- 1Psychology Department, Faculty of Medicine, Universidad Alfonso X El Sabio, Madrid, Spain
- 2School for Doctoral Studies and Research in Biomedicine, Universidad Europea de Madrid, Faculty of Biomedical and Health Sciences, Madrid, Spain
- 3Department of Nursing, Human Nutrition and Dietetics, Universidad Europea de Madrid, Madrid, Spain
- 4Program in Biomedicine, Translational Research and New Health Technologies, School of Medicine, University of Malaga, Malaga, Spain
- 5Psychology Department, Faculty of Medicine, Alfonso X el Sabio University, Madrid, Spain
- 6Faculty of Health Sciences, Universidad Alfonso X el Sabio, Madrid, Spain
Schizophrenia is a persistent mental health condition that, while presenting challenges, underscores the dynamic nature of cognitive functions and encourages a unique perspective on how individuals engage with their surroundings. Social networks, as a means of communication of great importance at the present time, are for this type of people a way of interacting with their environment with a high level of security. The aim is to find out how schizophrenia is dealt with in different social networks and to differentiate between different types of articles dealing with the use of Facebook, X (former Twitter), YouTube, TikTok, Instagram, and Weibo. A total of 45 articles to i) Social networks used, ii) Country of analyzed users, iii) age of the users analyzed, iv) focus of the analyzed manuscript (mental health literacy, stigmatization, detection of patterns associated with schizophrenia, and Harmful substance use). It was observed that 45.45% of the studies analyzed were conducted in the USA population, followed by UK and China (13.64%). The most analyzed social networks were those based on audiovisual communication (60%). Furthermore, the two main foci addressed in these articles were: stigmatization of schizophrenia with 16 articles (35.55%), following by the prediction of schizophrenia-detecting patterns with 15 articles (33.33%) and the use of social networks to stigmatize people with schizophrenia (38%) and only 14 articles (31.11%) were focused on mental health literacy. Likewise, it was found that there is great potential in the use of the analysis of the content generated, as possible predictors of the presence of this disease, which would allow rapid detection and intervention for psychosis and schizophrenia.
1 Introduction
Nowadays, social media is considered a powerful tool to obtain information. This role allows them to be seen as a way for many citizens to obtain health-related information (1). So, easy access to social networks allows people to actively engage in the communication process and stay connected with each other (2). This makes a source of social influence, with the ability to help people express opinions on topics of interest, but to change attitudes and perceptions about situations (3) related to education or health among others (4, 5).
Schizophrenia is considered as a persistent mental health condition, characterized by shifts in perception, self-awareness, and behavior (6). People with schizophrenia may encounter challenges in social interactions or leading an independent life (7). However, it is crucial to note that interventions aimed at holistic recovery, which address cognitive and social aspects rather than just symptom reduction (7), enable people with schizophrenia to actively participate and integrate into social life.
As a result, people often face widespread social stigma related to their condition, which makes them hesitant to seek help from healthcare professionals for fear of being labeled as mentally ill (8, 9). In this context, the combination of social prejudice and the T anonymity offered by online platforms has led to the formation of support communities such as online community (10). An example of this has been described during the COVID-19 pandemic, and the ensuing lockdown, where some patients perceived the lockdown positively, as it improved their relationship with staff, fostered a greater sense of community and emphasized self-awareness and treatment (11). However, pandemic-induced stress and negative affect, as well as isolation, were associated with an increased risk of psychotic symptoms (12).
It is important to note that social networks can play a critical role as a resource for health-related information when needed (13, 14). Given this potential, it is important to take advantage of social networks to identify these individuals and offer them support (15, 16).
Nowadays some social networks are playing an increasingly important role in the search for health information (14, 17), especially among young (18) and young adults (18-24 years of age), who use them very frequently and spend considerable time in platforms such as Instagram, YouTube, Twitter or TikTok (18–20).
However, the main drawback commonly described for health information disseminated through social networks is the lack of quality and reliability (20, 21). Individuals may be vulnerable to unreliable content and may not have adequate tools to successfully filter reliable information from untrue content (4). In terms of mental health, another perspective should also be considered: individuals’ exposure on social networks can lead to social contagion, or peer pressure, to conform to certain behaviors or actions (21–23). Not to mention that some statements on social networks may promote rejection towards people with mental illnesses, as may be the case with people diagnosed with schizophrenia (23, 24).
Beyond the negative effects associated with information disseminated through social networks, we should highlight the potential use of these platforms as channels to promote mental health care for people with disorders such as schizophrenia. Social networks, serves as a way of communication for people, through participation in online networking that helps provide emotional support and a sense of belonging, as well as the opportunity to share experiences with people facing similar challenges (16). Social networks, also serve as outlets to provide reliable information generated by mental health professionals (14, 24), or to disseminate information to raise awareness and reduce the stigma associated with mental illness (14, 25).
To the best of the researchers’ knowledge, there is no previous review that has analyzed how users of different social networks, whether text-based, such as Twitter or Weibo, or audio-visual, such as Youtube, TikTok or Instagram, deal with schizophrenia. Whether they are people who suffer from it, family members or other users. We believe that this review will help to better understand how schizophrenia is addressed in different social networks.
The present research was three main aims, were: i) analysis the existing international literature on schizophrenia and social networks, ii) to analyze how schizophrenia is handled by users from different social networks. assessing information on the countries where the studies took place, the age of the people included in the studies, or whether these articles were associated with stigmatization or other approaches to schizophrenia, and iii) Comparing whether the COVID-19 lockdown is reflected in any way in the documents published during that period.
2 Materials and methods
2.1 Search methodology
To identify studies of interest, a bibliographic search was conducted covering a wide range of published health-related research from the following databases: Medline, Web of Science and Scopus. The time frame of the study included articles published from January 1, 2017, to December 13, 2022.
The search terms used in the different databases were focused on obtaining the appropriate result to answer the objectives set out in this study, these are as follows: terms used for schizophrenia included “schizophrenia*” OR “psychosis”, On the other hand, search terms related to social networks included: “social networks” OR “Facebook” OR “Twitter” OR “Instagram” OR “Weibo” OR “TikTok” OR “YouTube”. In relation to social networks, and more specifically about Twitter. At the time of the search this social network kept this name since the denomination of X was given by Elon Musk in July 2023.
An example of the search syntax used in SCOPUS search engine was as follows: (schizophrenia OR psychotic disorders OR psychotic) AND (social networks OR Facebook OR Twitter OR Instagram OR Weibo OR TikTok OR Youtube)
The following criteria were defined to retrieve eligible studies for this review. Inclusion criteria were defined as: i) articles written in English, ii) articles published in a peer-reviewed journal, iii) articles that clearly stated the methodology and results, iv) articles in which the researchers used Facebook, Twitter, Instagram, Weibo, TikTok or YouTube to obtain results, v) articles published the last 6 years, between January 1, 2017 and December 31, 2022. One of the aims of the present study was to observe whether the blockade triggered by the COVID-19 pandemic had any effect on the use of social networks associated with schizophrenia. To this end, given that the blockade was declared in many countries in the year 2020, from January to April (26), we aimed to approach the study from 3 years before the first blockade to 3 years after.
