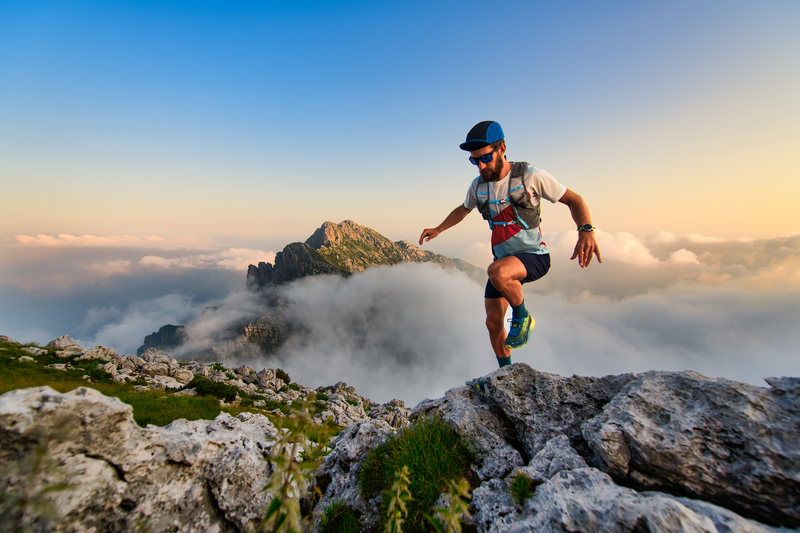
95% of researchers rate our articles as excellent or good
Learn more about the work of our research integrity team to safeguard the quality of each article we publish.
Find out more
ORIGINAL RESEARCH article
Front. Psychiatry , 20 November 2023
Sec. Public Mental Health
Volume 14 - 2023 | https://doi.org/10.3389/fpsyt.2023.1264174
Objectives: Observational studies have demonstrated that household income is associated with morbidity of mental disorders. However, a causal relationship between the two factors remains unclear. Therefore, we investigated the causal relationship between household income status and genetic liability of mental disorders using a bidirectional Mendelian randomization (MR) study.
Methods: This MR study included a large cohort of the European population from publicly available genome-wide association study datasets. A random-effects inverse-variance weighting model was used as the main standard, with MR-Egger regression, weighted median, and maximum likelihood estimations performed concurrently as supplements. Sensitivity analysis, consisting of heterogeneity and horizontal pleiotropy tests, was performed using Cochran’s Q test, MR-Egger intercept, and MR-PRESSO tests to ensure the reliability of the conclusions.
Results: A higher household income tended to be associated with a lower risk of genetic liability for depression (odds ratio [OR]: 0.655, 95% confidence interval [CI] = 0.522–0.822, p < 0.001) and anxiety disorder (OR: 0.666, 95% CI = 0.526–0.843, p < 0.001). No associations were observed for schizophrenia (OR: 0.678, 95% CI = 0.460–1.000, p = 0.05), panic disorder (OR: 0.837, 95% CI = 0.445–1.577, p = 0.583), insomnia (OR: 1.051, 95% CI = 0.556–1.986, p = 0.877), obsessive-compulsive disorder (OR: 1.421, 95% CI = 0.778–2.596, p = 0.252), and bipolar disorder (OR: 1.126, 95% CI = 0.757–1.677, p = 0.556). A reverse MR study showed no reverse causal relationship between psychiatric disorders and household income. Sensitivity analysis verified the reliability of the results.
Conclusion: Our results revealed that the population with a higher household income tended to have a minor risk of genetic liability in depression and anxiety disorders.
Mental disorders encompass a broad spectrum of illnesses, including schizophrenia, anxiety disorders, depressive disorders, and bipolar disorder, and represent a significant public health concern. These disorders are characterized by a combination of abnormal thoughts, perceptions, emotions, behaviors, and interpersonal relationships. According to 155 epidemiological investigations conducted in 59 countries, approximately 17.6% of adults experience a common mental disorder within a 12-month period. Moreover, the aggregated lifetime prevalence estimates from 85 surveys across 39 countries suggest that around 29.2% of individuals have experienced a common mental disorder at some point in their lives (1). Furthermore, mental disorders rank among the top 10 leading causes of global burden (2). The profound impact of these disorders on individuals, families, and societies cannot be overstated.
In the context of the global economic recession, the potential association between declining household income and mental disorders has gained increasing attention. Several observational studies have reported a correlation between household income and mental disorders (3–5). However, such observational studies come with inherent limitations such as a lack of randomization, measurement errors, challenges in controlling variables, and potential for bias (6). To date, limited evidence exists regarding the causal relationship between household income status and mental disorders, particularly owing to the scarcity of large-sample cohort studies. Considering the above, further research is needed to unravel the intricate relationship between household income and mental disorders, facilitating a comprehensive understanding of how household income impacts mental health outcomes. This knowledge will pave the way for the development of evidence-based interventions and policies aimed at mitigating the effects of household income disparities on mental health.
