- Department of Psychology, Renmin University of China, Beijing, China
Delay discounting, the decline in the subjective value of future rewards over time, has traditionally been understood through a tripartite neural network model, comprising the valuation, cognitive control, and prospection networks. To investigate the applicability of this model in a resting-state context, we employed a monetary choice questionnaire to quantify delay discounting and utilized resting-state functional magnetic resonance imaging (rs-fMRI) to explore the role of spontaneous brain activity, specifically regional homogeneity (ReHo), in influencing individual differences in delay discounting across a large cohort (N = 257). Preliminary analyses revealed a significant negative correlation between delay discounting tendencies and the ReHo in both the left insula and the right hippocampus, respectively. Subsequent resting-state functional connectivity (RSFC) analyses, using these regions as seed ROIs, disclosed that all implicated brain regions conform to the three principal networks traditionally associated with delay discounting. Our findings offer novel insights into the role of spontaneous neural activity in shaping individual variations in delay discounting at both regional and network levels, providing the first empirical evidence supporting the applicability of the tripartite network model in a resting-state context.
1 Introduction
Consider two everyday dilemmas: You’re aware of the long-term health benefits of physical exercise, yet the immediate allure of a riveting television show or an engaging video game keeps you anchored to the couch. Or, you’re a student facing an impending exam that could shape your academic future, but the temptation to socialize or dive into another round of gaming is strong. Given the choice, will you favor the immediate satisfaction or defer gratification for future gains? Often, when faced with a smaller, immediate reward (SIR), such as a riveting television show or another round of gaming, and a larger, delayed reward (LDR), such as health benefits from exercise or enhanced academic future, we tend to opt for the former. This tendency is termed delay discounting, a psychological construct defined by the diminishing subjective value of a reward as the waiting period increases (1). Delay discounting is found to be associated with a plethora of unhealthy behaviors, including drug addiction (2), obesity (3), internet addiction (4), gambling (5), and alcohol use disorder (6).
The systematic devaluation of future rewards is commonly understood to arise from the complex interplay among a tripartite neural network model: valuation, cognitive control, and prospection, as delineated in existing neuroscientific paradigms (7). The valuation network, composed of the ventral medial prefrontal cortex (vmPFC), medial orbitofrontal cortex (mOFC), ventral striatum (VS), and posterior cingulate gyrus (PCC), is considered crucial for valuation, with the insula playing a potential role (8, 9). The cognitive control network, encompassing the lateral prefrontal cortex (LPFC) and anterior cingulate gyrus (ACC), facilitates cognitive control, conflict monitoring, and strategy adaptation. The prospection network, primarily involving the medial temporal lobe areas like the hippocampus, facilitates future-oriented thinking. These networks are widely employed to explain delay discounting at the neural level (9–12). Nonetheless, the extent to which spontaneous neural activity relevant to delay discounting aligns with the theoretical underpinnings of the tripartite neural network model has yet to be elucidated. We posit that these principal neural networks, which are instrumental in governing delay discounting behavior, persist in their interactions during the resting state and may account for observed individual variances in delay discounting propensities.
Our research focuses on the regional homogeneity (ReHo) of spontaneous neural activity in a resting state. ReHo quantifies the temporal synchronicity between a specific voxel (a unit of three-dimensional space) and its neighboring voxels, serving as a metric for local functional connectivity. To test our hypothesis, we initially aimed to pinpoint brain regions implicated in delay discounting by examining the correlation between ReHo and delay discounting across each voxel in a substantial sample of participants (N = 257). Following the identification of salient brain regions, we employed these areas as seed regions to calculate whole-brain functional connectivity. This approach was designed to explore the interplay among neural networks at rest and elucidate their roles in shaping individual tendencies toward delay discounting.
2 Materials and methods
2.1 Participants
Our study enrolled 310 university students, ranging in age from 18 to 23 years (mean age = 20.36 years, SD = 0.85). Of these, 186 were females. Eight participants did not disclose their age. All participants had no reported history of neurological or psychiatric disorders. The study received approval from the Institutional Review Board of Beijing Normal University, and all participants provided informed consent before commencing the experiment.
2.2 Behavioral measures
2.2.1 Delay discounting - money choice questionnaire
We employed the Money Choice Questionnaire to evaluate the level of delay discounting among participants. This questionnaire, composed of 27 items, prompts participants to choose between smaller immediate rewards and larger delayed rewards. An example item is, “Would you prefer to receive ¥31 immediately following the experiment, or ¥85 after 7 days?” The questionnaire did not impose a time limit.
To encourage participants to make choices reflective of their genuine preferences, each participant was informed they would not only receive a reward after the experiment, but also a randomly determined reward corresponding to one of their 27 choices. In our study, the Money Choice Questionnaire was utilized to classify delayed rewards into three categories: small (S), medium (M), and large (L). Each category is associated with 10 distinct choice patterns, each linked to a specific ‘k’ value. Participants were assigned a ‘k’ value that corresponded to the highest proportion of their choices aligning with that value. Essentially, for each participant, we calculated the proportion of their choices consistent with each of the 10 ‘k’ values defined by the questionnaire. The ‘k’ value that demonstrated the highest consistency with the participant’s choices was then assigned to them. To ensure the precision of our data, only participants who exhibited a consistency rate of 85% or higher in their responses were included in our analysis.
Although the participants’ delay discounting rates in our study were determined by aligning their choices with the 10 specific ‘k’ values, the significance of the ‘k’ value itself warrants a detailed explanation. The hyperbolic discounting model, expressed as V = A/(1 + k*D), is a foundational mathematical model in delay discounting research (13). In this formula, A represents the delayed reward, D the delay duration, and V the present value of reward A at delay D. This model delineates the reduction in subjective value of a future reward (V) as the delay (D) increases in relation to the actual reward value (A), where ‘k’ is an indicator of an individual’s delay discounting level. A higher ‘k’ value, for a given A and D, results in a lower present value (V), indicating greater levels of delay discounting and impulsivity. However, it is crucial to recognize that in the hyperbolic discounting model, the distribution of ‘k’ values is typically skewed (14–16). The subjective value V does not surpass the actual value A after a brief delay and becomes negative after an extended delay (14). Therefore, in our analysis, we employed the median as a measure of central tendency and applied a natural logarithm transformation to the ‘k’ values, ensuring a normal distribution of the calculated Ln(k) (17).
2.2.2 Intelligence measurement - Raven’s advanced progressive matrices
Previous meta-analytic research has suggested a negative correlation between intelligence and delay discounting—higher intelligence associates with lower delay discounting (18). Moreover, fMRI studies have reported that greater self-control, reflected as lower delay discounting, correlates with higher intelligence (19). General intelligence may influence the preference for immediate rewards, thus reducing impulsivity in delay discounting tasks (20). To consider intelligence’s impact on delay discounting, we utilized Raven’s Advanced Progressive Matrices to measure participants’ intelligence. This test, comprising 36 items, requires participants to select the missing figure to complete a 3 × 3 matrix. We used the number of correct responses provided within a 30-minute window as the intelligence score.