Exclusion criteria were defined as i) non-research articles (editorials, commentaries, preprints, abstracts, proceedings, book reviews, reviews, etc.), ii) articles focusing on psychiatric disorders other than schizophrenia, iii) articles focusing on social networks not included in the inclusion criteria.
2.2 Data collection
After retrieving the studies from the databases, duplicate reports were removed, and the titles and abstracts of the remaining articles were screened to exclude studies that did not meet the eligibility criteria. To avoid error and bias, three independent researchers conducted the review process to identify articles that met the inclusion criteria, using the Zotero bibliographic reference manager, which allows for the detection and elimination of duplicate articles (27). Titles and abstracts were then analyzed to exclude irrelevant articles. Finally, the full texts were evaluated using PRISMA criteria (28), to determine whether the articles met the eligibility criteria. During this selection phase, any disagreements among the investigators were resolved by discussion and consultation with a reviewer who was not actively involved in the study selection. The quality assessment for each studied was assessed using the tool of the National Heart, Lung, and Blood Institute (NIH) (https://www.nhlbi.nih.gov/health-topics/study-quality-assessment-tools) for cross-sectional studies that included 14 criteria (29). Two independent researchers assessed each article. We rated the studies as ‘Good’ if the count was ≥11, ‘Fair’ if the count was between 5 and 10, and ‘Poor’ if the count was ≤4. The studies generally had a fair quality (moderate risk of bias). The reliability of this analysis was evaluated using an analysis to assess the correlation between valuations, the Cohen’s kappa coefficient (0.72), which implies a good correlation between the valuations.
2.3 Data analysis
After selecting the relevant articles, the data were compiled in a Microsoft Excel spreadsheet. Due to the heterogeneity of the studies included in the present analysis, a narrative synthesis was performed according to context analysis associated with schizophrenia. We grouped the manuscript into the following categories: i) Social networks, where the type of social network used in the studies was detailed. ii)Nationality of users analyzed in the manuscripts. iii) Age of the users analyzed in the manuscript. iv) Main focus of the analyzed manuscript,
Within the fourth category, the focus revised were: i) Mental Health Literacy, focusing on the use of social networks as, use for schizophrenia education, ii) Prediction/detection of patterns associated with schizophrenia, iii) Addictions. iv) Stigmatization & Trivialization of schizophrenia, Stigmatization, is understood as the exposure of how social networks can be used to disseminate biased information about schizophrenia and negatively target schizophrenia patients. Trivialization is understood as considering the disease as unimportant and downplaying its implications for those who suffer from it.
Finally, for data analysis, descriptive and inferential statistics were used via the Jamovi (Version 2.3.11, The Jamovi Project, Sydney, Australia). The categorical variables included in the present study and derived from the subcategories defined in the qualitative approach, have been expressed as the total number of individuals and proportions. Furthermore, the comparison between groups was performed with a non-parametric test, the chi-square test, since this test does not require homoscedasticity in the data and permits the evaluation of dichotomous independent variables. The statistical level of significance was set at p < 0.05.
3 Results
3.1 Selection of evidence sources
The search strategy yielded 184 articles. After eliminating duplicates, a total of 89 articles were screened for eligibility criteria (Figure 1). Of these, 44 articles were eliminated due to lack of assessment, leaving 45 articles for full-text analysis (Table 1).
Of the articles removed, the 29 that were initially withdrawn were because they met one of the exclusion criteria, such as being a book chapter. The remaining 15 articles were removed after an in-depth review when it was found that the articles did not focus on schizophrenia but on psychosis, without specifying their association with schizophrenia or dealing with social networks other than those asked, such as reddit, or using mobile phone messaging applications.
3.2 Social networks related to schizophrenia
When analyzing the social networks in this study, it was found that the most frequent social network was Twitter, appearing in 54.17% of the documents (n=26). It was followed by Facebook, present in 37.5% (n=18) of the documents. Youtube appeared in 20.83% (n=10), followed by Instagram which appeared in 8.33% of the documents (n=4).
Finally, Weibo, another text-only social network exclusively accessible in China, appeared in 2.08% of the documents (n=1). It is noteworthy that the social network Tiktok, at the time of this review, will not have any documents.
Furthermore, when the number of social networks was analyzed, we observed that several studies, 87.5% (n=42) focused on only one social network, while the 12.5% (n=6) focused on two or more social networks (Table 1). Finally, in relation to the typology of social networks, it was found that 77.08% (n=37) of the documents analyzed social networks focused on the use of text. This compares to 16.67% (n=8) that analyzed audiovisual social networks. The remaining 6.25% (n=3) analyzed social networks of both types (Table 1).
It is striking that TikTok, the social network currently booming worldwide, is not being used to share information related to schizophrenia. The most relevant platforms for this topic are Twitter, Instagram or YouTube, platforms that focus on sharing videos or audiovisual content. Therefore, we can say that this is a central aspect when it comes to disseminating information to the population.
3.3 Geolocation and age of studies, about schizophrenia, included
Regarding the geographical location of the studies, it was found that 56% (n=28) did not provide this category of information, 40% (n=20 was targeted at users coming from a single country, and, finally, the 4% (n=2) analyzed users from different countries.
Of the 22 countries that provided the countries of origin of the users analyzed, the country that appeared most frequently was the USA, which appeared in 45.45% (n=10) of the manuscripts. This was followed by China (n=3) and United Kingdom (n=3) with 13.64%. Finally, Australia, Canada, France, Indonesia, Japan, Norway, Singapore, and Turkey appeared in only one study (see Figure 2).
About age, only 13 (27.08%) of the 48 studies included provided information on the age of the users analyzed. However, it is important to note that the age range of users was observed to be very disparate between the different studies, making comparison between studies complexes. It is worth noting that, about the adolescent population, there are 4 studies that indicate that they analyzed populations aged 13, 15, and 16 years and older. Thus, of those studies that do analyze age, only 4 studies (30.77% of total studies that provide the participants’ age) indicated that they analyzed users who indicated an age of less than 18 years, but in no case did they focus exclusively on children or adolescents (Table 1). Likewise, no study defining age indicated that groups of people aged 65 years or older were analyzed. Finally, 6 studies (46.15%) were found to focus on the group defined as adults aged no more than 35 years. 1 study (7.14%) focused on adults over 34 years old (Table 1).
3.4 Approaches to schizophrenia conversation on social networks
The results are developed below according to the previously defined categories.