Mendelian randomization (MR) is a statistical method extensively employed in epidemiology and genetics studies to discern causal relationships between exposure factors and outcomes (7, 8). MR is based on Mendel’s law of inheritance, which describes how genetic variants are allocated randomly during meiosis (9). MR utilizes instrumental variables, notably genetic variations such as single nucleotide polymorphisms (SNPs) linked to a risk factor of concern (e.g., household income status), to explore whether the chosen risk factor has a causal impact on the outcome of interest (e.g., mental disorders) (10). In the absence of randomized controlled trials (RCTs), MR studies serve as an alternative strategy for causal inference because genetic variants are subject to random assignment during meiosis mirroring the RCT process. Consequently, MR has advantages over traditional observational studies, minimizing confounding risks and elucidating reverse causality, rendering it a powerful instrument for exploring causality in epidemiological research (11). Furthermore, MR studies have demonstrated their efficacy in probing the causal connections among behavioral exposure, educational attainment, household income circumstances, and a broad spectrum of diseases (12–14).
The primary objective of this study was to investigate the bidirectional causal relationship between genetic predisposition associated with household income status and the occurrence of common mental disorders. This investigation was conducted utilizing an MR approach, leveraging a substantial cohort of individuals from the European population as sourced from publicly accessible genome-wide association study (GWAS) datasets.
To achieve impartial results, an MR study depends on three fundamental assumptions: (1) Relevance—the selected genetic instrumental variables (IVs) are significantly associated with the exposure factor; (2) Independence—the IVs are independent of potential confounders associated with exposure factors and outcomes; and (3) Exclusion restriction—the IVs affect the outcomes only through the exposure factor (15).
We conducted 14 separate MR analyses designed to explore the bidirectional association between annual household income status and seven mental disorders, namely, schizophrenia, depression, anxiety disorder, panic disorder, insomnia, obsessive-compulsive disorder, and bipolar disorder. The forward MR study was performed using a random-effects inverse-variance weighting (IVW) model (16) as the primary standard and three other models [MR-Egger regression (17), weighted median (18), and maximum likelihood (19)] as supplements to evaluate the potential causal relationships between household income status as exposure factor and the seven mental disorders. The reverse MR study, applying the same standard and analysis models as the forward MR study, was performed to evaluate the potential causal relationship between the seven mental disorders as exposure factors and household income status (Figure 1).
Figure 1. Description of the bidirectional MR study. Red represents the forward MR analyses, with household income status as exposure and mental disorders as outcomes. Blue represents the reverse MR analyses, with mental disorders as exposures and household income status as outcome.
The evidential threshold for MR analysis was defined as p < 0.004 (0.05/14) according to the Bonferroni correction method. p < 0.05 was considered significant in the sensitivity analysis. The results are reported as odds ratios (ORs) with corresponding 95% confidence intervals (CIs) and p-values, as well as scatter plots. The R v4.0.3 software, including the “TwoSampleMR” (20) and “MR-PRESSO” (21) packages, was used to process and visualize the study data and findings.
The study was conducted using data from a large sample cohort of the European population obtained from a publicly available GWAS dataset. The genetic information used in this study was extracted from the Integrative Epidemiology Unit GWAS database1 (22), which is a publicly available GWAS summary database. Therefore, the requirement for ethical committee approval was waived. The GWAS summary dataset “average total household income before tax” represented the household income status of 397,751 samples originally from the UK Biobank database. The annual household income was divided into five intervals: <18,000; 18,000–30,999; 31,000–51,999; 52,000–100,000; and >100,000 pounds sterling. We followed the sample size and prioritized timeliness to make the best choices whenever possible. Detailed information on all GWAS datasets is listed in Table 1. GWAS datasets of household income and the seven mental disorders were chosen from different consortiums to decrease potential bias caused by sample overlap. In addition, to minimize racial mismatches, all GWAS datasets involved in this study predominantly included populations of European ancestry.
The IVs were SNPs filtered according to the afore-mentioned three pivotal assumptions of MR studies. First, the SNPs were matched using a genome-wide statistical significance threshold (p < 5 × 10−8). Second, the corresponding linkage disequilibrium was tested to confirm the presence of SNPs in the linkage disequilibrium state as well as the independence of SNPs by trimming them within a 0–10,000-kb window at a threshold of r2 < 0.001. Third, to evaluate the assumption that the IVs affect the outcomes only through the exposure factor, potential phenotypes that may have been relevant to the IVs were investigated by searching the human genotype-phenotype association database (27). Fourth, SNPs identified as IVs were further matched with those in the outcome GWAS dataset to establish genetic associations. The summary SNP-phenotype and SNP-outcome statistics were harmonized to ensure effect size alignment, and palindromic SNPs were excluded. Finally, F-statistics (>10) were used to evaluate the strength of the IVs and avoid the influence of weak instrumental bias (28).
