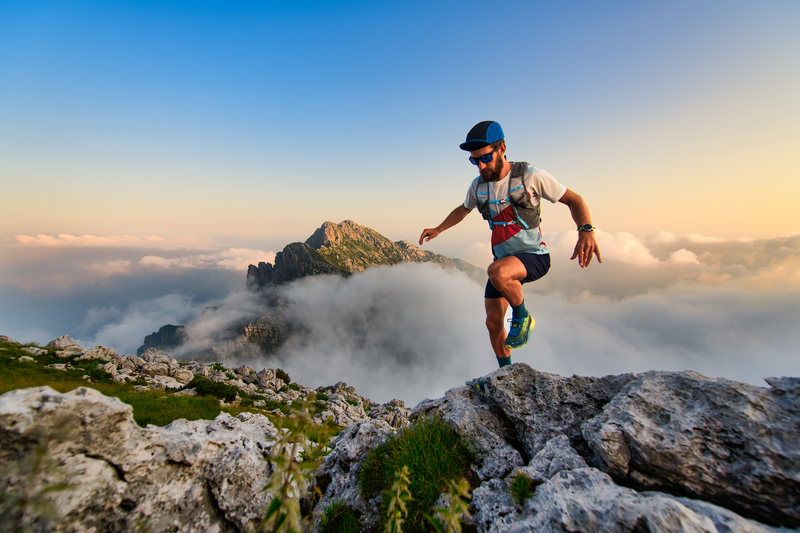
94% of researchers rate our articles as excellent or good
Learn more about the work of our research integrity team to safeguard the quality of each article we publish.
Find out more
OPINION article
Front. Psychiatry , 30 April 2024
Sec. Sleep Disorders
Volume 15 - 2024 | https://doi.org/10.3389/fpsyt.2024.1340843
In the modern society, where the pace of life is unrelenting and stress levels are escalating, sleep-related issues have garnered significant attention (1–4). Sleep, intricately linked to overall health, plays a pivotal role in psychological, physiological, and cognitive functions. Leveraging the National Health and Nutrition Examination Survey (NHANES) database for sleep-related research has emerged as a prominent area of academic interest. Current studies have explored the association between sleep and different health outcomes, such as mortality (5), aging process (6), inflammation (7), metabolic health (8), cardiovascular function (9), cognitive performance (10), and mental health (11).
However, a great number of world sleep researchers, especially doctors in the sleep medicine field, are not aware of the value of NHANES in exploring sleep health. One of the primary hurdles researchers face when delving into sleep health using the NHANES database is the lack of comprehensive knowledge about the sleep-related variables it contains. The database encompasses an extensive array of health-related data, making it challenging for researchers to pinpoint which specific variables pertain to sleep. Another significant challenge lies in the uncertainty surrounding the distribution of sleep-related variables across different survey years within the NHANES database. Given that not all sleep variables are present in a single year cycle, choosing the appropriate year to search for specific variables of interest holds significant importance. Finally, understanding the relationship between sleep-related variables and various health indicators presents a challenge. Researchers may encounter difficulties in designing studies that effectively tease out the influence of sleep on specific health indicators, given the multitude of confounding variables that may be at play.
This study aims to summarize key research methods and insights for utilizing the NHANES database in exploring sleep and its associated health indicators. Firstly, it seeks to uncover sleep-related variables pertaining to sleep duration, quality, and disturbances. Secondly, it aims to compile information on the distribution of these variables across different survey years.
NHANES, conducted by the National Center for Health Statistics (NCHS), is a nationwide and cross-sectional initiative aimed at assessing the health and nutritional status of the American populace (12). NHANES collects data from the non-institutionalized civilian population and releases this information in two-year cycles. This extensive database encompasses diverse participants from various racial, age, and socio-economic backgrounds, ensuring its broad representativeness and applicability.
In this section, we summarize tools and methods for sleep assessment in different year cycles of NHANES 2005-2018. The sleep questions were conducted at participants’ homes during the household interview using the Computer-Assisted Personal Interviewing (CAPI) system (2). Within this database, data related to sleep are primarily collected through the following methods, which is shown in Table 1.
Referring to previous literature (13–15), NHANES employs several validated questionnaire tools, such as sleep duration assessment and sleep disorders questionnaires to evaluate participants’ sleep patterns and quality.
Sleep Duration: The database includes self-reported sleep duration (in h for hours) in year cycles from 2005-2018. Participants are queried about their nightly sleep duration, providing a snapshot of how much sleep they typically receive. It should be noted that since 2017, the NHANES records sleep and wake time on weekdays and weekends, which allows more perspective to analyze the impact of weekend sleep supplementation on health outcomes.