2.3 Image acquisition
We collected imaging data using a Siemens MAGNETOM Trio 3T scanner equipped with a 12-channel phased-array head coil at the Brain Imaging Research Center, Beijing Normal University, Beijing, China. The resting-state scan included 240 consecutive echo-planar imaging (EPI) volumes (TR = 2000 ms; TE = 30 ms; flip angle = 90°; number of slices = 33; matrix = 64 × 64; FOV = 200 × 200 mm²; acquisition voxel size = 3.125 × 3.125 × 3.6 mm³). Additionally, we acquired high-resolution T1-weighted images using a magnetization-prepared gradient echo sequence (MPRAGE: TR/TE/TI = 2530/3.39/1100 ms; flip angle = 7°; matrix = 256×256; number of slices = 128; voxel size = 1 × 1 × 1.33 mm³) to facilitate spatial registration. During the scanning session, we instructed participants to close their eyes, maintain stillness, stay awake, and refrain from engaging in purposeful thinking.
2.4 Image data preprocessing
Resting-state fMRI data underwent preprocessing using the FMRI Expert Analysis Tool (FEAT Version 5.98), part of FMRIB’s Software Library (FSL, http://www.fmrib.ox.ac.uk/fsl). The preprocessing involved several steps: head motion correction (each volume was aligned to the central volume of the image series using MCFLIRT), spatial smoothing (applying a Gaussian kernel of 6-mm FWHM), intensity normalization, and linear trend removal. To mitigate the impact of physiological noise, including artifacts related to head motion, cardiac and respiratory cycles, our study employed a regression of 18 nuisance signals. These signals were derived from cerebrospinal fluid, white matter, global brain average, and motion correction parameters, as suggested in previous studies (21, 22). Specifically, our nuisance regressors encompassed the average cerebrospinal fluid signal, average white matter signal, global signal from the entire brain, six parameters from rigid-body head motion correction, and their derivatives (23). This approach in data preprocessing was meticulously chosen to address both linear and non-linear effects of head motion, thus substantially bolstering the reliability and validity of our study’s outcomes.
The registration of each participant’s resting-state fMRI to their anatomical images was accomplished using FMRIB’s Linear Image Registration Tool (FLIRT) to generate a 6 degree-of-freedom affine transformation matrix. The registration of each participant’s anatomical images to the Montreal Neurological Institute (MNI) space was accomplished by employing FLIRT to compute a 12 degree-of-freedom linear affine matrix (24, 25). In addition, considering the sensitivity of low-frequency fluctuations to spontaneous brain activity in gray matter regions (26), we defined a gray mask with a probability threshold of 0.5 in SPM8. The resulting gray mask incorporated a total of 128,190 voxels.
2.5 Statistical analysis
In our initial cohort, exclusions were made as follows: 37 participants due to incomplete scan data, 5 participants for demonstrating less than 85% consistency in the delay discounting task (17), 6 participants for lacking intelligence test data, 1 participant for missing age information, 1 participant for an intelligence score exceeding ±3 standard deviations, and 3 participants for head motion exceeding ±3 standard deviations. Following these exclusions, the final dataset consisted of 257 participants (157 females). Their ages ranged from 18 to 23 years (mean = 20.35, SD = 0.87), intelligence scores spanned from 15 to 34 (mean = 25.74, SD = 3.99). In our study, we employed Framewise Displacement (FD), measured in millimeters (mm), as the parameter for assessing head motion. Following the exclusion of participants with head motion exceeding ±3 standard deviations, as previously described, the head motion parameters for those included in the final dataset were as follows: The FD values ranged from 0.036 to 0.213 mm, with an average of 0.102 mm and a standard deviation of 0.033 mm. So that, our study did not encompass participants whose mean FD value was greater than 0.3mm. Consequently, the head motion of the participants included in our study remained within acceptable levels (27). The kurtosis and skewness of age (− 0.21, 0.06) and Raven scores (− 0.32, − 0.11) ranged from −1 to +1, indicating both age and intelligence data conformed to a normal distribution (28).
2.5.1 Regional homogeneity-delay discounting correlation analysis
Regional Homogeneity (ReHo), a voxel-based measure of brain activity, operates on the assumption that the temporal pattern of a given voxel is akin to its neighboring voxels (29). This methodology aligns with the hypothesis that intrinsic brain activity is reflected by clusters of voxels rather than isolated voxels (30). In this study, we utilized the Kendall’s coefficient of concordance (KCC) as a metric to gauge ReHo (31). After controlling for age, sex, intelligence, and head motion parameters, we computed the correlation between the natural logarithm of k (Ln(k)) and ReHo, which measures the regional homogeneity of the whole-brain Blood-Oxygen-Level-Dependent (BOLD) signal. Multiple comparison correction in our study was conducted using the Gaussian Random Field theory (GRF) as implemented in the DPABI software (32). GRF is an established method in the domain of neuroimaging data analysis, particularly adept for handling 3D data. This approach is notable for its consideration of the spatial structure of neuroimaging data, which enhances the accuracy of multiple comparison corrections. For our analysis, we set the significance thresholds for both voxel level and cluster level evaluations at p < 0.05.
2.5.2 Resting-state functional connectivity-delay discounting correlation analysis
Building upon the ReHo-delay discounting correlation analysis, we sought to further investigate the neural network associated with delay discounting during resting-state by employing resting-state functional connectivity (RSFC). Initially, the clusters identified in the ReHo-delay discounting correlation analysis were used as seeds. To ensure an adequate capture of the BOLD signal changes within the clusters, we created a sphere (radius of 5 mm) centered on the voxel demonstrating the most robust ReHo-delay discounting correlation within the cluster (33). The mean time series of the overlap between this cluster and the sphere were then extracted. Subsequently, we computed the functional connectivity by correlating the mean time series obtained from the seed with the time series of each voxel across the whole brain, while controlling for head motion, age, gender, and intelligence. Ultimately, for each seed, we identified the neural network involved in delay discounting by assessing the correlation between its functional connectivity and delay discounting for each voxel in the whole brain. In our analysis, the r-maps were converted into T-score maps. For the purpose of multiple comparison correction, we utilized the GRF as implemented in the DPABI software, following the guidelines of Yan et al. (32). We established the significance threshold for voxel level analysis at p < 0.05. Recognizing the importance of minimizing type I errors (false positives), a more stringent threshold of p < 0.01 was adopted at the cluster level. This approach aimed to achieve a balance between sensitivity and specificity in our statistical analysis, within the constraints of the current methodology and data.
3 Results
The delay discounting of the participants was denoted by the Ln(k) value, with higher Ln(k) values signaling elevated impulsivity levels, indicative of a preference for immediate and smaller rewards. The average Ln(k) for the participants was − 5.2, accompanied by a standard deviation of 1.53. The Ln(k) distribution followed a normal distribution pattern with a kurtosis of − 0.09 and skewness of − 0.07, both within the − 1 to 1 range. This attests to the reliability of using Ln(k) as a measure of delay discounting to probe the neural mechanisms of individuals in a resting state (28).