Sensitivity analysis was performed to measure the reliability and stability of the conclusions. The sensitivity analysis consisted of (1) Cochran’s Q test (according to the IVW or MR-Egger regression models); (2) horizontal pleiotropy test using an MR-Egger intercept (29) and MR-PRESSO test (21); and (3) “leave-one-out” test (each SNP was dropped successively, and the IVW analysis was repeated to identify whether any specific SNP drove the estimate of the causal relationship).
The numbers of SNPs ultimately identified as IVs in the different outcome datasets were 42 for obsessive-compulsive disorder; 43 for schizophrenia, depression, anxiety disorder, insomnia, and panic disorder; and 44 for bipolar disorder. The F-statistic scores of all selected SNPs were >10 (obsessive-compulsive disorder: 57.43, schizophrenia: 57.64, depression: 57.77, anxiety disorder: 57.77, insomnia: 57.77, panic disorder: 57.77, and bipolar disorder: 57.49), indicating a low risk of weak instrument bias.
Using the random-effects IVW model results as the primary standard, a higher household income tended to lower the risk of genetic liability in depression (OR: 0.655, 95% CI = 0.522–0.822, p < 0.001). Similarly, the result of the random-effects IVW model suggested a significant difference for anxiety disorder (OR: 0.666, 95% CI = 0.526–0.843, p < 0.001). These findings were supported by the maximum likelihood model. However, no significant differences were reported in the MR-Egger regression and weighted median models. In addition, no associations were observed for schizophrenia (OR: 0.678, 95% CI = 0.460–1.000, p = 0.05), panic disorder (OR: 0.837, 95% CI = 0.445–1.577, p = 0.583), insomnia (OR: 1.051, 95% CI = 0.556–1.986, p = 0.877), obsessive-compulsive disorder (OR: 1.421, 95% CI = 0.778–2.596, p = 0.252), and bipolar disorder (OR: 1.126, 95% CI = 0.757–1.677, p = 0.556). Detailed information is displayed in the forest plot in Figure 2 and illustrated as a scatterplot in Supplementary Figure S1.
Figure 2. Forward MR study results illustrated by forest plot. The causal relationship between household income status and mental disorders, evaluated using an MR study. OR, odds ratio; CI, confidence interval; MR, Mendelian randomization; SNP, single nucleotide polymorphism.
The results of Cochran’s Q test indicated heterogeneity among the IVs for schizophrenia and bipolar disorder (Table 2). However, no heterogeneity was reported for the remaining five mental disorders, especially for depression and anxiety disorder. Meanwhile, we used the random-effects IVW model to minimize the effect of heterogeneity in the MR study. No horizontal pleiotropy was detected using the MR-Egger intercept or MR-PRESSO test (Table 2). In addition, the “leave-one-out” method indicated that no specific SNP among the IVs significantly affected the overall results (Supplementary Figure S2). In general, the sensitivity analysis verified the robustness of the conclusions.
The reverse MR study ultimately identified a total of 205, 13, 1, and 0 SNPs as IVs for schizophrenia; bipolar disorder; depression; and anxiety disorders, panic disorder, obsessive-compulsive disorder, and insomnia, respectively.
Based on the results of the random-effects IVW model and Bonferroni correction standard, the findings of the reverse MR study suggested no reverse causal relationship between the mental disorders and household income.
Various epidemiological studies have reported a strong correlation between economic status and mental disorders at both national and individual levels (30–32). However, the establishment of a causal relationship requires further investigation. To the best of our knowledge, this study represents a pioneering effort to explore the causal impact of household income status on mental disorders using a bidirectional two-sample MR study design. In summary, the MR study findings indicated that individuals with higher household incomes tended to have reduced genetic liability for depression and anxiety disorders, as indicated by the random-effects IVW model and Bonferroni correction standard. However, no significant associations were observed for schizophrenia, panic disorder, insomnia, obsessive-compulsive disorder, or bipolar disorder. Additionally, the reverse MR analysis did not provide substantial evidence to suggest a potential causal effect of mental disorders on household income status.
Consistent with our findings, compelling evidence suggests a correlation between household income and the incidence of depression (33, 34). Few longitudinal studies have investigated the link between household income and depression risk. These investigations have diverged in research methodologies and outcome measures, yielding different findings. Notably, the Canadian National Population Health Survey’s longitudinal analysis identified an elevated likelihood of major depressive episodes among working men aged 35–74 years with low household incomes, in contrast to their peers (35). Conversely, the Stockholm Youth Cohort Study reported an increased risk of depression among adolescents from economically disadvantaged households (36). Results from the Gutenberg longitudinal general study revealed a heightened risk of developing depressive symptoms 2.5 years later in individuals with lower household net incomes who were initially devoid of baseline depression symptoms (37). In contrast to these prior investigations, our study employed MR to mitigate confounding factors. Importantly, our research benefited from a substantially larger sample size, enhancing the reliability of our conclusions.