Sleep Disturbances: NHANES delves into various sleep disturbances, encompassing issues like difficulty falling asleep, insomnia, sleep apnea, early morning awakenings, and instances of restless legs. This comprehensive evaluation allows for the identification of specific challenges individuals may face in achieving restful sleep and aids in identifying potential cases of obstructive sleep apnea. Moreover, NHANES assesses symptoms associated with Restless Leg Syndrome (RLS), including uncomfortable sensations in the legs, especially during periods of rest or inactivity. Understanding RLS prevalence provides valuable insights into this common sleep-related movement disorder.
Influence of Sleep Disturbances: This part includes different feelings of sleep disturbances, such as tiredness, leg jerks, and daytime sleepiness. Taking daytime sleepiness as an example, the questionnaire includes inquiries about daytime sleepiness, which can be indicative of sleep-related disorders or insufficient sleep duration. Understanding the influence of sleep troubles provides critical insights into the impact of sleep patterns on daily functioning.
NHANES year cycles 2011-2014 introduced accelerometer-based sleep measurement such as physical activity monitors (PAM), which monitor movement and gravitational forces, providing data on physical activity levels, sedentary behavior, and sleep patterns.
There are multiple advantages to accelerometer-based sleep measurement. Unlike self-reported sleep data, accelerometry offers an objective and quantitative assessment of sleep parameters, reducing potential biases associated with subjective reporting. In addition, accelerometers enable continuous monitoring of sleep patterns (at least for one week), allowing for the assessment of changes over time. This objective measurement significantly enhances the accuracy and reliability of sleep-related information. There are several key sleep parameters obtained via accelerometry:
Sleep Timing: By assessing activity levels throughout the night, accelerometers provide information on sleep onset and offset times, aiding in the characterization of sleep-wake patterns.
Sleep Duration: Accelerometers provide a reliable estimate of total sleep duration. By analyzing periods of minimal movement, researchers can discern when individuals are in a state of restful sleep.
Sleep Efficiency: This metric assesses the percentage of time spent asleep while in bed. It helps differentiate between time spent in bed and actual time asleep, offering a more nuanced understanding of sleep quality.
Awakening Frequency: Accelerometry allows for the identification of awakenings during the night, providing valuable insights into sleep continuity and potential disruptions.
Sleep Onset Latency: This parameter measures the time it takes for an individual to transition from wakefulness to sleep once in bed. It offers crucial information on the ease of falling asleep.
In summary, the integration of accelerometers within the NHANES database represents a significant advancement in sleep research. This objective measurement approach enhances the precision and scope of sleep-related information, allowing for more robust analyses and deeper insights into the complex interplay between sleep and overall health in the American population. A recent publication has validated the single-item self-reported sleep duration in NHANES by comparing it with wrist-worn accelerometer data. The study suggested that associations between sleep duration and other health outcomes identified using NHANES data should undergo further examination using more accurate and valid measures of sleep duration (16, 17). However, one limitation worth noting is that relying solely on accelerometers does not allow for the differentiation between different sleep stages, such as rapid eye movement (REM) sleep and non-rapid eye movement (NREM) sleep. To gain a more comprehensive understanding of sleep patterns and their impact, polysomnography (PSG) emerges as a valuable tool, particularly in populations with shortened sleep durations, enabling a deeper insight into sleep architecture and stage distribution.
From the perspective of the association between sleep and chronic diseases, leveraging NHANES, researchers can delve into the relationship between sleep and chronic diseases such as cardiovascular ailments and diabetes. Studies have revealed a positive correlation between inadequate sleep and the incidence of chronic diseases (5–9), underscoring the significance of maintaining good sleep for prevention and management. Additionally, NHANES offers a wealth of mental health information, including data on depressive symptoms and anxiety levels. Research indicates a close link between sleep quality and mental well-being, with poor sleep potentially acting as a significant factor in various mental health issues (18). Currently, there is also evidence that environmental exposure, nutritional status, and physical activity all have influence on sleep health (19–22). Furthermore, NHANES permits researchers to conduct sleep studies on specific demographic groups such as children, adolescents, and the elderly (23–25). By comparing sleep characteristics across different age groups, a more comprehensive understanding of the impact of sleep at various life stages can be gained. However, NHANES was a cross-sectional design, which limited causal interference. Thus, further longitudinal studies are warranted to assess the association between sleep patterns and various health outcomes over time.