To investigate all brain regions implicated in delay discounting at a resting state, we computed the correlation between regional homogeneity (ReHo) and delay discounting in each voxel of the entire brain across all subjects. Post adjustment for head motion, age, and sex, we discovered a significant negative correlation of Ln(k) with two regions: the left insula (cluster size = 569; MNI coordinate: − 28, 0, − 16; GRF corrected, two tailed, voxel level p < 0.05, cluster level p < 0.05) and the right hippocampus (cluster size = 404; MNI coordinate: 16, − 14, − 16; GRF corrected, two tailed, voxel level p < 0.05, cluster level p < 0.05). This outcome implies that the insula and hippocampus contribute to individual differences in delay discounting at a resting state. More specifically, stronger ReHo of the insula and hippocampus translates to lower delay discounting, and an increased propensity to consider future choices of delayed rewards. In this analysis, no other significant results were identified.
Individual intelligence is associated with the functioning of several brain regions, and both the hippocampus (34–37) and the insula (36, 38) have demonstrated associations with intelligence. Consequently, to control for the confounding influence of individual differences in intelligence on the associations between delay discounting and spontaneous brain activity in both the left insula and the right hippocampus, we performed supplementary analyses using intelligence as a covariate. Upon controlling for intelligence, head motion, age, and gender, we still observed a significant association between the left insula and delay discounting (cluster size = 570; MNI coordinate: − 40, 18, − 12; GRF corrected, two tailed, voxel level p < 0.05, cluster level p < 0.05; Figure 1A), and the right hippocampus and delay discounting (cluster size = 405; MNI coordinate: 16, − 14, − 16; GRF corrected, two tailed, voxel level p < 0.05, cluster level p < 0.05; Figure 1B). Such findings suggest that the relationship between the left insula and delay discounting, and the right hippocampus and delay discounting at a resting state is stable and is not attributed to variations in intelligence. Notably, the relationships between the left insula and delay discounting, as well as the right hippocampus and delay discounting, were not found to be statistically significant when analyzed using a permutation test with threshold-free cluster enhancement (PT TFCE), involving 1,000 permutations and adhering to a family-wise error rate (FWER) of less than 0.05.
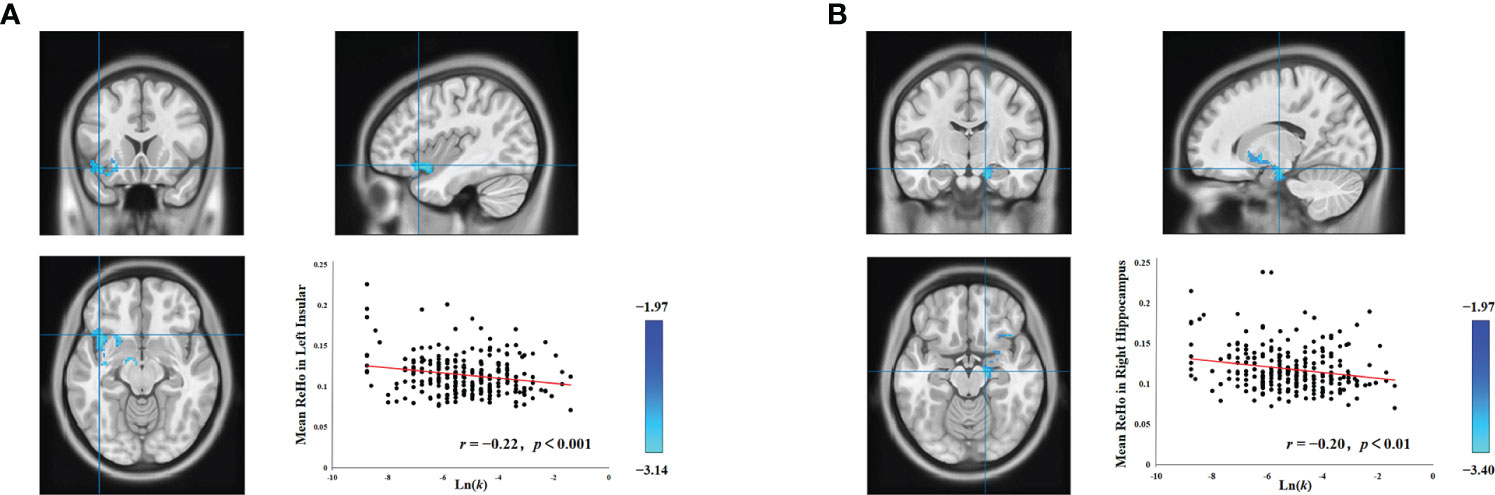
Figure 1 Associations between delay discounting and neural activity in the left insula and right hippocampus. (A) A cluster in the left insula exhibited a significant negative correlation between ReHo values and the Ln(k) values. (B) Similarly, a cluster in the right hippocampus also displayed a significant negative correlation between ReHo values and the Ln(k) values. For illustrative purposes, the scatter plot shows the correlations, adjusted for covariates including intelligence, age, gender, and head motion. Each data point represents an individual participant.
Upon establishing the roles of the left insula and the right hippocampus in delay discounting during resting states, we used these ROIs separately as seed regions to investigate the neural network associated with delay discounting via whole-brain resting-state functional connectivity (RSFC). Specifically, we assessed the correlation between the Ln(k) values and RSFC for each voxel relative to the left insula and right hippocampus across all participants. These analyses were conducted after controlling for variables such as intelligence, age, gender, and head motion. We found that the RSFC between the left insula and the right insula was significantly positively correlated with participants’ Ln(k) values (r = 0.20, p < 0.01; Table 1, Figure 2; the right insula cluster size: 560; MNI coordinate: 46, − 6, 8; GRF corrected, two tailed, voxel level p < 0.05, cluster level p < 0.01). Specifically, increased RSFC between the left and right insula was associated with higher Ln(k) values, indicating a greater tendency for participants to opt for smaller, immediate rewards (SIRs) due to a steeper discounting of future values. Conversely, when the right hippocampus served as the seed ROI, we identified significant positive or negative correlations between the RSFC of the right hippocampus and five discrete clusters in the vmPFC, PCC, and LPFC with participants’ Ln(k) values, as illustrated in Table 1; Figure 2. These results underscore the modulatory effect of resting-state functional connectivity among these neural regions on individuals’ delay discounting.