According to data from the United States Centers for Disease Control and Prevention, 15.8% of adults in families below the federal poverty line experienced depression between 2013 and 2016. By contrast, this proportion decreased to 3.5% in families with incomes above 400% of the federal poverty line (38). Depression results from a multifactorial interplay involving genetic, biochemical, neurobiological, and psychosocial factors in various dimensions (39, 40). Therefore, the relationship between household income and depression is likely to involve interactions among multiple mechanisms. Low household income can result in heightened psychological stress, diminished social support, and other associated challenges (3, 41). Individuals may face higher burdens of chronic stress and social inequality, thereby fostering an environment conducive to elevated levels of chronic inflammatory responses, a recognized risk factor for the manifestation of depression symptoms (42–45). Economic pressure and material deprivation can also result in feelings of low mood, increased self-esteem, and diminished hope (46, 47). Prolonged stress can consistently elevate the activity of stress response systems, such as the hypothalamic-pituitary-adrenal axis (48, 49). These elevations may lead to the abnormal release of adrenergic hormones such as cortisol, thereby increasing the risk of depression (50, 51). Low income may also be associated with GABAergic and serotonergic neurotransmission (52). Depression is linked to abnormalities in the function of these neurotransmitter systems, and low-income individuals may have abnormal activity in these systems, thereby facilitating the development of depression (53, 54). Additionally, children from low-income families may experience more stress and adverse events, leading to changes in gene expression and increased susceptibility to depression (55–58). Financial constraints may cause difficulties for families to meet basic needs such as food, housing, and healthcare. Subsequently, inadequate nutrition and delayed brain development during childhood, stemming from poverty, can further amplify the likelihood of developing depression in adulthood (59). Based on our findings, strategies and interventions to prevent depression can be improved.
Studies on the relationship between household income and anxiety have yielded consistent findings (60, 61). Although previous studies have indicated a potential link between household income and anxiety, they were unable to establish any causality. Our study demonstrated a significant negative causal relationship between household income and anxiety, indicating that individuals with a higher household income exhibited a lower level of anxiety. A previous study reported that participants with an annual household income below $20,000 faced an elevated risk of developing anxiety during the 3-year follow-up compared with those earning $70,000 or more annually (3). The study also found that reduced household income was associated with an increased risk of mental disorders. Lower household income often engenders heightened financial stress, leading to apprehension concerning prospects (62). A higher household income typically provides greater access to healthcare, education, and social support systems. Sufficient access to these resources aids individuals to effectively manage and confront stressors, thereby reducing anxiety levels. Furthermore, a higher household income can enhance educational opportunities and bolster employment prospects and financial security (41, 63). Conversely, limited access to education and employment opportunities stemming from low household income could engender anxiety regarding the future. Families with low household incomes reside in disadvantaged conditions, rendering them more susceptible to environmental pollution, extreme temperatures, and challenging sleep environments (64). Moreover, women and children within impoverished households are at heightened risks of experiencing traumatic events and enduring violence perpetrated by other family members (65, 66). The cumulative impact of these factors increases their vulnerability to anxiety disorders.
Similar to other illnesses, depression and anxiety can potentially lead to economic consequences, resulting in direct or indirect declines in individual income (34). However, our study, which explored bidirectional causal relationships, did not observe unidirectional causal relationships between depression, anxiety, and household income. Specifically, our findings suggested that depression and anxiety may not significantly reduce household income. Currently, there is a lack of research investigating the impact of depression and anxiety on household income. Although depression and anxiety may directly affect individual income, their effects on household income may not necessarily manifest if other household members do not experience anxiety or depression. As households often include multiple earners or diverse income sources, the presence of other earners can mitigate the impact of depression and anxiety on household income. These findings imply that providing financial support to individuals living alone may be more beneficial than for those living with others.
The bidirectional MR study design has the substantial advantage of effectively avoiding the impact of reverse causality and reducing residual confounding factors. Nevertheless, several limitations inherent in this study should be recognized. First, the GWAS dataset used was primarily drawn from populations of European descent to avoid confounding factors due to population stratification. Consequently, the current findings may not be generalizable to other ethnic groups, and additional research is necessary to understand how these outcomes apply to diverse populations. Second, the biological functions of SNPs as IVs and how they aggravate depression and anxiety remain unclear and require further investigation. Finally, the current MR study did not include sub-group analysis for individuals at high risk of depression and anxiety. Incorporating such an analysis could have enhanced the study’s practical utility by offering a more comprehensive understanding of how household income affects diverse population subgroups. This, in turn, would have facilitated the development of more targeted policies and support measures. Therefore, the results of the present study should be interpreted with caution.
