- 1Swansea University Medical School, Swansea, United Kingdom
- 2King’s College London, Institute of Psychiatry, Psychology and Neuroscience, London, United Kingdom
- 3South London and Maudsley National Health Service (NHS) Foundation Trust, London, United Kingdom
Introduction
It is fair to say that the application of machine learning (ML) in healthcare has not been smooth. The field of ML has let down the medical community and the wider public in many respects: from research that is clinically irrelevant (1) or applying flawed methodologies (2), to non-transparent sharing of data with industry (3, 4). Success stories do exist across a range of physical health specialties, but they currently remain a minority (5, 6).
In hindsight, the pitfalls for ML in medical research are hardly surprising. Epidemiology (medicine’s own approach) is underpinned by statistics and hypothesis testing, designed to maintain ethical etiquette, ensure robust, unbiased results, and produce strong evidence and knowledge in measured phenomena – at least in principle (7). It therefore aims to understand the ‘true’ mechanisms connecting exposures and outcomes (features and targets in ML jargon) and naturally gravitates towards simpler, easier to interpret models. ML has its own established methodology (8), but one that is fundamentally different, geared towards solving problems and developing applications (9). It therefore pursues maximum accuracy at predicting the outcome and naturally prefers complex, more powerful models. The different use of logistic regression by both fields illustrates this. While epidemiology takes special care with correlated independent variables and directs its attention to the estimated coefficients, ML mostly disregards these and focusses on predictive power. Overall, while both epidemiology and ML rely on data to obtain their results, their core principles are at odds. Nevertheless, appropriately introducing ML elements into epidemiological research is possible and guidelines have been published (10).
Mental health has been a target for ML, with the number of ML mental health publications increasing dramatically since, 2017 (Figure 1), and the research community is rightly expectant of its impact. However, the challenges are amplified: (1) losing sight of mental health objectives, over-promising on data processing and problem-solving (9); (2) technical hurdles of multiple underlying biases and often heightened privacy requirements (11); and (3) difficulties building, validating and approving ML-enabled clinical devices for diseases with insufficiently clear underlying mechanisms (12). Overall, it is the responsibility of individual researchers and institutions alike to demonstrate the value of ML for mental health. Here, we reflect on these ideas and their corresponding steps within the workflow of ML mental health research (Figure 2), in the hope of bringing awareness to the field and to elicit further conversations.
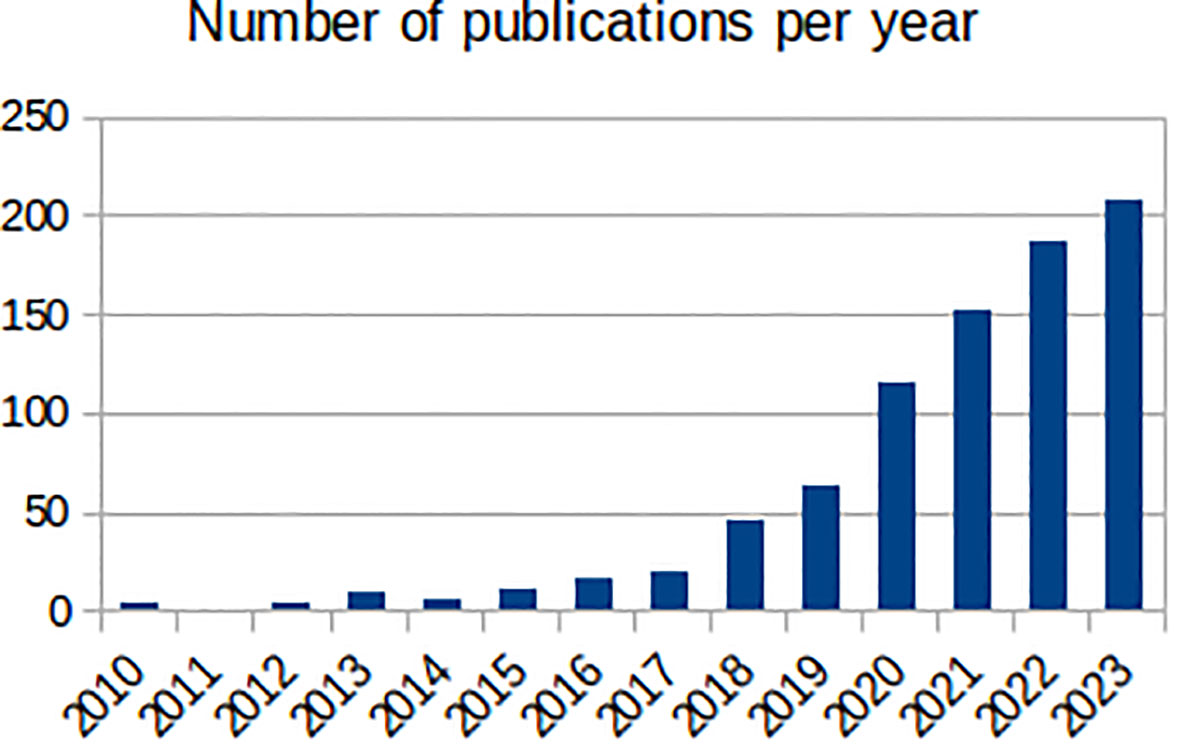