When analyzing the category of Mental Health literacy, it was observed that a total of 16 documents focused on addressing the use of social networks (Table 2). It should be noted that 13 social networks that used text were analyzed, compared to 6 articles that used social networks based on audiovisual communication (Table 2).
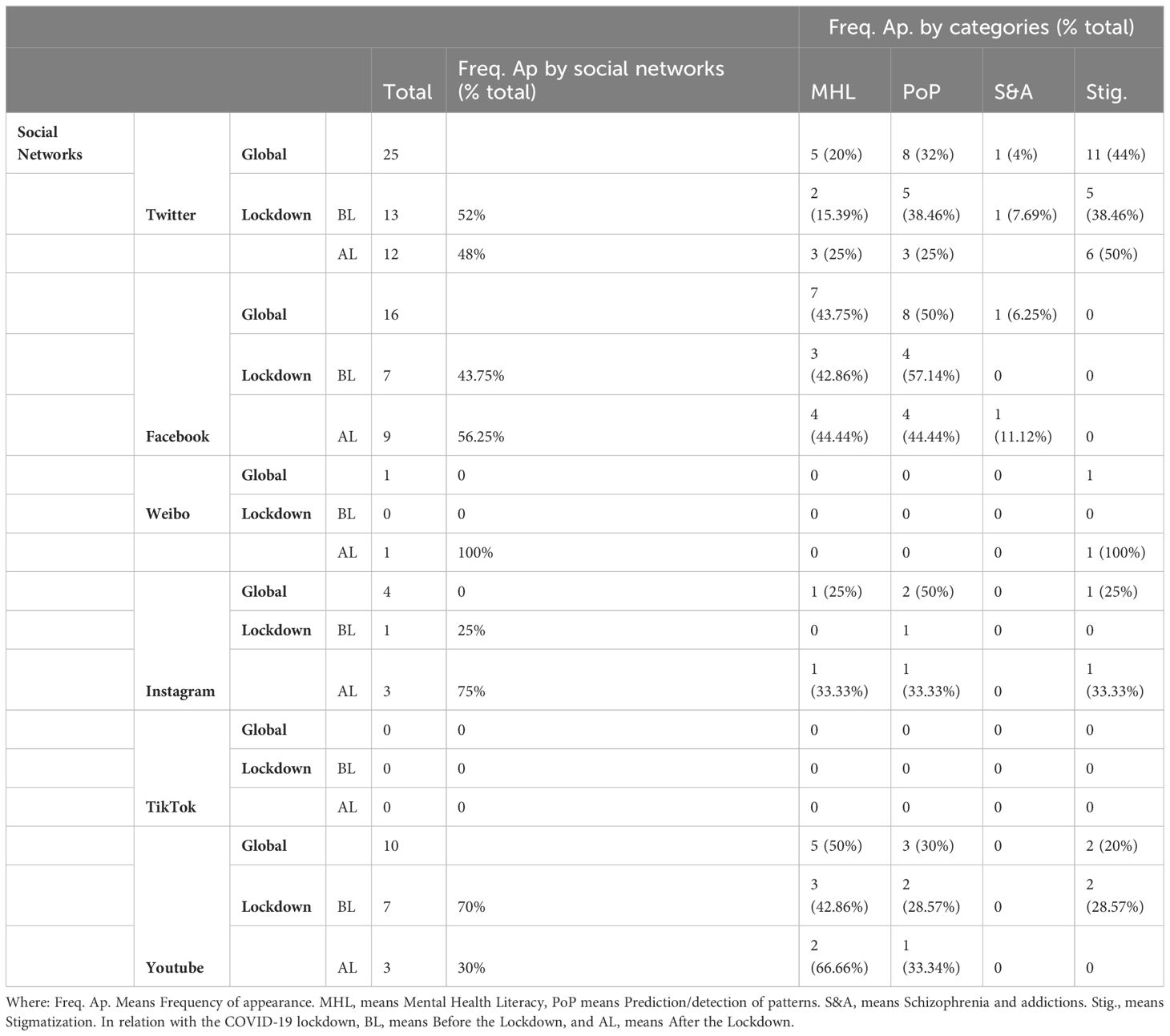
Table 2 Social networks associated with the focus of the manuscripts included in the systematic review.
It is found that authors such as Wong, KTG, et al. (30) who are based on a text-based social network such as Facebook, indicate that people with schizophrenia make little use of social networks, making it difficult to use them to develop a mental health education intervention. This data contrasts with other studies, which do indicate that the use and presence of social networks supports mental health education (52, 54, 58–60, 66, 69).
It Is worth highlighting the study by Delanys, S., et al. (43) in the study they carried out on Twitter, which defined that training on what and how to use medical terms appropriately was the responsibility of healthcare professionals.
Finally, the work of Retnowat, Y (61)., focuses on the analysis of the communication and training strategy on mental health focused on schizophrenia, on Facebook.
When assessing the use of audiovisual social networks and their use to develop training activities in mental health, two documents were found that support psychoeducational intervention on the social network Youtube (34, 62, 65, 68). A Likewise, in relation to YouTube, Nour, M.M., et al. (64) analyze the importance of YouTube as a tool for mental health training for future medical professionals. Finally, Lam, N.H.T. & Woo, B.K.P. (36), demonstrated that the use of psychoeducation advertisements on Instagram had the same or better results than training on Facebook. A total of 15 articles were found to focus on identifying patterns that would allow them to detect schizophrenia through social network messages (Table 1).
Within these documents, could be identified those that focused on the development of machine learning as an analysis strategy to detect patterns that facilitate the identification of people with possible behaviors associated with schizophrenia (27, 28, 37, 38, 53). As Ridout & Campbell (48) suggested, social networks could be used to test whether there is a change in the communication patterns of users depending on their participation in interventions to address schizophrenia, through social networks. In other cases, we even found the detection of possible suicidal behavior in users diagnosed with schizophrenia (57) (Table 1).
It is observed that social networks that use writing as the primary means of communication remain the most important in developing pattern detection algorithms to identify potential schizophrenia patients.
When we analyzed the trivialization and stigmatization continue to be a key element when talking about schizophrenia on social networks since, according to the data (Table 1). Furthermore, 15 documents (37.5%) analyze these concepts with the aim of making them visible and educating the population.
It was observed that the social network where this type of behavior by users occurred most frequently was Twitter, which accounted for 80% of the documents (n=12), followed by Youtube with 6.66% (n=1) as the next most frequently used social network to disseminate stigmatizing information about schizophrenia. Finally, it was found that both Weibo (n=1) and Instagram with 6.67% each (n=1).
When analyzing articles focusing on Twitter, studies were found where machine learning was used to detect Tweets containing inappropriate information about schizophrenia that induced confusion and even stigmatization of people with schizophrenia (28, 38, 39).
Furthermore, it was observed that the most frequent method of categorizing stigmatization, and schizophrenia and its diagnosis, was a categorization done by manuscript’s authors (26, 35, 37, 38, 41, 45, 47, 49). Finally, the assessment of stigmatization was provided by health professionals, who participate in social networks and talk about their experiences in finding information about schizophrenia (44).