This study utilized extensive datasets comprising millions of individual samples and a bidirectional MR design to investigate the causal relationship between household income status and mental disorders. The results revealed that individuals from high-income households may have a decreased genetic liability for depression and anxiety. These findings underscore the importance of incorporating household income disparities into medical reimbursement policies and prioritizing efforts to improve equitable access to and availability of medical services for individuals residing in low-income households.
The original contributions presented in the study are included in the article/Supplementary material, further inquiries can be directed to the corresponding author.
The genetic information used in this study was extracted from the Integrative Epidemiology Unit GWAS database (https://gwas.mrcieu.ac.uk/), which is a publicly available GWAS summary database. Therefore, the requirement for ethical committee approval was waived.
GL: Conceptualization, Writing – original draft. WL: Investigation, Methodology, Writing – original draft. XZ: Methodology, Software, Writing – review & editing. JL: Project administration, Supervision, Writing – review & editing.
The author(s) declare that no financial support was received for the research, authorship, and/or publication of this article.
We would like to acknowledge the participants and investigators of the IEU OpenGWAS project, the UK Biological Bank and the FinnGen studies for their contributions, and share for scientific research.
The authors declare that the research was conducted in the absence of any commercial or financial relationships that could be construed as a potential conflict of interest.
All claims expressed in this article are solely those of the authors and do not necessarily represent those of their affiliated organizations, or those of the publisher, the editors and the reviewers. Any product that may be evaluated in this article, or claim that may be made by its manufacturer, is not guaranteed or endorsed by the publisher.
The Supplementary material for this article can be found online at: https://www.frontiersin.org/articles/10.3389/fpsyt.2023.1264174/full#supplementary-material
MR, Mendelian randomization; SNP, Single nucleotide polymorphism; GWAS, Genome-wide association study; IV, Instrumental variable; IVW, Inverse-variance weighting; OR, Odds ratio; CI, Confidence interval.
1. Steel, Z, Marnane, C, Iranpour, C, Chey, T, Jackson, JW, Patel, V, et al. The global prevalence of common mental disorders: a systematic review and meta-analysis 1980-2013. Int J Epidemiol. (2014) 43:476–93. doi: 10.1093/ije/dyu038
2. Collaborators, GMD. Global, regional, and national burden of 12 mental disorders in 204 countries and territories, 1990–2019: a systematic analysis for the global burden of disease study 2019. Lancet Psychiatry. (2022) 9:137–50. doi: 10.1016/S2215-0366(21)00395-3
3. Sareen, J, Afifi TO, McMillan, KA, and Asmundson, GJ. Relationship between household income and mental disorders: findings from a population-based longitudinal study. Arch Gen Psychiatry. (2011) 68:419–27. doi: 10.1001/archgenpsychiatry.2011.15
4. Frasquilho, D, Matos, MG, Salonna, F, Guerreiro, D, Storti, CC, Gaspar, T, et al. Mental health outcomes in times of economic recession: a systematic literature review. BMC Public Health. (2016) 16:115. doi: 10.1186/s12889-016-2720-y
5. Phongsavan, P, Chey, T, Bauman, A, Brooks, R, and Silove, D. Social capital, socio-economic status and psychological distress among Australian adults. Soc Sci Med. (2006) 63:2546–61. doi: 10.1016/j.socscimed.2006.06.021
6. Grimes, DA, and Schulz, KF. Bias and causal associations in observational research. Lancet. (2002) 359:248–52. doi: 10.1016/S0140-6736(02)07451-2
7. Sanderson, E, Richardson, TG, Morris, TT, Tilling, K, and Davey, SG. Estimation of causal effects of a time-varying exposure at multiple time points through multivariable mendelian randomization. PLoS Genet. (2022) 18:e1010290. doi: 10.1371/journal.pgen.1010290
8. Lawlor, DA, Harbord, RM, Sterne, JA, Timpson, N, and Davey, SG. Mendelian randomization: using genes as instruments for making causal inferences in epidemiology. Stat Med. (2008) 27:1133–63. doi: 10.1002/sim.3034