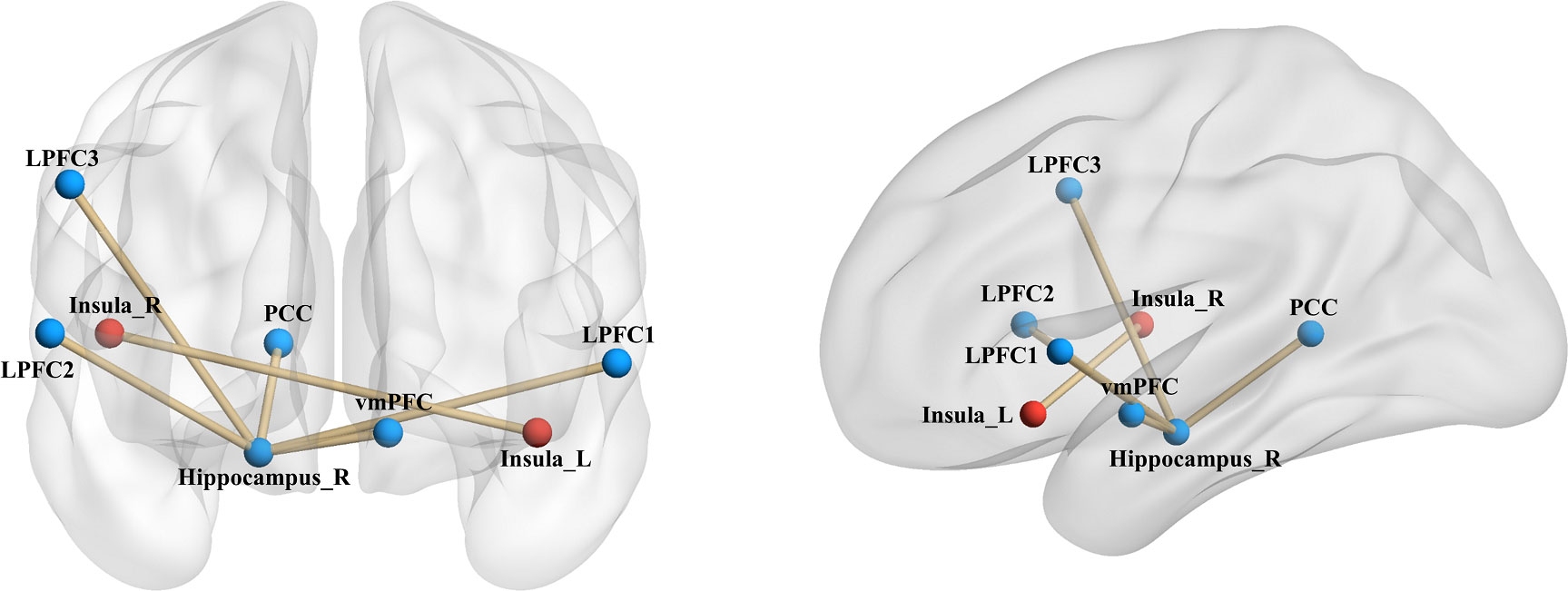
Figure 2 Seed-Based RSFC analysis highlighting associations with delay discounting. Utilizing the left insula and the right hippocampus as seed regions, distinct connectivity patterns were observed. The RSFC between the left insula and the right insula, denoted by red markers in the figure, was significantly positively correlated with the Ln(k) values. With respect to the right hippocampus as a seed region, its RSFC with both the vmPFC and the PCC demonstrated a significant negative correlation with the Ln(k) values. Conversely, connectivity with three clusters in the LPFC showed a significant positive correlation with the Ln(k), as indicated by blue markers in the figure. Nodes and edges representing these connectivity patterns are overlaid on inflated cortical surface maps, generated using BrainNet Viewer (39).
4 Discussion
This study employed rs-fMRI to probe the brain networks involved in individual differences in delay discounting, using a substantial sample of healthy university students. Initially, we observed the significant negative correlations between regional homogeneity (ReHo) and delay discounting, particularly within the left insula and the right hippocampus. Stronger regional homogeneity of spontaneous activity in these brain regions was associated with lower delay discounting, implying a higher likelihood of choosing delayed rewards. Further exploration of the neural networks involved in delay discounting at rest was conducted using the left insula and the right hippocampus as seed regions. Interestingly, all the brain regions implicated in individual differences in delay discounting that we identified in the resting-state functional connectivity (RSFC) analysis belong to the three principal networks: the valuation, cognitive control, and prospection networks. This study is the first to elucidate, from the perspective of spontaneous neural activity, how these three neural networks interact with each other to influence individual variability in delay discounting.
Drawing on empirical evidence from cognitive neuroscience methodologies such as fMRI, previous research has posited that three principal neural networks (valuation, cognitive control, and prospection) are predominantly engaged in modulating delay discounting behavior (40–42). Moreover, Mehta et al. (43) conducted a connectome-wide association study (CWAS) to explore the interplay between RSFC and delay discounting in a demographic spanning 9 to 23 years. Their study pinpointed the posterior cingulate cortex (PCC), ventromedial prefrontal cortex (vmPFC), and lateral prefrontal cortex (LPFC) as crucial elements of these neural networks, substantially involved in delay discounting. Such findings provide additional validation for our research. Nonetheless, there remains a notable gap in empirical literature concerning the validation of this tripartite neural network framework in the context of spontaneous neural activity. Our current study aims to bridge this gap. Employing comprehensive whole-brain analyses at both regional and network scales, it is noteworthy that all identified brain regions associated with delay discounting fell exclusively within these three principal networks, as illustrated in Figure 3. Particularly, our study’s unique contribution is in delineating the interconnections among these principal networks through an RSFC analysis.
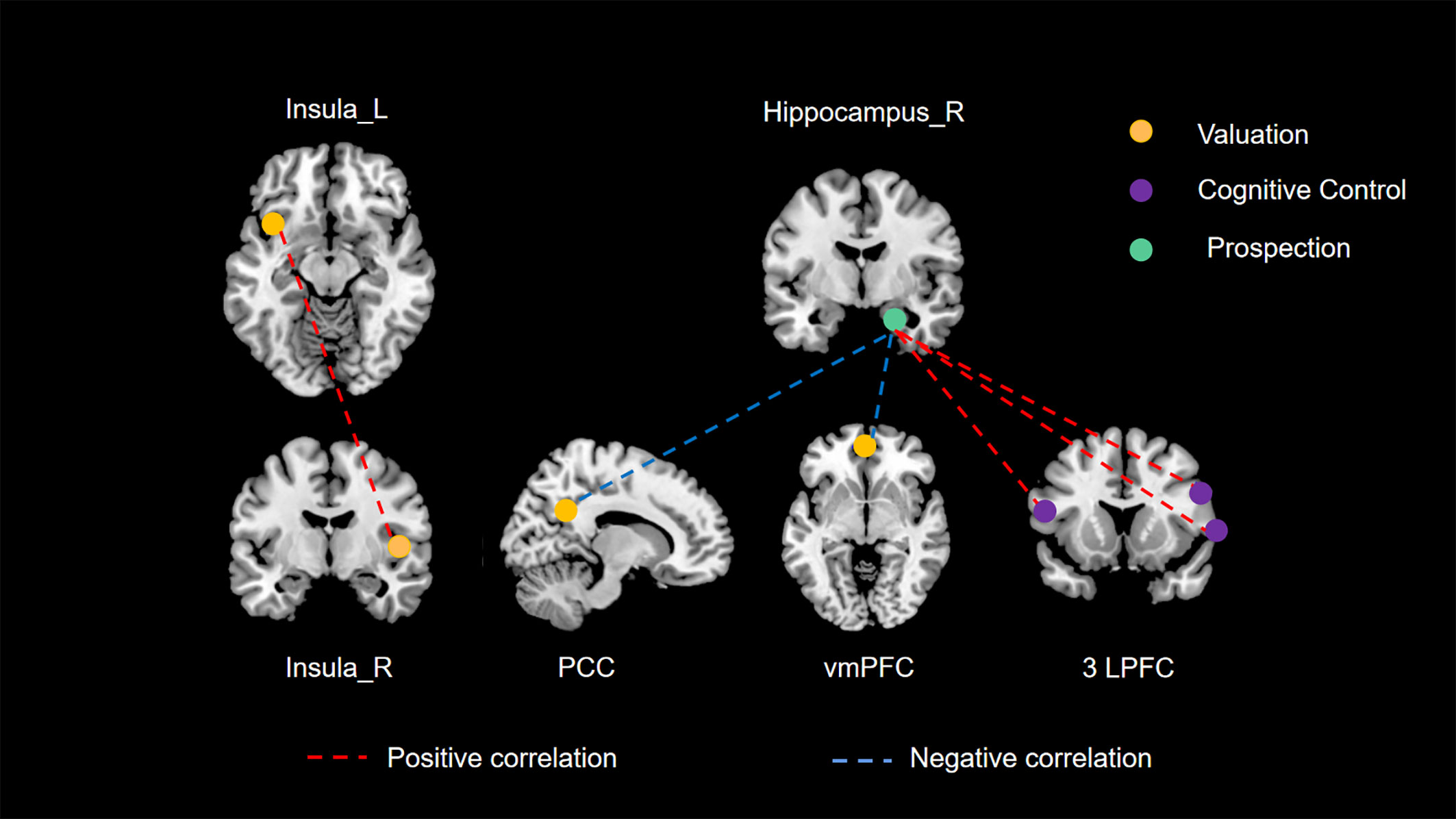
Figure 3 The three principal networks underlying delay discounting. This illustration delineates the three principal networks implicated in delay discounting: the valuation network (represented in yellow), the cognitive control network (represented in purple), and the prospection network (represented in green). The various colored dashed lines signify distinct relationships between resting-state functional connectivity (RSFC) and delay discounting. Specifically, red dashed lines denote significant positive correlations between functional connectivity and delay discounting across different brain regions, whereas blue dashed lines illustrate significant negative correlations.