The analysis of Youtube (53) and Instagram (21), indicates that comments on videos uploaded about schizophrenia were analyzed. Furthermore, on the social network Weibo, an algorithm was developed that allowed the detection of messages that included pejorative words or that diminished the importance of schizophrenia in posts on that social network (40).
Finally, we found 2 articles that focused on analyzing the role of addictions and their relationship with schizophrenia, specifically both articles pertain to writing-based social networks. Fekih-Romdhane, F., et al. (32) assess, through Facebook, the relationship of people with schizophrenia with the risk of excessive use of technology and social networks and possible addictive behaviors. On the other hand, Hswen, Y., et al. (33) examined the possibility that Twitter users who define themselves as people with schizophrenia were more likely to write and post about smoking and to indicate that they used tobacco.
3.5 Social networks and categorization of articles schizophrenia conversation on social networks
It is important to emphasize that many of these manuscripts were focused on pattern identification on text-based social networks. Twitter and Weibo appear as analyzed social networks in a total of 435 occasions in the documents found, compared to the use of more audiovisual social networks, such as Instagram, Youtube or TikTok, which appear only in 14 documents.
With respect to text-based social networks, the most used is Twitter, in 25 documents, followed by Facebook with 16. Both are widely used for pattern prediction due to the ease of training models for text recognition, approach observed in 7 documents dealing with Facebook (43.75% of the analyzed documents), and Twitter with a total of 5 documents (20% of the total number of documents dealing with Twitter) (Table 2). Finally, in both types of networks, Twitter and Facebook are the only ones that address the topic of schizophrenia and addictions (Table 2).
It is necessary to highlight the role of Twitter as a social network where many documents focused on the analysis of the stigmatization of schizophrenia are generated (n=11), following by Prediction of patterns with 8 documents (32% of documents that analyze Twitter). Finally, Mental Health Literacy is discussed in 5 documents (20%) that analyze Twitter.
With respect to audiovisual social networks, it is interesting to note that they appear in only 14 documents, of which YouTube is the most frequently addressed with 10 documents, followed by Instagram with 4. It is striking that there is no document that addresses the analysis of TikTok (Table 2). The most addressed topic on YouTube is Mental Health Literacy, with 5 documents (50% of the papers addressing YouTube). Pattern analysis for the prediction of behaviors associated with schizophrenia, is observed in both YouTube (n=3; 30%), and Instagram (n=2; 50%). With respect to Stigmatization of schizophrenia, it is YouTube (n=2; 20%) the network that is most focused on this action (Table 2).
When comparing the possible effect of the lockdown on the publication of the number of articles, as well as their subject matter, it is observed that 24 articles (53.33%) were published before the lockdown compared to 21 published afterwards (46.67%).
Comparison of the different categories published between the two periods (Table 2) shows that there is no significant difference between the two periods (X2 = 2.69; p=0.442).
4 Discussion
The purpose of this manuscript is to provide an overview of the current literature on social networks and schizophrenia. To this end, we have organized the following section according to the most frequently mentioned categories in the previous review.
4.1 Influence of lockdown
It was observed that, although the total number of documents increased in the period after the lockdown, this increase was not significant in terms of the subject matter addressed.
Although there may have been an increase in the study of topics associated with stigmatization (12) or mental health education (11, 12), there was no significant increase between the two periods. It can be deduced that the interest in analyzing the role of social networks in the approach to schizophrenia remained the same.
4.2 Detection of schizophrenia patterns on social networks
The analysis of words, or word combinations, represents an advance in the diagnosis and prediction of possible states of schizophrenia (7–9). Potential therapeutic benefits in its diagnosis have been observed in the social network Twitter. This tool has used a combination of clinical assessment and machine learning, finding significant linguistic differences between people who reported having schizophrenia (14, 30, 38, 43). Healthcare professionals, such as physicians, evaluated the Twitter users’ posts to determine the authenticity of the diagnosis.
In addition, an increased use of first-person pronouns, but also of second-person pronouns, was found in these social network’s texts, which may be indicative of changes in the way individuals think about themselves in relation to others and may be experiencing a worsening of psychotic symptoms (30, 32).
Other studies show that patients with schizophrenia posted images on Instagram with significantly lower saturation, coloration, and number of faces-that is, the images tended to be darker- (14, 61). When assessing the characteristics of the images that were shared on social networks by people with schizophrenia, it was found that: i) when it came to selecting colors, red and black were most frequent, but also ii) in the images in which people appeared, it was found that the faces were blurred (14). There is also a tendency to include differences in the way images are cropped, affecting height and width. These profiles attract fewer followers, possibly due to these differences in image and color characteristics, making their profiles less attractive to other users (14).
It is important to highlight that, beyond changes at the linguistic level, the social networks activity provides digital representations of clinically significant behavioral patterns, potentially associated with psychotic disorders and incipient relapse. Significant increases in tagging behavior and the number of friendships in the month prior to hospitalization for relapse, as well as increased posting activity after midnight and in the early morning, have been identified as signs of inappropriate and disorganized social behavior, often seen when the state of individuals with psychosis worsen (31).
Hswen Y et al (38), linked health behavior such as smoking among social networks users to schizophrenia. Twitter users who identified themselves with schizophrenia spectrum disorder tweeted about smoking more frequently than randomly selected Twitter users from the general population.
Although most of the studies use text-based social networks, it is important to note that audiovisual social networks are those that, to date, have a higher frequency of use (19). Because of this, the need to better understand how these contents are used for the detection of patterns that allow the detection of possible behaviors associated with schizophrenia is essential. In many cases these analyses are based on content analysis of the videos by mental health professionals (65). Likewise, in social networks such as YouTube, it is possible to perform analyses of the interactions of the people who view the videos with respect to them. This allows the introduction of the machine learning process on word analysis (60)
Therefore, analysis of word choice on social networks could help clinicians identify individuals at high risk for schizophrenia before the onset of clinically significant symptoms. Understanding linguistic differences and incremental changes in social networks could create important opportunities for intervention (43, 60). However, although all these data provide clues to possible behavioral changes in patients with schizophrenia, to date it is not clear exactly how social behaviors are manifested through social networks (32, 70).
Newer technologies, such as artificial intelligence and machine learning, will play a central role in future prevention strategies, and finding the right balance between security and ethics will be a major challenge (14, 32, 43). While social networks alone cannot diagnose psychiatric disorders, nor replace the fundamental role of a healthcare professional in psychiatric assessment, they can potentially be used in conjunction with physician information to support clinical decision making (3).