9. Emdin, CA, Khera, AV, and Kathiresan, S. Mendelian Randomization. JAMA. (2017) 318:1925–6. doi: 10.1001/jama.2017.17219
10. Bowden, J, and Holmes, MV. Meta-analysis and Mendelian randomization: a review. Res Synth Methods. (2019) 10:486–96. doi: 10.1002/jrsm.1346
11. Lee, K, and Lim, CY. Mendelian randomization analysis in observational epidemiology. J Lipid Atheroscler. (2019) 8:67–77. doi: 10.12997/jla.2019.8.2.67
12. Chen, X, Hong, X, Gao, W, Luo, S, Cai, J, Liu, G, et al. Causal relationship between physical activity, leisure sedentary behaviors and COVID-19 risk: a Mendelian randomization study. J Transl Med. (2022) 20:1–12. doi: 10.1186/s12967-022-03407-6
13. Böckerman, P, Viinikainen, J, Pulkki-Råback, L, Hakulinen, C, Pitkänen, N, Lehtimäki, T, et al. Does higher education protect against obesity? Evidence using Mendelian randomization. Prev Med. (2017) 101:195–8. doi: 10.1016/j.ypmed.2017.06.015
14. Zheng, X, Yang, Y, Chen, J, and Lu, B. Dissecting the causal relationship between household income status and genetic susceptibility to cardiovascular-related diseases: insights from bidirectional mendelian randomization study. BMC Public Health. (2023) 23:749. doi: 10.1186/s12889-023-15561-7
15. Davies, NM, Holmes, MV, and Davey, SG. Reading Mendelian randomisation studies: a guide, glossary, and checklist for clinicians. BMJ. (2018) 362:k601. doi: 10.1136/bmj.k601
16. Burgess, S, Dudbridge, F, and Thompson, SG. Combining information on multiple instrumental variables in Mendelian randomization: comparison of allele score and summarized data methods. Stat Med. (2016) 35:1880–906. doi: 10.1002/sim.6835
17. Bowden, J, Davey Smith, G, and Burgess, S. Mendelian randomization with invalid instruments: effect estimation and bias detection through egger regression. Int J Epidemiol. (2015) 44:512–25. doi: 10.1093/ije/dyv080
18. Bowden, J, Davey Smith, G, Haycock, PC, and Burgess, S. Consistent estimation in Mendelian randomization with some invalid instruments using a weighted median estimator. Genet Epidemiol. (2016) 40:304–14. doi: 10.1002/gepi.21965
19. Nguyen, LT, Schmidt, HA, von Haeseler, A, and Minh, BQ. IQ-TREE: a fast and effective stochastic algorithm for estimating maximum-likelihood phylogenies. Mol Biol Evol. (2015) 32:268–74. doi: 10.1093/molbev/msu300
20. Hemani, G, Zheng, J, Elsworth, B, Wade, KH, Haberland, V, Baird, D, et al. The MR-base platform supports systematic causal inference across the human phenome. elife. (2018) 7:e34408. doi: 10.7554/eLife.34408
21. Verbanck, M, Chen, C-Y, Neale, B, and Do, R. Detection of widespread horizontal pleiotropy in causal relationships inferred from Mendelian randomization between complex traits and diseases. Nat Genet. (2018) 50:693–8. doi: 10.1038/s41588-018-0099-7
22. Elsworth, B, Lyon, M, Alexander, T, Liu, Y, Matthews, P, Hallett, J, et al. The MRC IEU open GWAS data infrastructure. bio Rxiv. (2020). doi: 10.1101/2020.08.10.244293
23. Bycroft, C, Freeman, C, Petkova, D, Band, G, Elliott, LT, Sharp, K, et al. The UK biobank resource with deep phenotyping and genomic data. Nature. (2018) 562:203–9. doi: 10.1038/s41586-018-0579-z
24. Trubetskoy, V, Pardiñas, AF, and Qi, T. Mapping genomic loci implicates genes and synaptic biology in schizophrenia. Nature. (2022) 604:502–8.
25. Kurki, MI, Karjalainen, J, Palta, P, Sipilä, TP, Kristiansson, K, Donner, K, et al. Finn gen: unique genetic insights from combining isolated population and national health register data. Med Rxiv. (2022). doi: 10.1101/2022.03.03.22271360
26. Stahl, EA, Breen, G, Forstner, AJ, McQuillin, A, Ripke, S, Trubetskoy, V, et al. Genome-wide association study identifies 30 loci associated with bipolar disorder. Nat Genet. (2019) 51:793–803. doi: 10.1038/s41588-019-0397-8
27. Yuan, S, Carter, P, Bruzelius, M, Vithayathil, M, Kar, S, Mason, AM, et al. Effects of tumour necrosis factor on cardiovascular disease and cancer: a two-sample Mendelian randomization study. EBioMedicine. (2020) 59:102956. doi: 10.1016/j.ebiom.2020.102956
28. Wu, F, Huang, Y, Hu, J, and Shao, Z. Mendelian randomization study of inflammatory bowel disease and bone mineral density. BMC Med. (2020) 18:312. doi: 10.1186/s12916-020-01778-5
