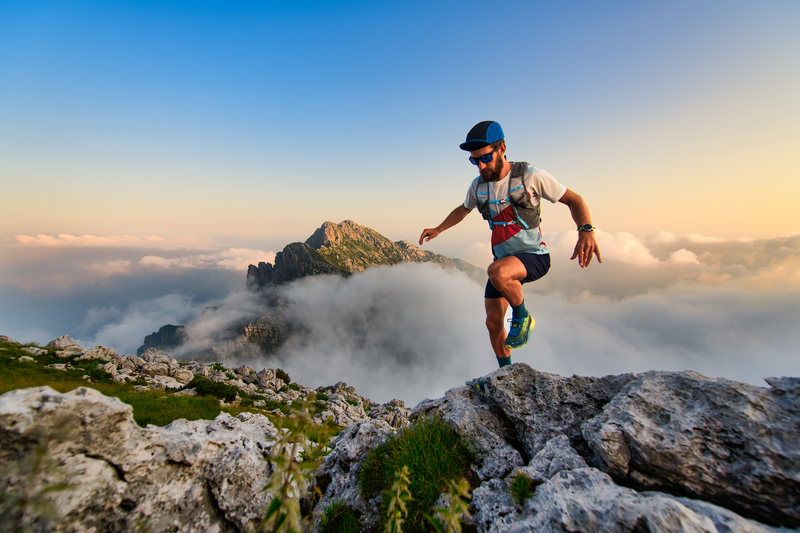
95% of researchers rate our articles as excellent or good
Learn more about the work of our research integrity team to safeguard the quality of each article we publish.
Find out more
EDITORIAL article
Front. Psychiatry , 29 August 2023
Sec. Psychological Therapy and Psychosomatics
Volume 14 - 2023 | https://doi.org/10.3389/fpsyt.2023.1276222
This article is part of the Research Topic Assessments and Measures in Psychotherapy Research: Going Beyond Self-Report Data View all 12 articles
Editorial on the Research Topic
Assessments and measures in psychotherapy research: going beyond self-report data
Good clinical decision-making during case conceptualization, treatment selection, and the adjustment of the therapeutic strategy over the course of treatment can improve the effectiveness of psychotherapy. To support therapists making such decisions, measurement-based and data-informed psychological therapy, which relies on prediction algorithms, can be implemented (1). In recent decades, the statistical methods used to create these algorithms have improved rapidly, e.g., with the introduction of machine learning (ML) into psychotherapy research (2). However, these advanced methods quickly reach their limits when the data base is insufficient to realize their full potential to predict outcomes and derive clinical recommendations. Indeed, psychotherapeutic processes are rich on various channels, including verbal and nonverbal exchanges between patients and therapists, emotional expressions, somatic-motor activity, and physiological processes. These different modalities convey important information about patients' mental states, thoughts, and emotions and can lead to a more in-depth understanding of psychotherapy processes and outcomes. However, most measurement-based prediction algorithms have been calculated based on self-report data. Although they are the cornerstone of psychotherapy research, standardized subjective self-report measures have critical shortcomings, including limited patient self-insight, response tendencies, and cognitive biases (e.g., memory bias) (3, 4). To better support therapists with the most accurate models and recommendations, we must go beyond self-report questionnaires. In the following sections, we will summarize assessments and measures in psychotherapy research that can capture important information about patients and treatment in addition to and beyond self-report questionnaires, thus providing novel data for evidence-based and data-informed psychotherapy.
With advancing technology and digitization, video recordings can provide more objective information about the patient, therapist, and treatment non-invasively and with high temporal resolution. Observation methods can be applied to video recordings to capture verbal information and behavior (e.g., therapeutic interventions) (5), as well as non-verbal cues, such as movement (e.g., movement-based attunement) (6), gestures, and facial expressions. Methodological studies evaluating the validity of these measures are crucial to gain insight into what we are assessing (7). Three papers in the Research Topic examine video- and observer-based methods. Maaß et al. validated a brief rating scale to reliably and applicably assess basic psychotherapeutic communication skills in clinical training. Diaz et al. applied an observational coding system measuring relationship-building behaviors between therapist and clients and modeled affective dynamics within the dyads. Terhürne et al. introduce a video-analysis system for automated emotion recognition and examined associations between system ratings of emotional valence and arousal and self-reports, change processes, and symptoms.