In conclusion, NHANES stands as a robust resource for investigating the relationship between sleep patterns and overall health. Its extensive data repository offers a myriad of opportunities for researchers to delve into this critical intersection. With the continued refinement of methodologies and the incorporation of advanced assessment tools, NHANES is poised to play an even more significant role in advancing our understanding of sleep and health.
YY: Writing – review & editing, Writing – original draft, Validation, Supervision, Methodology, Investigation, Funding acquisition, Formal analysis, Conceptualization. YC: Writing – review & editing, Writing – original draft, Validation, Methodology, Investigation, Formal analysis, Conceptualization. MW: Conceptualization, Investigation, Formal analysis, Methodology, Validation, Writing – review & editing.
The author(s) declare financial support was received for the research, authorship, and/or publication of this article. This study was supported by the National Social Science Fund of China (Grant number BIA190201, general program of pedagogy: research on the financing and investment management of China’s “Double First-Class” universities under the new normal) and “Cross-discipline supporting program” from the School of Social Science, Tsinghua University.
The authors declare that the research was conducted in the absence of any commercial or financial relationships that could be construed as a potential conflict of interest.
All claims expressed in this article are solely those of the authors and do not necessarily represent those of their affiliated organizations, or those of the publisher, the editors and the reviewers. Any product that may be evaluated in this article, or claim that may be made by its manufacturer, is not guaranteed or endorsed by the publisher.
1. Siegel JM. Sleep function: an evolutionary perspective. Lancet Neurol. (2022) 21:937–46. doi: 10.1016/S1474-4422(22)00210-1
2. Di H, Guo Y, Daghlas I, Wang L, Liu G, Pan A, et al. Evaluation of sleep habits and disturbances among us adults, 2017-2020. JAMA Netw Open. (2022) 5:e2240788. doi: 10.1001/jamanetworkopen.2022.40788
3. Giannos P, Prokopidis K, Candow DG, Forbes SC, Celoch K, Isanejad M, et al. Shorter sleep duration is associated with greater visceral fat mass in us adults: findings from nhanes, 2011-2014. Sleep Med. (2023) 105:78–84. doi: 10.1016/j.sleep.2023.03.013
4. You Y, Chen Y, Zhang Q, Yan N, Ning Y, Cao Q. Muscle quality index is associated with trouble sleeping: A cross-sectional population based study. BMC Public Health. (2023) 23:489. doi: 10.1186/s12889-023-15411-6
5. Huyett P, Siegel N, Bhattacharyya N. Prevalence of sleep disorders and association with mortality: results from the nhanes 2009-2010. Laryngoscope. (2021) 131:686–9. doi: 10.1002/lary.28900
6. Wang X, Xu Y, Li X, Mansuri A, McCall WV, Liu Y, et al. Day-to-day deviations in sleep parameters and biological aging: findings from the nhanes 2011-2014. Sleep Health. (2023) 9(6):940–946. doi: 10.1016/j.sleh.2023.07.018
7. You Y, Chen Y, Fang W, Li X, Wang R, Liu J, et al. The association between sedentary behavior, exercise, and sleep disturbance: A mediation analysis of inflammatory biomarkers. Front Immunol. (2022) 13:1080782. doi: 10.3389/fimmu.2022.1080782
8. Smiley A, King D, Bidulescu A. The association between sleep duration and metabolic syndrome: the nhanes 2013/2014. Nutrients. (2019) 11(11):2582. doi: 10.3390/nu11112582
9. Cash RE, Crowe RP, Goldberg SA, Patrick C, Wells-Whitworth LM, Barger LK, et al. Association between sleep characteristics, ideal cardiovascular health, and systemic inflammation, nhanes 2017-2018. J Sleep Res. (2022) 31:e13497. doi: 10.1111/jsr.13497
10. You Y, Liu J, Wang D, Fu Y, Liu R, Ma X. Cognitive performance in short sleep young adults with different physical activity levels: A cross-sectional fnirs study. Brain Sci. (2023) 13(2):171. doi: 10.3390/brainsci13020171
11. You Y, Wei M, Chen Y, Fu Y, Ablitip A, Liu J, et al. The association between recreational physical activity and depression in the short sleep population: A cross-sectional study. Front Neurosci. (2023) 17:1016619. doi: 10.3389/fnins.2023.1016619
12. Nhanes questionnaires, datasets, and related documentation. Cdc.Gov. Available at: https://wwwn.cdc.gov/Nchs/Nhanes/.
13. Weaver TE, Laizner AM, Evans LK, Maislin G, Chugh DK, Lyon K, et al. An instrument to measure functional status outcomes for disorders of excessive sleepiness. Sleep. (1997) 20:835–43.