29. Burgess, S, and Thompson, SG. Interpreting findings from Mendelian randomization using the MR-egger method. Eur J Epidemiol. (2017) 32:377–89. doi: 10.1007/s10654-017-0255-x
30. Jadnanansing, R, de Beurs, E, Etwaroo, K, Blankers, M, Dwarkasing, R, Peen, J, et al. A survey of depression and anxiety disorders in urban and rural Suriname. BMC Public Health. (2022) 22:51. doi: 10.1186/s12889-021-12454-5
31. Jenkins, R, Bhugra, D, Bebbington, P, Brugha, T, Farrell, M, Coid, J, et al. Debt, income and mental disorder in the general population. Psychol Med. (2008) 38:1485–93. doi: 10.1017/S0033291707002516
32. Lang, IA, Llewellyn, DJ, Hubbard, RE, Langa, KM, and Melzer, D. Income and the midlife peak in common mental disorder prevalence. Psychol Med. (2011) 41:1365–72. doi: 10.1017/S0033291710002060
33. Akhtar-Danesh, N, and Landeen, J. Relation between depression and sociodemographic factors. Int J Ment Health Syst. (2007) 1:4. doi: 10.1186/1752-4458-1-4
34. Ridley, M, Rao, G, Schilbach, F, and Patel, V. Poverty, depression, and anxiety: causal evidence and mechanisms. Science. (2020) 370:eaay0214. doi: 10.1126/science.aay0214
35. Wang, JL, Schmitz, N, and Dewa, CS. Socioeconomic status and the risk of major depression: the Canadian National Population Health Survey. J Epidemiol Community Health. (2010) 64:447–52. doi: 10.1136/jech.2009.090910
36. Wirback, T, Möller, J, Larsson, JO, Galanti, MR, and Engström, K. Social factors in childhood and risk of depressive symptoms among adolescents - a longitudinal study in Stockholm, Sweden. Int J Equity Health. (2014) 13:96. doi: 10.1186/s12939-014-0096-0
37. Schlax, J, Jünger, C, Beutel, ME, Münzel, T, Pfeiffer, N, Wild, P, et al. Income and education predict elevated depressive symptoms in the general population: results from the Gutenberg health study. BMC Public Health. (2019) 19:430. doi: 10.1186/s12889-019-6730-4
38. Brody, DJ, Pratt, LA, and Hughes, JP. Prevalence of depression among adults aged 20 and over: United States, 2013–2016. NCHS Data Brief. (2018):1–8.
39. Remes, O, Mendes, JF, and Templeton, P. Biological, psychological, and social determinants of depression: a review of recent literature. Brain Sci. (2021) 11:11. doi: 10.3390/brainsci11121633
40. Kendall, KM, Van Assche, E, Andlauer, TFM, Choi, KW, Luykx, JJ, Schulte, EC, et al. The genetic basis of major depression. Psychol Med. (2021) 51:2217–30. doi: 10.1017/S0033291721000441
41. Cooper, K, and Stewart, K. Does household income affect children’s outcomes? A systematic review of the evidence. Child Indic Res. (2020) 14:981–1005. doi: 10.1007/s12187-020-09782-0
42. Patel, V, Burns, JK, Dhingra, M, Tarver, L, Kohrt, BA, and Lund, C. Income inequality and depression: a systematic review and meta-analysis of the association and a scoping review of mechanisms. World Psychiatry. (2018) 17:76–89. doi: 10.1002/wps.20492
43. Baum, A, Garofalo, J, and Yali, AM. Socioeconomic status and chronic stress: does stress account for SES effects on health? Ann N Y Acad Sci. (1999) 896:131–44. doi: 10.1111/j.1749-6632.1999.tb08111.x
44. Nowakowski, AC, and Sumerau, JE. Swell foundations: fundamental social causes and chronic inflammation. Sociol Spectr. (2015) 35:161–78. doi: 10.1080/02732173.2014.1000554
45. Berk, M, Williams, LJ, Jacka, FN, O’Neil, A, Pasco, JA, Moylan, S, et al. So depression is an inflammatory disease, but where does the inflammation come from? BMC Med. (2013) 11:1–16. doi: 10.1186/1741-7015-11-200
46. Davis, CG, and Mantler, J. The consequences of financial stress for individuals, families, and society. Ottawa: Centre for Research on Stress, Coping and Well-being Carleton University (2004).
47. Hatcher, J, Rayens, MK, Peden, AR, and Hall, LA. Predictors of depression for low-income African American single mothers. J Health Disparit Res Pract. (2008) 2:6.
48. Leistner, C, and Menke, A. Hypothalamic-pituitary-adrenal axis and stress. Handb Clin Neurol. (2020) 175:55–64.
49. Dunlavey, CJ. Introduction to the hypothalamic-pituitary-adrenal Axis: healthy and dysregulated stress responses, developmental stress and neurodegeneration. J Undergrad Neurosci Educ. (2018) 16:R59–r60.