Figure 1 Number of annual publications found by PubMed (https://pubmed.ncbi.nlm.nih.gov/) between, 2010 and, 2023 with the terms “machine learning” and one of “mental health”, “mental illness”, “depression”, “anxiety”, “bipolar disorder, “schizophrenia”, “psychotic disorder”, “ADHD” or “autism” in the title.
The ideal target for ML
Factors affecting an individual’s mental health extend far beyond the clinical setting and are numerous, with complex interactions. Social, demographic, and economic factors and people’s psychological make-up carry as much or more weight in estimating risk of mental health outcomes as medical symptoms, biological factors, and previous health (e.g., the effect of loneliness in suicidal thoughts and self-harm) (13). The complexity of these relationships is typified in suicide research, where a meta-analysis of risk factors identified little progress in prevention over a span of 50 years (14). Consequently, the heavy reliance of classical statistics on prior expert knowledge and model assumptions is another important limiting factor in mental health research. Progress in data provision and data linkage has addressed some of the challenges of mental health research (i.e., providing better population coverage and a wider range of risk factors) but has brought additional challenges such as larger volumes of data, lower data quality, increased missing data and unstandardised phenotypes (15, 16). Furthermore, the field of mental health is evolving, and expert consensus is lacking on the taxonomy of psychiatric diagnosis (17) or on preferred ‘transdiagnostic’ clinical phenotypes (18).
The complexity and wide reach of its disease models are why mental health might particularly benefit from ML. ML is better equipped than classical statistics to deal with large numbers of factors, complex (i.e., non-linear) interactions, and noise (i.e., low quality or missing data, unstandardised phenotypes) (19). A data-driven approach is of particular value (20), such as deep learning techniques (21), and ML could be pivotal in evidence provision for diagnostic taxonomies or clinical phenotypes. However, this requires demonstrable evidence on applied clinical validity.
Keeping sight of mental health aims and objectives
Single studies of ML predicting an outcome from a given dataset, and therefore only presenting performance results of these models, are of limited interest for mental health research (22). More valuable applications seek to improve our understanding of the disease (e.g., risk factors or time trends) and/or identify intervention opportunities. Therefore, researchers working on ML mental health should strive to: (1) extract new clinical insights from their models; (2) validate such insights with supplementary statistical analyses, and (3) contextualise their findings in the existing clinical literature. Completing all three objectives in full is not always possible, but researchers should make an honest effort on each of them and, when unsuccessful, acknowledge it as a limitation of their research.