4.3 Schizophrenia stigma
The stigmatization of schizophrenia is an issue that we find to be addressed more frequently. This disease is constantly inappropriately mentioned because it is often stigmatized and trivialized (24, 33, 42–44, 46, 49–53) (Not only do patients suffer from psychosis (40), but antipsychotic treatments (42) are also the subject of negative sentiments and judgments by Twitter users (46, 49–53), further demonstrating the persistence of social stigma toward schizophrenia and its treatment (42).
The use of word analysis and machine learning application to detect conversation that use words, adjectives in example, associated to a negative conception of schizophrenia or psychosis. On conversations on social networks (5, 39), analysis methods are also employed to facilitate the automatic detection of stigma toward schizophrenia on social networks (33, 44). The traditional media, as newspapers or television, are a central point for stigmatization of mental health conditions (52), with social networks being a new therapeutic tool to support the disclosure of stigmatized conditions.
According to Li et al (45), 26.22% of relevant Weibo postings showed stigmatizing attitudes towards schizophrenia. Jilka et al (43), also showed that almost half of the public tweets related to schizophrenia in their study were classified as stigmatizing. Alvarez-Mon et al (44), found that terms related to psychosis were associated with a significantly higher number of tweets with negative content, just as people with schizophrenia were more often perceived under negative sentiments, such as unpredictable and dangerous (40–43).
Within these negative tweets, three different stereotypes were identified, suggesting that this disorder hinders the ability to perform some tasks. These are: incoherence, due to the behavioral instability suffered in this disorder; dangerousness, the idea is conveyed that people with the disorder represent a danger to those around them, and relativization, underestimating the suffering of this disorder (43). Regarding the expressions related to the stigma associated with schizophrenia, a greater use of words related to social processes, such as the word “partner,” a more frequent use of the word “kill,” and anger, such as the term “hate”, were noted. Such patterns of language use indicate a higher level of negative sentiment, which may fit into two elements of stigma processes, cognitive dissociation, and negative emotional reactions (40) also describing tweets with negative polarity, such as “Psychiatry breaks people down even more” (43).
These findings confirm the existence of stigma and negative prejudice associated with psychotic disorders. For example, the terms “depression” and “autism” are less likely to be misused than the terms “psychosis” and “psychopath” and “schizophrenia” and “schizophrenic” (43–45). Promoting awareness of the use of words that perpetuate mental health stigma may be beneficial in reducing the stigmatization associated with any mental illness (45, 46).
Education has a positive effect on reducing public stigma towards adults with mental illness. Early identification and intervention in people with psychosis can be achieved through widespread information campaigns and easily accessible services (46, 48).
4.4 Mental health literacy
Studies show that there are many users disseminating beneficial information on social networks, increasing public education about schizophrenia and disease management, but also connecting people through organized events focused on this debilitating disorder (35, 39, 41, 48). It is therefore necessary to integrate therapeutic functions using social networks (18) as an aspect of social functioning in psychosis, allowing for the establishment of a more reliable and valid dimension (54, 55).
Responsible use of Twitter by healthcare professionals will increase the visibility of research findings and ensure that up-to-date evidence is easily accessible (41, 48). As the most widely used social network in public health, Twitter can contribute to increasing schizophrenia literacy and academic information in mental health (59, 60). Facebook can be used to track public health awareness of schizophrenia-related issues (35, 41, 54, 70, 71), but too as a tool to know and educate about the pharmacotherapy in elderly, with mental health disorders (68). YouTube can be an educational medium through videos that appeal to younger generations, who are more vulnerable to certain psychiatric disorders (39, 67, 70). These videos may not only help to reduce the length of time that a mental illness goes untreated but may also help to slowly overcome the stigma associated with these disorders (39, 41, 66). However, they will not be suitable for use as educational tools in isolation, as media portrayals of mental illness strongly influence public understanding and may contribute to the harmful stigma associated with psychiatric diagnoses (66).
Therefore, to effectively engage and inform the public on psychiatry-related topics, adequate knowledge based on scientific evidence must be implemented. Healthcare professionals have a responsibility to post content on social networks that fosters mental health awareness (39, 41, 66, 67, 70).
4.5 The use of social networks by patients with schizophrenia
Many people with schizophrenia are active users of social networks (18, 48), which may have a positive effect on their subjective well-being, as they develop extensive social networks, communicate frequently with other users, and share personal experiences of living with a mental illness and learning from others (51). Acceptance and usability of the reviewed platforms by patients with schizophrenia were generally high, as were perceptions of usefulness and safety (51, 53, 54).
In analyzing social networks, we see that these technologies promote social connectedness, information seeking and provision, creativity, and recreation. However, there is also the possibility of developing problems related to excessive use. People with mental disorders may be more likely to have psychological risk factors for behavioral addictions (37, 38). These include loneliness, depressive symptoms, or lower life satisfaction, as well as demographic risk factors such as unemployment, low educational attainment, and unmarried marital status. However, the potential benefits of social networks use, such as continued cognitive stimulation and community engagement during periods of increased impairment, may outweigh these risks (18, 54, 55) Therefore, social networks may become a psychoeducational tool for patients with schizophrenia (55, 71).
4.6 Limitations and strengths
One of the limitations of this study is that, although we study the influence of social media at the present time, we do not analyze the evolution of the different social networks over time, to gain insight into which ones will be more relevant in the future, and therefore which ones we should pay more attention to as healthcare professionals. An example of this may be the fact that the review was not designed to include from the very beginning the moment when the included social networks were opened to societal participation.
However, a notable strength of the study is that it analyzes the most influential social platforms today, rather than limiting it to just one of them. It also considers multiple diseases, providing a broader view of the current context.
Reviews like this are the first steps that we can take in research to provide a solution to a problem that is becoming increasingly widespread in society.
5 Conclusions
Social networks have transformed our daily lives around the world. As technology enables more cohesive and interconnected social networking, better methods of psychoeducational outreach are being developed. Social networks are gaining predictive value in the first episode of psychosis and in episodes of symptom exacerbation, including suicide prevention. They can help ensure that early manifestations of illnesses such as schizophrenia do not go unnoticed, or that severe symptoms in people living with the illness can be identified and addressed with appropriate treatment and support. It is therefore critical to acknowledge that digital platforms can serve as a complement to traditional approaches to disease detection, treatment, and management.
Our findings have shown that mental health stigma is commonplace on social networks. These channels can be used to develop intervention and information strategies aimed at modifying individual and societal health-related behaviors. There are great opportunities to leverage popular social networks platforms to support the mental health and well-being of people with mental illness. By demonstrating success in reaching people who identify themselves with mental illness on social networks, our study also highlights a potentially novel approach to engaging this at-risk group.
This is an emerging area of research. Efforts are clearly needed to determine the feasibility and effectiveness of mental health programs delivered via social networks, and it is also critical to consider risks to privacy, harm, and overall safety. Effective communication by healthcare professionals in disseminating information on social networks is integral to promoting early intervention for psychosis and schizophrenia.