Firstly, in our study, we found a significant positive correlation between the RSFC of the left and right insula and delay discounting behaviors. The insula is a crucial component of the valuation network, which involves several brain regions responsible for evaluating and appraising the intrinsic value of rewards (9, 44, 45). Increased activity within this network has been linked to impulsive choices and behaviors (7). Numerous studies have emphasized the insula’s significant role in the valuation process during decision-making (46, 47). In this context, enhanced RSFC between the left and right insular regions may suggest an increased integration and coordination within the valuation network. This augmented connectivity could potentially lead to a more efficient and rapid assessment of rewards, thus facilitating quicker response to reward-related stimuli. Consequently, this heightened state of responsiveness in the valuation network, as a result of increased insula connectivity, could predispose individuals to more impulsive decision-making. This is because the amplified insula communication may accelerate the valuation process, making immediate rewards appear more salient and desirable, and thus skewing choices towards impulsivity. This effect might overshadow the consideration of long-term outcomes, leading to a preference for immediate gratification over delayed rewards.
Secondly, the hippocampus, a central structure in the prospection network, interacts with the valuation and cognitive control networks. The ventromedial prefrontal cortex (vmPFC) and posterior cingulate cortex (PCC) are core regions within the valuation network, playing a role in individual value representation (48–52). The hippocampus-dominated prospection network is thought to be closely intertwined with the valuation network, as certain areas within the valuation network also belong to the prospection network (7). The prospection network is concerned with future planning and the projection of oneself into future scenarios. Consequently, the right hippocampus-vmPFC RSFC and right hippocampus-PCC RSFC may reflect the cooperative function of the prospection and valuation networks. Our study reveals that a stronger connectivity between the prospection network and the valuation network is associated with a greater propensity for individuals to opt for delayed rewards. This may be attributed to the prospection network’s emphasis on the future consequences of current choices (53, 54). Enhanced connectivity with the valuation network appears to inhibit the latter’s facilitative effect on impulsive choices, thereby aiding individuals in making decisions that favor long-term benefits over immediate gratification.
Furthermore, the lateral prefrontal cortex (LPFC), a crucial component of the cognitive control network, plays a pivotal role in managing decision-making complexities, particularly when choices bear comparable values (7). It primarily functions as a conflict monitor, aiding in the resolution of decision-making conflicts (55, 56). In our study, we observed a positive correlation between the RSFC of the right hippocampus and all three LPFC clusters in relation to delay discounting. This suggests that stronger RSFC between the right hippocampus and these LPFC clusters is associated with increased impulsivity. To elucidate further, the hippocampus is integral in encoding and retrieving memories (57, 58), and its interaction with the LPFC is critical for integrating past experiences into current decision-making processes. When the RSFC between these regions is enhanced, it could imply an overemphasis on immediate experiences or rewards at the expense of long-term considerations. This heightened connectivity may lead to a dominance of immediate rewards in decision-making, overshadowing the rational evaluation of delayed outcomes typically modulated by the LPFC. Consequently, this imbalance, where the cognitive control exerted by the LPFC is compromised by the amplified influence of the hippocampus, may result in a bias towards impulsive choices. This scenario highlights a potential mechanism whereby increased hippocampal-LPFC connectivity disrupts the equilibrium between immediate and future reward evaluation, steering decisions towards impulsivity.
Methodologically, our study supplements existing research on the neural networks involved in delay discounting during rest in three significant ways. Firstly, capitalizing on our substantial participant sample, we executed a whole-brain analysis at both the regional and neural network levels. This diverges from the common practice of merely selecting specific regions of interest for exploration (10, 59–63). A whole-brain-based analysis allows for a more comprehensive identification of the neural networks implicated in delay discounting. Secondly, in the computation of functional connectivity, we determined the mean time series within the region where regional homogeneity (ReHo) demonstrated the strongest correlation with delay discounting. We centered a 5-mm radius sphere in this region (33, 64–67). This method enhances the representativeness of our results and provides a valuable contribution to the study of neural networks involved in delay discounting. Thirdly, we considered the established connection between individual delay discounting and intelligence, where individuals with lower intelligence exhibit higher delay discounting (68, 69). Consequently, we controlled for the influence of intelligence when computing correlations, thus lending greater credibility to our findings.
However, this study also has certain limitations. Firstly, a significant limitation of our study pertains to the reliance on the threshold level selected for multiple comparisons correction. While our results demonstrated significance under more liberal thresholds, they did not sustain this significance when subjected to more stringent multiple comparisons correction. Specifically, at both the regional and network levels, the outcomes did not meet the rigorous voxel-level threshold of less than 0.01 (GRF correction). Additionally, our results were also unable to pass the permutation test with a p-value threshold of less than 0.05. This limitation accentuates a core challenge in neuroimaging research: balancing the identification of true effects (sensitivity) against the prevention of false positives (specificity). The employment of stringent thresholds, aimed at reducing false positive rates, concurrently elevates the risk of missing true small effect sizes, which were the primary focus of our investigation. Moreover, our adoption of a whole-brain correction approach, while methodologically rigorous, encountered difficulties in detecting small effect sizes due to the extensive correction base. This methodological choice necessitates a cautious interpretation of our findings, particularly concerning their replicability and applicability to other studies and diverse populations. It is crucial to consider these factors when evaluating the implications and generalizability of our research findings within the broader scientific context. Secondly, in our examination of the role of spontaneous neural activity in shaping individual variations in delay discounting at both regional and network levels, one notable limitation is the exclusion of socioeconomic status (SES) as a variable. Prior studies have highlighted the relevance of both subjective and objective SES in influencing delay discounting behaviors. For instance, research has shown that individuals with a higher perceived social status (subjective SES) tend to exhibit lower levels of delay discounting (70, 71). Similarly, objective measures of SES, such as parental education levels, have been linked to variations in delay discounting, as demonstrated in studies like Mehta et al. (43). Our study’s focus on neural mechanisms did not encompass the potential modulatory effects of SES, which could be a significant factor in delay discounting. Recognizing this, we suggest that future investigations in this field would benefit from integrating SES into their analytical frameworks to provide a more comprehensive understanding of the neural underpinnings of delay discounting.