Each video recording also provides one or more audio tracks containing verbal and paraverbal information. Linguistic expressions in a session reveal patient and therapist thoughts and emotions and provide information about the dyadic interaction, which can be extracted and analyzed via natural language processing. Speech content is transcribed either manually by human raters or automatically and thus transferred into text form. These texts can be evaluated by qualitative linguistic analyses or according to predefined categories such as sentiments (8). Paraverbal parameters, e.g., speech rate, speech frequency, and vocal arousal, can be extracted from the audio files to examine intra- and interpersonal emotion dynamics and other therapeutic processes (9, 10). Four papers in this Research Topic analyze verbal or paraverbal features. Broadbent et al. identified clients at risk of suicide using natural language processing on data from a text-based crisis encounter and mobile tipline app. Egozi et al. applied observational measures of attachment and therapeutic distance to transcripts of video-recorded patient and therapist narratives about their therapeutic relationship. Lee et al. examined transcribed session recordings regarding the use of discourse particles, which indicate the formality in language, and its association with observer-rated therapist empathy. Opladen et al. validated fundamental frequency f 0, which is a commonly used index for emotional activation, as a marker of arousal, valence, and distress during a body exposure session.
Considering the close association between psychological and physical processes, biological and physiological variables can offer further useful information. Variables such as heart rate variability (HRV) or electrodermal activity (EDA) allow an objective and continuous recording of stress or emotional arousal during the therapy session. Additionally, patient and therapist co-activate and co-regulate their physiological responses (11). The availability of smartwatches and other wearable devices means that these measurements no longer represent a noticeable intrusion into the therapeutic setting (12). Three papers in this Research Topic rely mainly on data from physiological measures. Andorfer et al. assessed the psychophysiological stress response during a social-evaluative speaking task via HRV, heart rate, and blood pressure to evaluate the effectiveness of a mindfulness-based intervention. Nyman-Salonen et al. provide a narrative review of opportunities and challenges associated with measuring embodied variables in psychotherapy, which focuses on the sympathetic nervous system and body movements. Looking to the future, Hollandt et al. present the protocol of a pilot study planning to examine the dyadic synchrony of heart rate, EDA, and electroencephalogram via wearable devices during two experimental emotion-processing tasks.
The measures discussed above can be collected passively before, during, and after treatment. Patients do not have to do anything other than participate in the regular course of therapy, meaning that these assessments often involve less effort for patients than self-report questionnaires. However, additional information about patients can also be obtained via more active assessment methods such as experimental tasks. Psychological tasks can, for example, include implicit association tests as an indirect measure of pathological attitudes and cognitions or change processes such as outcome expectations (13). In this Research Topic, one paper applied an experimental task. Amano et al. assessed reaction times and the ratio of the number of responses for positive valence in the future thinking task as potential objective measures of treatment process and outcome.
This Research Topic highlights the range of possible assessments and measures in psychotherapy that go beyond self-reports. The 11 studies aimed at validating these measures as well as measuring and predicting treatment processes and outcomes. The next step must involve the multimodal and multimethod assessment of patient and treatment information. Some studies included here have already collected measures from varying channels (e.g., Opladen et al.), and introduce methods to integrate information from different modalities (e.g., Nyman-Salonen et al.). With the advancing digitization and technologization of psychotherapeutic settings, the implementation of these measures into routine care is becoming more feasible. Nevertheless, it requires time, resources, and improved scientific training for practitioners to be able and feel confident making clinical decisions supported by these new sources of data (1).
BS: Conceptualization, Writing—original draft, Writing—review and editing. JU: Writing—review and editing. DA-S: Writing—review and editing.
This work was supported by the German Research Foundation (DFG) under Grant Nos. LU 660/19-1 and LU 660/16-1.
We thank the authors of the 11 papers published in this Research Topic for their valuable contributions and the referees for their rigorous reviews. We also thank Kaitlyn Poster for proofreading the manuscript.
The authors declare that the research was conducted in the absence of any commercial or financial relationships that could be construed as a potential conflict of interest.
All claims expressed in this article are solely those of the authors and do not necessarily represent those of their affiliated organizations, or those of the publisher, the editors and the reviewers. Any product that may be evaluated in this article, or claim that may be made by its manufacturer, is not guaranteed or endorsed by the publisher.