Author contributions
CS-L: Conceptualization, Data curation, Formal analysis, Writing – original draft. BJ-G: Conceptualization, Formal analysis, Writing – original draft. CR-N: Data curation, Formal analysis, Writing – review & editing. IF-Q: Methodology, Writing – original draft. ER-G: Methodology, Writing – review & editing. CT-D: Visualization, Writing – original draft. IH-P: Conceptualization, Supervision, Writing – review & editing.
Funding
The author(s) declare that no financial support was received for the research, authorship, and/or publication of this article.
Conflict of interest
The authors declare that the research was conducted in the absence of any commercial or financial relationships that could be construed as a potential conflict of interest.
Publisher’s note
All claims expressed in this article are solely those of the authors and do not necessarily represent those of their affiliated organizations, or those of the publisher, the editors and the reviewers. Any product that may be evaluated in this article, or claim that may be made by its manufacturer, is not guaranteed or endorsed by the publisher.
References
1. Hruska J, Maresova P. Use of social networks platforms among adults in the United States-Behaviour on social networks. Societies. (2020) 10:27. doi: 10.3390/soc10010027
2. Rosenberg H, Syed S, Rezaie S. The Twitter pandemic: The critical role of Twitter in the dissemination of medical information and misinformation during the COVID-19 pandemic. CJEM. (2020) 22:418–21. doi: 10.1017/cem.2020.361
3. Saha K, Torous J, Ernala SK, Rizuto C, Stafford A, De Choudhury M. A computational study of mental health awareness campaigns on social networks. Transl Behav Med. (2019) 9:1197–207. doi: 10.1093/tbm/ibz028
4. Conway M. Ethical issues in using twitter for public health surveillance and research: developing a taxonomy of ethical concepts from the research literature. J Med Internet Res. (2014) 16:e290. doi: 10.2196/jmir.3617
5. Biedermann F, Fleischhacker WW. Psychotic disorders in DSM-5 and ICD-11. CNS Spectr. (2016) 21:349–54. doi: 10.1017/S1092852916000316
6. Wölwer W, Frommann N, Loew A, Kamp A, Weide K, Bechdolf A, et al. Efficacy of integrated social cognitive remediation vs neurocognitive remediation in improving functional outcome in schizophrenia: Concept and design of a multicenter, single-blind RCT (The ISST Study). Front Psychiatry. (2022) 13:909370. doi: 10.3389/fpsyt.2022.909370
7. Ben-Zeev D, Young MA, Corrigan PW. DSM-V and the stigma of mental illness. J Ment Health. (2010) 19:318–27. doi: 10.3109/09638237.2010.492484
8. Corrigan PW, Druss BG, Perlick DA. The impact of mental illness stigma on seeking and participating in mental health care. Psychol Sci Public Interest. (2014) 15:37–70. doi: 10.1177/1529100614531398
9. VÄlimäki M, Athanasopoulou C, Lahti M, Adams CE. Effectiveness of social media interventions for people with schizophrenia: A systematic review and meta-analysis. J Med Internet Res. (2016) 18:e92. doi: 10.2196/jmir.5385
10. Barlati S, Nibbio G, Vita A. Schizophrenia during the COVID-19 pandemic. Curr Opin Psychiatry. (2021) 34:203–10. doi: 10.1097/YCO.0000000000000702
11. Mengin AC, Allé MC, Koning E, Pham B, Park S, Berna F, et al. Facing the pandemic and lockdown: an insight on mental health from a longitudinal study using diaries. Schizophr. (2022) 8:22. doi: 10.1038/s41537-022-00222-2
12. Bora K, Das D, Barman B, Borah P. Are internet videos useful sources of information during global public health emergencies? A case study of YouTube videos during the 2015-16 Zika virus pandemic. Pathog Glob Health. (2018) 112:320–8. doi: 10.1080/20477724.2018.1507784
13. Rovetta A, Bhagavathula AS. COVID-19-related web search behaviors and infodemic attitudes in Italy: infodemiological study. JMIR Public Health Surveill. (2020) 6:e19374. doi: 10.2196/19374
14. Jankowski SE, Yanos P, Dixon LB, Amsalem D. Reducing public stigma towards psychosis: A conceptual framework for understanding the effects of social contact based brief video interventions. Schizophr Bull. (2023) 49:99–107. doi: 10.1093/schbul/sbac143
15. Strous RD, Koppel M, Fine J, Nachliel S, Shaked G, Zivotofsky AZ. Automated characterization and identification of schizophrenia in writing. J Nerv Ment Dis. (2009) 197:585–8. doi: 10.1097/NMD.0b013e3181b09068
16. Gao J, Zheng P, Jia Y, Chen H, Mao Y, Chen S, et al. Mental health problems and social medias exposure during COVID-19 outbreak. PloS One. (2020) 15:e0231924. doi: 10.1371/journal.pone.0231924
17. Ridout B, Campbell A. The use of social networking sites in mental health interventions for young people: systematic review. J Med Internet Res. (2018) 20:e12244. doi: 10.2196/12244
18. Turner PG, Lefevre CE. Instagram use is linked to increased symptoms of orthorexia nervosa. Eat Weight Disord - Stud Anorex Bulim Obes. (2017) 22:277–84. doi: 10.1007/s40519-017-0364-2
19. Lee Ventola C. Social media and health care professionals: Benefits, risks, and best practices. P T. (2014) 39:491–520.
20. Segado-Fernández S, Lozano-Estevan MDC, Jiménez-Gómez B, Ruiz-Nuñez C, Jiménez Hidalgo PJ, Fernández-Quijano I, et al. Health literacy and critical lecture as key elements to detect and reply to nutrition misinformation on social media: Analysis among Spanish Healthcare Professionals. Int J Environ Res Public Health. (2023) 20:23. doi: 10.3390/ijerph20010023
21. Astorri E, Clerici G, Gallo G, Raina P, Pelliai A. Online extreme challenges putting children at risk: what we know to date. Minerva Pediatr (Torino.). (2023) 75:98–109. doi: 10.23736/S2724-5276.22.06892-6
22. Martínez V, Jiménez-Molina A, Gerber MM. Social contagion, violence, and suicide among adolescents. CurrOpin Psychiatry. (2023) 6:237–42. doi: 10.1097/YCO.0000000000000858
23. Battaglia A, Mamak M, Goldberg JO. The impact of social media coverage on attitudes towards mental illness and violent offending. J Community Psychol. (2022) 50:2938–49. doi: 10.1002/jcop.22807
24. Fonseka LN, Woo BKP. Social media and schizophrenia: An update on clinical applications. World J Psychiatry. (2022) 1287:897–903. doi: 10.5498/wjp.v12.i7.897
25. Koh D. COVID-19 lockdowns throughout the world. Occup Med. (2020) 70(5):322. doi: 10.1093/occmed/kqaa073
26. Morán JM, Santillán-García A, Herrera-Peco I. SCRUTATIOM: how to detect retracted literature included in systematic reviews and meta-analyses using SCOPUS© and ZOTERO©. Gac Sanit. (2022) 36:64–6. doi: 10.1016/j.gaceta.2020.06.012
27. Moher D, Liberati A, Tetzlaff J, Altman DG. Preferred reporting items for systematic reviews and meta-analyses: the PRISMA statement. Ann Intern Med. (2009) 151:264–9. W64.