This is not to say that research aiming at developing new ML algorithms and methodologies to process data with similar characteristics to those from mental health data (outlined below) are unimportant. Such research may naturally rely on mental health data, but the focus is on the fundamental characteristics of the data, not its mental health content – indeed, the research could have been completed using any other (non-mental health) data with the same fundamental characteristics. In this scenario, researchers should recognise that their work is about ML and not mental health, and this should be reflected in the focus of their papers and their targeted audience.
ML challenges when using mental health data
Data curation is a critical part in developing ML models for healthcare. Some of the steps involved in this process are identical to those seen in epidemiological research: determining the sample size through power calculations; assessing the quality of the variables; studying bias in the patterns of missing data and recording practices; and evaluating the representation of the study population by the study sample. Other data curation steps are more specific to ML: the need for larger volumes of data, especially for complex models (23); comprehensive evaluation of outcome variable quality (24); data partition strategies for model building and validation (in ML jargon training and testing; cross-validation, often done repeatedly to improve robustness and generalizability of the results) (25); and considering additional security measures to prevent data inference from the ML model itself (in ML jargon membership inference attacks) (26).
Many of the data curation steps described above are potentially more complex in mental health research. Recall and reporting biases are common in self-reported mental health data, and can lead to under- or overestimation of underlying associations (27). When these biases affect the outcome variable, the entire validity of the model can be compromised. With ML being a “data driven” approach, these biases can be especially damaging in ML applications. They should therefore be reduced as much as possible to improve the model’s performance and clinical validity, with the remaining bias carefully considered when assessing the results (24). Additionally, achieving participation and retention of participants in mental health research may also be challenging (28). The use of routinely collected electronic health records alleviates these issues to some extent; however, many important constructs of interest are subjective and can only be self-reported. Furthermore, minority groups (often the most affected by mental health inequalities) and those with more severe syndromes are frequently excluded and underserved (29). In addition, outcomes such as self-harm are known to be under-recorded in electronic health records (30). More generally, mental health data are viewed as relatively sensitive, partly due to the personal nature of the questions asked in a typical clinical assessment but also due to the stigma surrounding mental health conditions and consequent heightened privacy concerns – the public is slightly less inclined to share their mental health data for research compared to their physical health (31). This results in additional ethical and legal hurdles for mental health research (32), and more so for the application of ML due to its need of large volumes of data and the risk of models inadvertently carrying these data (26).
There is no easy fix for these problems, and, compared to most other medical specialties, mental health researchers, especially those applying ML, often need to: (1) focus more resources on their data curation strategy; (2) address bias in their data with statistical tools such as inverse probability weighing, which can be applied to both epidemiology (33) and ML (34) methods; and (3) have a stronger patient and public involvement and engagement plan (35).
ML enabled clinical mental health devices with unknowns
The path from the lab to the clinical setting for medical innovations is not simple. This is especially true for ML-enabled devices, and still under discussion (5, 36) with regulatory frameworks evolving (37). In fact, only a small proportion of the published clinical ML research has been focused on deployment (5); as of October 19, 2023, the United States Food and Drug Agency reports approving less than 700 ML-enabled medical devices (based on their summary descriptions) (38), although this is likely an underestimation due to bias in explicit reporting of ML methods (39).
The situation is exacerbated for clinical mental health devices, with less real-world deployments (40) and fewer FDA approved devices (6). This may reflect the currently restricted scope of such devices as a consequence of our limited knowledge of the mechanisms underlying mental disorders, at least relative to other specialties (12). Without such knowledge, ML models are often fed a wide range of risk factors suspected to be related to the outcome (or in the hope that they will be of value during prediction). The assumption here is that if a model accurately predicts the outcome, it must be a true representation of the real-world phenomena described by the data. However, the data may contain variables that are confounders or act as proxies to latent variables, thus rendering the assumption unfair. When the potential risk factors fed to the ML algorithm lack evidence supporting and explaining their relationship with the outcome (as it is often the case), the clinical validity of the resulting ML-enabled mental health device remains to be proven, regardless of its accuracy. However, with the clinical knowledge laid down, healthcare professionals and patients will be more likely to accept the black box quality of ML models (41), and ML will have a clearer path to developing mental health solutions.