In summary, by integrating results at the regional and neural network levels, this study contributes preliminary evidence illuminating how the valuation network, the cognitive control network, and the prospection network interact during resting state to influence individual delay discounting. Future research should delve deeper into this area. Firstly, although our whole-brain analysis only implicated certain brain regions within the three networks, this does not preclude the potential involvement of other brain regions within these networks. Their effects might approach statistical significance but fall short of our current threshold. Therefore, future investigations might select regions of interest within these three networks for in-depth examination. Secondly, our participant cohort comprised healthy university students. Future research could consider extending the focus to groups characterized by high impulsivity levels (i.e., high delay discounting), such as individuals with addiction. Insights at the neural level from such studies could be valuable for devising effective intervention and treatment strategies. Thirdly, delay discounting is traditionally utilized as a method for measuring impulsivity (72, 73). Importantly, prior research has identified a relationship between impulsivity, as measured by the Barratt Impulsiveness Scale, and in-scanner motion. Therefore, future research endeavors could be directed towards exploring delay discounting as a behavioral indicator of impulsivity. This would particularly include probing whether increased impulsivity, as manifested in delay discounting tasks, is associated with elevated in-scanner motion.
Data availability statement
The raw data supporting the conclusions of this article will be made available by the authors, without undue reservation.
Ethics statement
The studies involving humans were approved by Institutional Review Board of Beijing Normal University in Beijing, China. The studies were conducted in accordance with the local legislation and institutional requirements. The participants provided their written informed consent to participate in this study.
Author contributions
SJ: Writing – original draft, Data curation. FY: Data curation, Writing – original draft. XL: Conceptualization, Writing – review & editing.
Funding
The author(s) declare financial support was received for the research, authorship, and/or publication of this article. The research was financially supported by the Renmin University of China’s New Faculty Startup Fund (15XNLF07) and the China Postdoctoral Science Foundation (2015M571186).
Conflict of interest
The authors declare that the research was conducted in the absence of any commercial or financial relationships that could be construed as a potential conflict of interest.
Publisher’s note
All claims expressed in this article are solely those of the authors and do not necessarily represent those of their affiliated organizations, or those of the publisher, the editors and the reviewers. Any product that may be evaluated in this article, or claim that may be made by its manufacturer, is not guaranteed or endorsed by the publisher.
References
1. Kirby KN, Maraković NN. Modeling myopic decisions: evidence for hyperbolic delay-discounting within subjects and amounts. Organizational Behav Hum Decision Processes (1995) 64(1):22–30. doi: 10.1006/obhd.1995.1086
2. Gray JC, MacKillop J. Impulsive delayed reward discounting as a genetically-influenced target for drug abuse prevention: a critical evaluation. Front Psychol (2015) 6:1104. doi: 10.3389/fpsyg.2015.01104
3. Amlung M, Petker T, Jackson J, Balodis I, MacKillop J. Steep discounting of delayed monetary and food rewards in obesity: a meta-analysis. psychol Med (2016) 46(11):2423–34. doi: 10.1017/s0033291716000866
4. Cheng Y-S, Ko H-C, Sun C-K, Yeh P-Y. The relationship between delay discounting and Internet addiction: A systematic review and meta-analysis. Addictive Behav (2021) 114:106751. doi: 10.1016/j.addbeh.2020.106751
5. Stea JN, Hodgins DC, Lambert MJ. Relations between delay discounting and low to moderate gambling, cannabis, and alcohol problems among university students. Behav Processes (2011) 88(3):202–5. doi: 10.1016/j.beproc.2011.09.002
6. Bailey AJ, Gerst K, Finn PR. Delay discounting of losses and rewards in alcohol use disorder: The effect of working memory load. Psychol Addictive Behav (2018) 32(2):197–204. doi: 10.1037/adb0000341
7. Peters J, Büchel C. The neural mechanisms of inter-temporal decision-making: understanding variability. Trends Cogn Sci (2011) 15(5):227–39. doi: 10.1016/j.tics.2011.03.002
8. Carter RM, Meyer JR, Huettel SA. Functional neuroimaging of intertemporal choice models: A review. J Neurosci. Psychol. Economics (2010) 3(1):27–45. doi: 10.1037/a0018046
9. Stanger C, Elton A, Ryan SR, James GA, Budney AJ, Kilts CD. Neuroeconomics and adolescent substance abuse: individual differences in neural networks and delay discounting. J Am Acad Child Adolesc Psychiatry (2013) 52(7):747–755.e746. doi: 10.1016/j.jaac.2013.04.013
10. Rosch KS, Mostofsky SH, Nebel MB. ADHD-related sex differences in fronto-subcortical intrinsic functional connectivity and associations with delay discounting. J Neurodev Disord (2018) 10(1):34. doi: 10.1186/s11689-018-9254-9
11. Chen Z, Becker B, Qin P, Lei W, Chen J, Liu P, et al. Neural networks during delay discounting as trans-disease marker: A meta-analytical review. J Psychiatr Res (2021) 139:62–70. doi: 10.1016/j.jpsychires.2021.05.008
12. Yu M, Liu T, Shangguan F, Sui J, Shi J. The neurodevelopment of delay discounting for monetary rewards in pre-adolescent children. Sci Rep (2021) 11(1):8337. doi: 10.1038/s41598-021-87282-z
13. Mazur JE. An adjusting procedure for studying delayed reinforcement. In: The effect of delay and of intervening events on reinforcement value. Hillsdale, NJ, US: Lawrence Erlbaum Associates, Inc (1987). p. 55–73.