14. O’Connor GT, Lind BK, Lee ET, Nieto FJ, Redline S, Samet JM, et al. Variation in symptoms of sleep-disordered breathing with race and ethnicity: the sleep heart health study. Sleep. (2003) 26:74–9.
15. You Y, Chen Y, Liu R, Zhang Y, Wang M, Yang Z, et al. Inverted U-shaped relationship between sleep duration and phenotypic age in us adults: A population-based study. Sci Rep. (2024) 14:6247. doi: 10.1038/s41598-024-56316-7
16. Lee PH. Validation of the national health and nutritional survey (Nhanes) single-item self-reported sleep duration against wrist-worn accelerometer. Sleep Breath. (2022) 26:2069–75. doi: 10.1007/s11325-021-02542-6
17. You Y, Liu J, Li X, Wang P, Liu R, Ma X. Relationship between Accelerometer-Measured Sleep Duration and Stroop Performance: A Functional near-Infrared Spectroscopy Study among Young Adults. PeerJ. (2024) 12:e17057. doi: 10.7717/peerj.17057
18. Izci-Balserak B, Zhu B, Wang H, Bronas UG, Gooneratne NS. Independent associations between sleep duration, gamma gap, and cognitive function among older adults: results from the nhanes 2013-2014. Geriatr Nurs. (2022) 44:1–7. doi: 10.1016/j.gerinurse.2021.12.019
19. You Y, Chen Y, Zhang Y, Zhang Q, Yu Y, Cao Q. Mitigation role of physical exercise participation in the relationship between blood cadmium and sleep disturbance: A cross-sectional study. BMC Public Health. (2023) 23:1465. doi: 10.1186/s12889-023-16358-4
20. Deng MG, Liu F, Liang Y, Chen Y, Nie JQ, Chai C, et al. Associations of serum zinc, copper, and selenium with sleep disorders in the american adults: data from nhanes 2011-2016. J Affect Disord. (2023) 323:378–85. doi: 10.1016/j.jad.2022.11.088
21. You Y, Chen Y, Wei M, Tang M, Lu Y, Zhang Q, et al. Mediation role of recreational physical activity in the relationship between the dietary intake of live microbes and the systemic immune-inflammation index: A real-world cross-sectional study. Nutrients. (2024) 16(6):777. doi: 10.3390/nu16060777
22. You Y, Wang R, Li J, Cao F, Zhang Y, Ma X. The role of dietary intake of live microbes in the association between leisure-time physical activity and depressive symptoms: A population-based study. Appl Physiol Nutr Metab. (2024). doi: 10.1139/apnm-2023-0550
23. You Y, Mo L, Tong J, Chen X, You Y. The role of education attainment on 24-hour movement behavior in emerging adults: evidence from a population-based study. Front Public Health. (2024) 12:1197150. doi: 10.3389/fpubh.2024.1197150
24. Lloyd-Jones DM, Ning H, Labarthe D, Brewer L, Sharma G, Rosamond W, et al. Status of cardiovascular health in us adults and children using the american heart association’s new “Life’s essential 8” Metrics: prevalence estimates from the national health and nutrition examination survey (Nhanes), 2013 through 2018. Circulation. (2022) 146:822–35. doi: 10.1161/CIRCULATIONAHA.122.060911
Keywords: NHANES, cross-sectional study, sleep, methods, insights
Citation: You Y, Chen Y and Wei M (2024) Leveraging NHANES database for sleep and health-related research: methods and insights. Front. Psychiatry 15:1340843. doi: 10.3389/fpsyt.2024.1340843
Received: 22 November 2023; Accepted: 10 April 2024;
Published: 30 April 2024.
Edited by:
Xiaofei Hu, Army Medical University, ChinaReviewed by:
Brian K. Gehlbach, The University of Iowa, United StatesCopyright © 2024 You, Chen and Wei. This is an open-access article distributed under the terms of the Creative Commons Attribution License (CC BY). The use, distribution or reproduction in other forums is permitted, provided the original author(s) and the copyright owner(s) are credited and that the original publication in this journal is cited, in accordance with accepted academic practice. No use, distribution or reproduction is permitted which does not comply with these terms.
*Correspondence: Yanwei You, eXl3MjJAbWFpbHMudHNpbmdodWEuZWR1LmNu
†These authors have contributed equally to this work
Disclaimer: All claims expressed in this article are solely those of the authors and do not necessarily represent those of their affiliated organizations, or those of the publisher, the editors and the reviewers. Any product that may be evaluated in this article or claim that may be made by its manufacturer is not guaranteed or endorsed by the publisher.
Research integrity at Frontiers
Learn more about the work of our research integrity team to safeguard the quality of each article we publish.