Individual and collective responsibility
Researchers have a responsibility to demonstrate that, when correctly applied, ML can lead to improved knowledge and care of mental health disorders. To achieve this, ML practitioners must work in close collaboration with mental health epidemiologists and clinicians, and actively seek their input to protocol design and data interpretation. Crucially, they need to acknowledge that data fed into ML models represent personal experiences, to be aware of the particular sensitivities of mental health data, and to learn to handle these data responsibly above and beyond legislated privacy and security requirements. Conversely, mental health researchers seeking to engage with ML must avoid being blinded by the hype. Instead, they must continue to adhere to the main methodological principles of epidemiology and mental health research, and scrutinise any ML models generated (42). They should also be cautious of utilizing easy-to-use ML libraries and tools without the appropriate training, as these have led to the abuse and misuse of ML by non-experts (43).
Organisations and large projects could play a key role in ensuring that the fields of mental health and ML interact as described here. For example, DATAMIND (the MRC funded, UK Hub for Mental Health Data Science; www.datamind.org.uk) brings the issues outlined above to the attention of the field of mental health research at large, holding regular meetings and conferences with a wide range of stakeholders, and providing mental health data science workshops for early career researchers. DATAMIND is also developing a set of standardised mental health phenotypes to be used by the scientific community (44) and contributing to the cataloguing of available mental health data resources to improve discoverability and accessibility (45). Crucially, DATAMIND achieves this in close collaboration with academics, healthcare professionals, industry, and, most importantly, patients and people with lived experiences.
Concluding remarks
Overall, the opportunity of using ML in mental health is not cost-free. As described, it introduces complexity, especially in mental health research, and additional workflow steps. Therefore, its application in healthcare generally, and in mental health particularly, needs to be justified. Ideally, this should be done at the planning stage, evidencing why the use of ML is needed to solve an existing problem that is hindering research: for example, to reduce an original set of available measurements to a size that is more manageable for traditional statistical regression (46). Alternatively, the benefits of using ML over conventional statistical methods can be treated as a hypothesis to be tested as part of the research project: for example, by comparing how well ML and statistical models fit the used data.
Beyond the hype, ML can genuinely play a central role in the future of psychiatry and mental healthcare. However, this depends on researchers applying ML responsibly and avoiding the mistakes seen in its application to other medical specialties.
Author contributions
MDPB: Writing – review & editing, Writing – original draft, Conceptualization. RS: Writing – review & editing. AJ: Writing – review & editing.
Funding
The author(s) declare financial support was received for the research, authorship, and/or publication of this article. All authors were funded by the Medical Research Council and Health Data Research UK (Grant DATAMIND: Data Hub for Mental Health INformatics research Development, with Ref.: MR/W014386/1). RS is additionally part-funded by: i) the NIHR Maudsley Biomedical Research Centre at the South London and Maudsley NHS Foundation Trust and King’s College London; ii) the National Institute for Health Research (NIHR) Applied Research Collaboration South London (NIHR ARC South London) at King’s College Hospital NHS Foundation Trust; iii) the UK Prevention Research Partnership (Violence, Health and Society; MR-VO49879/1), an initiative funded by UK Research and Innovation Councils, the Department of Health and Social Care (England) and the UK devolved administrations, and leading health research charities.
Conflict of interest
AJ chairs the National Advisory Group on Suicide and Self-harm Prevention to Welsh Government. RS declares research funding/support from Janssen, GSK and Takeda in the last 3 years.
The remaining author declares that the research was conducted in the absence of any commercial or financial relationships that could be construed as a potential conflict of interest.
Publisher’s note
All claims expressed in this article are solely those of the authors and do not necessarily represent those of their affiliated organizations, or those of the publisher, the editors and the reviewers. Any product that may be evaluated in this article, or claim that may be made by its manufacturer, is not guaranteed or endorsed by the publisher.