28. National heart lung and blood institute. (2013). Available online at: https://www.nhlbi.nih.gov/health-topics/study-quality-assessment-tools.
29. Franco OH, Calkins ME, Giorgi S, Ungar LH, Gur RE, Kohler CG, et al. Feasibility of mobile health and social media -based interventions for young adults with early psychosis and clinical risk for psychosis: survey study. JMIR Form Res. (2022) 6:e30230. doi: 10.2196/30230
30. Birnbaum ML, Ernala SK, Rizvi AF, De Choudhury M, Kane JM. A collaborative approach to identifying social media markers of schizophrenia by employing machine learning and clinical appraisals. J Med Internet Res. (2017) 19:e289. doi: 10.2196/jmir.7956
31. Birnbaum ML, Ernala SK, Rizvi AF, Arenare ER, Van Meter A, De Choudhury M, et al. Detecting relapse in youth with psychotic disorders utilizing patient-generated and patient-contributed digital data from Facebook. NpjSchizophr. (2019) 5:17. doi: 10.1038/s41537-019-0085-9
32. Ashok N, Manoj T, Nandini U. Unique and dynamic approach to predict schizophrenia disease using machine learning. In: Bhoi AK, Mallick PK, Balas VE, Prasad Mishra BS, editors. Advances in systems, control and automations. (2021). p. 479–92. doi: 10.1007/978-981-15-8685-9_50
33. Kelly DL, Spaderna M, Hodzic V, Nair S, Kitchen C, Werkheiser AE, et al. Blinded clinical ratings of social media data are correlated with in-person clinical ratings in participants diagnosed with either depression, schizophrenia, or healthy controls. Psychiatry Res. (2020) 294:113496. doi: 10.1016/j.psychres.2020.113496
34. Wong K,TG, Liu D, Balzan R, King D, Galletly C. Smartphone and internet access and utilization by people with schizophrenia in south Australia: quantitative survey study. JMIR Ment Health. (2020) 7:e11551. doi: 10.2196/11551
35. Birnbaum ML, Norel R, Van Meter A, Ali AF, Arenare E, Eyigoz E, et al. Identifying signals associated with psychiatric illness utilizing language and images posted to Facebook. NPJSchizophr. (2020) 6:38. doi: 10.1038/s41537-020-00125-0
36. Fekih-Romdhane F, Away R, Jahrami H, Cheour M. Internet addiction is associated with psychological distress in highly schizotypal students. Early Interv Psychiatry. (2022) 17(7):681–91. doi: 10.1111/eip.13362
37. Hswen Y, Naslund JA, Chandrashekar P, Siegel R, Brownstein JS, Hawkins JB. Exploring online communication about cigarette smoking among Twitter users who self-identify as having schizophrenia. Psychiatry Res. (2017) 257:479–84. doi: 10.1016/j.psychres.2017.08.002
38. Lustig A, Brookes G, Hunt D. Social semiotics of gangstalking evidence videos on youTube: multimodal discourse analysis of a novel persecutory belief system. JMIR Ment Health. (2021) 8:e30311. doi: 10.2196/30311
39. Passerello GL, Hazelwood JE, Lawrie S. Using Twitter to assess attitudes to schizophrenia and psychosis. BJPsych Bull. (2019) 43:158–66. doi: 10.1192/bjb.2018.115
40. Alvarez-Mon MA, Donat-Vargas C, Santoma-Vilaclara J, Anta L, deGoena J, Sanchez-Bayona R, et al. Efficacy of instagram in promoting psychoeducation in the chinese-speaking population. Health Equity. (2020) 4:114–6. doi: 10.1089/heq.2019.0078
41. Alvarez-Mon MA, Donat-Vargas C, Santoma-Vilaclara J, Anta L deGoena J, Sanchez-Bayona R, et al. Assessment of antipsychotic medications on social media: machine learning study. Front Psychiatry. (2021) 12:737684. doi: 10.3389/fpsyt.2021.737684
42. Jilka S, Odoi CM, Van Bilsen J, Morris D, Erturk S, Cummins N, et al. Identifying schizophrenia stigma on Twitter: a proof of principle model using service user supervised machine learning. Schizophrenia. (2022) 8:1. doi: 10.1038/s41537-021-00197-6
43. Alvarez-Mon MA, Llavero-Valero M, Sánchez-Bayona R, Pereira-Sánchez V, Vallejo-Valdivielso M, Monserrat J, et al. Areas of interest and stigmatic attitudes of the general public in five relevant medical conditions: thematic and quantitative analysis using twitter. J Med Internet Res. (2019) 21:e14110. doi: 10.2196/14110
44. Li A, Jiao D, Liu X, Zhu TA. Comparison of the psycholinguistic styles of schizophrenia-related stigma and depression-related stigma on social media: content analysis. J Med Internet Res. (2020) 22:e16470. doi: 10.2196/16470
45. Kara UY, Şenel, Kara B. Schizophrenia on Turkish Twitter: an exploratory study investigating misuse, stigmatization and trivialization. Soc Psychiatry Psychiatr Epidemiol. (2022) 57:531–9. doi: 10.1007/s00127-021-02112-x
46. Sarioglu E, Diab M, Pauselli L, Compton M, Coppersmith G. Predictive linguistic features of schizophrenia. In: Ide N, Herbelot A, Màrquez L, editors. Proceedings of the 6th Joint Conference on Lexical and Computational Semantics (*SEM 2017). (Vancouver, Canada: Association for computational Linguistics). (2023). p. 241–50.