14. Rachlin H, Raineri A, Cross D. Subjective probability and delay. J Exp Anal Behav (1991) 55(2):233–44. doi: 10.1901/jeab.1991.55-233
15. Myerson J, Green L. Discounting of delayed rewards: Models of individual choice. J Exp Anal Behav (1995) 64(3):263–76. doi: 10.1901/jeab.1995.64-263
16. Richards JB, Zhang L, Mitchell SH, de Wit H. Delay or probability discounting in a model of impulsive behavior: Effect of alcohol. J Exp Anal Behav (1999) 71(2):121–43. doi: 10.1901/jeab.1999.71-121
17. Kirby KN, Petry NM, Bickel WK. Heroin addicts have higher discount rates for delayed rewards than non-drug-using controls. J Exp Psychol Gen (1999) 128(1):78–87. doi: 10.1037/0096-3445.128.1.78
18. Shamosh NA, Gray JR. Delay discounting and intelligence: A meta-analysis. Intelligence (2008) 36(4):289–305. doi: 10.1016/j.intell.2007.09.004
19. Shamosh NA, DeYoung CG, Green AE, Reis DL, Johnson MR, Conway ARA, et al. Individual differences in delay discounting. psychol Sci (2008) 19(9):904–11. doi: 10.1111/j.1467-9280.2008.02175.x
20. Bailey AJ, Gerst K, Finn PR. Intelligence moderates the relationship between delay discounting rate and problematic alcohol use. Psychol Addictive Behav (2020) 34(1):175–81. doi: 10.1037/adb0000471
21. Fox MD, Snyder AZ, Vincent JL, Corbetta M, Van Essen DC, Raichle ME. The human brain is intrinsically organized into dynamic, anticorrelated functional networks. Proc Natl Acad Sci (2005) 102(27):9673–8. doi: 10.1073/pnas.0504136102
22. Biswal BB, Mennes M, Zuo X-N, Gohel S, Kelly C, Smith SM, et al. Toward discovery science of human brain function. Proc Natl Acad Sci (2010) 107(10):4734–9. doi: 10.1073/pnas.0911855107
23. Kong XZ, Wang X, Pu Y, Huang L, Hao X, Zhen Z, et al. Human navigation network: the intrinsic functional organization and behavioral relevance. Brain Structure Funct (2016) 222(2):749–64. doi: 10.1007/s00429-016-1243-8
24. Jenkinson M, Smith S. A global optimization method for robust affine registration of brain images. Med Image Anal (2001) 5(2):143–56. doi: 10.1016/S1361-8415(01)00036-6
25. Jenkinson M, Bannister P, Brady M, Smith S. Improved optimization for the robust and accurate linear registration and motion correction of brain images. NeuroImage (2002) 17(2):825–41. doi: 10.1006/nimg.2002.1132
26. Biswal B, Yetkin FZ, Haughton VM, Hyde JS. Functional connectivity in the motor cortex of resting human brain using echo-planar MRI. Magnetic Resonance Med (1995) 34(4):537–41. doi: 10.1002/mrm.1910340409
27. Power JD, Mitra A, Laumann TO, Snyder AZ, Schlaggar BL, Petersen SE. Methods to detect, characterize, and remove motion artifact in resting state fMRI. Neuroimage (2014) 84:320–41. doi: 10.1016/j.neuroimage.2013.08.048
28. Marcoulides GA, Hershberger SL. Multivariate Statistical Methods: A First Course. Hillsdale: Lawrence Erlbaum Associates, Inc (1997).
29. Zang Y, Jiang T, Lu Y, He Y, Tian L. Regional homogeneity approach to fMRI data analysis. NeuroImage (2004) 22(1):394–400. doi: 10.1016/j.neuroimage.2003.12.030
30. Agarwal S, Sair HI, Pillai JJ. The resting-state functional magnetic resonance imaging regional homogeneity metrics—Kendall's coefficient of concordance-regional homogeneity and coherence-regional homogeneity—Are valid indicators of tumor-related neurovascular uncoupling. Brain Connectivity (2017) 7(4):228–35. doi: 10.1089/brain.2016.0482
32. Yan CG, Wang XD, Zuo XN, Zang YF. DPABI: data processing & Analysis for (Resting-state) brain imaging. Neuroinformatics (2016) 14(3):339–51. doi: 10.1007/s12021-016-9299-4
33. Holmes C, Owens M, Beach SRH, McCormick M, Hallowell E, Clark US, et al. Peer influence, Frontostriatal connectivity, and delay discounting in African American emerging adults. Brain Imaging Behav (2020) 14(1):155–63. doi: 10.1007/s11682-018-9977-y
34. Colom R, Stein JL, Rajagopalan P, Martínez K, Hermel D, Wang Y, et al. Hippocampal structure and human cognition: Key role of spatial processing and evidence supporting the efficiency hypothesis in females. Intelligence (2013) 41(2):129–40. doi: 10.1016/j.intell.2013.01.002
35. Zhu B, Chen C, Dang X, Dong Q, Lin C. Hippocampal subfields' volumes are more relevant to fluid intelligence than verbal working memory. Intelligence (2017) 61:169–75. doi: 10.1016/j.intell.2017.02.003
36. Cox SR, Ritchie SJ, Fawns-Ritchie C, Tucker-Drob EM, Deary IJ. Structural brain imaging correlates of general intelligence in UK Biobank. Intelligence (2019) 76:101376. doi: 10.1016/j.intell.2019.101376
37. Saha S, Pagnozzi A, Bradford D, Fripp J. Predicting fluid intelligence in adolescence from structural MRI with deep learning methods. Intelligence (2021) 88:101568. doi: 10.1016/j.intell.2021.101568
38. Takeuchi H, Taki Y, Hashizume H, Sassa Y, Nagase T, Nouchi R, et al. Cerebral blood flow during rest associates with general intelligence and creativity. PloS One (2011) 6(9):e25532. doi: 10.1371/journal.pone.0025532
39. Xia M, Wang J, He Y. BrainNet Viewer: a network visualization tool for human brain connectomics. PloS One (2013) 8(7):e68910. doi: 10.1371/journal.pone.0068910
40. Wang S, Zhou M, Chen T, Yang X, Chen G, Gong Q. Delay discounting is associated with the fractional amplitude of low-frequency fluctuations and resting-state functional connectivity in late adolescence. Sci Rep (2017) 7(1):10276. doi: 10.1038/s41598-017-11109-z
41. Lv C, Qiang W, Chen C, Qiu J, Xue G, He Q. The regional homogeneity patterns of the dorsal medial prefrontal cortex predict individual differences in decision impulsivity. NeuroImage (2019) 200:556–61. doi: 10.1016/j.neuroimage.2019.07.015
42. Koban L, Lee S, Schelski DS, Simon M-C, Lerman C, Weber B, et al. An fMRI-based brain marker of individual differences in delay discounting. J Neurosci (2023) 43(9):1600–13. doi: 10.1523/JNEUROSCI.1343-22.2022
43. Mehta K, Pines A, Adebimpe A, Larsen B, Bassett DS, Calkins ME, et al. Individual differences in delay discounting are associated with dorsal prefrontal cortex connectivity in children, adolescents, and adults. Dev Cogn Neurosci (2023) 62:101265. doi: 10.1016/j.dcn.2023.101265
44. Bartra O, McGuire JT, Kable JW. The valuation system: A coordinate-based meta-analysis of BOLD fMRI experiments examining neural correlates of subjective value. NeuroImage (2013) 76:412–27. doi: 10.1016/j.neuroimage.2013.02.063
45. Engelmann JB, Berns GS, Dunlop BW. Hyper-responsivity to losses in the anterior insula during economic choice scales with depression severity. psychol Med (2017) 47(16):2879–91. doi: 10.1017/s0033291717001428
46. Prévost C, Pessiglione M, Météreau E, Cléry-Melin M-L, Dreher J-C. Separate valuation subsystems for delay and effort decision costs. J Neurosci (2010) 30(42):14080–90. doi: 10.1523/jneurosci.2752-10.2010