References
1. Roberts M, Driggs D, Thorpe M, Gilbey J, Yeung M, Ursprung S, et al. Common pitfalls and recommendations for using machine learning to detect and prognosticate for COVID-19 using chest radiographs and CT scans. Nat Mach Intell. (2021) 3:199–217. doi: 10.1038/s42256-021-00307-0
2. Varoquaux G, Cheplygina V. Machine learning for medical imaging: methodological failures and recommendations for the future. NPJ digital Med. (2022) 5:48. doi: 10.1038/s41746-022-00592-y
3. NewScientist. Revealed: Google AI has access to huge haul of NHS patient data (2016). Available online at: https://www.newscientist.com/article/2086454-revealed-google-ai-has-access-to-huge-haul-of-nhs-patient-data/.
4. BBC News. Project Nightingale: Google accesses trove of US patient data (2019). Available online at: https://www.bbc.co.uk/news/technology-50388464.
5. Drysdale E, Dolatabadi E, Chivers C, Liu V, Saria S, Sendak M, et al. (2019). Implementing AI in healthcare, in: Vector-SickKids Health AI Deployment Symposium, Toronto. Canada: Vector Institute and the Hospital for Sick Children
6. Benjamens S, Dhunnoo P, Meskó B. The state of artificial intelligence-based FDA-approved medical devices and algorithms: an online database. NPJ digital Med. (2020) 3:118. doi: 10.1038/s41746-020-00324-0
7. Shy CM. The failure of academic epidemiology: witness for the prosecution. Am J Epidemiol. (1997) 145:479–84. doi: 10.1093/oxfordjournals.aje.a009133
8. Lones MA. How to avoid machine learning pitfalls: a guide for academic researchers - v3. arXiv preprint arXiv:2108.02497. (2023). doi: 10.48550/arXiv.2108.02497
9. Emanuel EJ, Wachter RM. Artificial intelligence in health care: will the value match the hype? Jama. (2019) 321:2281–2. doi: 10.1001/jama.2019.4914
10. Hamilton AJ, Strauss AT, Martinez DA, Hinson JS, Levin S, Lin G, et al. Machine learning and artificial intelligence: Applications in healthcare epidemiology. Antimicrobial Stewardship Healthcare Epidemiol. (2021) 1:e28. doi: 10.1017/ash.2021.192
11. Morgan K, Page N, Brown R, Long S, Hewitt G, Del Pozo-Banos M, et al. Sources of potential bias when combining routine data linkage and a national survey of secondary school-aged children: a record linkage study. BMC Med Res Method. (2020) 20:1–13. doi: 10.1186/s12874-020-01064-1
12. Lee EE, Torous J, De Choudhury M, Depp CA, Graham SA, Kim HC, et al. Artificial intelligence for mental health care: clinical applications, barriers, facilitators, and artificial wisdom. Biol Psychiatry: Cogn Neurosci Neuroimaging. (2021) 6:856–64. doi: 10.1016/j.bpsc.2021.02.001
13. John A, Lee SC, Solomon S, Crepaz-Keay D, McDaid S, Morton A, et al. Loneliness, coping, suicidal thoughts and self-harm during the COVID-19 pandemic: A repeat cross-sectional UK population survey. BMJ Open. (2021) 11:e048123. doi: 10.1136/bmjopen-2020-048123
14. Franklin JC, Ribeiro JD, Fox KR, Bentley KH, Kleiman EM, Huang X, et al. Risk factors for suicidal thoughts and behaviors: A meta-analysis of 50 years of research. psychol Bull. (2017) 143:187. doi: 10.1037/bul0000084
15. Lee CH, Yoon HJ. Medical big data: Promise and challenges. Kidney Res Clin Pract. (2017) 36:3. doi: 10.23876/j.krcp.2017.36.1.3
16. Herrett E, Thomas SL, Schoonen WM, Smeeth L, Hall AJ. Validation and validity of diagnoses in the General Practice Research Database: A systematic review. Br J Clin Pharmacol. (2010) 69:4–14. doi: 10.1111/j.1365-2125.2009.03537.x
17. Kim YK, Park SC. Classification of psychiatric disorders. In: Frontiers in Psychiatry: Artificial Intelligence, Precision Medicine, and Other Paradigm Shifts USA: Springer. (2019). p. 17–25. doi: 10.1007/978-981-32-9721-0
18. Dalgleish T, Black M, Johnston D, Bevan A. Transdiagnostic approaches to mental health problems: Current status and future directions. J consulting Clin Psychol. (2020) 88:179. doi: 10.1037/ccp0000482
19. Song X, Mitnitski A, Cox J, Rockwood K. Comparison of machine learning techniques with classical statistical models in predicting health outcomes. In: MEDINFO. Amsterdam: IOS Press (2004). p. 736–40.
