte Bellinghausen, Y2hhcmxvdHRlLmJlbGxpbmdoYXVzZW5AdW5pLWR1ZS5kZQ==
†ORCID: Charlotte Bellinghausen, https://orcid.org/0000-0001-7831-8754
Bernhard Schröder, https://orcid.org//0000-0002-7847-1393
Reinhold Rauh, https://orcid.org/0000-0003-3053-1163
Andreas Riedel, https://orcid.org/0009-0001-4977-2752
Peter Birkholz, https://orcid.org/0000-0003-0167-8123
Ludger Tebart van Elst, https://orcid.org/0000-0002-2274-5447
Thomas Fangmeier, https://orcid.org/0009-0007-7966-9215