47. Vestergaard MD, Schultz W. Retrospective valuation of experienced outcome encoded in distinct reward representations in the anterior insula and amygdala. J Neurosci (2020) 40(46):8938–50. doi: 10.1523/jneurosci.2130-19.2020
48. Peters J, Büchel C. Overlapping and distinct neural systems code for subjective value during intertemporal and risky decision making. J Neurosci (2009) 29(50):15727–34. doi: 10.1523/jneurosci.3489-09.2009
49. Liu L, Feng T. The neural predictors of choice preference in intertemporal choice. Brain Res (2012) 1436:92–100. doi: 10.1016/j.brainres.2011.12.018
50. Massar SAA, Libedinsky C, Weiyan C, Huettel SA, Chee MWL. Separate and overlapping brain areas encode subjective value during delay and effort discounting. NeuroImage (2015) 120:104–13. doi: 10.1016/j.neuroimage.2015.06.080
51. Lopez-Guzman S, Konova AB, Glimcher PW. Computational psychiatry of impulsivity and risk: how risk and time preferences interact in health and disease. Philos Trans R Soc B: Biol Sci (2018) 374(1766):20180135. doi: 10.1098/rstb.2018.0135
52. Wang Q, Wang Y, Wang P, Peng M, Zhang M, Zhu Y, et al. Neural representations of the amount and the delay time of reward in intertemporal decision making. Hum Brain Mapp (2021) 42(11):3450–69. doi: 10.1002/hbm.25445
53. Benoit RG, Gilbert SJ, Burgess PW. A neural mechanism mediating the impact of episodic prospection on farsighted decisions. J Neurosci (2011) 31(18):6771–9. doi: 10.1523/JNEUROSCI.6559-10.2011
54. Race E, Keane MM, Verfaellie M. Losing sight of the future: Impaired semantic prospection following medial temporal lobe lesions. Hippocampus (2013) 23(4):268–77. doi: 10.1002/hipo.22084
55. van den Bos W, McClure SM. Towards a general model of temporal discounting. J Exp Anal Behav (2013) 99(1):58–73. doi: 10.1002/jeab.6
56. Loganathan K, Ho ETW. Value, drug addiction and the brain. Addictive Behav (2021) 116:106816. doi: 10.1016/j.addbeh.2021.106816
57. Greicius MD, Krasnow B, Boyett-Anderson JM, Eliez S, Schatzberg AF, Reiss AL, et al. Regional analysis of hippocampal activation during memory encoding and retrieval: fMRI study. Hippocampus (2003) 13(1):164–74. doi: 10.1002/hipo.10064
58. Eldridge LL, Engel SA, Zeineh MM, Bookheimer SY, Knowlton BJ. A dissociation of encoding and retrieval processes in the human hippocampus. J Neurosci (2005) 25(13):3280–6. doi: 10.1523/JNEUROSCI.3420-04.2005
59. Li N, Ma N, Liu Y, He XS, Sun DL, Fu XM, et al. Resting-state functional connectivity predicts impulsivity in economic decision-making. J Neurosci (2013) 33(11):4886–95. doi: 10.1523/jneurosci.1342-12.2013
60. Guo Y, Feng T. The mediating role of LPFC–vmPFC functional connectivity in the relation between regulatory mode and delay discounting. Behav Brain Res (2015) 292:252–8. doi: 10.1016/j.bbr.2015.06.035
61. Xin Y, Xu P, Aleman A, Luo Y, Feng T. Intrinsic prefrontal organization underlies associations between achievement motivation and delay discounting. Brain Structure Funct (2020) 225(2):511–8. doi: 10.1007/s00429-019-01982-x
62. Broulidakis MJ, Golm D, Cortese S, Fairchild G, Sonuga-Barke E. Default mode network connectivity and attention-deficit/hyperactivity disorder in adolescence: Associations with delay aversion and temporal discounting, but not mind wandering. Int J Psychophysiol (2022) 173:38–44. doi: 10.1016/j.ijpsycho.2022.01.007
63. Xiao Z, Chen Z, Chen W, Gao W, He L, Wang Q, et al. Maladaptive changes in delay discounting in males during the COVID-19 pandemic: the predictive role of functional connectome. Cereb Cortex (2022) 32(20):4605–18. doi: 10.1093/cercor/bhab505
64. Wang JH, Zuo XN, Gohel S, Milham MP, Biswal BB. Graph theoretical analysis of functional brain networks: test-retest evaluation on short- and long-term resting-state functional MRI data. PloS One (2011) 6(7):e68910. doi: 10.1371/journal.pone.0021976
65. Chodkowski BA, Cowan RL, Niswender KD. Imbalance in resting state functional connectivity is associated with eating behaviors and adiposity in children. Heliyon (2016) 2(1):e0058. doi: 10.1016/j.heliyon.2015.e00058
66. Holmes CJ, Barton AW, MacKillop J, Galván A, Owens MM, McCormick MJ, et al. Parenting and salience network connectivity among African Americans: A protective pathway for health-risk behaviors. Biol Psychiatry (2018) 84(5):365–71. doi: 10.1016/j.biopsych.2018.03.003
67. Jung WH, Lee TY, Kim M, Lee J, Oh S, Lho SK, et al. Sex differences in the behavioral inhibition system and ventromedial prefrontal cortex connectivity. Soc Cogn Affect Neurosci (2022) 17(6):571–8. doi: 10.1093/scan/nsab118
68. de Wit H, Flory JD, Acheson A, McCloskey M, Manuck SB. IQ and nonplanning impulsivity are independently associated with delay discounting in middle-aged adults. Pers Individ Dif (2007) 42(1):111–21. doi: 10.1016/j.paid.2006.06.026
69. O'Brien L, Albert D, Chein J, Steinberg L. Adolescents prefer more immediate rewards when in the presence of their peers. J Res Adolescence (2011) 21(4):747–53. doi: 10.1111/j.1532-7795.2011.00738.x
70. Ishii K. Subjective socioeconomic status and cigarette smoking interact to delay discounting. Springerplus (2015) 4:1–5. doi: 10.1186/s40064-015-1361-4
71. Ishii K, Eisen C, Hitokoto H. The effects of social status and culture on delay discounting. Japanese psychol Res (2017) 59(3):230–7. doi: 10.1111/jpr.12154
72. Stoeckel LE, Murdaugh DL, Cox JE, Iii EWC, Weller RE. Greater impulsivity is associated with decreased brain activation in obese women during a delay discounting task. Brain Imaging Behav (2013) 7:116–28. doi: 10.1007/s11682-012-9201-4
Keywords: delay discounting, ReHo, functional connectivity, the tripartite network model, resting-state fMRI
Citation: Ji S, Yang F and Li X (2024) Spontaneous neural activity in the three principal networks underlying delay discounting: a resting-state fMRI study. Front. Psychiatry 15:1320830. doi: 10.3389/fpsyt.2024.1320830
Received: 16 October 2023; Accepted: 17 January 2024;
Published: 02 February 2024.
Edited by:
Yosuke Morishima, University of Bern, SwitzerlandReviewed by:
Luke Norman, National Institute of Mental Health (NIH), United StatesTakuya Ishida, The University of Tokyo, Japan
Copyright © 2024 Ji, Yang and Li. This is an open-access article distributed under the terms of the Creative Commons Attribution License (CC BY). The use, distribution or reproduction in other forums is permitted, provided the original author(s) and the copyright owner(s) are credited and that the original publication in this journal is cited, in accordance with accepted academic practice. No use, distribution or reproduction is permitted which does not comply with these terms.
*Correspondence: Xueting Li, li.xueting@ruc.edu.cn