47. Delanys S, Benamara F, Moriceau V, Olivier F, Mothe J. Psychiatry on twitter: content analysis of the use of psychiatric terms in french. JMIR Form Res. (2022) 6:e18539. doi: 10.2196/18539
48. Jansli SM, Hudson G, Negbenose E, Erturk S, Wykes T, Jilka S. Investigating mental health service user views of stigma on Twitter during COVID-19: a mixed-methods study. J Ment Health. (2022) 31:576–84. doi: 10.1080/09638237.2022.2091763
49. Robinson P, Turk D, Jilka S, Cella M. Measuring attitudes towards mental health using social media: investigating stigma and trivialization. Soc Psychiatry Psychiatr Epidemiol. (2019) 54:51–8. doi: 10.1007/s00127-018-1571-5
50. Naslund JA, Aschbrenner KA. Risks to Privacy With Use of Social networks: Understanding the Views of Social networks Users With Serious Mental Illness. Psychiatr Serv. (2019) 70:561–8. doi: 10.1176/appi.ps.201800520
51. Bowen M, Lovell A. Stigma: the representation of mental health in UK newspaper Twitter feeds. J Ment Health. (2021) 30:424–30. doi: 10.1080/09638237.2019.1608937
52. Ernala SK, Labetoulle T, Bane F, Birnbaum ML, Rizvi AF, Kane JM, et al. (2018). Characterizing audience engagement and assessing its impact on social networks disclosures of mental illnesses, In: Proc Int AAAI Conf Web Soc Media, Vol. 12. doi: 10.1609/icwsm.v12i1.15027
53. Abdel-Baki A, Lal SD, Charron O, Stip E, Kara N. Understanding access and use of technology among youth with first-episode psychosis to inform the development of technology-enabled therapeutic interventions: Use of technology in early psychosis. Early Interv Psychiatry. (2017) 11:72–6. doi: 10.1111/eip.12250
54. Bhoi D, Thakkar A. Impact of sentiment analysis tools to improve patients' life in critical diseases. In: Computational intelligence for machine learning and healthcare informatics. (USA: Wiley Scrivener) (2020). p. 239–52.
55. Colder Carras M, Mojtabai R, Cullen B. Beyond social networks: A cross-sectional survey of other internet and mobile phone applications in a community psychiatry population. J PsychiatrPract. (2018) 24:127–35. doi: 10.1097/PRA.0000000000000297
56. Sangeorzan I, Andriopoulou P, Livanou M. Exploring the experiences of people vlogging about severe mental illness on YouTube: An interpretative phenomenological analysis. J Affect Disord. (2019) 246:422–8. doi: 10.1016/j.jad.2018.12.119
57. Merchant RM, Asch DA, Crutchley P, Ungar LH, Guntuku SC, Eichstaedt JC, et al. Evaluating the predictability of medical conditions from social networks posts. PloS One. (2019) 14:e0215476. doi: 10.1371/journal.pone.0215476
58. Hswen Y, Naslund JA, Brownstein JS, Hawkins JB. Monitoring online discussions about suicide among twitter users with schizophrenia: exploratory study. JMIR Ment Health. (2018) 5:e11483. doi: 10.2196/11483
59. Hernandez MY, Hernandez M, Lopez DH, Gamez D, Lopez SR. What do health providers and patients tweet about schizophrenia? Early Interv Psychiatry. (2020) 14:613–8. doi: 10.1111/eip.12888
60. Rekhi G, Ang MS, Lee J. Clinical determinants of social networks use in individuals with schizophrenia. PloS One. (2019) 14:e0225370. doi: 10.1371/journal.pone.0225370
61. Saha K, Weber I, Birnbaum ML, De Choudhury M. Characterizing awareness of schizophrenia among facebook users by leveraging facebook advertisement estimates. J Med Internet Res. (2017) 19:e156. doi: 10.2196/jmir.6815
62. Lampropoulos D, Aim MA, Apostolidis T. Rights, identity, neoliberalism: an exploratory and critical analysis of french-speaking schizophrenia pages on facebook. Papers Soc Rep. (2022) 31(1):2–1.
63. Retnowati Y. Communication strategy of ICCS (Indonesian community care for schizophrenia) in giving psycho-education to people with schizophrenia and their families. KnE Soc Sci. (2017) 2:321. doi: 10.18502/kss.v2i4.903
64. Lam NHT, Tsiang JTH, Woo BKP. Exploring the role of youTube in disseminating psychoeducation. Acad Psychiatry. (2017) 41:819–22. doi: 10.1007/s40596-017-0835-9
65. Kumar D, Jha M. YouTube videos on psychosocial interventions for schizophrenia. Psychosis. (2018) 10:220–4. doi: 10.1080/17522439.2018.1497694
66. Nour MM, Nour MH, Tsatalou OM, Barrera A. Schizophrenia on youTube. Psychiatr Serv. (2017) 68:70–4. doi: 10.1176/appi.ps.201500541
67. Godwin HT, Khan M, Yellowlees P. The educational potential of youTube. Acad Psychiatry. (2017) 41:823–7. doi: 10.1007/s40596-017-0809-y
68. Aguiar JP, Gama, Marques J, Leufkens HGM, Alves da Costa F. Healthcare professionals' Views on the management of medication complexities in the elderly with mental health disorders: A cross-sectional study. Front Psychiatry. (2022) 13:885216. doi: 10.3389/fpsyt.2022.885216
69. Saha K, Sugar B, Torous J, Abrahao B, Kıcıman E, De Choudhury MA. Social networks study on the effects of psychiatric medication use. Proc Int AAAI Conf Weblogs Soc Media. (2019) 13:440–51. doi: 10.1609/icwsm.v13i01.3242
70. Woo BKP, Kung EA. YouTube video intervention as) mHealth to promote first-episode psychosis education to Chinese. Asian J Psychiatry. (2018) 33:38–9. doi: 10.1016/j.ajp.2018.02.021
71. Hansen H, Stige SH, Moltu C, Johannessen JO, Joa I, Dybvig S, et al. We all have a responsibility": a narrative discourse analysis of an information campaign targeting help-seeking in first episode psychosis. Int J Ment Health Syst. (2019) 13:32. doi: 10.1186/s13033-019-0289-4
Keywords: disinformation, health literacy, mental health, schizophrenia, social networks
Citation: Suárez-Llevat C, Jiménez-Gómez B, Ruiz-Núñez C, Fernández-Quijano I, Rodriguez-González EM, de la Torre-Domingo C and Herrera-Peco I (2024) Social networks use in the context of Schizophrenia: a review of the literature. Front. Psychiatry 15:1255073. doi: 10.3389/fpsyt.2024.1255073
Received: 10 July 2023; Accepted: 29 April 2024;
Published: 31 May 2024.
Edited by:
Ingrid Melle, University of Oslo, NorwayReviewed by:
Christina Athanasopoulou, University of West Attica, GreeceJusto Emilio Pinzon Espinosa, Fundació Pere Mata Terres de l’Ebre, Spain
Ninni Ihalainen, Turku University Hospital, Finland
Lauri Kuosmanen, University of Eastern Finland, Finland
Copyright © 2024 Suárez-Llevat, Jiménez-Gómez, Ruiz-Núñez, Fernández-Quijano, Rodriguez-González, de la Torre-Domingo and Herrera-Peco. This is an open-access article distributed under the terms of the Creative Commons Attribution License (CC BY). The use, distribution or reproduction in other forums is permitted, provided the original author(s) and the copyright owner(s) are credited and that the original publication in this journal is cited, in accordance with accepted academic practice. No use, distribution or reproduction is permitted which does not comply with these terms.
*Correspondence: Iván Herrera-Peco, aWhlcnJwZWNAdWF4LmVz
†These authors have contributed equally to this work