20. Liang Y, Zheng X, Zeng DD. A survey on big data-driven digital phenotyping of mental health. Inf Fusion. (2019) 52:290–307. doi: 10.1016/j.inffus.2019.04.001
21. Wang X, Zhao Y, Pourpanah F. Recent advances in deep learning. Int J Mach Learn Cybernetics. (2020) 11:747–50. doi: 10.1007/s13042-020-01096-5
22. Tornero-Costa R, Martinez-Millana A, Azzopardi-Muscat N, Lazeri L, Traver V, Novillo-Ortiz D. Methodological and quality flaws in the use of artificial intelligence in mental health research: Systematic review. JMIR Ment Health. (2023) 10:e42045. doi: 10.2196/42045
23. Riley RD, Ensor J, Snell KI, Harrell FE, Martin GP, Reitsma JB, et al. Calculating the sample size required for developing a clinical prediction model. Bmj. (2020) 368. doi: 10.1136/bmj.m441
24. Chen PHC, Liu Y, Peng L. How to develop machine learning models for healthcare. Nat materials. (2019) 18:410–4. doi: 10.1038/s41563-019-0345-0
25. Tougui I, Jilbab A, El Mhamdi J. Impact of the choice of cross-validation techniques on the results of machine learning-based diagnostic applications. Healthcare Inf Res. (2021) 27:189–99. doi: 10.4258/hir.2021.27.3.189
26. Shokri R, Stronati M, Song C, Shmatikov V. (2017). Membership inference attacks against machine learning models, in: 2017 IEEE symposium on security and privacy (SP), USA: IEEE. pp. 3–18.
27. Rhodes AE, Fung K. Self-reported use of mental health services versus administrative records: care to recall? Int J Methods Psychiatr Res. (2004) 13:165–75. doi: 10.1002/mpr.172
28. Granero Pérez R, Ezpeleta L, Domenech JM. Features associated with the non-participation and drop out by socially-at-risk children and adolescents in mental-health epidemiological studies. Soc Psychiatry Psychiatr Epidemiol. (2007) 42:251–8. doi: 10.1007/s00127-006-0155-y
29. Rees S, Fry R, Davies J, John A, Condon L. Can routine data be used to estimate the mental health service use of children and young people living on Gypsy and Traveller sites in Wales? A feasibility study. PLoS One. (2023) 18:e0281504. doi: 10.1371/journal.pone.0281504
30. Arensman E, Corcoran P, McMahon E. The iceberg model of self-harm: new evidence and insights. Lancet Psychiatry. (2018) 5:100–1. doi: 10.1016/S2215-0366(17)30477-7
31. Jones LA, Nelder JR, Fryer JM, Alsop PH, Geary MR, Prince M, et al. Public opinion on sharing data from health services for clinical and research purposes without explicit consent: an anonymous online survey in the UK. BMJ Open. (2022) 12:e057579. doi: 10.1136/bmjopen-2021-057579
32. Ford T, Mansfield KL, Markham S, McManus S, John A, O'reilly D, et al. The challenges and opportunities of mental health data sharing in the UK. Lancet Digital Health. (2021) 3:e333–6. doi: 10.1016/S2589-7500(21)00078-9
33. John A, Lee SC, PuChades A, Del Pozo-Baños M, Morgan K, Page N, et al. Self-harm, in-person bullying and cyberbullying in secondary school-aged children: A data linkage study in Wales. J Adolescence. (2023) 95:97–114. doi: 10.1002/jad.12102
34. Lee BK, Lessler J, Stuart EA. Improving propensity score weighting using machine learning. Stat Med. (2010) 29:337–46. doi: 10.1002/sim.3782
35. Banerjee S, Alsop P, Jones L, Cardinal RN. Patient and public involvement to build trust in artificial intelligence: A framework, tools, and case studies. Patterns. (2022) 3(6). doi: 10.1016/j.patter.2022.100506
36. Rouger M. AI improves value in radiology, but needs more clinical evidence. Healthcare IT News (2020). Available at: https://www.healthcareitnews.com/news/emea/ai-improves-value-radiology-needs-more-clinical-evidence.