50. Nandam, LS, Brazel, M, Zhou, M, and Jhaveri, DJ. Cortisol and major depressive disorder-translating findings from humans to animal models and Back. Front Psych. (2019) 10:974. doi: 10.3389/fpsyt.2019.00974
51. Dienes, KA, Hazel, NA, and Hammen, CL. Cortisol secretion in depressed, and at-risk adults. Psychoneuroendocrinology. (2013) 38:927–40. doi: 10.1016/j.psyneuen.2012.09.019
52. Hill, WD, Davies, NM, Ritchie, SJ, Skene, NG, Bryois, J, Bell, S, et al. Genome-wide analysis identifies molecular systems and 149 genetic loci associated with income. Nat Commun. (2019) 10:5741. doi: 10.1038/s41467-019-13585-5
53. Werner, FM, and Covenas, R. Classical neurotransmitters and neuropeptides involved in major depression: a review. Int J Neurosci. (2010) 120:455–70. doi: 10.3109/00207454.2010.483651
54. Pan, JX, Xia, JJ, Deng, FL, Liang, WW, Wu, J, Yin, BM, et al. Diagnosis of major depressive disorder based on changes in multiple plasma neurotransmitters: a targeted metabolomics study. Transl Psychiatry. (2018) 8:130. doi: 10.1038/s41398-018-0183-x
55. Parker, N, Wong, AP, Leonard, G, Perron, M, Pike, B, Richer, L, et al. Income inequality, gene expression, and brain maturation during adolescence. Sci Rep. (2017) 7:7397. doi: 10.1038/s41598-017-07735-2
56. Avanci, J, Assis, S, Oliveira, R, and Pires, T. Childhood depression: Exploring the association between family violence and other psychosocial factors in low-income Brazilian schoolchildren. Child Adolesc Psychiatry Ment Health. (2012) 6:1–9. doi: 10.1186/1753-2000-6-26
57. Weder, N, Zhang, H, Jensen, K, Yang, BZ, Simen, A, Jackowski, A, et al. Child abuse, depression, and methylation in genes involved with stress, neural plasticity, and brain circuitry. J Am Acad Child Adolesc Psychiatry. (2014) 53:417–24e5. doi: 10.1016/j.jaac.2013.12.025
58. Kaufman, J, Wymbs, NF, Montalvo-Ortiz, JL, Orr, C, Albaugh, MD, Althoff, R, et al. Methylation in OTX2 and related genes, maltreatment, and depression in children. Neuropsychopharmacology. (2018) 43:2204–11. doi: 10.1038/s41386-018-0157-y
59. Blair, C, and Raver, CC. Poverty, stress, and brain development: new directions for prevention and intervention. Acad Pediatr. (2016) 16:S30–6. doi: 10.1016/j.acap.2016.01.010
60. Huato, J, and Chavez, A. Household income, pandemic-related income loss, and the probability of anxiety and depression. East Econ J. (2021) 47:546–70. doi: 10.1057/s41302-021-00199-3
61. Vine, M, Vander Stoep, A, Bell, J, Rhew, IC, Gudmundsen, G, and McCauley, E. Associations between household and neighborhood income and anxiety symptoms in young adolescents. Depress Anxiety. (2012) 29:824–32. doi: 10.1002/da.21948
62. Roll, SP, Taylor, SH, and Grinstein-Weiss, M. Financial anxiety in low-and moderate-income households: Findings from the household financial survey. (2016).
63. Wolla, SA, and Sullivan, J. Education, income, and wealth. Page One Economics® (2017). Available at: https://research.stlouisfed.org/publications/page1-econ/2017/01/03/education-income-and-wealth
64. Bjelland, I, Krokstad, S, Mykletun, A, Dahl, AA, Tell, GS, and Tambs, K. Does a higher educational level protect against anxiety and depression? The HUNT study. Soc Sci Med. (1982) 66:1334–45. doi: 10.1016/j.socscimed.2007.12.019
65. Haushofer, J, Ringdal, C, Shapiro, JP, and Wang, XY. Income changes and intimate partner violence: Evidence from unconditional cash transfers in Kenya. National Bureau of Economic Research (2019). Available at: https://www.nber.org/papers/w25627
Keywords: household income status, causal relationship, Mendelian randomization study, depression, anxiety disorder
Citation: Liu G, Liu W, Zheng X and Li J (2023) The higher the household income, the lower the possibility of depression and anxiety disorder: evidence from a bidirectional Mendelian randomization study. Front. Psychiatry. 14:1264174. doi: 10.3389/fpsyt.2023.1264174
Received: 24 July 2023; Accepted: 14 September 2023;
Published: 20 November 2023.
Edited by:
Shen Liu, Anhui Agricultural University, ChinaCopyright © 2023 Liu, Liu, Zheng and Li. This is an open-access article distributed under the terms of the Creative Commons Attribution License (CC BY). The use, distribution or reproduction in other forums is permitted, provided the original author(s) and the copyright owner(s) are credited and that the original publication in this journal is cited, in accordance with accepted academic practice. No use, distribution or reproduction is permitted which does not comply with these terms.
*Correspondence: Junyan Li, anlsZWUxOTgwQDE2My5jb20=
Disclaimer: All claims expressed in this article are solely those of the authors and do not necessarily represent those of their affiliated organizations, or those of the publisher, the editors and the reviewers. Any product that may be evaluated in this article or claim that may be made by its manufacturer is not guaranteed or endorsed by the publisher.
Research integrity at Frontiers
Learn more about the work of our research integrity team to safeguard the quality of each article we publish.