1. Lutz W, Schwartz B, Delgadillo J. Measurement-based and data-informed psychological therapy. Annu Rev Clin Psychol. (2022) 18:71–98. doi: 10.1146/annurev-clinpsy-071720-014821
2. Aafjes-van Doorn K, Kamsteeg C, Bate J, Aafjes, M. A scoping review of machine learning in psychotherapy research. Psychother Res. (2021) 31:92–116. doi: 10.1080/10503307.2020.1808729
3. Kazdin AE. Evidence-based treatment and practice: new opportunities to bridge clinical research and practice, enhance the knowledge base, and improve patient care. Am Psychol. (2008) 63:146–59. doi: 10.110.1037/0003-066X.63.3.146
4. Levine LJ, Safer MA. Sources of bias in memory for emotions. Curr Dir Psychol Sci. (2002) 11:169–73. doi: 10.1111/1467-8721.00193
5. Boyle K, Deisenhofer A-K, Rubel JA, Bennemann B, Weinmann-Lutz B, Lutz W. Assessing treatment integrity in personalized CBT: the inventory of therapeutic interventions and skills. Cogn Behav Therapy. (2020) 49:210–27. doi: 10.1080/16506073.2019.1625945
6. Schwartz B, Rubel JA, Deisenhofer A-K, Lutz W. Movement-based patient-therapist attunement in psychological therapy and its association with early change. Digit Health. (2022) 8:20552076221129098. doi: 10.1177/20552076221129098
7. Schoenherr D, Paulick J, Worrack S, Strauss BM, Rubel JA, Schwartz B, et al. Quantification of nonverbal synchrony using linear time series analysis methods: lack of convergent validity and evidence for facets of synchrony. Behav Res Methods. (2019) 51:361–83. doi: 10.3758/s13428-018-1139-z
8. Tanana M, Dembe A, Soma CS, Atkins D, Imel Z, Srikumar V. Is sentiment in movies the same as sentiment in psychotherapy? Comparisons using a new psychotherapy sentiment database. In: Proceedings of the Third Workshop on Computational Linguistics and Clinical Psychology. San Diego, CA: Association for Computational Linguistics (2016).
9. Nof A, Amir O, Goldstein P, Zilcha-Mano S. What do these sounds tell us about the therapeutic alliance: acoustic markers as predictors of alliance. Clin Psychol Psychother. (2021) 28:807–17. doi: 10.1002/cpp.2534
10. Paz A, Rafaeli E, Bar-Kalifa E, Gilboa-Schectman E, Gannot S, Laufer-Goldshtein B, et al. Intrapersonal and interpersonal vocal affect dynamics during psychotherapy. J Consult Clin Psychol. (2021) 89:227–39. doi: 10.1037/ccp0000623
11. Prinz J, Rafaeli E, Reuter JK, Bar-Kalifa E, Lutz W. Physiological activation and co-activation in an imagery-based treatment for test anxiety. Psychother Res. (2022) 32:238–48. doi: 10.1080/10503307.2021.1918353
12. Hehlmann MI, Schwartz B, Lutz T, Gómez Penedo JM, Rubel JA, Lutz W. The use of digitally assessed stress levels to model change processes in CBT - a feasibility study on seven case examples. Front Psychiatry. (2021) 12:613085. doi: 10.3389/fpsyt.2021.613085
Keywords: data-informed decision-making, video analysis, natural language processing, physiology, observational measures, psychological tasks
Citation: Schwartz B, Uhl J and Atzil-Slonim D (2023) Editorial: Assessments and measures in psychotherapy research: going beyond self-report data. Front. Psychiatry 14:1276222. doi: 10.3389/fpsyt.2023.1276222
Received: 11 August 2023; Accepted: 21 August 2023;
Published: 29 August 2023.
Edited and reviewed by: Veena Kumari, Brunel University London, United Kingdom
Copyright © 2023 Schwartz, Uhl and Atzil-Slonim. This is an open-access article distributed under the terms of the Creative Commons Attribution License (CC BY). The use, distribution or reproduction in other forums is permitted, provided the original author(s) and the copyright owner(s) are credited and that the original publication in this journal is cited, in accordance with accepted academic practice. No use, distribution or reproduction is permitted which does not comply with these terms.
*Correspondence: Brian Schwartz, c2Nod2FydHpiQHVuaS10cmllci5kZQ==
Disclaimer: All claims expressed in this article are solely those of the authors and do not necessarily represent those of their affiliated organizations, or those of the publisher, the editors and the reviewers. Any product that may be evaluated in this article or claim that may be made by its manufacturer is not guaranteed or endorsed by the publisher.
Research integrity at Frontiers
Learn more about the work of our research integrity team to safeguard the quality of each article we publish.