37. United Kingdom Medicines and Healthcare products Regulatory Agency. Software and AI as a Medical Device Change Programme – Roadmap (2023). Available online at: https://www.gov.uk/government/publications/software-and-ai-as-a-medical-device-change-programme/software-and-ai-as-a-medical-device-change-programme-roadmap.
38. United States Food and Drug Agency. Artificial Intelligence and Machine Learning (AI/ML)-Enabled Medical Devices (2024). Available online at: https://www.fda.gov/medical-devices/software-medical-device-samd/artificial-intelligence-and-machine-learning-aiml-enabled-medical-devices.
39. Muehlematter UJ, Bluethgen C, Vokinger KN. FDA-cleared artificial intelligence and machine learning-based medical devices and their 510 (k) predicate networks. Lancet Digital Health. (2023) 5:e618–26. doi: 10.1016/S2589-7500(23)00126-7
40. Koutsouleris N, Hauser TU, Skvortsova V, De Choudhury M. From promise to practice: Towards the realisation of AI-informed mental health care. Lancet Digital Health. (2022) 4:e829–40. doi: 10.1016/S2589-7500(22)00153-4
42. Al-Zaiti SS, Alghwiri AA, Hu X, Clermont G, Peace A, Macfarlane P, et al. A clinician’s guide to understanding and critically appraising machine learning studies: a checklist for Ruling Out Bias Using Standard Tools in Machine Learning (ROBUST-ML). Eur Heart Journal-Digital Health. (2022) 3:125–40. doi: 10.1093/ehjdh/ztac016
43. Riley P. Three pitfalls to avoid in machine learning. Nature. (2019) 572:27–9. doi: 10.1038/d41586-019-02307-y
44. DATAMIND collection of phenotypes in HDR-UK Phenotype Library. Available online at: https://phenotypes.healthdatagateway.org/phenotypes/?collections=27.
45. Catalogue of mental health measures. Available online at: https://www.cataloguementalhealth.ac.uk/.
Keywords: mental health, epidemiology, machine learning, research methods, challenges and opportunities
Citation: DelPozo-Banos M, Stewart R and John A (2024) Machine learning in mental health and its relationship with epidemiological practice. Front. Psychiatry 15:1347100. doi: 10.3389/fpsyt.2024.1347100
Received: 30 November 2023; Accepted: 22 February 2024;
Published: 11 March 2024.
Edited by:
Hugo Schnack, Utrecht University, NetherlandsReviewed by:
Seyed Mostafa Kia, Tilburg University, NetherlandsCopyright © 2024 DelPozo-Banos, Stewart and John. This is an open-access article distributed under the terms of the Creative Commons Attribution License (CC BY). The use, distribution or reproduction in other forums is permitted, provided the original author(s) and the copyright owner(s) are credited and that the original publication in this journal is cited, in accordance with accepted academic practice. No use, distribution or reproduction is permitted which does not comply with these terms.
*Correspondence: Marcos DelPozo-Banos, bS5kZWxwb3pvYmFub3NAc3dhbnNlYS5hYy51aw==